Abstract
The evolution of the surface topography of a calcite crystal subject to dissolution is documented through in situ real-time imaging obtained via atomic force microscopy (AFM). The dissolution process takes place by exposing the crystal surface to deionized water. AFM data allow detection of nucleation and expansion of mono- and multilayer rhombic etch pits and are employed to estimate the spreading rate of these structures. Spatially heterogeneous distributions of local dissolution rate are evaluated from the difference between topographic measurements taken at prescribed time intervals. We rest on a stochastic framework of analysis viewing the dissolution rate as a generalized sub-Gaussian (GSG) spatially correlated random process. Our analysis yields: (i) a quantitative assessment of the temporal evolution of the statistics of the dissolution rates as well as their spatial increments; (ii) a characterization of the degree of spatial correlation of dissolution rates and of the way this is linked to the various mechanisms involved in the dissolution process and highlighted through the experimental evidences. Our results indicate that the parameters driving the statistics of the GSG distribution and the spreading rate of the multilayer pits display a similar trend in time, thus suggesting that the evolution of these structures imprints the statistical features of local dissolution rates.
Article Highlights
-
We investigate dynamics of dissolution patterns on a calcite crystal in contact with deionized water via AFM imaging
-
Temporal behavior of parameters of our statistical model is consistent with surface pattern evolution
-
A nested model for the spatial correlation of rates embeds multiple mechanisms driving dissolution rate.
Similar content being viewed by others
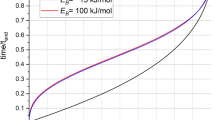
Avoid common mistakes on your manuscript.
1 Introduction
Proper assessment of reactive processes at solid–liquid interfaces is of critical importance for the characterization of flow and transport in porous media, as these drive possible alterations of key physical attributes of the hosting formation, such as porosity, permeability, or storage (Hommel et al. 2018). As such, detailed knowledge of dissolution/precipitation reaction kinetics has relevant implications in several industrial and environmental contexts including, e.g., aquifer contamination (Bianchi Janetti et al. 2013), geothermal energy exploitation (Erol et al. 2019), enhanced (conventional and unconventional) management of subsurface energy resources (Khather et al. 2020), or geological storage of CO2 (Daval 2018). Calcite has been a major target of kinetic characterization studies, due to its widespread presence in geological environments and its affinity to incorporate trace elements in the solution, acting as a potential sink for heavy metals and pollutants (Heberling et al. 2014; Renard et al. 2019).
A bulk powder experiment is typically considered a pillar for the estimation of dissolution rates (Luttge et al. 2013), which are inferred by monitoring changes in dissolved solute concentrations. While these experiments are simple and characterized by a remarkable level of reproducibility, an intrinsic difficulty is related to the observation that measured changes in mass must be normalized by the powder surface area to obtain proper units of rate, i.e., mass per unit area per unit time. Large discrepancies between dissolution rates evaluated under identical laboratory conditions are documented (Arvidson et al. 2003) and are attributed to the way the powder surface area is computed and to the actual portion of the mineral surface that is available for the reaction (Luttge et al. 2013; Luttge 2005).
The use of high-resolution imaging techniques such as vertical scanning interferometry (VSI) and atomic force microscopy (AFM) enables the observation of the surface topography of dissolving minerals at the molecular level. These techniques are contributing to enhance our knowledge of the main mechanisms driving reactions at fluid–solid interfaces and have been extensively employed for the investigation of calcite dissolution in aqueous solution (see, e.g., Anabaraonye et al. 2019; Bibi et al. 2018; Bouissonnié et al. 2018; Fischer et al. 2012; Smith et al. 2013 for VSI; Dong et al. 2020; Offeddu et al. 2014; Renard et al. 2019; Renard et al. 2015; Teng 2004; Xu et al. 2010 for AFM). While AFM is more limited in terms of observation window as compared to VSI (maximum size being in the order of 10 and 100 µm, respectively), it is characterized by an enhanced level of lateral resolution (minimum pixel size being in the order of 10 and 100 nm, for AFM and VSI, respectively) and is more suited for in situ and real-time detection of surface topography and atomic-resolution forces.
Measurements of average surface retreat with respect to a local reference (i.e., a region covered by a non-reactive mask embedded on the sample) can be employed to estimate a mean dissolution rate, 〈R〉 \([{\text{mol}} \, {\text{m}}^{ - 2} \, {\text{s}}^{ - 1} ]\), as
where \(\Delta h \, [{\text{m}}]\) is the surface retreat evaluated with respect to the reference and 〈 〉 denotes spatial averaging; \(\Delta t\) [s] is the duration of the experiment; and \(V_{m} [{\text{m}}^{3} \,{\text{mol}}^{ - 1} ]\) is calcite molar volume. This approach enables one to overcome difficulties/uncertainties typical of the bulk powder approach and associated with the need to estimating the surface area. However, relying on average quantities of the kind associated with Eq. 1 leads to a complete loss of information about the often marked degree of heterogeneity of local surface topography (and hence rate) values that is revealed by high-resolution imaging techniques. Heterogeneities at the microscopic level result from local inhomogeneities and defects on the crystal structure. These are usually regarded as sources of intrinsic variability and lead to uneven distributions of surface free energy (i.e., cohesive energy at the calcite surface). Their impact on the dissolution process has been identified as the ultimate cause of the wide range of average rate estimates under given experimental conditions (Luttge et al. 2013).
Since the density and the distribution of defects on the mineral surface displays traits which are typical of random processes, there is a growing need for a change in perspective when developing experimentally driven interpretive models of such processes. A shift of paradigm towards the reliance on a stochastic approach has been encouraged by several authors (Fischer et al. 2014, 2012; Luttge et al. 2019, 2013) to capture the spatial variability of dissolution rates. Fischer et al. (2012) introduced the concept of rate spectra (i.e., the frequency distribution of local rate values in the domain) as a tool to preserve the variety of surface features contributing to the overall dissolution process. In this sense, these authors interpret the ensuing frequency distribution of rates as a convolution of modes, each representing a basic kinetic component. This aspect has been further investigated by other studies where rate spectra are extrapolated from (i) AFM in situ imaging of surface topography on dissolving calcite in limestone pores (Levenson and Emmanuel 2013) and dolostone (Emmanuel 2014); (ii) VSI topography data under far-from-equilibrium conditions taken in situ and in real time on the surface of a calcite crystal (Bibi et al. 2018) or ex situ on a calcite-cemented sandstone (Trindade Pedrosa et al. 2019); and (iii) digital holographic microscopy (DHM) for in situ topography acquisitions on a calcite crystal in contact with deionized water (Brand et al. 2017).
