Abstract
Background
Danhong injection (DHI), which is one of the most well-known Traditional Chinese Medicine (TCM) injections, widely used to treat unstable angina (UA). However, its underlying pharmacological mechanisms need to be further clarified.
Methods
In the present study, network pharmacology was adopted. Firstly, the relative compounds were obtained by a wide-scaled literatures-mining and potential targets of these compounds by target fishing were collected. Then, we built the UA target database by DisGeNET, DigSee, TTD, OMIM. Based on data, protein-protein interaction (PPI) analysis, GO and KEGG pathway enrichment analysis were performed and screen the hub targets by topology. Furthermore, evaluation of the binding potential of key targets and compounds through molecular docking.
Results
The results showed that 12 ingredients of DHI and 27 putative known therapeutic targets were picked out. By systematic analysis, identified 4 hub targets (TNF, TLR4, NFKB1 and SERPINE1) mainly involved in the complex treating effects associated with coagulation and hemostasis, cell membrane region, platelet alpha granule, NF-kappa B signaling pathway and TNF signaling pathway.
Conclusion
The results of this study preliminarily explained the potential targets and signaling pathways of DHI in the treatment of UA, which may help to laid a good foundation for experimental research and further clinical application.
Similar content being viewed by others
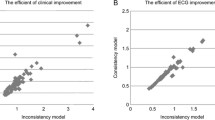
Background
According to American Heart Association statistics, cardiovascular and cerebrovascular diseases are the leading causes of death, imposing immense health, financial and emotional burdens on the world [1, 2]. UA, ST-segment elevation myocardial infarction (STEMI) and non–ST-segment elevation myocardial infarction (NSTEMI) have been collectively described acute coronary syndromes (ACS) [3]. However, although UA has clinical evidence of myocardial ischemia, the key characteristic is without significant myocardial injury [4,5,6]. Previous studies have shown that the pathogenesis of unstable angina is mainly related to platelet activation and aggregation and the inflammatory response-induced decline in the stability of atherosclerotic plaque [7, 8]. Generally, UA is usually controlled by antiplatelet medications, antithrombin, antianginal and thrombolytic therapy in clinic [3, 9]. For example, as a thiophene pyridine derivative, clopidogrel can block the activation of P2Y12 adenosine diphosphate (ADP) receptor on platelets and effectively diminish platelet aggregation [10]. Nevertheless, if patients had received clopidogrel within 5 days before undergoing coronary artery bypass grafting (CABG), the risk of major bleeding will increase [11].
TCM is a clinically proven medical practice that has been in existence for thousands of years [12,13,14]. DHI is a mixed extraction of Salvia miltiorrhiza (DS; Dan Shen; family: Lamiaceae) and Carthami Flos (HH; Hong Hua; family: Compositae /Asteraceae) [15,16,17]. DHI has been widely used as an important adjuvant for the treatment of cardiovascular and cerebrovascular diseases in China [14, 18, 19]. Even more importantly, a previous study demonstrated that DHI combined with conventional medicines could improve the electrocardiogram and reduce the symptoms of angina for the treatment of UA [20].
Network pharmacology with systematic and holistic characteristics has become a promising method to explain the complex interactions between herbs and diseases at the system level [21,22,23]. To summarize, this study aimed to identify the potential targets and pathways of DHI as a therapy against UA using the network pharmacology approach, and systematically elucidate the mechanism of DHI in the treatment of UA. The detailed workflow was shown in Fig. 1.
Methods
Identification of DHI compound
To collect the chemical ingredients of DHI, PubMed (https://www.ncbi.nlm.nih.gov/pubmed/), and China National Knowledge Infrastructure Database (CNKI, http://www.cnki.net/) were applied. Furthermore, the PubChem [24] (https://pubchem.ncbi.nlm.nih.gov/), and ChemDraw [25] (http://www.chemdraw.com.cn/) was used to find Canonical simplified molecular input line entry specification (SMILES) information of the compounds.
Screening compound targets for DHI
We searched the SuperPred [26] (http://prediction.charite.de/), SwissTargetPrediction [27] (http://www.swisstargetprediction.ch/) and BATMAN-TCM [28] (http://bionet.ncpsb.org/batman-tcm/) for potential targets related to DHI compounds. What’s more, the UniProt [29] (http://www.uniprot.org/) was utilized to convert the protein name of the DHI bioactive ingredients to the gene names. The search results were filtered to retain only studies conducted on “Homo sapiens (Human)” so that the names can be standardized and data duplicates deleted.
Collection of target proteins associated with UA
Upload “Unstable angina”, “UA”, and “Unstable angina Pectori” as search terms to the DigSee (http://210.107.182.61/geneSearch/) [30], DisGeNET (http://www.disgenet.org/search) [31], OMIM (https://omim.org/) [32] and Therapeutic Target Database (TTD, https://db.idrblab.org/ttd/) [33]. In addition, the species was set to “Homo sapiens (Human)”. The intersection between the UA-related human gene and the target gene of the active compound was retained for further analysis.
