Abstract
Despite the availability of newer approaches, traditional hierarchical clustering remains very popular in genetic diversity studies in plants. However, little is known about its suitability for molecular marker data. We studied the performance of traditional hierarchical clustering techniques using real and simulated molecular marker data. Our study also compared the performance of traditional hierarchical clustering with model-based clustering (STRUCTURE). We showed that the cophenetic correlation coefficient is directly related to subgroup differentiation and can thus be used as an indicator of the presence of genetically distinct subgroups in germplasm collections. Whereas UPGMA performed well in preserving distances between accessions, Ward excelled in recovering groups. Our results also showed a close similarity between clusters obtained by Ward and by STRUCTURE. Traditional cluster analysis can provide an easy and effective way of determining structure in germplasm collections using molecular marker data, and, the output can be used for sampling core collections or for association studies.
Similar content being viewed by others
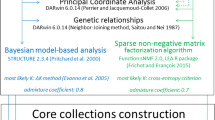
Avoid common mistakes on your manuscript.
Introduction
Information about the structure of germplasm collections is of great importance for both the conservation and utilization of genetic resources collected in genebanks. Because of the diverse nature of genebank germplasm materials (landraces, selected lines from landraces, elite breeding lines, released varieties, wild and weedy relatives of the cultigen, and genetic stocks from different areas of origin), they provide all relevant allelic diversity necessary for plant improvement. These materials are therefore very suitable for example for association studies (D’hoop et al. 2010). However, the large numbers of accessions accumulated in genebanks reduce the efficiency and effectiveness with which these genetic resources can be exploited. The approach of forming core collections (core sub-sets) was introduced to solve the above problem. Frankel (1984) defined a core collection as a limited set of accessions representing, with minimum repetitiveness, the genetic diversity of a crop species and its wild relatives. Determination of the genetic structure (partitioning) of heterogeneous germplasm collections is an essential component in the sampling of core collections since partitioning of germplasm collections before sampling ensures that both the genetic and the ecological spectra of germplasm collections are fully represented in core collections (Brown 1995; van Hintum et al. 2000). In addition, it may be necessary to associate accessions in the core collection with the entire collection; the association can be based on the group structure.
The determination of genetic structures of germplasm collections is also an important aspect of association studies (Wang et al. 2005; Shriner et al. 2007). General agreement exists among researchers that incorporating population structure into statistical models used in association mapping is necessary to avoid false positives (Pritchard et al. 2000b; Flint-Garcia et al. 2003; Zhu et al. 2008). The general model for association mapping can be written as “phenotype = marker + genotype + error”, and test for a marker effect is equivalent to testing for a QTL. Typically, genotype is a random factor whose effects are structured by kinship or population structure. This simple model can be improved by incorporating information on the relationships between the genotypes a.k.a. population structure. The relationship between phenotype and marker can be tested within the different groups (e.g. Remington et al. 2001; Simko et al. 2004) or genetic groups can be used as an extra factor or as a covariate in modeling the relationship (e.g. Thornsberry et al. 2001; Wilson et al. 2004). Yu et al. (2006) went further by introducing a mixed model approach which incorporates both population structure (Q) and kinship (K) in modeling the relationship between phenotype and marker. Another important method for incorporating population structure in association studies involves the use of principal components (Price et al. 2006).
Whether the genetic structure is needed for use in sampling core collections or for association studies, an important challenge still is the choice of a method for determining the genetic structure of germplasm collections. In the past, determination of the genetic structure of germplasm collections has mainly been done using traditional multivariate statistical methods such as cluster analysis, principal component analysis, and multidimensional scaling, usually based on agronomic data (Peeters and Martinelli 1989; Franco et al. 1997, 2005, 2006).
In recent years, many new methods have been developed especially for studying structure in natural populations using molecular markers, e.g. STRUCTURE (Pritchard et al. 2000a), PCA (Patterson et al. 2006) and PCO-MC (Reeves and Richards 2009). These methods can also be used for studying genetic structure in germplasm collections. However, traditional hierarchical clustering is still a very popular method for studying genetic diversity in crop species (see D’hoop et al 2010; Barro-Kondombo et al. 2010; Perumal et al. 2007; Folkertsma et al. 2005). Its popularity stems from the fact that it requires little computer time compared to other methods, it is available in many general statistical packages, it is frequently used in different types of applications and it is easy to understand. Moreover, it does not require genetic assumptions such as Hardy–Weinberg or linkage equilibrium. Hierarchical clustering requires decisions about the distance measure, the clustering algorithm, and the evaluation of dendrograms, amongst others. Most evaluations of the performance of hierarchical clustering methods were based on data sets of limited size (Milligan and Cooper 1985). In addition, most studies carried out to evaluate the performance of hierarchical clustering methods with respect to germplasm collections were on non-molecular marker data (Peeters and Martinelli 1989; Franco et al. 1997, 2005, 2006). We are not aware of any study in which the performance of hierarchical clustering techniques was evaluated specifically using molecular marker data. With the expected reduction in the cost of genotyping, we will be faced with datasets of thousands of accessions genotyped with several molecular markers, therefore, there is strong need to evaluate the performance of the traditional hierarchical clustering techniques using large sets of molecular marker data. The structure of genetic diversity in germplasm collections is totally different compared to natural populations. It is not clear how traditional clustering will perform under different factors affecting genetic diversity like migration and reproductive system of the materials that constitute germplasm collections. As pointed out by Mohammadi (2003), very few studies in plant genetic diversity have critically analyzed the performance of different clustering procedures especially with respect to molecular markers.