First attempts at identifying a suitable model for the interpretation of rate spectra rely on the generalized extreme value (GEV) distribution (Brand et al. 2017; Emmanuel 2014). Brand et al. (2017) highlight a dependency between the distribution parameters and the temporal window across which dissolution rates are evaluated. Pollet-Villard et al. (2016) and Siena et al. (2020) show examples of the application of geostatistics for the study of the spatial heterogeneity of reactive processes data at the microscopic level. Pollet-Villard et al. (2016) rely on common variogram analyses of topography data measured experimentally through AFM imaging of a dissolving orthoclase surface in far-from-equilibrium conditions. Siena et al. (2020) interpret the statistics of VSI-based measurements of calcite surface topography subject to dissolution in close-to-equilibrium conditions within the recently developed statistical framework of analysis relying on a generalized sub-Gaussian (GSG) formulation (Riva et al. 2015). The latter constitutes a modeling approach which enables one to characterize jointly the statistical behavior of a given (spatially heterogeneous) quantity, \(Y({\mathbf{x}})\) (x denoting a vector of spatial coordinates), and its spatial (or temporal) increments, \(\Delta Y({\mathbf{s}}) = Y({\mathbf{x}} + {\mathbf{s}}) - Y({\mathbf{x}})\) evaluated at multiple separation distances (or lags), s. This approach has been shown to fully embed statistical features which have been documented by observations of a broad spectrum of hydrogeological variables [e.g., porosity (Guadagnini et al. 2014; Painter 1996; Riva et al. 2015), permeability (Painter 1996; Riva et al. 2013b, a) or hydraulic conductivity (Guadagnini et al. 2013; Liu and Molz 1997; Meerschaert 2004)] and resulting in a clear scale-dependent behavior of the sample distributions (and associated statistical moments) of spatial increments (see also Sect. 2.4 for additional details).
Here, we aim at characterizing the spatial variability of the dissolution rate inferred from in situ and real-time AFM imaging of a calcite sample, cleaved along the \(\left\{ {104} \right\}\) surface plane (where indices 104 characterize the plane orientation, curly brackets denoting inclusion of all planar surfaces that, due to the symmetry of the crystal lattice, are equivalent to plane (104)), in contact with deionized water. The reaction is initially in far-from-equilibrium conditions. As the fluid in contact with the sample is static, the degree of saturation of the solution with respect to the dissolved phase progressively increases within a boundary layer at the fluid/solid interface. Thus, a transition from a surface-dominated to a diffusion-dominated reaction takes place (see also, e.g., Renard et al. 2019). Our main goal is to investigate the effects of this transition on surface topography patterns driven by dissolution and on the degree of spatial heterogeneity of the associated rate within a temporal interval immediately after the renewal of the solution in contact with the sample in the AFM cell. The analysis rests on (i) the evaluation of the statistical behavior of the observed local rate values and of the associated spatial increments and (ii) the identification of quantitative metrics to assess the temporal evolution of rate heterogeneity.
2 Materials and Methods
2.1 Calcite Dissolution Mechanisms
Reactivity of calcite \(\left\{ {104} \right\}\) surface is of primary interest in natural settings. On this plane, calcite exhibits steps oriented along \([\overline{4}41]\) and \([48\overline{1}]\) crystallographic directions (where the overbar represents a negative crystallographic index) and is characterized by a rhombohedral symmetry. Two parallel edges of an etch pit form differing angles with the terrace surface, indicated as acute (\([\overline{4}41]_{ - }\) and \([48\overline{1}]_{ - }\)) and obtuse (\([\overline{4}41]_{ + }\) and \([48\overline{1}]_{ + }\)) steps, respectively. The difference in atomic configurations of these steps leads to a distinct material removal rate, dissolution of acute steps being slower than the one associated with obtuse steps (Harstad and Stipp 2007). Calcite dissolution can take place by progressive retreat of preexisting steps or etch pit nucleation. The latter can be originated from either surface defects or can occur randomly on a flat terrace. Spontaneous pit nucleation requires a higher amount of energy with respect to the defect-assisted process, which, in turn, requires a higher energy than step retreat. As a consequence, the prevailing dissolution mode is directly related to the distance of the reaction from equilibrium, as quantified through the saturation state, \(\Omega = {{{\text{IAP}}} \mathord{\left/ {\vphantom {{{\text{IAP}}} {K_{{{\text{sp}}}} }}} \right. \kern-\nulldelimiterspace} {K_{{{\text{sp}}}} }}\), where \({\text{IAP}} = a_{{{\text{Ca}}^{2 + } }} a_{{{\text{CO}}_{3}^{2 - } }}\) is the ion activity product for calcite and \(K_{{{\text{sp}}}} = a_{{{\text{Ca}}^{2 + } ,{\text{eq}}}} a_{{{\text{CO}}_{3}^{2 - } ,{\text{eq}}}}\) is the solubility product constant, obtained as the product of the species activities at equilibrium. By definition, \(\Omega\) approaches unity as the system tends to thermodynamic equilibrium. As suggested by several authors (Bouissonnié et al. 2018; Kristensen et al. 2004; Teng 2004), at extreme undersaturation conditions (\(\Omega < 0.007\)) the chemical potential is such that the energy barrier associated with spontaneous pits nucleation can be overcome and shallow pits (having depths of a single layer) are allowed to form at random locations on the terraces. For a lower degree of undersaturation, i.e., \(0.007 < \Omega < 0.41 - 0.54\), the nucleation of etch pits can be originated only at locations corresponding to linear defects in the crystal lattice, and dissolution takes place both by nucleation at these locations and by step retreat. Within this saturation range, a transition from rhombic to triangular pit shape is documented at \(\Omega \approx 0.1 - 0.2\), as a consequence of progressive roundings of the obtuse-obtuse corners (Harstad and Stipp 2007). Dissolution takes place by means of step retreat under close-to-equilibrium conditions (i.e., for \(\Omega > 0.41 - 0.54\)), the terraces not being involved in the reaction.
2.2 Experimental Settings
A calcite sample has been prepared immediately before the experiment session by cleaving a crystal of Iceland spar (Mexico), pressing along the \(\left\{ {104} \right\}\) plane with a razor blade. The sample (having size \(\sim 5 \times 3 \times 1{\text{ mm}}^{{3}}\)) is secured on a slide and fluxed with nitrogen, to remove possible residues resulting from the cleavage operation. A Viton O-ring is mounted on the AFM support to seal the volume of the fluid cell, the latter being open to air and centered about the sample. Topography measurements are acquired with an AFM (Keysight 5500 apparatus) in contact mode. The tips (Bruker; model: RESPA-40) used in the study are made of Si and are specifically designed for contact mode acquisition. The cantilever (k = 5 N/m) has an Al coating to enhance the laser back reflection. A constant scan frequency (at 2.2 Hz) is employed to cover an area of \(6 \times 6\) µm2 discretized according to a \(512 \times 512\) grid (pixel size, dl = 11.7 nm). Only top-down scans are considered for our analyses. The dissolution process is started by exposing the crystal surface to deionized MilliQ water (18.2 \({\text{M}}\Omega \cdot {\text{cm}}\)) for a preliminary period of 1 h and 30 min. The volume of fluid enclosed in the cell (~2 ml) is connected to a system of syringes allowing replacement of the solution in contact with the sample. The sequence of measurements analyzed starts after the renewal of the fluid volume in the cell and spans a temporal window of width T = 30 min, the acquired images being spaced by a uniform time step \(\Delta t = 5\min\).