Protein-protein interaction (PPI) network construction
The names of putative UA/compound targets were submitted to STRING 11.0 database [34] (https://string-db.org/) as a central protein, which stores information about protein interactions. Only “Homo sapiens” proteins with the confidence score higher than 0.7 were picked out.
Network establishment and module analysis
To characterize the therapeutic mechanisms of DHI against UA from a network target perspective, the Cytoscape 3.7.1 [35] (https://cytoscape.org/) were employed to construct six visualization networks as follows: (1) DHI compound-predicted target network; (2) UA-associated target network; (3) Compound- DHI/UA putative therapeutic target network; (4) DHI-UA PPI network; (5) Module analysis network; (6) Drug- key compounds- hub targets-pathways network. The “degree” is regarded as the number of edges connected to it [21, 36]. The “edges” stand for the interaction, association, or any other well-defined relationship [37]. Moreover, the “betweenness” indicates the amount of shortest paths that go through a given node [38, 39]. Besides, the “closeness” emblematizes the inverse of the sum of the distances from one node to the other [40, 41]. The higher the quantitative value of a node’s network parameters, such as degree, betweenness, and closeness, the more important the node is.
Molecular Complex Detection (MCODE) algorithms can find dense regions of interaction in PPI networks based on complex connection data [42]. In the present study, we identified the dense regions of DHI-UA PPI network according to the default parameters of MCODE (Degree Cutoff = 2; Node Score Cutoff = 0.2; K-Core = 2; Max. Depth = 100) [43]. Whereafter, the hub genes in each significant module were further analyzed.
Functional enrichment analysis
To evaluate the role of potential core targets by bioinformatic annotation, the R 3.6.1 software with the Bioconductor package was manipulated, including Gene Ontology (GO) knowledgebase (http://geneontology.org/), Kyoto Encyclopedia of Genes and Genomes (KEGG) pathway enrichment analysis (https://www.genome.jp/kegg/) [44,45,46].
Molecular docking simulation
Initially, AutoDockTools 1.5.6 was employed to set the number of rotatable bonds for 12 small molecule compounds obtained [47]. Subsequently, collecting the protein conformation is performed in the Protein Data Bank database (PDB, https://www.rcsb.org/) [48]. The screening conditions were set as follows: (1) the protein structure is obtained by X-crystal diffraction; (2) the crystal resolution of the protein is less than 3 Å; (3) preferential selection of protein structures reported in the literature of molecular docking; (4) the organism comes from Homo sapiens. Based on the above conditions, a total of 11 core target protein PDB IDs were gathered. At the same time, the Notepad++ (https://notepad-plus-plus.org/) and AutoDockTools were applied to not only remove water molecules and pro-ligand small molecules, but also hydrogenate and charge. Finally, molecular docking calculations were performed using Autodock Vina 1.1.2 [49]. The PyMol 2.3.2 (https://pymol.org/2/) software were wielded to visualize the docking results [50, 51].
Results
Compound- putative target network
After deleting duplicate data, a total of 12 major compounds were collected as candidate compounds (Table 1) [52]. And all of the chemical constituents of DHI in Table S1. After supplementing and eliminating the targets obtained, a total of 372 compound-associated targets were identified. By analyzing the DHI compound- predicted target network, we found that the number of nodes was 384 (12 compound nodes, 372 compound-associated target nodes), and the number of edges was 708 (Fig. 2(a)). As shown in Fig. 2(a), a single target can be co-regulated by a variety of compounds to trigger the biological effects, which may play a vital role in treating UA. For example, CA12 were modulated by Rosmarinic acid, Salvianolic acid B and so on.
Analysis of the active compounds of DHI and putative DHI-UA targets. (a) Compound- predicted target network of DHI. (b) The UA targets’ network plotting. (c) Compound- DHI/UA putative therapeutic target network plotting. The size of the nodes is directly proportional to the degree of the nodes. (The software of Cytoscape 3.7.1 was used to generate the figure)
UA targets’ network and DHI-UA PPI network
There are 194 targets related to UA retrieved from DigSee, DisGeNet, OMIM and TTD database, which uploaded to the Cytoscape for network mapping (Fig. 2(b)). The UA targets’ network was constructed of 195 nodes (1 UA nodes, 194 UA target nodes) and 194 edges. Furthermore, by intersecting the two networks of Fig. 2(a) and Fig. 2(b), 27 DHI/UA putative therapeutic targets were obtained (Fig. 2(c, d)). Then, these targets brought in the STRING database to set up the PPI network (Fig. 3(a)). The network had 66 nodes, which interacted with 340 edges. From yellow to purple, the degree was increasing, and thicker edges denoted the stronger interactions. According to average degree value > 10, average betweenness > 0.024 and average closeness > 0.44, a total of 8 potential core targets were procured (Table 2). Based on topological analysis, the results together indicated that the top mutual target proteins had multiple beneficial biological functions for treating UA at the molecular level.