Several methods for evaluating the results of hierarchical clustering techniques exist. When performing hierarchical cluster analysis, we are interested in answering some of the following questions: (1) is there agreement between the original distances and the distances between individuals as represented by the dendrogram (2) what can the dendogram tell us about structure in the data set and (3) what is the optimum number of clusters for a given data set? One of the most popular measures of agreement between the original distances and the distances in dendrogram is the cophenetic correlation coefficient (CPCC) (Sokal and Rohlf 1962); another measure is the stress criterion of Kruskal (1964). Only a few measures for the presence of hierarchical structure can be found in the literature. Kaufman and Rousseeuw (1990) proposed the agglomerative coefficient (AC) as a criterion for measuring the amount of hierarchical structure in the data. A large number of methods have been proposed to deal with the optimum-number-of-clusters problem. A classical study is that of Milligan and Cooper (1985) who examined the performance of 30 of such criteria. Since then many criteria for determining the optimal number of clusters were introduced: the silhouette statistic (Rousseeuw 1987), Krzanowski and Lai’s index (Krzanowski and Lai 1988), the gap method (Tibshirani et al. 2001), the Clest method (Dudoit and Fridlyand 2002), the jump method (Sugar and James 2003) and the weighted gap method (Yan and Ye 2007). In general, little attention has been paid to the behavior of the above measures and methods in relation to molecular marker data from germplasm collections. A literature search indicated that so far no study tried to relate the amount of genetic structure in a germplasm collections to the performance of hierarchical cluster analysis techniques. The main objective of our study is to determine a relationship between dendogram evaluation criteria such as CPCC, AC to subgroup differentiation (genetic structure). In addition, we also compared the performance of hierarchical clustering techniques with model-based clustering methods.
In this paper, the merits of hierarchical clustering techniques for application in germplasm collections will be considered. The “Materials and methods” contains a brief description and overview of clustering techniques, the evaluation criteria and the methods used for generating simulated data. The real data set used for illustration in this paper is also described. In the results section, we present results of cluster analysis of both real and simulated data sets. We compare the results of two traditional hierarchical clustering techniques (UPGMA and Ward) with the model-based cluster analysis program STRUCTURE (Pritchard et al. 2000a), and show using simulated data how different evaluation criteria of hierarchical cluster analysis are related to subpopulation differentiation.
Materials and methods
Motivation of the study
This study was motivated by the need to study genetic diversity of several important food crops under the Generation Challenge Programme-GCP (http://www.generationcp.org). The Generation Challenge Programme is a broad network of partners from international agricultural research institutes and national agricultural research programs collectively working to improve crop productivity in the developing world, especially environments prone to drought, low soil fertility, pests and diseases. All the real data sets used in this study were generated under GCP subprogram I—Crop Genetic Diversity.
Data
Real data
The real data that will be used to illustrate methods consist of 1,014 accessions of coconut (Cocos nucifera) genotyped with 30 SSR markers. The accessions were collected from different regions of the world: West Africa (32), North America (52), South Asia (62), Latin America (72), Central America and the Caribbean (109), East Africa (124), South East Asia (183) and the Pacific Islands (380). Coconut is a diploid, mainly out-crossing species. Most of the accessions in this collection were indicated as tall; 43 dwarf accessions were present mainly from South East Asia. Dwarf coconuts have a high degree of self-fertilization. Because of its usefulness, coconut has been extensively distributed around the world. For this study, the coconut data were selected because it contained larger numbers of accessions of each of the diverse origins (a typical genebank germplasm collection).
Two additional data sets, on potato (Solanum species) and common bean (Phaseolus vulgaris), are described, analyzed and discussed in the Electronic Supplementary Material, Appendix 2. The potato data (233 accessions; 50 SSR markers) contained several unique accessions which act like outliers. All accessions used in this study are diploid. Unlike coconut and potato, common bean is a predominantly selfing species. The common bean data (603 accessions; 36 SSR markers) consist of accessions of two distinct types, Mesoamerican and Andean.
Simulated data
Marker data were simulated by SimuPOP (Peng and Kimmel 2005), a forward-time population genetic simulation environment. We used a finite island (Wright 1931) and a stepping stone (Kimura 1953) migration models. In each generation, random mating (with 2% selfing) was assumed to produce a diploid genotype for 30 unlinked loci for each individual, which had a certain probability of migrating to another subpopulation. We simulated 1,000 individuals in five subpopulations of varying subpopulation differentiation levels (differentiation between subpopulations was determined by migration rates and number of generations). The migration rates used in this study were 0, 1 and 2 migrants per subpopulation. At each of the 30 loci, the average allele frequency of coconut data was used as the starting allele frequency for the simulation. Within each parameter set, all the loci had the same mutation dynamics, which occurs according to a K-allele model (KAM). Under the KAM model, there are K possible allelic states, and any allele has a constant probability of mutating into any of the other K − 1 allelic states (Crow and Kimura 1970). A mutation rate of 2 × 10−5 with 50 possible allelic states was used in the simulation. The mutation parameters were set to mimic highly polymorphic markers such as SSR markers. However, in this case, the role of mutation is very limited since we used a limited number of generations in the simulation. In addition to using alleles from real data as starting frequencies for simulation, the numbers of generations for the simulations were restricted (from 5 to 200 generations) to mimic the situation of agricultural crops in the genebanks. Full information about the whole set of simulations is given in the Electronic Supplementary Material, Appendix 3.