Real-time AFM imaging during calcite dissolution is demanding and requires performing several experiments for the identification of the ideal setup. A proper calibration of the AFM scanning rate has to be performed upon considering a tradeoff between the quality of the acquisition and the dissolution time scale. We performed 10 experiments on diverse samples, all cleaved from the same block of Iceland spar. We observed a consistent evolution of the surface patterns in all of these experiments (not shown). The collection of topography data included in this study enables us to (i) capture the evolution of multiple surface features observed in all of the experiments and (ii) obtain an excellent microscope stability across the whole temporal window investigated.
In the AFM system adopted, the piezo-scan in the x–y plane is associated with the displacement of the tip and not of the sample. This element, together with the small size of the scanned area and a preliminary warm-up phase of about 1 h prior to starting image acquisition, contributed to reduce horizontal drifts to negligible values.
2.3 Surface Topography Data and Spatial Distributions of Rate
The spatial distribution of surface elevations at a given time t, \(z(x,y,t)\), is expressed as the sum of an average value, \(\left\langle {z(t)} \right\rangle\), independent of location \((x,y)\), and a local zero-mean fluctuation, \(z^{^{\prime}} (x,y,t)\). Recalling Eq. 1, the spatial distribution of dissolution rates can be obtained as:
The term \(\left\langle {R(t)} \right\rangle\) represents a uniform (average, global) dissolution rate on the whole surface and can be evaluated only through knowledge of \(\left\langle {z(t)} \right\rangle\) and \(\left\langle {z(t - \Delta t)} \right\rangle\) or having at our disposal an inert (i.e., non-reactive) region on the surface, whose elevation can be used as a common reference for all times; the second term, \(R^{^{\prime}} (x,y,t)\), is informative about the spatial variability of the rates.
AFM yields topography data with high vertical resolution (in the order of 0.1 nm). As reported by Marinello et al. (2010), a preliminary detrend on data is often required, to remove spurious contributions, such as the slope of the sample support, which may be non-negligible at the considered level of resolution, or bow distortion due to the bending of the piezo-tube of the AFM scanner, which might result in an artificial curvature of the surface. The actual surface elevation, z, can be derived from data, according to \(z(x,y,t) = z_{meas} (x,y,t) - S(x,y,t)\), \(z_{meas}\) and S being the measured value and the overall distortion, respectively.
In our experiments, the entire surface of the crystal is in contact with the liquid in the cell and is therefore prone to reaction. The overall distortion can be inferred only by fitting the measured data with a given polynomial function. Hence, the ensuing trend, T(x, y, t), necessarily includes also the average elevation, i.e., \(T(x,y,t) = S(x,y,t) + \left\langle {z(t)} \right\rangle\). This, in turn, implies that the detrended data:
correspond to \(z^{^{\prime}} (x,y,t)\). Since we lose all information about \(\left\langle {z(t)} \right\rangle\), the spatial map of rates obtained from the difference of the topography measurements at two diverse observation times corresponds to the dissolution rate fluctuations:
which is then subject to our statistical analyses. Even as this does not enable us to obtain absolute rate estimates, it provides insights on their characteristic spatial variability.
2.4 Statistical Modeling Framework
We consider a given quantity of interest, \(Y({\mathbf{x}})\), to be expressed as the sum of an ensemble mean, \(\left\langle Y \right\rangle\), and a local zero-mean fluctuation, \(Y^{^{\prime}} ({\mathbf{x}})\). Here, we rest on the generalized sub-Gaussian (GSG) model proposed by (Riva et al. 2015) to capture the scaling features exhibited by the probability distributions of incremental data, \(\Delta Y({\mathbf{s}}) = Y({\mathbf{x}} + {\mathbf{s}}) - Y({\mathbf{x}})\). The latter have been shown to be characterized by sample distributions whose tails and peak tend to become, respectively, heavier and sharper as the lag, s, decreases (see, e.g., Guadagnini et al. 2018 and references therein). We recall that the concepts of peakedness and tailedness are both typically related to the heaviness of the distribution tails, a heavy-tailed pdf being a distribution whose tails are not bounded by the exponential pdf (the opposite holds for fat-tailed pdfs). Here, we illustrate the key analytical expressions of the GSG theoretical framework, the complete set of details about their derivation being available in Riva et al. (2015).
The GSG model considers:
\(G({\mathbf{x}})\) and \(U({\mathbf{x}})\) being a zero-mean (typically correlated) Gaussian random field and a nonnegative subordinator independent of G. The Gaussian field is characterized by a scale parameter, \(\sigma_{G}\), i.e., the standard deviation of G, and a correlation function, \(\rho_{G}\), that typically decreases as the separation distance between two points increases. Consistent with Riva et al. (2015) and Siena et al. (2019), we consider \(U({\mathbf{x}})\) to be characterized by a log-normal distribution:
\(\alpha < 2\) being the shape parameter. The resulting distributional form for \(Y^{^{\prime}} ({\mathbf{x}})\) corresponds to a normal-log-normal (NLN) model:
with second and fourth statistical moments expressed as:
The standardized kurtosis, which provides a measure of the degree of sharpness of the distribution peak, can be expressed as:
Hence, the probability density function (pdf) of \(Y^{^{\prime}}\) is leptokurtic for \(\alpha < 2\) and tends to a Gaussian pdf (with \(\kappa_{{Y^{^{\prime}} }} = 3\)) for \(\alpha \to 2\).
The pdf of spatial increments, \(\Delta Y({\mathbf{s}})\), at lag s is:
with \(r = \sqrt {u_{1}^{2} + u_{2}^{2} - 2\rho_{G}^{{}} u_{1} u_{2} }\).
Second- and fourth-order moments and standardized kurtosis are defined for each lag as:
It can be noted that \(\kappa_{\Delta Y}\) depends on the lag through the correlation function of G, i.e., \(\rho_{G}\), yielding pdfs of incremental data that scale with lag. In summary, the GSG model is fully characterized by (i) a shape parameter \(\alpha\), controlling the peakedness (and tailedness) of the distribution, (ii) a scale parameter \(\sigma_{G}\), related to the width of the distribution, and (iii) a correlation parameter, \(\rho_{G}\), which is responsible of the scaling nature of increment pdfs.
