Abstract
Background
Malaria transmission, primarily mediated by Anopheles gambiae, persists in Dar es Salaam (DSM) despite high coverage with bed nets, mosquito-proofed housing and larviciding. New or improved vector control strategies are required to eliminate malaria from DSM, but these will only succeed if they are delivered to the minority of locations where residual transmission actually persists. Hotspots of spatially clustered locations with elevated malaria infection prevalence or vector densities were, therefore, mapped across the city in an attempt to provide a basis for targeting supplementary interventions.
Methods
Two phases of a city-wide population-weighted random sample of cross-sectional household surveys of malaria infections were complemented by two matching phases of geographically overlapping, high-resolution, longitudinal vector density surveys; spanning 2010–2013. Spatial autocorrelations were explored using Moran’s I and hotspots were detected using flexible spatial scan statistics.
Results
Seven hotspots of spatially clustered elevated vector density and eight of malaria infection prevalence were detected over both phases. Only a third of vectors were collected in hotspots in phase 1 (30 %) and phase 2 (33 %). Malaria prevalence hotspots accounted for only half of malaria infections detected in phase 1 (55 %) and phase 2 (47 %). Three quarters (76 % in phase 1 and 74 % in phase 2) of survey locations with detectable vector populations were outside of hotspots. Similarly, more than half of locations with higher infection prevalence (>10 %) occurred outside of hotspots (51 % in phase 1 and 54 % in phase 2). Vector proliferation hazard (exposure to An. gambiae) and malaria infection risk were only very loosely associated with each other (Odds ratio (OR) [95 % Confidence Interval (CI)] = 1.56 [0.89, 1.78], P = 0.52)).
Conclusion
Many small, scattered loci of local malaria transmission were haphazardly scattered across the city, so interventions targeting only currently identifiable spatially aggregated hotspots will have limited impact. Routine, spatially comprehensive, longitudinal entomological and parasitological surveillance systems, with sufficient sensitivity and spatial resolution to detect these scattered loci, are required to eliminate transmission from this typical African city. Intervention packages targeted to both loci and hotspots of transmission will need to suppress local vector proliferation, treat infected residents and provide vulnerable residents with supplementary protective measures against exposure.
Similar content being viewed by others
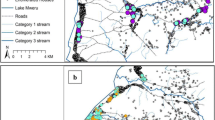
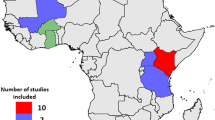
Background
In the city of Dar es Salaam, considerable progress has recently been made to ensure high coverage of standard interventions like rapid diagnostic tests (RDTs), artemisinin-based combination therapy (ACT), long-lasting insecticidal nets (LLINs) and other supplementary vector control measures, such as mosquito-proofed housing and regular application of microbial larvicides [1–5]. Nevertheless, stable residual malaria transmission persists (Msellemu et al. pers.comm. and [6]), so new or improved strategies for controlling malaria vectors [7–9] and parasites [8, 10] will be required to achieve elimination. However, even the most effective intervention measures can only be effective if they are delivered to locations where residual transmission persists [11]. Here the study describes how hotspots of spatially clustered locations with elevated malaria infection prevalence and vector densities were mapped across the city of Dar es Salaam, in an attempt to provide a basis for targeting supplementary interventions.
Methods
Study area
The study was conducted in the urban and peri-urban area of Dar es Salaam city. The city is Tanzania’s commercial and economic capital located on the shores of the Indian Ocean (Fig. 1). Dar es Salaam has 4.4 million inhabitants [12] and is amongst the world’s ten fastest growing cities [13] with an annual growth rate of 5.6 % [12]. However this growth is unplanned, resulting in about 70 % of residents living in informal settlements [14]. At the time of this study, Dar es Salaam had a mean human infection prevalence of approximately 10 %, arising from modest levels of transmission intensity, mediated by remarkably low vector densities, predominantly Anopheles gambiae sensu stricto [5, 15]. Administratively, the city comprises three municipalities namely Ilala, Kinondoni and Temeke and it is divided into 90 wards [12] (Fig. 1). The study area comprised 71 out of these 90 wards, covering 498.2 km2 of the city with approximately 3.6 million residents. Of the study wards, 15 were in phase I and all 71 wards were in phase II. The initial 15 were central wards to which larviciding was originally implemented under the Urban Malaria Control Programme (UMCP) [16] and comprehensively scaled up by the preceding operational research programme in new 56 wards [17]. In total the study covered the whole of urban and peri-urban area of Dar es Salaam city (Fig. 1). Wards are further divided into smaller neighbourhood units called mitaa (a Kiswahili word for street, written in the singular form as mtaa) [18]. Each mtaa is subdivided into ten cell units (TCUs), comprising clusters of approximately 10–20 houses, although some TCUs contain a much larger number of houses [17].
With the exception of a break in activities while the programme was restructured between October and December 2010, Bacillus thuringiensis var. israelensis (Bti) was applied over the full course of the study, to the same 15 wards previously assessed during the operational research phase of the UMCP between 2004 and 2008, and this preventative service was scaled up to cover an additional 56 adjacent wards in January 2012 (Fig. 2). A coated granule formulation was used up to July 2011 (VectoBac®, Valent BioSciences Corporation), following which a pre-diluted aqueous suspension formulation (Bactivec®, Labiofam®) was used instead.