Distance
In this paper, we used genetic distances (D) based on the proportion of shared alleles (PSA) where D = 1 − PSA, and
where in diploids \( f_{1ma} \) and \( f_{2ma} \) are the frequencies of allele a (a = 1, 2…A m ; A m ≤ 4) for molecular marker m (m = 1, 2…M) in individuals 1 and 2, respectively, and \( f_{1ma} ,\,f_{2ma} \, = \,0,\,{\tfrac{1}{2}}\;{\text{or}}\, 1 \). For more information on the proportion of shared alleles as similarity measure, see Bowcock et al. (1994), Chakraborty and Jin (1994) and Chang et al. (2009). The effect of distance measures on the grouping of accessions will be considered in another paper.
Clustering techniques
Hierarchical clustering techniques
From the literature on determination of the structure of plant germplasm collections, the most popular clustering methods are Unweighted Pair Group Method with Arithmetic Mean (UPGMA; (Sokal and Michener 1958)) and Ward’s method (Ward 1963). For the purpose of this study, only these two hierarchical clustering methods (hereafter referred to as UPGMA and Ward) will be discussed; both methods are well described in Kaufman and Rousseeuw (1990) and Johnson and Wichern (2002).
The differences between hierarchical clustering algorithms lie mainly in how the distances between pairs of objects or clusters are defined. In UPGMA, the distance between two clusters is defined as the unweighted mean of the distances between all pairs of accessions, one from each cluster. At each step, the two nearest clusters are joined. Ward employs analysis of variance (ANOVA) approach for calculating the distances between clusters. For each pair of clusters, the sum of squared deviations between each accession and the centre of the new cluster (error sum of squares) is calculated and the pair of clusters that yields the lowest error sum of squares is merged. In other words, at each step, in the clustering process, the effect of the union of every possible pair of clusters is considered, and the two clusters that produce the smallest increase in error sum of squares are joined. It should be noted that both UPGMA and Ward use Lance and William’s recurrence formula (Lance and Williams 1967) to operate directly on any distance matrix.
Model-based clustering techniques
The most popular model-based clustering technique is STRUCTURE (Pritchard et al. 2000a; Falush et al. 2003, 2007). STRUCTURE assumes a model with K populations; K may be unknown. It is assumed that within populations loci are in linkage equilibrium and Hardy–Weinberg equilibrium; STRUCTURE assigns individuals to populations to achieve this.
Evaluation criteria
Cophenetic Correlation Coefficient
The Cophenetic Correlation Coefficient (CPCC) is a product–moment correlation coefficient between cophenetic distances and distance matrix (input distance matrix) obtained from the data. The cophenetic distance between two accessions is defined as the distance at which two accessions are first clustered together in a dendrogram going from the bottom to the top. The CPCC, therefore, measures the relationships between the original pair wise distance between accessions (true distances) and pair wise distances between accessions predicted using the dendogram. Farris (1969) proved algebraically that among the traditional hierarchical clustering algorithms, UPGMA always produces the highest CPCC; earlier this was shown empirically by Sokal and Rohlf (1962).
Agglomerative coefficient
The Agglomerative Coefficient (AC) described by Kaufman and Rousseeuw (1990), is one of the methods proposed for quantifying hierarchical structure. The agglomerative coefficient is defined as
where d average denotes the average distance at which each object merges with one or more objects for the first time, d final is the distance at which all the objects are merged into one cluster. It is clear from the formula that AC is highly affected by the distance (d final) at the final merger of the algorithm, i.e. as long as the value of d final is high relative to d average, AC will always be close to one. The use of AC in plant diversity studies is quite limited but it has been used in other fields.
Determining the optimal number of clusters
Milligan and Cooper (1985) evaluated 30 rules for determining the optimal number of clusters. For illustration, one of the best six methods according to Milligan and Cooper (1985), the point biserial correlation, will be compared with the average silhouette coefficient proposed by Rousseeuw (1987). The two criteria were chosen because of their easy interpretation. The Point-Biserial Correlation (PBC) (Milligan 1981) is defined as the correlation between corresponding entries in the original distance matrix and a matrix consisting of zeros and ones indicating whether two objects are in the same cluster or not. This is an easy measure of the resemblance between the distance matrix and the resulting tree.
The Average Silhouette Coefficient (ASC) (Rousseeuw 1987) combines the concepts of cluster cohesion and separation; it relates distances between objects within the same cluster with distances between objects in different clusters. The silhouette coefficient (s) of an object is calculated as: \( s = (b - a)/\max (b,a),\) where a is the average distance of an object to all the objects in the same cluster and b is the minimum average distance between an object to objects in any of the other clusters.
The average silhouette coefficient for each cluster is calculated by averaging the silhouette coefficients of all the objects in the cluster. An overall measure of the quality of the clustering is obtained by computing the average silhouette coefficient over of all objects in the data. Other criteria for determining the optimum number of clusters are discussed in the supplementary material (Appendices 1, 2 and 3). In applying the criteria for determining optimum numbers of clusters, each dendrogram was cut into a specified number of clusters K (= 2, 3,…, 10) and values of the criteria for determining the number of clusters were calculated and plotted against K. For both PBC and ASC, the number of clusters (K) at which the plot of K versus the value of the criterion is maximum is considered as the optimum number of cluster for a given data set. It should be noted that all these criteria do not directly test for the presence of one cluster (K = 1).
Data analysis
Real data
After performing cluster analysis using UPGMA and Ward, CPCC and AC were calculated. The results from hierarchical cluster analysis were also compared with the results from STRUCTURE with regard to cluster composition and appropriate number of clusters.
STRUCTURE was run under the assumption of an admixture model with independent allele frequency model. No prior information was used. Calculations were carried with the number of subgroups K ranging from 2 to 10 with 3 independent repeats for each K and with 100,000 iterations of which the first 30,000 were used as burn-in.