Here, we set \(Y^{^{\prime}} = R^{^{\prime}} (x,y,t)\) and provide estimates of the parameters of the GSG model to interpret the dissolution rate datasets at each given time t. We follow Riva et al. (2015) and perform model parameter estimation through the method of moments upon relying jointly on sample statistics of both \(R^{^{\prime}}\) and \(\Delta R(s)\) data. Replacing \(\left\langle {Y^{^{\prime}2} } \right\rangle\),\(\left\langle {\Delta Y^{2} } \right\rangle\), and \(\left\langle {\Delta Y^{4} } \right\rangle\) in Eqs. 8, 12, and 13 with their counterparts, \(M_{2}^{{R^{^{\prime}} }}\), \(M_{2}^{\Delta R}\), and \(M_{4}^{\Delta R}\) inferred from the available data yields:
Note that values of the scale and shape parameters are expected to be (approximately) constant with s, the Gaussian field correlation decreasing as s increases. We assess the temporal evolution of the strength of the spatial correlation of reaction rates upon relying on the estimates of \(\rho_{G}\) as a function of the lag and evaluating the key parameters of suitable theoretical models employed to interpret these.
3 Results
3.1 Evolution of Calcite Dissolution Patterns
Figure 1 depicts the AFM (friction) image acquired after a preliminary exposure of the calcite surface to MilliQ water for 1 h and 30 min. The observation time associated with Fig. 1 is hereafter denoted as t = t0 and corresponds to the time immediately before the renewal of the solution in contact with the mineral surface. The most evident feature is the presence of precipitates superimposed to the crystallographic steps that can be seen in the background. The formation of precipitates is compatible with the development of a supersaturated zone within a boundary layer at the solid/fluid interface (Renard et al. 2019).
Figure 2 collects the images acquired at a uniform time step, \(\Delta t\) = 5 min. Each plot is associated with the time ti (i = 1, …, 6) elapsed between the fluid replacement operation and the end of the acquisition of the i-th image. The key features associated with these results are illustrated in the following:
-
At time t1 = 5 min (Fig. 2a), the precipitates observed at time t0 have been dissolved and the underlying steps of the crystal become clearly visible. Several rhombic monolayer pits (mPs), whose appearance is typically associated with high undersaturation, begin to develop (with particular reference to the wider terraces). The edges of a large multilayer pit (denoted as MP1) are visible on the lower-right corner of the image.
-
Time t2 = 10 min (Fig. 2b) is characterized by an increased density of mPs that have also widened with respect to t1, new mPs nucleating at seemingly random locations and in the center of the existing ones. A new multilayer pit (denoted as MP2) starts to develop at the top left corner. Terrace steps on the surface appear to be stable.
-
No newly formed mPs are detectable at time t3 = 15 min (Fig. 2c). The existing ones widen and coalesce, their shape mutating from rhombic to triangular. The \([\overline{4}41]_{ - }\) acute step of MP1 is expanding slowly, whereas MP2 has spread considerably along the \([48\overline{1}]_{ + }\) obtuse step direction and the margin of a third MP (MP3) is seen to invade the domain of investigation right below MP2. The step profiles close to these two pits begin to evolve.
-
Most of the mPs are no longer visible at time t4 = 20 min (Fig. 2d), as they are essentially merged with other pits or with the edges of retreating steps. Compared to the previous image, the shape of the step profiles has changed significantly and in an irregular fashion, due to the progressive inclusion of mPs of differing sizes. The margin of a high step (visible along the top of the investigated region) seems to prevent further spreading of the obtuse step \([48\overline{1}]_{ + }\) of MP2. The expansion of the \([48\overline{1}]_{ + }\) edge of MP3 results in the merging of this MP with MP2.
-
No isolated mPs can be seen at time t5 = 25 min (Fig. 2e). The spreading of MPs slows down considerably. Dissolution is basically occurring solely by step retreat.
-
At time t6 = 30 min (Fig. 2f), no relevant differences with respect to the pattern observed at t5 can be seen, with the only exception of the evolution of the steps, the distance between neighboring ones being generally reduced.
The dissolution pattern on the crystal surface is strongly related to the driving dissolution mechanisms detailed in Sect. 2.1, these being in turn affected by the calcite concentration at the fluid/solid interface. The evolution described above is consistent with the expected temporal increase of concentration within the boundary layer. As opposed to what can be observed at t = t0 (Fig. 1), no precipitates are detected at t = t6. This could suggest that local supersaturation of the boundary layer has not been attained yet. Otherwise, this could also be ascribed to the effect of the scanning probe on the investigated area, as the AFM tip may displace precipitates that are weakly connected to the surface (see also Guren et al. 2020, and Renard et al. 2019). Spreading of MP1 takes place only along the acute step and is characterized by a lower extent than what can be noted for MP2 and MP3. Based on the behavior observed for MP2 at t3 and t4, we can assume that its growth is limited by the presence of larger steps or pits, even as the overall width of our observation window does not enable us to completely verify this hypothesis.
3.2 Evaluation of the Pit Spreading Rate
A measure of surface reactivity is given by the spreading rate (\(v\) [nm/s]) of the etch pits. The latter can be estimated through the variation of the separation distance between opposite sides of the pit within a given time interval (Ruiz-Agudo and Putnis 2012). We rely on pairs of consecutive images and consider all isolated (i.e., not aggregated) monolayer pits to obtain an average value, \(\overline{v}\), and the associated standard deviation. Table 1 lists the results of the evaluation of the spreading rate. As highlighted in Fig. 2, most of the mPs are short-lived. As such, the number of elements upon which \(\overline{v}\) can be evaluated decreases sharply over time, none of these structures being detected for t > 20 min. The results listed in Table 1 show that the mean and standard deviation of \(v\) monotonically decrease in time, the observed values being consistent with those documented in previous experiments concerning calcite {104} and MilliQ water (Guren et al. 2020; Harstad and Stipp 2007).
The rate of expansion of multilayer pits can be used as an additional element according to which one can estimate the spreading rate, \(v\), on the investigated surface. Even as they do not fall entirely within our observation domain (Fig. 2), these structures are more persistent than their mPs counterparts, thus enabling one to obtain at least a rough estimate of step retreat velocity throughout the whole temporal window of the experiments. The retreat velocity evaluated by considering the spreading rates of acute and obtuse edges (denoted with yellow and green lines in Fig. 2, respectively) and then averaging these over the three MPs is depicted as a function of time in Fig. 3a. Figure 3b depicts the location of the MP margins at various observation times. As opposed to what observed for the shallow pits, the trend observed for these rates is not monotonically decreasing. It increases sharply between t = 10 and 20 min, while decreasing abruptly for longer times. Consistent with the findings of Harstad and Stipp (2007), MP spreading rates are considerably larger than those listed in Table 1 for mP spreading rates.