Data collection
This study is a secondary analysis of two distinct phases of parasitological and entomological survey data (Figs. 1, 2), the collection of which is described in detail elsewhere (Msellemu et al. pers. commun). Household cross sectional and longitudinal surveys were subsequently used to collect parasitological and entomological data respectively. While two different sampling frames were used for surveying adult mosquitoes and the infection prevalence, the latter was systematically overlaid upon the former in the first phase and the former was overlaid on the latter in the second phase of surveys because mosquito surveillance sites were reduced when fund for continuously monitoring mosquito vector densities ran out [19]. Infection with blood-stage Plasmodium falciparum parasites was tested for using RDTs (MAL-Pf®, ICT Diagnostics, Cape Town, South Africa).
The total number of households in each TCU within the mapped study area [17] was enumerated by census between January 2008 and May 2010. While awaiting completion of this enumeration of all the households in the study area, for selection of a population-weighted representative sample, a first phase of purposively-sampled household surveys were conducted. This first round of household parasitological surveys was carried out from March 2010 to September 2010; consisting of 291 housing compounds (median = 7 households), 156 of which were subjected to routine collection of adult mosquitoes (Msellemu et al. pers. commun), so that this novel system [19] for monitoring vector densities at high spatial resolution could be evaluated in terms of its epidemiological predictive power.
Following completion of the household enumeration, a second phase of household surveys was begun, which sampled much smaller numbers of larger population clusters, selected randomly as index TCUs. The TCUs were weighted according to their population size and selected using a weighted sampling strategy (Msellemu et al. pers. commun). Between Oct 2010 and May 2012, a second phase of surveys was completed which encompassed a total of 86 clusters centred on index TCUs selected at random in proportion to their estimated population size (median = 392 households). Where the TCU had less than 20 consenting household heads, a neighbouring TCU was chosen at random from where the remaining number of households required to complete the sampling cluster were selected and recruited in exactly the same way. In this phase 30 of the 86 index TCUs were subjected to routine collection of adult mosquitoes. Selection of these co-surveyed areas relied upon willingness of the index TCU leaders to participate in the follow up study to monitor mosquito vector densities and also in TCUs where the environment were supportive for placement of ITT [20].
Anopheles mosquitoes were correspondingly collected in two different phases. The first phase lasted from March to October 2010 and consisted of 615 different sampling locations. These locations were set up within the previously studied 15 wards of the Dar es Salaam UMCP [17], which was designed to establish and evaluate systems for routine larvicide application at programmatic scales [2, 5, 16]. Following a break in funding, the second phase of entomological surveys was initiated in May 2011 and sustained through January 2013, with far greater scale-up to span a total of 1398 locations across the entire study area. The collections of adult mosquitoes were routinely conducted at each location on an approximately monthly basis, using Ifakara Tent Traps (ITT) [20] applied through a community-based system as described in detail elsewhere [19].
Throughout the study period, only modest levels of physiological resistance to pyrethroids, and behavioural resistance or resilience [15] to indoor vector control measures by biting at dawn or dusk, were observed for the An. gambiae complex (Chaki et al. pers.comm.). Laboratory analysis of morphologically-identified specimens of An. gambiae sensu lato, by polymerase chain reaction [21], confirmed that this complex was predominantly composed of the nominate sibling species An. gambiae s.s. [86 % (328/382)], with the small remainder being Anopheles arabiensis [10 % (40/382)]. Anopheles gambiae s.s. is the most anthropophagic and efficient sibling species from this complex, and very few Anopheles funestus [3 % (4/382)] or other Anopheles [1 % (10/382)] were caught. Vector density was therefore expressed in terms of total numbers of An. gambiae complex specimens caught per trap per night per TCU for subsequent analyses, and considered representative of this dominant, nominate sibling species.
The spatial references for both mosquitoes and cross-sectional sampling locations were obtained using handheld global positioning systems (GPS) at an accuracy of 5 m. These were the basis for calculating the centroids of the sampling clusters. The TCU boundaries were mapped using a participatory mapping approach [17, 18].
Statistical analysis
Statistical analyses and data processing were performed using STATA (version 10; Statacorp). ArcGIS software (version 10; ESRI) was used to create spatial adjacency matrices, produce maps and perform exploratory spatial data analyses. FleXScan (version 3.1.2), an open source software [22] was used for the detection of spatial clusters (hotspots) in malaria infection prevalence and mosquito vector densities.
The global Moran’s I statistics (MI) [23] were employed to test for any prevailing spatial autocorrelations in mosquito vector densities and malaria infection prevalence. Spatial autocorrelation occurs when there is clustering (+ve Z-score) or dispersion (−ve Z-score) and this is significant at p ≤ 0.05 [23]. A p value > 0.05 suggests a homogeneous spatial pattern (random).