Simulated data
In this paper the analysis of variance (ANOVA) approach (algorithm described by (Yang 1998)) and implemented in Hierfstat package in R by (Goudet 2005) was used to calculate subgroup differentiation (F ST). To explore the relationships between F ST and clustering evaluation criteria, datasets from different simulations were pooled together and then grouped based on the strength of subgroup differentiation into groups (each containing 100 datasets) with similar realized values of F ST. Hierarchical cluster analysis was performed using Agglomerative Nesting (Agnes) procedure (Kaufman and Rousseeuw 1990) of the package Cluster of R.
The ability of UPGMA and Ward to recover the subpopulations in the simulated data was evaluated using overall cluster purity (Zhao and Karypis 2004). Overall purity was calculated as follows. Let \( p_{ij} = {\frac{{m_{ij} }}{{m_{i} }}} \) be the probability that a member of cluster i (i = 1, 2,…, I) belongs in reality to subpopulation j (j = 1, 2,…., J), m ij is the number of members of subpopulationj allocated to cluster i and m i is the number of members of cluster i. The purity for each cluster (p i ) is defined as the maximum probability of correct assignment of cluster i to one of the subpopulations, i.e. \( p_{i} \, = \,\mathop {\max }\limits_{j} \left( {p_{ij} } \right), \) and over all purity is defined as \( \sum\nolimits_{i = 1}^{k} {{\frac{{m_{i} }}{m}}\,p_{i} } \).
Results
Coconut
Both dendrograms (UPGMA and Ward) resulted into two major clusters (Fig. 1), but clear differences were evident within these clusters. For example, any attempt to produce more than two clusters from each dendogram result into groups of very different structures with UPGMA resulting into highly unbalanced clusters in terms of sizes, (many of the clusters contained one or two accessions) compared to Ward. UPGMA (CPCC = 0.82) preserved the original distance matrix better than Ward (CPCC = 0.74). The two dendrograms had very different values of AC (Ward: 0.97; UPGMA: 0.58).
Dendrograms for the coconut data, a Ward, b UPGMA. Dendrograms produced by Ward and UPGMA are clearly different with respect to branching. Ward dendrogram had Cophenetic Correlation Coefficient (CPCC) of 0.74 and Agglomerative Coefficient (AC) of 0.97 while UPGMA had CPCC of 0.82 and AC of 0.58. The two major clusters in the two dendrograms had similar compositions (Accessions associated with Indian and Atlantic Oceans versus those associated with the Pacific Ocean)
When applied to the Ward dendogram, both criteria for determining the optimum number of clusters (PBC and ASC) identified two as the optimal number of clusters for the coconut data (Fig. 2a, b). However, when applied to UPGMA dendrogram, PBC was not able to identify an optimum number of clusters, i.e. changing the number clusters from 2 to 10 produced very similar correlations (Fig. 2a). STRUCTURE (method by Evanno et al. 2005) also showed two as the optimum number of clusters (see Electronic Supplementary Material, Appendix 1).
a Plot of the Point-Biserial Correlation (PBC) versus the number of groups for the UPGMA and Ward dendograms for the coconut data. b Plot of the Average Silhouette Coefficient (ASC) versus the number of groups for the UPGMA and Ward dendograms for the coconut data. For both criteria, the number of groups (K) for which the criterion is maximum (or point where the plot flattens off) indicates the optimum number of clusters. Both criteria show two as the optimum number of clusters
Composition of clusters
The two major groups identified by both UPGMA and Ward contained accessions associated with the Pacific Ocean versus accessions associated with the Atlantic and Indian oceans. These two major groups were also observed when clustering was done using STRUCTURE (K = 2) (see Fig. 3). While further subdivision obtained from Ward’s dendrogram led to formation of clusters/groups which coincided with groups based on passport data (region of origin), this was not possible with UPGMA. In terms of grouping of accessions, the results from STRUCTURE are quite similar to those of Ward. In fact, for the number of groups (K) equal two, three or four, the groups formed by STRUCTURE were almost identical to those produced by cutting Ward’s tree to produce the same number of clusters (Fig. 3). For example, by specifying (K = 3), both STRUCTURE and Ward resulted into the following three groups: (1) accessions associated with the Atlantic and Indian oceans, (2) accessions from Central America (Panama), and (3) other accessions associated with the Pacific ocean. Similarity between groups formed by STRUCTURE and Ward was also observed for the potato data (see Electronic Supplementary Material).
a Bar plots for individual coconut accessions generated by cutting the Ward dendrogram into a specified number of clusters/groups; the numbers of clusters from top to bottom were 2, 3, 4 and 5. The clusters are represented by different colors. Each column represents one accession. The labels below the bar plots indicate the regions of origin of the coconut accessions. b Bar plots for individual coconut accessions generated by STRUCTURE 2.2 using the admixture model with independent allele frequency model based on 30 SSR markers; the numbers of clusters from top to bottom were 2, 3, 4 and 5. The groups are represented by different colors. Each bar is partitioned into segments indicating its genetic composition, the longer the segment the more an accession resembles one of the groups. The labels below the bar plots indicate the regions of origin of accessions
Simulated data
The two migration models (Island and Stepping stone) yielded identical results so only the results of the Island model will be shown. The simulated data sets varied greatly with respect to subpopulation differentiation with realized FST ranging from 0.010 to 0.431. In general, the values of CPCC increased with subgroup differentiation (expressed as F ST); UPGMA produced a consistently higher CPCC than Ward (Fig. 4). The difference in CPCC between UPGMA and Ward decreased with increasing subgroup differentiation. AC also increased with subpopulation differentiation for both UPGMA and Ward (Fig. 4). In this case, Ward showed a higher AC than UPGMA; Ward reached the maximum value of one with F ST just over 0.1, i.e. the curve flattens off much quickly.