3.3 Analysis and Statistical Modeling of Dissolution Rates
Figure 4 depicts spatial maps of rates \(R^{^{\prime}} (t_{j} ) = \frac{1}{{V_{m} }}\frac{{z^{^{\prime}} (x,y,t_{j} ) - z^{^{\prime}} (x,y,t_{j} - \Delta t)}}{\Delta t}\) (see Eq. 4) for all consecutive pairs of topography images. Analysis of these results clearly highlights that (i) values of \(R^{^{\prime}}\) associated with the MP spreading are much larger than their counterparts related to mPs expansion and step retreat across the (overall) flat terrace in between the MPs (see in particular Fig. 4c); and (ii) the largest support spanned by sample pdfs of rates is observed at an intermediate time, i.e., for \(R^{^{\prime}} (t_{4} )\). Sample probability density functions (pdfs) of \(R^{^{\prime}}\) for each of the sub-plots of Fig. 4 are depicted in Fig. 5. These pdfs exhibit common features, which have been detected also in previous studies (Bibi et al. 2018; Trindade Pedrosa et al. 2019). These include (i) the presence of a dominant peak, eventually accompanied by multiple local peaks and (ii) a positive skewness. Consistent with our discussion about Fig. 4, values of standard deviation tend to increase from t2 to t4 and then decrease for longer times.
As detailed in Sect. 2.4, we also consider the statistical characterization of the spatial increments, \(\Delta R\), which we evaluate at various separation distances between pairs of locations. For the purpose of our analysis, we consider omnidirectional increments, i.e., we set \(s \, = \left| {\mathbf{s}} \right|\), deferring the analysis of possible anisotropic behaviors to future studies. Figure 6 depicts sample pdfs of incremental data for three selected lags (s = 1, 10, and 100 dl). These distributions are generally more symmetric than their counterparts associated with \(R^{^{\prime}}\) (Fig. 5). It is clear that the shape of these pdfs varies with separation distance, their peak becoming sharper with decreasing lag, a feature which is common to corresponding results associated with a variety of other Earth science variables (see, e.g., Guadagnini et al. 2018; Siena et al. 2020 and references therein).
Sample pdfs (symbols) of rate increments, \(\Delta R(s,t_{j} )\) (j = 2, …6) evaluated at three selected lags, s. Interpretive models based on GSG distributions for incremental data (Eq. 11) are also depicted
All distributions are persistently leptokurtic, with \(\kappa_{\Delta Y}\) >> 3 even at large lags (s = 100 dl). The application of the method of moments (Eq. 15) yields estimates of the GSG model parameters that best represent the statistics associated with the distributions of the rates jointly with those of their increments. As expected, the estimated shape and scale parameters do not change significantly with the lag (not shown). The results related to the theoretical formulations of the GSG pdfs of \(R^{^{\prime}}\) and \(\Delta R(s)\) with parameters estimated through the method of moments show a remarkable agreement with their sample counterparts (see Figs. 5 and 6, respectively). As an additional element to support the analysis, we note that, while the method of moments is simple and straightforward in its application, parameter estimates obtained through maximum likelihood (ML) applied on \(R^{^{\prime}}\) and \(\Delta R(s)\) data provide results of similar quality (not shown).
Results obtained upon relying on a GEV model:
that has been adopted in previous works for the interpretation of rate spectra (Brand et al. 2017; Emmanuel 2014) are also depicted in Fig. 5 as a further term of comparison. Here, k, \(\sigma\), and \(\mu\) correspond to the shape, scale, and location parameter, respectively, and are estimated through a classical ML procedure. Visual inspection of Fig. 5 suggests that relying on a GEV model can provide results of similar quality to those that can be obtained through the GSG model with reference to sample pdfs of \(R^{^{\prime}}\). Otherwise, we note that the GEV model does not include information about the statistical behavior of incremental values, a feature which is naturally embedded in the GSG framework. The Kullback–Leibler divergence (Kullback and Leibler 1951), \(D_{KL}\), is then employed to compare quantitatively the performances of the two interpretive models. This metric can be used to measure the amount of information lost by representing the empirical distribution associated with the available data with a given theoretical model. Hence, low values of \(D_{KL}\) correspond to high degrees of similarity between sample and modeled distributions. Table 2 lists the results of this analysis, suggesting that the GSG model outperforms the GEV model for all observation times, with the exception of t4 = 20 min, where one can see that both analytical models provide a good representation of the upper tail while the GEV captures the peak of the sample pdf more closely that its GSG-based counterpart.
Figure 7 depicts the temporal behavior of estimated shape (Fig. 7a) and scale (Fig. 7b) parameters obtained for the GSG and GEV models. Shape parameters, \(\alpha\) and k, linked to the tailedness of the distributions, respectively, display a minimum and a maximum for t ≈ 15–20 min. This pattern is consistent (for k) with the one exhibited by the spreading rate of the MPs (Fig. 3a), the corresponding results documented for \(\alpha\) being characterized by a trough, mirroring the temporal behavior of k. Therefore, these results suggest that the time evolution of these structures has a major effect on the statistical distribution of \(R^{^{\prime}}\). The temporal trend exhibited by \(\sigma_{G}\) indicates an increased spreading of the distribution of rates at t4, whereas the peak of the GEV scale parameter, \(\sigma\), is attained at a later time (i.e., t5).
The evolution of the spatial correlation of rates can be inferred from the analysis of \(\rho_{G}\) vs lag at various observation times (Fig. 8). The skill of a single-parameter exponential model:
to represent the observed correlation is compared against the results obtained through two selected formulations of nested structures, whose components are associated with standard stationary variograms:
Each of these models, respectively, composed by a spherical and a Gaussian model (Eq. 18) and a spherical and a hole-effect model (Eq. 19), is defined in terms of three parameters: (i) the range of each component, a1 and a2, and (ii) the coefficient c, which determines the relative contribution (or weight) of each component. We note that these formulations appear to be more complex than the exponential model (Eq. 17). Nevertheless, we consider these due to the usefulness of nested variogram structures to interpret spatially settings where multiple processes, each characterized by their own degree of spatial persistence, can jointly contribute to the resulting heterogeneous pattern of the overall system.
The effectiveness of the models corresponding to Eqs. 17–19 is evaluated within a ML framework. While estimates of model parameters are obtained through minimization of the negative log-likelihood (NLL; see e.g., Carrera and Neuman 1986) criterion, the performance of a given model is ranked according to the well-established Kashyap (KIC) model discrimination criterion (Kashyap 1982):
Here, NP is the number of parameters associated with a given model and \(\left| {\mathbf{Q}} \right|\) is the determinant of the covariance matrix of the ML parameter estimation errors. Relying on KIC enables one to consider the quality of model fit to observations (via NLL) while jointly penalizing models with large NP and fully considering the quality of parameter estimates. The latter aspect is embedded in \(\left| {\mathbf{Q}} \right|\), which acts as a term penalizing models with small variance (i.e., large expected information content per observation) of parameter estimates (Ye et al. 2008). In this context, model ranking is performed according to increasing values of KIC, lower values of the latter corresponding to more skillful models.