Local spatial clustering (hotspots) in mosquito densities and malaria infection prevalence were detected using the flexible spatial scan statistic (FleXScan) developed by Tango and Takahashi [24]. Flexible scan statistics create both circular and irregular shaped clusters. Since Dar es Salaam is a low transmission area, and these typical settings tend to restrict high transmission to patchier spatial scales, it was preferred to use flexible scan clustering method to enable detection of actual noncircular clusters even at highly focalized spatial scales [25]. This method identifies clusters based on a spatial weight matrix in which the detected cluster is allowed to be flexible in shape while at the same time confined within relatively small neighbourhoods of each sampling unit [25]. In developing the matrix file, spatial relationships were conceptualized based on Delaunay Triangulation, with Euclidian distance. Similar to Kulldorff’s spatial scan statistics [26], the method uses a circular moving and systematically scanning window, in which not only the whole area inside the window can be considered as a potential cluster, but also spatially connected areas inside the window, which makes it possible to detect irregular-shaped clusters [24]. The analysis parameters were set to purely spatial analysis, scanning for clusters with high rates. Hotspots were defined as spatially aggregated TCUs with significantly higher than average levels of malaria infection prevalence or An. gambiae mosquito densities [11]. The median values of malaria infection prevalence i.e. 12.05 % for phase 1 and 8.49 % for phase 2 were used as cut-off values in stratifying the prevalence data at TCU level, to allow analysis based on a binary logistic variable, assuming a binomial distribution for this outcome, with prevalence above the median in each phase coded as 1 and below the median coded as 0. The likelihood of clusters in mosquito densities was calculated assuming a Poisson distribution. The numerator was aggregated counts of adult female An. gambiae mosquitoes per TCU and the denominator was expected population of An. gambiae mosquitoes per TCU. Clusters of malaria infection prevalence were detected using a binomial statistical model. Statistical significance for the identification of both primary and secondary clusters was set at p < 0.05, which was explored by means of Monte Carlo replication of data sets under the null hypothesis with at least 9999 replications to ensure adequate power for defining those clusters [27, 28]. The relative risk (RR) which is the standardized risk ratio of observed mean catches of An. gambiae or prevalent infections over the expected mean or prevalent infections (“expected number of cases within the cluster when the null hypothesis is true, that is, when the risk is the same inside and outside the cluster”) was also presented (Table 2).
Ethical considerations
Only participants giving informed consent were included in the study, including the mosquito catchers and the house owners where the catches took place, as well as the participants in the epidemiological cross-sectional parasite surveys. Participants found to be infected with malaria parasites during the surveys were treated with artemether-lumefantrine (Coartem®; Novartis Pharma AG, Basel, Switzerland) following national treatment policies and guidelines. Ethical approval for all procedures implanted in this study was obtained from the Institutional Review Board of the Ifakara Health Institute (Approval A.50), the Medical Research Coordination Committee of the Tanzanian National Institute of Medical Research (Approval NIMR/HQ/R.8a/Vol.IX/801), the Research Ethics Committee of the Liverpool School of Tropical Medicine (09.60) and the Human Research Ethics Committee (HSEC) at University of the Witwatersrand (M120835).
Results
A total of 12,170 person nights (function of number of traps per night) of community-based ITT capture were conducted from March 2010 to January 2013. A total of 382 female An. gambiae were caught with an overall average catch per trap night of 0.024 (in phases I (0.064) and II (0.012). Out of 11,187 individuals who were tested for malaria infections; 1237 (11.1 %) were positive malaria cases and 9950 (88.9 %) were negative cases. The overall mean malaria prevalence was (11 %); in phase I the mean prevalence was 12.7 % (381/2754) and II was 10.8 % (854/8433).
Spatial autocorrelation
Spatial dependences were observed in all outcome variables (Table 1). The noted positive spatial autocorrelation in both measured outcomes indicated a tendency towards clustering, meaning that spatial samples of An. gambiae mosquito densities or malaria prevalence that were nearby to each other in space were more similar than would be expected by random chance (Table 1).
Spatial clustering in Anopheles gambiae mosquitoes
In the first phase of the survey, six spatially aggregated clusters of elevated An. gambiae density were found in the central, western and southern parts of the city (Fig. 2a). These clusters were small in size and highly focalized (median = 5 survey locations, range = 1–23), with only three out of the six containing more than one survey location. Of the detected clusters, only one cluster consisting of a single location occurred outside of the 15 wards covered by larviciding (Fig. 2a), confirming that targeted selection of these wards for the initial operational research phase of the UMCP [5, 17] was appropriately planned. The clusters of relatively high An. gambiae density detected in this phase consisted of less than a tenth of all survey locations, but accounted for almost one third of all An. gambiae caught (Table 2). Nevertheless, the remaining two thirds of all An. gambiae were caught outside these clusters (Table 2), and three quarters of the locations where any An. gambiae were caught (76 %; 95/125) were haphazardly scattered outside of these clusters, across a further small minority (9 %; 57/615) of all sampling units (Fig. 2a).
After one year of continued larviciding and extension of the survey sampling frames, all six of the An. gambiae hotspots detected in phase 1 could no longer be detected (Fig. 2c), consistent with epidemiological analyses indicating larviciding succeeded in suppressing populations of these hazardous malaria vectors [3]. Despite this generally encouraging picture of success for suppression of An. gambiae, one new, much larger cluster emerged in phase 2 in the north-eastern parts of the city (Fig. 2c) within some of the 15 wards with long-established larvicide delivery systems. As in phase 1, the one large cluster observed in phase 2 accounted for only one third of all An. gambiae collected (Table 2). In this second phase, the majority (73.7 %; 42/57) of scattered survey-locations where any An. gambiae were caught occurred outside of detected hotspots of spatially-aggregated clusters of elevated vector density.