a Relationship between Cophenetic Correlation Coefficient (CPCC) and subgroup differentiation (FST) for the simulated data. b Relationship between Agglomerative Coefficient (AC) and subgroup differentiation (FST) for the simulated data. Each data point is the average of 100 datasets with similar subgroup differentiation
Identification of the optimum number of groups
Cutting of UPGMA trees resulted into highly unbalanced clusters (one or two clusters containing the majority of accessions with several other clusters with 1 or 2 accessions like in real data); only results for Ward is presented. The performance of the criteria for determining optimum number of clusters also depended on the amount of subgroup differentiation (Fig. 5). With relatively weak population differentiations (F ST < 0.08), all methods performed quite poorly in identifying the correct number of groups. At low differentiation levels, most criteria for determining optimum number of clusters gave two as the appropriate number of clusters. We also noticed that for a number of data sets with weak subgroup differentiations the values of criteria for determining optimum number of clusters either kept rising or falling, or kept fluctuating to an extent which did not allow determination of an optimum number of clusters. At higher levels of population differentiation (F ST > 0.2), the performances became similar.
From Fig. 6, it can be observed that Ward performed well in recovering the subpopulations. Except for relatively weak subpopulation differentiation (F ST < 0.05), by cutting the trees into five groups, Ward produced clusters of which over 90% of the members were from one subpopulation. The poor performance of UPGMA methods in recovering the original subpopulations, even with high subgroup differentiation, is because UPGMA produced highly unbalanced clusters.
Plot showing the difference in ability of Ward and UPGMA to recover known subgroups in the data based on cluster purity. Each point is based on 100 datasets of similar F ST values. Data sets with zero migration rates were excluded since we were mainly interested in low to medium subgroup differentiation
Discussion
This paper shows that, if used with care, traditional cluster analysis provides a simple and powerful tool for determining the genetic structure of germplasm collections using molecular marker data. Traditional cluster analysis is available in many standard statistical packages and does not require special purpose software like STRUCTURE. In addition, when clustering individual accessions, the performance of hierarchical clustering techniques depends only on subgroup differentiation, not on the migration models used to simulate the data, provided that discrete subgroups are present.
Based on our results, CPCC can be used as an indicator for the strength of subgroup differentiation. A high CPCC (\( {\text{CPCC}} \ge 0.8 \)) with both UPGMA and Ward is an indication of the presence of reliable population structure in the data. Although it has been shown theoretically and empirically that UPGMA always produce dendograms with a higher CPCC than other clustering algorithms (Farris 1969), our simulation results showed that, if distinct groups exist, the difference in CPCC between UPGMA and Ward is expected to be small and this difference gets smaller as subgroup differentiation increases. The differences in CPCC between Ward and UPGMA in real data also appear to reflect the degree of distinction between the groups in the data. For example, the common bean data with two distinct groups (Mesoamerican versus Andean) had a much smaller difference (0.07) in CPCC between Ward and UPGMA compared to potato data (0.17) with many unique accessions. For taxonomic applications (see, Rohlf 1992), it is recommended that CPCC should be very high (\( {\text{CPCC}} \ge 0.9 \)) for a dendrogram to be useful. Our results indicate that when clustering large numbers of accessions the CPCC obtained using Ward is not likely to be greater than 0.85 unless the subpopulations are highly differentiated (F ST > 0.25). This is due to the fact that Ward tends to form balanced clusters which may include outlying accessions (Jobson 1992); UPGMA tends to form unbalanced clusters assigning outlying accessions to separate clusters.
The usefulness of AC as a method for quantifying the amount of hierarchical structure in the data appears to be quite limited especially when applied to Ward. For Ward, the distance at which all clusters finally join is often much larger than the distance at which objects are joined in a cluster for the first time. All the three real data sets show very similar AC (0.97, 0.94, and 0.90 for coconut, potato and common beans respectively) with Ward but marked differences observed for UPGMA (0.58, 0.77, and 0.67 for coconut, potato and common beans respectively). Several studies in the literature have also obtained high AC values (≥0.95) with Ward and have used these results to either justify the use of Ward clustering algorithms or to conclude that there is substantial amount of structure in the data (Fan et al, 2004, Cushman et al 2010, Negro et al 2010). Based on our results which showed that Ward can result in a high AC even for a homogenous population, these conclusions can be misleading. We suggest that further modification should be made before AC can be used in conjunction with Ward. It should be noted that AC was initially proposed to describe the strength of the hierarchical structure as obtained by UPGMA (Kaufman and Rousseeuw 1990). The rather low values of AC (\( < 0.75 \)) obtained from UPGMA dendrograms even for highly differentiated subgroups could be attributed to a chaining effect (tendency of a clustering algorithm to pick out long string-like clusters (see, Johnson and Wichern (2002)) caused by outliers. UPGMA dendrograms with high CPCC but a very low AC value (<0.6) often indicate the presence of many unique accessions or small groups of accessions (together with two or more large groups). The use of CPCC and AC (only with UPGMA) together can roughly tell us the degree of fit, the presence and strength of subgroup differentiation.