Figure 8a–e depicts the values of \(\rho_{G}\) estimated at various times along the experiment together with the results obtained through the calibrated theoretical models. A qualitative inspection of these plots suggests that the nested formulations outperform the simple exponential model. This is quantitatively supported by the ML results collected in Table 3, where one can note that a nested structure composed by a spherical and a hole effect model (Eq. 19) is always ranked as best among the three models assessed. This result imbues us with confidence about the possibility to include in our interpretive model the richness of mechanisms underlying the system evolution through the superimposition of diverse correlation structures. We note that the contribution of a given component of a nested structure to acting processes can be assessed in simple large scale sedimentary settings, where these can be related to temporal sequences of depositional processes (e.g., Salamon et al. 2007 and references therein). As this is the first time, to the best of our knowledge, that these types of analyses are performed for scenarios of the kind we focus upon, disentangling the way components of nested structures can be associated with the action of mechanisms described in Sect. 2.1 and 3.1 poses significant challenges. We present preliminary interpretations in the following, noting that future works will be keyed to further support these results with additional data.
Following the results illustrated above, we focus on the parameters of the most highly ranked model (Eq. 19) and analyze their evolution in time. Figure 9a, b depict the behavior of \(\left( {c_{1} = \frac{c}{{\sigma_{G}^{2} }}, \, a_{1} } \right)\) and \(\left( {c_{2} = 1 - \frac{c}{{\sigma_{G}^{2} }}, \, a_{2} } \right)\), i.e., relative contribution and range of component 1 and 2 in Eq. (19), respectively. It can be noted that \(a_{2} > > a_{1}\) at all times, components 1 and 2 being, respectively, related to short- and long-range correlation. These results show that a1 tends to increase with time, ranging from 60 to 160 dl, indicating that short-range correlations increase as the dissolution pattern on the terraces evolves from being monolayer-pit to step retreat dominated. Otherwise, the long-range correlation parameter, a2, displays an oscillatory behavior within the range 640–800 dl, showing a steep increase only at t = t6. Length scales in this range are comparable with the distance between the multilayer pits developing on the opposite corners of the observation window. Additional evidence about a possible relationship between the dynamics of multilayer pits and the long-range component of \(\rho_{G}\) can be inferred from the analysis of parameters c1 and c2. Our results document that: (i) both components have similar weight (\(c_{1} \approx c_{2} \approx 0.5\)) at t = t1; and (ii) the long-range component becomes dominant at t4 = 20 min, its relevance decreasing for t > t4 (attaining the value c2 = 0.22 at t6 = 30 min). Comparison of these results with those depicted in Fig. 3a evidences that the relative importance of the long-range component follows the same trend displayed by the MPs spreading rate.
Figure 9 provides a clear indication supporting a conceptual picture according to which the dynamics of multilayer pits markedly and quantifiably affect the frequency distribution as well as the spatial correlation of rates. Our findings further support the benefit of relying on a modeling framework capable of jointly embedding the statistical behavior of rates and of the associated increments.
4 Conclusions
We monitor the evolution of the surface topography of a calcite crystal subjected to dissolution through in situ real-time AFM measurements. Differences between topography maps at given time intervals yields quantitative spatial distributions of dissolution rates, R', at various observation times elapsed from the renewal of the fluid (deionized water) in contact with the mineral surface. The established experimental protocol enabled us to achieve the main objective of the study, corresponding to the characterization of the spatially heterogeneous distribution of rates. The latter is assessed with a stochastic context which relies on a generalized sub-Gaussian interpretive model (Riva et al. 2015), providing a unified framework of analysis for the probability distributions of the rate (R′) and its spatial increments (\(\Delta R\)) evaluated at various separation distances (or lags).
The evolution of surface patterns evidenced by AFM images is consistent with the temporal increase of the solution saturation in a boundary layer at the fluid/solid interface. The spreading rate evaluated on monolayer pits is monotonically decreasing in time. Otherwise, the multilayer pit expansion rate is highest at an intermediate time (t = 20 min) during the experiment and then displays a steep decrease. A similar trend is also documented by (i) the shape parameter and (ii) the scale parameter of the GSG model employed for the joint characterization of the statistical behavior of R' and \(\Delta R\).
We provide qualitative and quantitative results about the relationship between the parameters of the GSG stochastic model and the dynamics of multilayer pits, documenting that the evolution of these structures significantly affects the statistical features of dissolution rates.
Relying on the Kullback–Leibler divergence metric, we find that our GSG model generally shows a higher affinity to the sample probability distribution of \(R^{^{\prime}}\) than the generalized extreme values (GEV) model which has been previously used (e.g., Brand et al. 2017). We emphasize that the GSG formulation offers the additional advantage of fully embedding the features of the probability distributions of both R' and \(\Delta R\) in a unified and consistent manner, an element which is not included in the above mentioned interpretations based on the GEV model. The adopted GSG model relies on a log-normal subordinator, which has been already tested for the interpretation of the spatial statistics of a wide range of data (e.g., Riva et al, 2015; Siena et al. 2019). We note that our theoretical framework includes the possibility of selecting a general form of the subordinator (Siena et al. 2020). The application of alternative formulations of the GSG model on dissolution rates will be the subject of future investigations.
One should also consider that the nature of the dissolution process at the microscopic level leads to the development of characteristic patterns (i.e., mono/multilayer pits or steps) whose main traits can be somehow categorized deterministically to some extent. Therefore, a synthetic generation of rate random fields purely on the basis of parameter values identified from the interpretation of sample statistics would necessarily be incomplete in some cases. In this context, Pollet-Villard et al. (2016) highlighted the importance of characterizing the spatial correlation of key variables driving mineral dissolution processes. These authors develop a numerical model to describe dissolution and ground model calibration on the comparison between sample variograms evaluated on experimental data of surface topography and its numerically based counterpart. In our work, the stochastic characterization of \(\Delta R\) data yields critical information about the spatial correlation of the rate field through the correlation function (\(\rho_{G}\)) associated with the GSG model. Among the various theoretical models analyzed for the interpretation of \(\rho_{G}\), we find that a nested structure with a short-range and a long-range correlation component (see Eq. 19) is consistently ranked as best according to rigorous model identification criteria. The temporal behavior of these characteristic length scales appears to be linked to the evolutionary dynamics of step retreat/monolayer pits and multilayer pit structures documented in the experiments. Our results reveal the impact that the diverse dissolution patterns can have on the correlation structure of reaction rates. This information can potentially lead to the development of future flexible numerical models, which can have the capability of taking into account multiple length scales resulting from the occurrence of diverse reaction mechanisms.
Availability of Data and Material
The data are available online: https://data.mendeley.com/datasets/jkthhkg9k2/draft?a=10eb0ad6-2b3f-42ad-83b2-0252a3f8c694.
Code availability
Not applicable.