Spatial clusters of human malaria infection prevalence
In phase 1, two spatially aggregated clusters of elevated malaria prevalence were detected (Fig. 2b). The primary cluster was a large one, located at the south of the city centre on either side of Msimbazi river valley. The only secondary cluster occurred immediately to the north of the primary cluster, in the eastern wards of Hanna Nassif, Mwananyamala and Kinondoni (Figs. 1, 2b). These clusters encompassed approximately two fifths of all survey locations and slightly more than half of all detected cases of malaria infection (Fig. 2b; Table 2). Nevertheless, the remaining majority (60 %) of locations with elevated (>10 %) prevalence, and almost half (45 %) of all detected malaria infections) occurred outside of these clusters haphazardly across disparate parts of the city (Fig. 2b; Table 2). These consisted of 50.8 % (67/132) of survey locations with higher prevalence. More than half (56.8 %, 37/65) of the survey locations in the clusters of higher malaria prevalence detected in phase 1, occurred within wards with active larviciding.
Although there was a substantial drop of malaria prevalence from the outset of phase 2, consistent with the results of detailed epidemiological analyses (Msellemu et al. pers. commun), more clusters (six) of malaria prevalence were detected (Fig. 2d). The primary cluster did not change from its previous location, but was dramatically reduced in size compared to phase 1. The remaining five clusters were in small (<1 km across) or consisted of long narrow linear pockets, distributed across peripheral parts of the city that had not previously been surveyed. In this second phase, these clusters of elevated malaria prevalence included only one fifth of all survey locations but contained almost half of all detected cases of malaria infections (Table 2). However, the remaining majority of detected infections nevertheless occurred outside of these clusters (Table 2), mostly [54.3 % (19/35)] in small, haphazardly scattered single locations with relatively high malaria prevalence (>10 %).
Association between vector density and human infection prevalence
Although the data frames for entomological and epidemiological surveys used in this study were not entirely comparable, it is nevertheless remarkable just how dissimilar the clustering patterns are for human malaria infection prevalence and densities of the main vector in both survey phases (Fig. 2). Despite the major differences in spatial and temporal resolution of the entomological and parasitological data, it was nevertheless possible to examine their mutual association in survey locations where both types of surveys were conducted. Overall population-wide prevalence at these locations was not associated with local vector density (Odds Ratio (OR) [95 % Confidence interval (CI)] = 1.56 [0.89, 1.78], P = 0.52)). Indeed, in many locations with elevated prevalence (>10 %), no An. gambiae were caught despite having been surveyed at least 6 times with tent traps (17.7 % (20/113) in phase 1 and 13.2 % (10/76) in phase 2). Conversely, only a minority of co-surveyed locations (26.8 % (11/42)) in phase 1 and 37.5 % (3/8) in phase 2) where An. gambiae were detected had human infection prevalence exceeding 10 %.
Discussion
The results presented here suggest it may not be possible to eliminate malaria transmission in Dar es Salaam, if interventions are only targeted to spatially clustered hotspots that could be detected with current methods for cross-sectional household surveys and longitudinal entomological surveillance. Even though two very different sampling frames were used in the two phases (Fig. 2), geographic cluster analysis consistently excluded over two thirds of all vectors caught and almost half of all malaria infections detected (Table 2). Of course, by definition, these spatial aggregations of elevated transmission account for even lower fractions of all surveyed locations (Table 2), so they do represent useful initial priority areas for geographically targeting new or improved interventions. However, these detected clusters of vector density and parasitaemia accounted for insufficient levels of aggregation to satisfy the “80–20 rule” [29] that is classically used to justify targeting of interventions to high-risk groups. So while targeting only the clusters of elevated vector density or human infection prevalence could tackle substantial portions of malaria transmission in Dar es Salaam, to achieve the ambitious goal of eliminating malaria transmission [30], or even the vector itself [31], would also require targeting of all the additional small, scattered locations where local transmission persists.
The haphazard distribution of this very sparse vector population is fully consistent with the known opportunistic oviposition habits of An. gambiae generally [32, 33] and in Dar es Salaam specifically [34–36]. This species can complete development from egg to adult in less than a week in almost any water body which lasts long enough, is exposed to the sun and is not heavily contaminated with organic waste [36, 37]. In order to eliminate transmission hazard, larval source management, or other supplementary vector control measures [7–9], would therefore need to not only target the obvious large clusters of high An. gambiae density, but also these smaller, haphazardly-distributed pockets of vector proliferation.
The scattered and sporadic distribution of malaria transmission hazard and risk in this modestly endemic setting is also consistent with previous reports from elsewhere in Africa [6, 38, 39]. Elimination of malaria from such contexts will therefore require continuous, longitudinal surveillance of both entomological and epidemiological indicators at fine geographic scales, to give dynamic, high-resolution maps of all the small, sometimes transient, hotspots of persistent transmission, so new or improved interventions can be delivered in a targeted and timely manner [6, 40]. The need for very fast response times to react to the unpredictable occurrence of unstable hot spots of largely symptomatic malaria [6] is perhaps best illustrated by the recent work from elsewhere on the east African coast, showing that targeted responses must be implemented within a month of occurrence to capture these cases in a meaningful way [38].