The poor performance of criteria for determining the number of clusters may be explained by the presence of weak, and often subgroup differentiation found in many germplasm collections. Accessions in genebanks are no random samples but selections based on factors such as geographical distribution/location, accessibility or even perceived uniqueness. The inability of criteria to determine the optimum number of groups or clusters in a dataset is not limited to hierarchical cluster analysis techniques. Falush et al. (2003, 2007) stated that the method for determining the number of populations in STRUCTURE most often fails in real-world data sets due to various reasons (e.g. isolation by distance or inbreeding). The tendency for these criteria to show two as an optimal number clusters for the real data could be attributed to the presence of dominant groups (Evanno et al 2005; Yan and Ye 2007). In the cases where dominant groups overshadow minor subdivision, sequential detection of structure as described by Yan and Ye (2007) could offer solutions. Based on the poor performance of criteria for determining optimum number of clusters with UPGMA, it is clear that when the cluster sizes are highly unequal, as will often be the case in germplasm collections, applying criteria for determining optimum number of clusters makes little sense. In the case of association studies, one way of getting around the problem of identifying optimal number of clusters could be to use the relatedness based on cophenetic distances (predicted pair wise distances between accessions) directly to correct for population structure just like kinship or other relatedness information is used (K matrix). Studies have shown that correcting for population structure using the K matrix may be sufficient (see Zhao et al. 2007; Stich et al 2008; Astle and Balding 2009). Our analysis show a high correlation between cophenetic distances and dissimilarity between accessions based on the first two axes of principal coordinate analysis (see Elecronic supplementary material, Appendix 2). However, further study is required to assess the usefulness of cophenetic distance in association mapping studies.
Our simulation results showed that Ward was very successful in recovering the original subgroups in the data if they were present and distinctly separated. In addition, because the nature of groups formed by Ward, the dendrograms can be evaluated using standard criteria such as those for determining the number of clusters. However, in the presence of many unique or intermediate accessions the groups formed by Ward will not be homogeneous. In this case, the differences in CPCC between UPGMA and Ward can be quite helpful in deciding which method to select. In situations in which both UPGMA and Ward have high CPCC (≥0.8), Ward will have many advantages over UPGMA. However, in a situation in which only UPGMA has CPCC ≥ 0.8 and there is a big difference (>0.1) in the values of CPCC between UPGMA and Ward, it will be preferable to use the groups formed by UPGMA.
In conclusion, traditional cluster analysis (UPGMA and Ward) provides an easy and effective way for determining structure in germplasm collections. In addition to being simple to apply (using standard statistical software) and simple to interpret, it is possible to determine the presence and strength of subgroup differentiation as well as the presence and influence of unique accessions in the collection. It provides a good alternative for STRUCTURE or PCA in association analyses. It can be combined easily with mixed model facilities that are available in standard statistical packages. Although our simulations were based on random mating, similarity of results between the real data from both out-crossing (coconut and potato) and selfing species (common bean) clearly indicate that traditional cluster analysis can be applied in both mating systems.
References
Astle W, Balding DJ (2009) Population structure and cryptic relatedness in genetic association studies. Stat Sci 24(4):451–471
Barro-Kondombo C, Sagnard F, Chantereau J, vom Brocke K, Durand P, Goze′ E, Zong JD (2010) Genetic structure among sorghum landraces as revealed by morphological variation and microsatellite markers in three agroclimatic regions of Burkina Faso. Theor Appl Genet 120:1511–1523
Bowcock AM, Ruiz-Linares A, Tomfohrde J, Minch E, Kidd JR, Cavalli-Sforza LL (1994) High-resolution of human evolutionary trees with polymorphic microsatellites. Nature 368:455–457
D’hoop BB, Paulo MJ, Kowitwanich K, Senger M, Visser RGF, van Eck HJ, van Eeuwijk FA (2010) Population structure and linkage disequilibrium unravelled in tetraploid potato. Theor Appl Genet 121:1151–1170
Brown AHD (1989) Core collections—a practical approach to genetic-resources management. Genome 31:818–824
Brown AHD (1995) The core collection at the crossroads. In: Hodgkin T, Brown AHD, van Hintum TJL, Morales EAV (eds) Core collections of plant genetic resources. Wiley, Chichester, pp 3–19
Chakraborty R, Jin L (1994) Determination of relatedness between individuals using DNA-fingerprinting (VOL 65, PG 875, 1993). Human Biol 66:363
Chang WH, Chu HP, Jiang YN, Li SH, Wang Y, Chen CH, Chen KJ, Lin CY, Ju YT (2009) Genetic variation and phylogenetics of Lanyu and exotic pig breeds in Taiwan analyzed by nineteen microsatellite markers. J Anim Sci 87:1–8
Cushman SA, McKelvey KS, Noon BR, McGarigal K (2010) Use of abundance of one species as a surrogate for abundance of others. Conserv Biol 24:830–840