References
Anabaraonye, B.U., Crawshaw, J.P., Trusler, J.P.M.: Brine chemistry effects in calcite dissolution kinetics at reservoir conditions. Chem. Geol. 509, 92–102 (2019). https://doi.org/10.1016/j.chemgeo.2019.01.014
Arvidson, R.S., Ertan, I.E., Amonette, J.E., Luttge, A.: Variation in calcite dissolution rates: geochim. Cosmochim. Acta. 67, 1623–1634 (2003). https://doi.org/10.1016/S0016-7037(02)01177-8
Bianchi Janetti, E., Dror, I., Riva, M., Guadagnini, A., Sanchez-Vila, X., Berkowitz, B.: Mobility and interaction of heavy metals in a natural soil. Transp. Porous Media. 97, 295–315 (2013). https://doi.org/10.1007/s11242-013-0125-2
Bibi, I., Arvidson, R., Fischer, C., Lüttge, A.: Temporal evolution of calcite surface dissolution kinetics. Minerals. 8, 256 (2018). https://doi.org/10.3390/min8060256
Bouissonnié, A., Daval, D., Marinoni, M., Ackerer, P.: From mixed flow reactor to column experiments and modeling: upscaling of calcite dissolution rate. Chem. Geol. 487, 63–75 (2018). https://doi.org/10.1016/j.chemgeo.2018.04.017
Brand, A.S., Feng, P., Bullard, J.W.: Calcite dissolution rate spectra measured by in situ digital holographic microscopy. Geochim. Cosmochim. Acta. 213, 317–329 (2017). https://doi.org/10.1016/j.gca.2017.07.001
Carrera, J., Neuman, S.P.: Estimation of aquifer parameters under transient and steady state conditions: 1. Maximum likelihood method incorporating prior information. Water Resour. Res. 22, 199–210 (1986). https://doi.org/10.1029/WR022i002p00199
Daval, D.: Carbon dioxide sequestration through silicate degradation and carbon mineralisation: promises and uncertainties. NPJ Mater. Degrad. 2, 11 (2018). https://doi.org/10.1038/s41529-018-0035-4
Dong, S., Berelson, W.M., Adkins, J.F., Rollins, N.E., Naviaux, J.D., Pirbadian, S., El-Naggar, M.Y., Teng, H.H.: An atomic force microscopy study of calcite dissolution in seawater. Geochim. Cosmochim. Acta. 283, 40–53 (2020). https://doi.org/10.1016/j.gca.2020.05.031
Emmanuel, S.: Mechanisms influencing micron and nanometer-scale reaction rate patterns during dolostone dissolution. Chem. Geol. 363, 262–269 (2014). https://doi.org/10.1016/j.chemgeo.2013.11.002
Erol, S., Fowler, S.J., Nehler, M., De Boever, E., Harcouët-Menou, V., Laenen, B.: An analytical algorithm of porosity–permeability for porous and fractured media: extension to reactive transport conditions and fitting via flow-through experiments within limestone and dolomite. Transp. Porous Media. 129, 343–383 (2019). https://doi.org/10.1007/s11242-019-01293-z
Fischer, C., Arvidson, R.S., Lüttge, A.: How predictable are dissolution rates of crystalline material? Geochim. Cosmochim. Acta. 98, 177–185 (2012). https://doi.org/10.1016/j.gca.2012.09.011
Fischer, C., Kurganskaya, I., Schäfer, T., Lüttge, A.: Variability of crystal surface reactivity: What do we know? Appl. Geochem. 43, 132–157 (2014). https://doi.org/10.1016/j.apgeochem.2014.02.002
Guadagnini, A., Neuman, S.P., Schaap, M.G., Riva, M.: Anisotropic statistical scaling of vadose zone hydraulic property estimates near Maricopa. Arizona. Water Resour. Res. 49, 8463–8479 (2013). https://doi.org/10.1002/2013WR014286
Guadagnini, A., Neuman, S.P., Schaap, M.G., Riva, M.: Anisotropic statistical scaling of soil and sediment texture in a stratified deep vadose zone near Maricopa. Arizona. Geoderma. 214–215, 217–227 (2014). https://doi.org/10.1016/j.geoderma.2013.09.008
Guadagnini, A., Riva, M., Neuman, S.P.: Recent advances in scalable non-gaussian geostatistics: the generalized sub-gaussian model. J. Hydrol. 562, 685–691 (2018). https://doi.org/10.1016/j.jhydrol.2018.05.001
Guren, M.G., Putnis, C.V., Montes-Hernandez, G., King, H.E., Renard, F.: Direct imaging of coupled dissolution-precipitation and growth processes on calcite exposed to chromium-rich fluids. Chem. Geol. 552, 119770 (2020). https://doi.org/10.1016/j.chemgeo.2020.119770
Harstad, A.O., Stipp, S.L.S.: Calcite dissolution: effects of trace cations naturally present in Iceland spar calcites. Geochim. Cosmochim. Acta. 71, 56–70 (2007). https://doi.org/10.1016/j.gca.2006.07.037
Heberling, F., Bosbach, D., Eckhardt, J.-D., Fischer, U., Glowacky, J., Haist, M., Kramar, U., Loos, S., Müller, H.S., Neumann, T., Pust, C., Schäfer, T., Stelling, J., Ukrainczyk, M., Vinograd, V., Vučak, M., Winkler, B.: Reactivity of the calcite–water-interface, from molecular scale processes to geochemical engineering. Appl. Geochem. 45, 158–190 (2014). https://doi.org/10.1016/j.apgeochem.2014.03.006
Hommel, J., Coltman, E., Class, H.: Porosity–permeability relations for evolving pore space: a review with a focus on (bio-)geochemically altered porous media. Transp. Porous Media. 124, 589–629 (2018). https://doi.org/10.1007/s11242-018-1086-2
Kashyap, R.L.: Optimal choice of AR and MA parts in autoregressive moving average models. IEEE Trans. Pattern Anal. Mach. Intell. (1982). https://doi.org/10.1109/TPAMI.1982.4767213
Khather, M., Saeedi, A., Myers, M.B., Giwelli, A.: Effects of CO2-saturated brine on the injectivity and integrity of chalk reservoirs. Transp. Porous Media. 135, 735–751 (2020). https://doi.org/10.1007/s11242-020-01498-7
Kristensen, R., Stipp, S.L.S., Refson, K.: Modeling steps and kinks on the surface of calcite. J. Chem. Phys. 121, 8511 (2004). https://doi.org/10.1063/1.1775771
Kullback, S., Leibler, R.A.: On information and sufficiency. Ann. Math. Stat. 22, 79–86 (1951). https://doi.org/10.1214/aoms/1177729694
Levenson, Y., Emmanuel, S.: Pore-scale heterogeneous reaction rates on a dissolving limestone surface. Geochim. Cosmochim. Acta. 119, 188–197 (2013). https://doi.org/10.1016/j.gca.2013.05.024
Liu, H.H., Molz, F.J.: Comment on “Evidence for non-Gaussian scaling behavior in heterogeneous sedimentary formations” by Scott Painter. Water Resour. Res. 33, 907–908 (1997). https://doi.org/10.1029/96WR03788
Luttge, A.: Etch pit coalescence, surface area, and overall mineral dissolution rates. Am. Mineral. 90, 1776–1783 (2005). https://doi.org/10.2138/am.2005.1734