Remote sensing to map environmental determinants of vector proliferation hazard has achieved considerable success at local scales [41–45] but often lacks sufficient spatial resolution to detect small habitats of the type commonly used by species like An. gambiae [32, 33]. Locally, in this specific context, quality-assured community-based systems for using Ifakara Tent Traps have been developed for more directly monitoring and mapping transmission hazard, in terms of adult vector densities [19]. Consequently, the entomological indicators monitored here were surveyed at higher spatial (mean distance of 174 m in phase 1 and 210 m in phase 2 between survey locations) and temporal (monthly) resolution than was the epidemiological indicator. While quality-assured, community-based mosquito-trapping schemes at sentinel housing clusters centred on health facilities have been evaluated in rural Zambia [46], this study in urban Tanzania remains the only example in which such comprehensive, high-resolution coverage of an entire region has been achieved, so the general applicability of this approach will require further assessment in a greater diversity of settings.
The active cross-sectional parasitological surveys used here to assess epidemiological trends in Dar es Salaam only provided temporal snapshots of malaria dynamics, at far too coarse a geographical resolution to guide routine malaria control operations because they were distributed across a population exceeding three million people and a surface area of almost 500 km2, but yielded results that are nevertheless consistent with those of other studies. On the coast of Kenya, similar annual cross-sectional surveys of much smaller populations within three small rural communities allowed mapping of stable hot spots of transmission that persisted from year to year, while active longitudinal cohort surveys to detect febrile malaria enabled mapping of sporadic, unstable transmission hot spots that occur unpredictably in different locations from year to year [34]. Furthermore, a variety of other studies using intensively collected cross-sectional [47], incidence cohort [39] or health facility [38, 44, 48, 49] data within small study areas have all illustrated how hot spots ubiquitously occur at scales far smaller than 1 km.
The inadequate spatial resolution of these active cross-sectional surveys when deployed on such large, programmatically-relevant scales is particularly clearly illustrated by the results of a recently piloted passive surveillance system for mapping of malaria cases in Dar es Salaam, by tracing the home residence locations of patients reporting to health facilities with acute malaria, which demonstrated that hot spots of malaria risk in Dar es Salaam can be less than 100 m across and associations of risk with topographic wetness index can occur at similar scales (Mlacha et al. unpublished). The surprising location of the small cluster of high diagnostic positivity identified in the ward of Buguruni illustrates how mapping clusters by tracing patient’s residence from facilities data could be an effective system for detecting enigmatic, haphazardly-scattered locations of hotspots of transmission (Mlacha et al. unpublished), like the ones shown to occur all across the city by the study presented in this manuscript. Similar approaches to risk mapping using passively collected health facility data have also been successfully applied in a variety of other resource-limited contexts [38, 43–45, 48–50], so this overall strategy may offer an affordable and broadly applicable means to map malaria transmission, that yields particularly high geographic resolution in this urban context where transmission is very focal and grass-roots local government systems provide very fine-scale geographic reference points that community members can readily relate to (Mlacha et al. unpublished). Beyond scaleability and affordability, the other major advantage of facility-based mapping of malaria cases is the ability to rapidly detect sporadic, unpredictably distributed flare-ups of malaria transmission in sufficient time (≤1 month) to enable effective targeting of responsive anti-parasite interventions [38], such as focal mass drug administration or mass screening and treatment [8, 10].
As observed elsewhere [51], clusters of malaria infection prevalence only partially coincided with those of An. gambiae in Dar es Salaam (Fig. 2). Indeed, population-wide malaria prevalence and An. gambiae densities in any given location were only loosely associated to each other, in spite of malaria primarily being locally transmitted in the city, rather than imported (Msellemu et al. pers. commun). The lack of an obvious overall relationship between spatial distribution of malaria and the level of hazard presented by local vector densities may, therefore, be largely explained by differences in city’s population resilience levels, such as housing conditions, human behaviour and utilization of interventions [4, 52, 53]. Indeed more detailed epidemiological analyses of these same data confirm that high malaria risk appears to require both hazard and vulnerability: prevalence does increase dramatically with increasing local vector density among the small minority of households living in houses without protective window screening, but not among more resilient households protected by complete window screening [4]. It is, therefore, likely that intervention packages targeted to both stable and unstable hot spots of malaria transmission will need to not only reduce or eliminate the hazard represented by local vector proliferation, presumably with supplementary application of new or improved vector population abatement measures, they will also need to actively treat all local residents who are already infected with malaria [8, 10] as well as improve the resilience of the most vulnerable residents by providing them with protective measures such as bed nets [54], window screening [55] or spatial repellents [56, 57].
Study limitations
The sampling frames for mosquito densities and human malaria infection prevalence were incomparable due to differences in geographic and temporal resolution. Similarly, the differences in sampling frames across the two phases made it impossible to compare and track temporal/spatial trends within same outcome variables. Comparison was only possible in a few sampling locations which were co-surveyed.