Crossa J, Franco J (2004) Statistical methods for classifying genotypes. Euphytica 137:19–37
Crow JF, Kimura M (1970) An introduction to population genetics theory. Harper and Row, New York
Dudoit S, Fridlyand J (2002) A prediction-based resampling method for estimating the number of clusters in a dataset. Genome Biol 3:research0036–research0036.21; doi:10.1186/gb-2002-3-7-research0036
Evanno G, Regnaut S, Goudet J (2005) Detecting the number of clusters of individuals using the software STRUCTURE: a simulation study. Mol Ecol 14:2611–2620
Falush D, Stephens M, Pritchard JK (2003) Inference of population structure using multilocus genotype data: linked loci and correlated allele frequencies. Genetics 164:1567–1587
Falush D, Stephens M, Pritchard JK (2007) Inference of population structure using multilocus genotype data: dominant markers and null alleles. Mol Ecol Notes 7:574–578
Fan JB, Yeakley JM, Bibikova M, Chudin E, Wickham E, Chen J, Doucet D, Rigault P, Zhang B, Shen R, McBride C, Li HR, Fu XD, Oliphant A, Barker DL, Chee MS (2004) A versatile assay for high-throughput gene expression profiling on universal array matrices. Genome Res 14:878–885
Farris JS (1969) On cophenetic correlation coefficients. Syst Zool 18(3):279–285
Flint-Garcia SA, Thornsberry JM, Buckler ES (2003) Structure of linkage disequilibrium in plants. Annu Rev Plant Biol 54:357–374
Folkertsma RT, Rattunde FH, Chandra S, Raju GS, Hash CT (2005) The pattern of genetic diversity of guinea-race Sorghum bicolor (L.) Moench landraces as revealed with SSR markers. Theor Appl Genet 111:399–409
Franco J, Crossa J, Villaseñor J, Taba S, Eberhart SA (1997) Classifying Mexican maize accessions using hierarchical and density search methods. Crop Sci 37:972–980
Franco J, Crossa J, Villaseñor J, Taba S, Eberhart SA (2005) A sampling strategy for conserving genetic diversity when forming core subsets. Crop Sci 45:1035–1044
Franco J, Crossa J, Warburton ML, Taba S, Eberhart SA (2006) Sampling strategies for conserving maize diversity when forming core subsets using genetic markers. Crop Sci 46:854–864
Frankel OH (1984) Genetic perspectives of germplasm conservation. In: Arber WK et al (eds) Genetic manipulation: impact on man and society. Cambridge University Press, Cambridge, pp 161–170
Goudet J (2005) HIERFSTAT, a package for R to compute and test hierarchical F-statistics. Mol Ecol 5:184–186
Gouesnard B, Bataillon TM, Decoux G, Rozale C, Schoen DJ, David JL (2001) MSTRAT: an algorithm for building germ plasm core collections by maximizing allelic or phenotypic richness. J Hered 92:93–94
Gower JC (1973) Classification problems. Bull Int Stat Inst 45:471–477
Jansen J, van Hintum TJL (2007) Genetic distance sampling: a novel sampling method for obtaining core collections using genetic distances with an application to cultivated lettuce. Theor Appl Genet 114:421–428
Jobson JD (1992) Applied multivariate data analysis, vol 2. Categorical and multivariate methods. Springer, New York
Johnson AR, Wichern DW (2002) Applied multivariate statistical analysis, 5th edn. Prentice Hall, New Jersey
Kaufman L, Rousseeuw PJ (1990) Finding groups in data. An introduction to cluster analysis. Wiley, New York
Kim KW, Chung HK, Cho GT, Ma KH, Chandrabalan D, Gwag JG, Kim TS, Cho EG, Park YJ (2007) PowerCore: a program applying the advanced M strategy with a heuristic search for establishing core sets. Bioinformatics 23:2155–2162
Kimura M (1953) “Stepping Stone” model of population. Ann Rept Nat Inst Genet Jpn 3:62–63
Kruskal JB (1964) Nonmetric multidimensional-scaling—a numerical method. Psychometrika 29:115–129
Krzanowski WJ, Lai YT (1988) A criterion for determining the number of groups in a data set using sum-of-squares clustering. Biometrics 44:23–34
Lance GN, Williams WT (1967) A general theory of classificatory sorting strategies I. Hierarchical system. Comput J 9:373–380
Lee C, Abdool A, Huang CH (2009) PCA-based population structure inference with generic clustering algorithms. BMC Bioinform 10(Suppl 1):S73
Milligan GW (1981) A Monte Carlo study of thirty internal criterion measures for cluster Analysis. Psychometrika 46:187–199
Milligan GW, Cooper MC (1985) An examination of procedures for determining the number of clusters in a data set. Psychometrika 50:159–179
Mohammadi SA (2003) Analysis of genetic diversity in crop plants—salient statistical tools and considerations. Crop Sci 43:1235–1248
Negro SS, Caudron AK, Dubois M, Delahaut P, Gemmell NJ (2010) Correlation between male social status, testosterone levels, and parasitism in a dimorphic polygynous mammal. PLoS ONE 5(9):e12507. doi:10.1371/journal.pone.0012507
Patterson N, Price AL, Reich D (2006) Population structure and eigen analysis. Plos Genet 2:e190
Peeters JP, Martinelli JA (1989) Hierarchical cluster analysis as a tool to manage variation in germplasm collections. Theor Appl Genet 78:42–48
Peng B, Kimmel M (2005) SimuPOP: a forward-time population genetics simulation environment. Bioinformatics 21:3686–3687
Perumal R, Krishnaramanujam R, Menz MA, Katile S, Dahlberg J, Magill CW, Rooney WL (2007) Genetic diversity among sorghum races and working groups based on AFLPs and SSRs. Crop Sci 47:1375–1383
Price A, Patterson N, Plenge R, Weinblatt M, Shadick N, Reich D (2006) Principal components analysis corrects for stratification in genome-wide association studies. Nat Genet 38:904–909
Pritchard JK, Stephens M, Donnelly P (2000a) Inference of population structure using multilocus genotype data. Genetics 155:945–959
