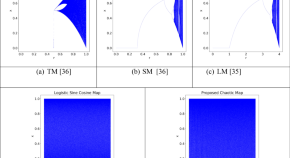
Collection
S.I. - Multi-modal Transformers
- Submission status
- Closed
Submission Guidelines: Authors should prepare their manuscript according to the Instructions for Authors available from the Multimedia Systems website. Authors should submit through the online submission site at Multimedia Systems and select “S.I. - Multi-modal Transformers" when they reach the “Article Type” step in the submission process. Submitted papers should present original, unpublished work, relevant to the topics of the special issue. All submitted papers will be evaluated on the basis of relevance, significance of contribution, technical quality, scholarship, and quality of presentation, by at least three independent reviewers. It is the policy of the journal that no submission, or substantially overlapping submission, be published or be under review at another journal or conference at any time during the review process. Final decisions on all papers are made by the Editor in Chief.
Editors
-
Feifei Zhang
Feifei Zhang is currently a professor at the School of Computer Science and Engineering, Tianjin University of Technology. Her research interests include multimedia content analysis, understanding, and applications, especially crossmodal image retrieval, visual question answering, and image captioning. She has authored or co-authored over 20 academic papers in international conferences and journals, including IEEE TIP, IEEE TMM, IEEE TCSVT, ACM TOMM, IEEE CVPR, and ACM MM.
-
An-An Liu
Dr. An-An Liu is currently a professor in the School of Electronic Information Engineering, Tianjin University, China, and the director of Institute of Image Information & Television, Ministry of Education. He used to be a visiting professor in the School of Computing, National University of Singapore, working with Prof. Mohan Kankanhalli, and the visiting scholar in the Robotics Institute, Carnegie Mellon University, working with Prof. Takeo Kanade. He respectively received his B.E. and Ph.D. degrees from Tianjin University, China, in 2005 and 2010. His research interests include cross-media computing and machine learning.
-
Xiaoshan Yang
Xiaoshan Yang received Ph.D. degree in pattern recognition and intelligent systems from Institute of Automation, Chinese Academy of Sciences in 2016. He is currently an Associate Professor with the Institute of Automation, Chinese Academy of Sciences. His research focuses on data-driven and knowledge-guided multimedia content understanding. He has authored or co-authored more than 50 journal/conference papers, most of them are IEEE/ACM transactions or CCF-A conferences, e.g., IEEE TMM, IEEE TIP, IEEE TCYB, ACM TOMM, IEEE CVPR, ACM MM and AAAI.
-
Min Xu
Dr. Min Xu is an Associate Professor at the School of Electrical and Data Engineering (SEDE), Faculty of Engineering and Information Technology (FEIT), University of Technology Sydney (UTS). She is currently the Leader of Visual and Aural Intelligence Laboratory within the Global Big Data Technologies Center (GBDTC) at UTS. Dr. Xu is a researcher in the fields of multimedia, computer vision and machine learning. She has published 170+ research papers in prestigious international journals and conferences, including IEEE T-PAMI, IEEE T-NNLS, IEEE T-MM, IEEE T-MC, PR, ICLR, CVPR, ICCV, ACM MM, AAAI and so on.
Articles (16 in this collection)
-
-
PointCMC: cross-modal multi-scale correspondences learning for point cloud understanding
Authors (first, second and last of 4)
- Honggu Zhou
- Xiaogang Peng
- Zizhao Wu
- Content type: Special Issue Paper
- Published: 30 April 2024
- Article: 138
-
Personalized time-sync comment generation based on a multimodal transformer
Authors
- Hei-Chia Wang
- Martinus Maslim
- Wei-Ting Hong
- Content type: Special Issue Paper
- Published: 30 March 2024
- Article: 105
-
GVA: guided visual attention approach for automatic image caption generation
Authors (first, second and last of 4)
- Md. Bipul Hossen
- Zhongfu Ye
- Md. Imran Hossain
- Content type: Special Issue Paper
- Published: 29 January 2024
- Article: 50
-
HCNNet: hybrid convolution neural network for automatic identification of ischaemia in diabetic foot ulcer wounds
Authors
- Sujit Kumar Das
- Suyel Namasudra
- Arun Kumar Sangaiah
- Content type: Special Issue Paper
- Published: 22 January 2024
- Article: 36
-
Yolov5s-MSD: a multi-scale ship detector for visible video image
Authors (first, second and last of 4)
- Yan-Tong Chen
- Yan-Yan Zhang
- Yang Liu
- Content type: Special Issue Paper
- Published: 12 January 2024
- Article: 3
-
A comprehensive survey on deep-learning-based visual captioning
Authors (first, second and last of 9)
- Bowen Xin
- Ning Xu
- An-An Liu
- Content type: Special Issue Paper
- Published: 21 September 2023
- Pages: 3781 - 3804
-
CTNet: hybrid architecture based on CNN and transformer for image inpainting detection
Authors
- Fengjun Xiao
- Zhuxi Zhang
- Ye Yao
- Content type: Special Issue Paper
- Published: 19 September 2023
- Pages: 3819 - 3832
-
Images denoising for COVID-19 chest X-ray based on multi-scale parallel convolutional neural network
Authors (first, second and last of 4)
- Noor Ahmed
- Rozina
- Abdul Raziq
- Content type: Special Issue Paper
- Published: 11 September 2023
- Pages: 3877 - 3890
-
Identification of haploid and diploid maize seeds using hybrid transformer model
Authors (first, second and last of 6)
- Emrah Dönmez
- Serhat Kılıçarslan
- Abdullah Elen
- Content type: Special Issue Paper
- Published: 05 September 2023
- Pages: 3833 - 3845
-
LET-Net: locally enhanced transformer network for medical image segmentation
Authors (first, second and last of 4)
- Na Ta
- Haipeng Chen
- Nuo Jin
- Content type: Special Issue Paper
- Open Access
- Published: 05 September 2023
- Pages: 3847 - 3861
-
Variable bit allocation method based on meta-heuristic algorithms for facial image compression
Authors
- Reza Khodadadi
- Gholamreza Ardeshir
- Hadi Grailu
- Content type: Special Issue Paper
- Published: 05 September 2023
- Pages: 3903 - 3930
-
Inceptr: micro-expression recognition integrating inception-CBAM and vision transformer
Authors
- Haoliang Zhou
- Shucheng Huang
- Yuqiao Xu
- Content type: Special Issue Paper
- Published: 31 August 2023
- Pages: 3863 - 3876
-
Asymmetric bi-encoder for image–text retrieval
Authors (first, second and last of 4)
- Wei Xiong
- Haoliang Liu
- Yu Zhang
- Content type: Special Issue Paper
- Published: 26 August 2023
- Pages: 3805 - 3818
-
View-target relation-guided unsupervised 2D image-based 3D model retrieval via transformer
Authors
- Jiacheng Chang
- Lanyong Zhang
- Zhuang Shao
- Content type: Special Issue Paper
- Open Access
- Published: 24 August 2023
- Pages: 3891 - 3901
-
Learning intra-inter-modality complementary for brain tumor segmentation
Authors (first, second and last of 5)
- Jiangpeng Zheng
- Fan Shi
- Congcong Wang
- Content type: Special Issue Paper
- Published: 16 July 2023
- Pages: 3771 - 3780