Conclusions
Small scattered locations of elevated malaria transmission and densities of vector mosquitoes were haphazardly scattered across the city, so interventions targeted only to hotspots identified by geographic cluster approaches with conventional entomological and epidemiological survey methods will probably have limited impact. Routine, spatially comprehensive, longitudinal entomological, parasitological surveillance systems, with sufficient sensitivity and high spatial and temporal resolution to detect those scattered locations with elevated infections and mosquito densities, will be required to eliminate transmission from this typical contemporary city of sub-Saharan Africa. Given the loose association observed between vector proliferation hazard and malaria infection risk, intervention packages targeted to hot spots of malaria transmission will need to not only suppress local vector proliferation and treat residents who are already infected, but also provide the most vulnerable population members with supplementary protective measures against exposure.
References
Geissbuhler Y, Chaki P, Emidi B, Govella NJ, Shirima R, Mayagaya V, et al. Interdependence of domestic malaria prevention measures and mosquito-human interactions in urban Dar es Salaam. Tanzania Malar J. 2007;6:126.
Geissbuhler Y, Kannady K, Chaki PP, Emidi B, Govella NJ, Mayagaya V, et al. Microbial larvicide application by a large-scale, community-based program reduces malaria infection prevalence in urban Dar es Salaam. Tanzania. PLoS ONE. 2009;4:e5107.
Maheu-Giroux M, Castro MC. Do malaria vector control measures impact disease-related behaviour and knowledge? evidence from a large-scale larviciding intervention in Tanzania. Malar J. 2013;12:422.
Ogoma SB, Kannady K, Sikulu M, Chaki PP, Govella NJ, Mukabana WR, et al. Window screening, ceilings and closed eaves as sustainable ways to control malaria in Dar es Salaam. Tanzania Malar J. 2009;8:221.
Maheu-Giroux M, Castro MC. Impact of community-based larviciding on the prevalence of malaria infection in Dar es Salaam. Tanzania PLoS ONE. 2013;8:e71638.
Bejon P, Williams TN, Liljander A, Noor AM, Wambua J, Ogada E, et al. Stable and unstable malaria hotspots in longitudinal cohort studies in Kenya. PLoS Med. 2010;7(7):e1000304.
Killeen GF. Characterizing, controlling and eliminating residual malaria transmission. Malar J. 2014;13:330.
WHO. Global technical strategy for malaria 2016–2030. Geneva: Global Malaria Programme. 2015.
WHO. Guidance note: control of residual malaria parasite transmission. Geneva: World Health Organization, Global Malaria Programme. 2014.
Bousema T, Okell L, Felger I, Drakeley C. Asymptomatic malaria infections: detectability, transmissibility and public health relevance. Nat Rev Microbiol. 2014;12:833–40.
Bousema T, Griffin JT, Sauerwein RW, Smith DL, Churcher TS, Takken W, et al. Hitting hotspots: spatial targeting of malaria for control and elimination. PLoS Med. 2012;9(1):e1001165.
NBS. National Population Census and Projetions. Dar es Salaam: National Bureau of Statistics, Ministry of Planning and Privatisation, United Republic of Tanzania, 2012.
UN-Habitat. State of the World Cities. Harmonious Cities 2008/2009. Nairobi: United Nations Human Settlements Development Programme, 2008.
UN-Habitat. State of the World’s Cities 2010/2011-Cities for All: Bridging the Urban Divide. Nairobi: United Nations Human Settlements Development Programme, 2010.
Govella N, Chaki P, Killeen G. Entomological surveillance of behavioural resilience and resistance in residual malaria vector populations. Malar J. 2013;12:124.
Fillinger U, Kannady K, William G, Vanek M, Dongus S, Nyika D, et al. A tool box for operational mosquito larval control: preliminary results and early lessons from the Urban Malaria Control Programme in Dar es Salaam. Tanzania Malar J. 2008;7:20.
Dongus S, Mwakalinga V, Kannady K, Tanner M, Killeen G. Participatory mapping as a component of operational malaria vector control in Tanzania. In: Maantay JA, McLafferty S, editors. Geospatial Analysis of Environmental Health Geotechnologies and the Environment, vol. 4. Netherlands: Springer; 2011. p. 321–36.
Dongus S, Nyika D, Kannady K, Mtasiwa D, Mshinda H, Fillinger U, et al. Participatory mapping of target areas to enable operational larval source management to suppress malaria vector mosquitoes in Dar es Salaam. Tanzania Int J Health Geogr. 2007;6:37.
Chaki P, Mlacha Y, Msellemu D, Muhili A, Malishee A, Mtema Z, et al. An affordable, quality-assured community-based system for high-resolution entomological surveillance of vector mosquitoes that reflects human malaria infection risk patterns. Malar J. 2012;11:172.
Govella NJ, Moore JD, Killeen GF. An exposure free tool for monitoring adult malaria mosquito populations. Am J Trop Med Hyg. 2010;83:596–600.
Scott JA, Brogdon WG, Collins FH. Identification of single specimens of the Anopheles gambiae complex by the polymerase chain reaction. Am J Trop Med Hyg. 1993;49:520–9.
