Abstract
This paper introduces a novel sampling method for obtaining core collections, entitled genetic distance sampling. The method incorporates information about distances between individual accessions into a random sampling procedure. A basic feature of the method is that automatically larger samples are obtained if accessions are further apart and smaller samples if accessions are closer together. Genetic distance sampling can be used in conjunction with predefined stratifications of the accessions. Sample sizes are determined automatically; they depend on the distances between accessions within strata. The method is applied to the collection of cultivated lettuce of the Centre for Genetic Resources, the Netherlands. In this paper, genetic distances between accessions are obtained using AFLP marker data. However, genetic distance sampling can be applied using any measure of genetic distance between accessions. Some properties of genetic distance sampling are discussed.




Similar content being viewed by others
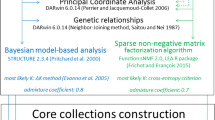
References
Bretting PK, Widrlechner MP (1995) Genetic markers and plant genetic resource management. Plant Breed Rev 31:11–86
Brown AHD (1989) Core collections: a practical approach to genetic resources management. Genome 31:818–823
Brown AHD (1995) The core collection at the crossroads. In: Hodgkin T, Brown AHD, van Hintum ThJL, Morales EAV (eds) Core collections of plant genetic resources. Wiley, Chichester, pp 3–19
Brown AHD, Schoen DJ (1994) Optimal strategies for core collections of plant genetic resources. In: Loeschcke V, Tomiuk J, Jain SK (eds) Conservation genetics. Birkhäuser, Basel, pp 357–370
Charmet G, Balfourier F (1995) The use of geostatistics for sampling a core collection of perennial ryegrass populations. Genet Resour Crop Evol 42:303–309
Franco J, Crossa J, Taba S, Shands H (2005) A sampling strategy for conserving genetic diversity when forming core subsets. Crop Sci 45:1035–1044
Frankel OH (1984) Genetic perspectives of germplasm conservation. In: Arber W, Llimensee K, Peacock WJ, Starlinger P (eds) Genetic manipulation: impact on man and society. Cambridge University Press, Cambridge, pp 161–170
Genstat Committee (2005) Genstat® Release 8. Reference manual. VSN International, Hemel Hempstead
Gouesnard B, Bataillon TM, Decoux G, Rozale C, Schoen DJ, David JL (2001) MSTRAT: an algorithm for building germplasm core collections by maximizing allelic or phenotypic richness. J Hered 92:93–94
Gower JC (1971) A general coefficient of similarity and some of its properties. Biometrics 27:857–871
van Hintum ThJL (2003) Molecular characterisation of a lettuce germplasm collection. In: Eucarpia leafy vegetables 2003, Proceedings of the Eucarpia meeting on leafy vegetables genetics and breeding, Noordwijkerhout, The Netherlands, 19–21 March 2003. Centre for Genetic Resources, Wageningen, pp 99–104
van Hintum ThJL van Treuren R (2002) Molecular markers: tools to improve genebank efficiency. Cell Mol Biol Lett 7:737–744
van Hintum ThJL, Brown AHD, Spillane C, Hodgkin T (2000) Core collections of plant genetic resources. International Plant Genetic Resources Institute, Rome
Jansen J, Verbakel H, Peleman J, van Hintum ThJL (2006) A note on the measurement of genetic diversity within genebank accessions of lettuce (Lactuca sativa L.) using AFLP markers. Theor Appl Genet (in press)
Marita JM, Rodriguez JM, Nienhuis J (2000) Development of an algorithm identifying maximally diverse core collections. Genet Resour Crop Evol 47:515–526
Schoen DJ, Brown AHD (1993) Conservation of allelic richness in wild crop relatives is aided by assessment of genetic markers. Proc Natl Acad Sci USA 90:10623–10627
Schoen DJ, Brown AHD (1995) Maximising genetic diversity in core collections of wild relatives of crop species. In: Hodgkin T, Brown AHD, van Hintum ThJL, Morales EAV (eds) Core collections of plant genetic resources. Wiley, Chichester, pp 55–76
Vos P, Hogers R, Bleeker M, Reijans M, van de Lee T, Hornes M, Frijters A, Pot J, Peleman J, Kuiper M, Zabeau M (1995) AFLP: a new technique for DNA fingerprinting. Nucleic Acids Res 23:4407–4414
Acknowledgments
The authors are very grateful to the editor and two referees, whose comments led to various improvements of the paper.
Author information
Authors and Affiliations
Corresponding author
Additional information
Communicated by A. Charcosset.
Appendix
Appendix
In order to provide a mathematical description of the relationship between the average band frequencies of AFLP markers in samples obtained using genetic distance sampling (y) and the corresponding band frequencies in the entire collection (x) a third-order polynomial was used:
This function provides enough flexibility to describe the relationship between y and x. Since the band frequency of non-polymorphic AFLP markers remain unchanged under any sampling procedure, f(0) = 0, leading to δ = 0, and f(1) = 1, leading to γ = 1 − α − β. As a consequence,
Since the simple matching coefficient treats the presence of bands in the same way as the absence of bands, it follows that f(1/2) = 1/2, leading to β = −3/2 α. As a consequence,
In order to achieve that the function f(x) is non-decreasing, the value of α should be smaller or equal to 4. If α is positive (negative), the slope of f(x) is greater (smaller) than unity if x is either close to 0 or 1.
Using the least-squares criterion, the function g(x) = f(x) − x can be fitted to the data by simple linear regression with zero intercept. For the data used in this study expression (1) did not provide a significantly better fit to the data compared to expression (2). Therefore, only results using expression (2) have been presented. It would also be possible to use weighted linear regression with weights proportional to 1/x(1 − x). This would give more weight to points with x close to 0 or 1 in comparison to points with x close to 1/2. For the current data this leads to even larger estimates of α.
Rights and permissions
About this article
Cite this article
Jansen, J., van Hintum, T. Genetic distance sampling: a novel sampling method for obtaining core collections using genetic distances with an application to cultivated lettuce. Theor Appl Genet 114, 421–428 (2007). https://doi.org/10.1007/s00122-006-0433-9
Received:
Accepted:
Published:
Issue Date:
DOI: https://doi.org/10.1007/s00122-006-0433-9