Pritchard JK, Stephens M, Rosenberg NA, Donnelly P (2000b) Association mapping in structured populations. Am J Hum Genet 67:170–181
Reeves PA, Richards CM (2009) Accurate inference of subtle population structure (and other genetic discontinuities) using principal coordinates. PLoS ONE 4:e4269
Remington DL, Thornsberry JM, Matsuoka Y, Wilson LM, Whitt SR, Doebley J, Kresovich S, Goodman MM, Buckler ES (2001) Structure of linkage disequilibrium and phenotypic associations in the maize genome. Proc Natl Acad Sci USA 98:11479–11484
Roger KB (1976) Mixture model tests for cluster analysis: accuracy of four agglomerative hierarchical methods. Psychol Bull 83:377–388
Rohlf FJ (1992) NTSYS-pc (Numerical Taxonomy and Multivariate Analysis System). Version 1.70. Exeter, Setauket
Rousseeuw PJ (1987) Silhouettes: a graphical aid to the interpretation and validation of cluster analysis. J Comput Appl Math 20:53–65
Shriner D, Vaughan LK, Padilla MA, Tiwari HK (2007) Problems with genome-wide association studies. Science 316:1840–1842
Simko I, Haynes KG, Ewing EE, Costanzo S, Christ BJ, Jones RW (2004) Mapping genes for resistance to Verticillium albo-atrum in tetraploid and diploid potato populations using haplotype association tests and genetic linkage analysis. Mol Genet Genom 271:522–531
Sokal RR, Michener C (1958) A statistical method for evaluating systematic relationships. Univ Kansas Sci Bull 38:1409–1438
Sokal RR, Rohlf FJ (1962) The comparison of dendrograms by objective methods. Taxon 11:33–40
Stich B, Möhring J, Hans-Peter Piepho, Heckenberger M, Buckler ES, Melchinger AE (2008) Comparison of mixed-model approaches for association mapping. Genetics 178:1745–1754
Sugar CA, James GM (2003) Finding the number of clusters in a dataset: an information-theoretic approach. J Am Stat Assoc 98:750–763
Thachuk C, Crossa J, Franco J, Dreisigacker S, Warburton M, Davenport GF (2009) Core Hunter: an algorithm for sampling genetic resources based on multiple genetic measures. BMC Bioinform 10:243
Thornsberry JM, Goodman MM, Doebley J, Kresovich S, Nielsen D, Buckler ES (2001) Dwarf8 polymorphisms associate with variation in flowering time. Nat Genet 28:286–289
Tibshirani R, Walther G, Hastie T (2001) Estimating the number of clusters in a data set via the gap statistic. J R Stat Soc B 63:411–423
van Hintum TJL, Brown AHD, Spillane C, Hodgkin T (2000) Core collections of plant genetic resources. IPGRI Technical Bulletin No.3. International Plant Genetic Resources Institute, Rome, Italy
Wang WYS, Barrat BJ, Clayton GG, Todd JA (2005) Genome-wide association studies: theoretical and practical concerns. Nat Rev Genet 6:109–118
Ward JH (1963) Hierarchical groupings to optimize an objective function. J Am Stat Assoc 58:236–244
Wilson LM, Whitt SR, Ibanez AM, Rocheford TR, Goodman MM, Buckler ESIV (2004) Dissection of maize kernel composition and starch production by candidate gene association. Plant Cell 16:2719–2733
Wright S (1931) Evolution in Mendelian populations. Genetics 16:97–159
Yan M, Ye K (2007) Determining the number of clusters using the weighted gap statistic. Biometrics 63:1031–1037
Yang R (1998) Estimating hierarchical F-statistics. Evolution 52:950–956
Yu J, Pressoir G, Briggs WH, Vroh BI I, Yamasaki M, Doebley JF, McMullen MD, Gaut BS, Nielsen DM, Holland JB, Kresovich S, Buckler ES (2006) A unified mixed-model method for association mapping that accounts for multiple levels of relatedness. Nat Genet 38:203–208
Zhao K, Aranzana MJ, Kim S, Lister C, Shindo C, Tang C, Toomajian C, Zheng H, Dean C, Marjoram P, Nordborg M (2007) An Arabidopsis example of association mapping in structured samples. PLoS Genet 3:e4
Zhao Y, Karypis G (2004) Empirical and theoretical comparisons of selected criterion functions for document clustering. Mach Learn 55:311–331
Zhu C, Gore M, Buckler ES, Yu J (2008) Status and prospects of association mapping in plants. Plant Genom 1:5–20
Acknowledgments
We thank two anonymous reviewers for their critical review of the manuscript. This work was supported by the Generation Challenge Programme under GCP subprogram I—Crop Genetic Diversity. We would like to thank various people who participated in generating the data used in this study especially Carmen de Vicente, Patricia Lebrun-Turquay (PI-coconut), Matthew Blair (PI-Common bean) and Marc Ghislain (PI-Potato).
Open Access
This article is distributed under the terms of the Creative Commons Attribution Noncommercial License which permits any noncommercial use, distribution, and reproduction in any medium, provided the original author(s) and source are credited.
Author information
Authors and Affiliations
Corresponding author
Additional information
Communicated by M. Frisch.
Electronic supplementary material
Below is the link to the electronic supplementary material.
Rights and permissions
Open Access This is an open access article distributed under the terms of the Creative Commons Attribution Noncommercial License (https://creativecommons.org/licenses/by-nc/2.0), which permits any noncommercial use, distribution, and reproduction in any medium, provided the original author(s) and source are credited.
About this article
Cite this article
Odong, T.L., van Heerwaarden, J., Jansen, J. et al. Determination of genetic structure of germplasm collections: are traditional hierarchical clustering methods appropriate for molecular marker data?. Theor Appl Genet 123, 195–205 (2011). https://doi.org/10.1007/s00122-011-1576-x
Received:
Accepted:
Published:
Issue Date:
DOI: https://doi.org/10.1007/s00122-011-1576-x