Tango T, Takahashi K. FleXScan software. https://sites.google.com/site/flexscansoftware/download_e (2015). Accessed Aug 15th 2015.
Moran P. The interpretation of statistical maps. J R Stat Soc B. 1948;19:243–51.
Tango T, Takahashi K. A flexibly shaped spatial scan statistic for detecting clusters. Int J Health Geogr. 2005;4:11.
Takahashi K, Tango T. An extended power of cluster detection tests. Stat Med. 2006;25:841–52.
Kuldorff M. Statistical methods for spatial epidemiology: tests for randomness. In: Gatrell A, Laytonen M, editors. GIS and health. London: Taylor and Francis; 1998. p. 49–62.
Huang L, Tiwari RC, Zou Z, Kulldorff M, Feuer EJ. Weighted Normal Spatial Scan Statistic for Heterogeneous Population Data. http://www.satscan.org/papers/huang-M2009.pdf (2008). Accessed 25th Sept 2014.
Kuldorff M. SaTScan User Guide for version 9.3. http://www.satscan.org/ (2010). Accessed 27th July 2014.
Woolhouse ME, Dye C, Etard JF, Smith T, Charlwood JD, Garnett GP, et al. Heterogeneities in the transmission of infectious agents: implications for the design of control programs. Proc Natl Acad Sci USA. 1997;94:338–42.
UN. From Aspiration to Action: what will it take to end malaria?. New York; 2015.
Killeen G, Seyoum A, Sikaala C, Zomboko A, Gimnig J, Govella N, et al. Eliminating malaria vectors. Parasit Vectors. 2013;6:172.
Gillies MT, De Meillon B. The Anophelinae of Africa south of the Sahara (Ethiopian Zoogeographical Region). S Afr Inst Med Res Annu Rep. 1968;54:1–343.
Holstein MH. Biology of Anopheles gambiae. Geneva: World Health Organization; 1954.
Fillinger U, Lindsay SW. Larval source management for malaria control in Africa: myths and reality. Malar J. 2011;10:353.
Vanek M, Shoo B, Mtasiwa D, Kiama M, Lindsay S, Fillinger U, et al. Community-based surveillance of malaria vector larval habitats: a baseline study in urban Dar es Salaam. Tanzania BMC Public Health. 2006;6:154.
Sattler M, Mtasiwa D, Kiama M, Premji Z, Tanner M, Killeen G, et al. Habitat characterization and spatial distribution of Anopheles sp. mosquito larvae in Dar es Salaam (Tanzania) during an extended dry period. Malar J. 2005;4:4.
Yaro A, Dao A, Adamou A, Crawford J, Ribeiro J, Gwadz R, et al. The distribution of hatching time in Anopheles gambiae. Malar J. 2006;5:19.
Bejon P, Williams TN, Nyundo C, Hay SI, Benz D, Gething PW, et al. A micro-epidemiological analysis of febrile malaria in Coastal Kenya showing hotspots within hotspots. eLife. 2014;3:e02130.
Gaudart J, Poudiougou B, Dicko A, Ranque S, Toure O, Sagara I, et al. Space-time clustering of childhood malaria at the household level: a dynamic cohort in a Mali village. BMC Public Health. 2006;6:286.
Snow J. On the mode of communication of cholera. Reprint: New York; 1965
Atieli HE, Zhou G, Lee MC, Kweka EJ, Afrane Y, Mwanzo I, et al. Topography as a modifier of breeding habitats and concurrent vulnerability to malaria risk in the western Kenya highlands. Parasit Vectors. 2011;4:241.
Balls MJ, Bodker R, Thomas CJ, Kisinza W, Msangeni HA, Lindsay SW. Effect of topography on the risk of malaria infection in the Usambara Mountains, Tanzania. Trans R Soc Trop Med Hyg. 2004;98:400–8.
Cohen JM, Ernst KC, Lindblade KA, Vulule JM, John CC, Wilson ML. Local topographic wetness indices predict household malaria risk better than land-use and land-cover in the western Kenya highlands. Malar J. 2010;9:328.
Sturrock HJW, Cohen JM, Keil P, Tatem AJ, Le Menach A, Ntshalintshali NE, et al. Fine-scale malaria risk mapping from routine aggregated case data. Malar J. 2014;13:421.
Cohen JM, Dlamini S, Novotny JM, Kandula D, Kunene S, Tatem AJ. Rapid case-based mapping of seasonal malaria transmission risk for strategic elimination planning in Swaziland. Malar J. 2013;12:61.
Sikaala C, Chinula D, Chanda J, Hamainza B, Mwenda M, Mukali I, et al. A cost-effective, community-based, mosquito-trapping scheme that captures spatial and temporal heterogeneities of malaria transmission in rural Zambia. Malar J. 2014;13:225.
Brooker S, Clarke S, Njagi JK, Polack S, Mugo B, Estambale B, et al. Spatial clustering of malaria and associated risk factors during an epidemic in a highland area of western Kenya. Trop Med Int Health. 2004;9:757–66.
Yeshiwondim A, Gopal S, Hailemariam A, Dengela D, Patel H. Spatial analysis of malaria incidence at the village level in areas with unstable transmission in Ethiopia. Int J Health Geogr. 2009;8:5.