Luttge, A., Arvidson, R.S., Fischer, C.: A stochastic treatment of crystal dissolution kinetics. Elements 9, 183–188 (2013). https://doi.org/10.2113/gselements.9.3.183
Luttge, A., Arvidson, R.S., Fischer, C., Kurganskaya, I.: Kinetic concepts for quantitative prediction of fluid-solid interactions. Chem. Geol. 504, 216–235 (2019). https://doi.org/10.1016/j.chemgeo.2018.11.016
Marinello, F., Carmignato, S., Voltan, A., Savio, E., De Chiffre, L.: Error sources in atomic force microscopy for dimensional measurements: taxonomy and modeling. J. Manuf. Sci. Eng. (2010). https://doi.org/10.1115/1.4001242
Meerschaert, M.M.: Fractional laplace model for hydraulic conductivity. Geophys. Res. Lett. 31, L08501 (2004). https://doi.org/10.1029/2003GL019320
Offeddu, F.G., Cama, J., Soler, J.M., Putnis, C.: V: Direct nanoscale observations of the coupled dissolution of calcite and dolomite and the precipitation of gypsum. Beilstein J. Nanotechnol. 5, 1245–1253 (2014). https://doi.org/10.3762/bjnano.5.138
Painter, S.: Evidence for non-Gaussian scaling behavior in heterogeneous sedimentary formations, (1996)
Pollet-Villard, M., Daval, D., Fritz, B., Knauss, K.G., Schäfer, G., Ackerer, P.: Influence of etch pit development on the surface area and dissolution kinetics of the orthoclase (001) surface. Chem. Geol. 447, 79–92 (2016). https://doi.org/10.1016/j.chemgeo.2016.09.038
Renard, F., Putnis, C.V., Montes-Hernandez, G., Ruiz-Agudo, E., Hovelmann, J., Sarret, G.: Interactions of arsenic with calcite surfaces revealed by in situ nanoscale imaging. Geochim. Cosmochim. Acta. 159, 61–79 (2015). https://doi.org/10.1016/j.gca.2015.03.025
Renard, F., Røyne, A., Putnis, C.V.: Timescales of interface-coupled dissolution-precipitation reactions on carbonates. Geosci. Front. 10, 17–27 (2019). https://doi.org/10.1016/j.gsf.2018.02.013
Riva, M., Neuman, S.P., Guadagnini, A.: Sub-gaussian model of processes with heavy-tailed distributions applied to air permeabilities of fractured tuff. Stoch. Environ. Res. Risk Assess. 27, 195–207 (2013a). https://doi.org/10.1007/s00477-012-0576-y
Riva, M., Neuman, S.P., Guadagnini, A.: New scaling model for variables and increments with heavy-tailed distributions. Water Resour. Res. 51, 4623–4634 (2015). https://doi.org/10.1002/2015WR016998
Riva, M., Neuman, S.P., Guadagnini, A., Siena, M.: Anisotropic scaling of berea sandstone log air permeability statistics. Vadose Zo. J. (2013b). https://doi.org/10.2136/vzj2012.0153
Ruiz-Agudo, E.R., Putnis, C.V.: Direct observations of mineral fluid reactions using atomic force microscopy: the specific example of calcite. Mineral. Mag. 76, 227–253 (2012). https://doi.org/10.1180/minmag.2012.076.1.227
Salamon, P., Fernàndez-Garcia, D., Gómez-Hernández, J.J.: Modeling tracer transport at the MADE site: the importance of heterogeneity. Water Resour. Res. (2007). https://doi.org/10.1029/2006WR005522
Siena, M., Guadagnini, A., Bouissonnié, A., Ackerer, P., Daval, D., Riva, M.: Generalized sub-gaussian processes: theory and application to hydrogeological and geochemical data. Water Resour. Res. (2020). https://doi.org/10.1029/2020WR027436
Siena, M., Riva, M., Giamberini, M., Gouze, P., Guadagnini, A.: Statistical modeling of gas-permeability spatial variability along a limestone core. Spat. Stat. 34, 100249 (2019). https://doi.org/10.1016/j.spasta.2017.07.007
Smith, M.E., Knauss, K.G., Higgins, S.R.: Effects of crystal orientation on the dissolution of calcite by chemical and microscopic analysis. Chem. Geol. 360–361, 10–21 (2013). https://doi.org/10.1016/j.chemgeo.2013.09.015
Teng, H.H.: Controls by saturation state on etch pit formation during calcite dissolution. Geochim. Cosmochim. Acta. 68, 253–262 (2004). https://doi.org/10.1016/S0016-7037(03)00423-X
Trindade Pedrosa, E., Kurganskaya, I., Fischer, C., Luttge, A.: A statistical approach for analysis of dissolution rates including surface morphology. Minerals. 9, 458 (2019). https://doi.org/10.3390/min9080458
Xu, M., Hu, X., Knauss, K.G., Higgins, S.R.: Dissolution kinetics of calcite at 50–70°C: an atomic force microscopic study under near-equilibrium conditions. Geochim. Cosmochim. Acta. 74, 4285–4297 (2010). https://doi.org/10.1016/j.gca.2010.04.066
Ye, M., Meyer, P.D., Neuman, S.P.: On model selection criteria in multimodel analysis. Water Resour. Res. (2008). https://doi.org/10.1029/2008WR006803
Funding
Open access funding provided by Politecnico di Milano within the CRUI-CARE Agreement. Information that explains whether and by whom the research was supported: No funds, grants, or other support was received.
Author information
Authors and Affiliations
Corresponding author
Ethics declarations
Conflicts of interest
The authors have no conflict of interest to declare.
Additional information
Publisher's Note
Springer Nature remains neutral with regard to jurisdictional claims in published maps and institutional affiliations.
Rights and permissions
Open Access This article is licensed under a Creative Commons Attribution 4.0 International License, which permits use, sharing, adaptation, distribution and reproduction in any medium or format, as long as you give appropriate credit to the original author(s) and the source, provide a link to the Creative Commons licence, and indicate if changes were made. The images or other third party material in this article are included in the article's Creative Commons licence, unless indicated otherwise in a credit line to the material. If material is not included in the article's Creative Commons licence and your intended use is not permitted by statutory regulation or exceeds the permitted use, you will need to obtain permission directly from the copyright holder. To view a copy of this licence, visit http://creativecommons.org/licenses/by/4.0/.
About this article
Cite this article
Siena, M., Bussetti, G., Recalcati, C. et al. Statistical Characterization of Heterogeneous Dissolution Rates of Calcite from In situ and Real-Time AFM Imaging. Transp Porous Med 140, 291–312 (2021). https://doi.org/10.1007/s11242-021-01624-z
Received:
Accepted:
Published:
Issue Date:
DOI: https://doi.org/10.1007/s11242-021-01624-z