Ernst K, Adoka S, Kowuor D, Wilson M, John C. Malaria hotspot areas in a highland Kenya site are consistent in epidemic and non-epidemic years and are associated with ecological factors. Malar J. 2006;5:78.
Kazembe LN, Kleinschmidt I, Sharp BL. Patterns of malaria-related hospital admissions and mortality among Malawian children: an example of spatial modelling of hospital register data. Malar J. 2006;5:93.
Bousema T, Drakeley C, Gesase S, Hashim R, Magesa S, Mosha F, et al. Identification of hot spots of malaria transmission for targeted malaria control. J Infect Dis. 2010;1:201.
Dongus S, Pfeiffer C, Metta E, Mbuyita S, Obrist B. Building multi-layered resilience in a malaria control programme in Dar es Salaam. Tanzania Prog Dev Stud. 2010;10:309–24.
Ogoma S, Ngonyani H, Simfukwe E, Mseka A, Moore J, Killeen G. Spatial repellency of transfluthrin-treated hessian strips against laboratory-reared Anopheles arabiensis mosquitoes in a semi-field tunnel cage. Parasit Vectors. 2012;5:54.
Augustincic PL, Petkovic J, Welch V, Ueffing E, Tanjong GE, Pardo PJ, et al. Strategies to increase the ownership and use of insecticide-treated bednets to prevent malaria. Cochrane Database Syst Rev. 201;3:CD009186.
Tusting L, Ippolito M, Willey B, Kleinschmidt I, Dorsey G, Gosling R, et al. The evidence for improving housing to reduce malaria: a systematic review and meta-analysis. Malar J. 2015;14:201.
Achee N, Bangs M, Farlow R, Killeen G, Lindsay S, Logan J, et al. Spatial repellents: from discovery and development to evidence-based validation. Malar J. 2012;11:164.
Govella N, Ogoma S, Paliga J, Chaki P, Killeen G. Impregnating hessian strips with the volatile pyrethroid transfluthrin prevents outdoor exposure to vectors of malaria and lymphatic filariasis in urban Dar es Salaam. Tanzania. Parasit Vectors. 2015;8:322.
Authors’ contributions
VMM led the design and implementation of the study, including data analysis, preparing maps and drafting of the manuscript. YPM, JMP, ZDM, AJL and DFM implemented the adult mosquito and household parasitology surveys, as well as data collection and management of the data, including spatial information. SD, BKDS, NJG, MC and GFK supervised the design and implementation of the study, interpretation of results, and preparation of the manuscript. All authors read and approved the final manuscript.
Acknowledgements
We thank the participatory mapping team who provided concrete baseline spatial data for hotspots detection in this study. We also thank the entire team who participated in both entomological and epidemiological surveys for their perseverance and commitment. Thanks to all the Dar es Salaam residents and their respective TCU, ward and municipal leaders for their tremendous support throughout the study. The digital elevation model used to derive hydrological and topographical variables in this study was kindly provided by Richard Sliuzas (ITC Enschede) and the University of Dortmund. Funding for collecting the entomological and epidemiological data used in this study was provided by the Bill and Melinda Gates Foundation through the Malaria Transmission Consortium and the European Union Seventh Framework Programme (FP7/2007-2013 grant agreement 265660). VMM was supported through a PhD fellowship from the Consortium for Advanced Research Training in Africa (CARTA), jointly led by the African Population and Health Research Centre and the University of the Witwatersrand with financial support from the Wellcome Trust (Grant No. : 087547/Z/08/Z), the Department for International Development (DfID) under the Development Partnerships in Higher Education (DelPHE), the Carnegie Corporation of New York (Grant No: B 8606), the Ford Foundation (Grant No: 1100-0399), Google.Org (Grant No: 191994), Sida (Grant No: 54100029) and MacArthur Foundation Grant No: 10-95915-000-INP. VMM was also supported by a Hassan Mshinda Career Development Fellowship, which was collaboratively funded by the Liverpool School of Tropical Medicine and the Ifakara Health Institute. DM, YM, NJG and GFK were all supported by fellowship awards from the Wellcome Trust at various stages of this study.
Competing interests
The authors declare that they have no competing interests.
Author information
Authors and Affiliations
Corresponding author
Rights and permissions
Open Access This article is distributed under the terms of the Creative Commons Attribution 4.0 International License (http://creativecommons.org/licenses/by/4.0/), which permits unrestricted use, distribution, and reproduction in any medium, provided you give appropriate credit to the original author(s) and the source, provide a link to the Creative Commons license, and indicate if changes were made. The Creative Commons Public Domain Dedication waiver (http://creativecommons.org/publicdomain/zero/1.0/) applies to the data made available in this article, unless otherwise stated.
About this article
Cite this article
Mwakalinga, V.M., Sartorius, B.K.D., Mlacha, Y.P. et al. Spatially aggregated clusters and scattered smaller loci of elevated malaria vector density and human infection prevalence in urban Dar es Salaam, Tanzania. Malar J 15, 135 (2016). https://doi.org/10.1186/s12936-016-1186-9
Received:
Accepted:
Published:
DOI: https://doi.org/10.1186/s12936-016-1186-9