Analysis of DHI-UA PPI network and functional enrichment analysis. (a) DHI-UA PPI network plotting. From yellow to purple, the degree was increasing, and thicker edges denoted the stronger interactions. The size of the nodes is directly proportional to the degree of the nodes. (b) GO enrichment analysis of DHI-UA PPI network. p-value< 0.01 and q-value< 0.05. (c) KEGG pathway analysis of DHI-UA PPI network. p-value< 0.05 and q-value< 0.05. (The software of Cytoscape 3.7.1 and R 3.6.1were used to generate the figure)
Module analysis and functional enrichment analysis
A network module or cluster is defined as a highly interconnected set of nodes that helps discover and reveal hidden biological information within the network [53]. In order to identify the potential mechanism of the 8 key targets, the DHI-UA PPI network was divided into 6 clusters. Ultimately, 3 modules with a score of ≥4.5were selected (Fig. 4(I)). And a total of 4 core targets (TNF, TLR4, NFKB1, SERPINE1) were clustered in these 3 modules.
Analysis of clusters and functional enrichment analysis. I: Clusters of the DHI-UA PPI network. (a) Module 1 (score = 16.375). (b) Module 2 (score = 5.000). (c) Module 3(score = 4.500). Red circles represent DHI-UA targets, and the remaining circles represent other human protein targets associated with disease treatment targets. II: GO enrichment analysis for each cluster. The top 10 GO terms were shown in the figure; p-value< 0.01 and q-value< 0.05; (d) GO enrichment analysis of Module 1. (e) GO enrichment analysis of Module 2. (f) GO enrichment analysis of Module 3. III: KEGG pathway enrichment analysis for each cluster. p-value< 0.05 and q-value< 0.05; (g): Pathway analysis of Module 1. (h) Pathway analysis of Module 2. (i) Pathway analysis of Module 3. The top 30 KEGG pathways were shown in the figure. The y-axis shows significantly enriched KEGG pathways, and x-axis shows the gene counts. (The software of Cytoscape 3.7.1 and R 3.6.1 were used to generate the figure)
Next, we performed GO enrichment analysis (p-value< 0.01 and q-value< 0.05) of the identified DHI-UA PPI network and 3 core modules to gain insights into the cellular component (CC), molecular function (MF) and biological processes (BP) that are affected in UA (Fig. 3(b) and Fig. 4(II)). The results indicated that module 1 was highly correlated with signal transduction, transcription factor activity, cell membrane region, tumor necrosis factor, and protein kinase. Module 2 was highly associated with chemokine. Module 3 was highly linked to blood coagulation, hemostasis, platelet alpha granule and serine-type peptidase activity (Table S2 and S3). Overall, the potential targets were highly connected with regulation of coagulation and hemostasis, cell membrane region, platelet alpha granule, peptidase activity and cofactor binding.
Furthermore, KEGG pathway enrichment analysis were carried out for the DHI-UA PPI network and 3 modules (p-value< 0.05 and q-value< 0.05) (Fig. 3(c) and Fig. 4(III)). The results demonstrated that module 1 was highly correlated with signal transduction, immune system, cardiovascular disease and infectious disease. Module 2 was highly associated with immune system, organismal systems, human diseases and cellular processes. Module 3 was highly linked to immune system and cancer (Table S4 and S5). In conclusion, we recognized 78 UA-related signaling pathways, NF-kappa B, TNF, complement and coagulation cascades, and toll-like receptor signaling pathway et al. Therefore, the results imply that DHI treats UA by participating in above BP, CC and MF and signaling pathway.
Molecular docking simulation
In this paper, four potential targets with five corresponding compounds were simulated by molecular docking, and the docking results were analyzed. Using Pymol software, these five compounds were observed to enter the active pocket of the protein (Fig. 5). Taking the top 2 predicted target-compound pairs in affinity (kcal/mol) as an example for analysis (Table 3). Rosmarinic acid small molecule mainly forms 9 hydrogen bonds with GLN-102, PRO-100, SER-99, TYR-115 and ARG-103 residues on TNF. Caffeic acid small molecule mainly forms 5 hydrogen bonds with ARG-460, PHE-483, ASN-486, GLU-485 and ASN-530 residues on TLR4.
Drug-key compounds- hub targets-pathway network construction
In order to systematically and holistically explain the mechanism of DHI in the treatment of UA, Cytoscape software was operated to construct a drug-key compounds- hub targets -pathway network (Fig. 6). As shown in Fig. 6, there were a total of 40 nodes and 81 edges. As a consequence, those pathways were tightly interacted with 4 hub targets (TNF, TLR4, NFKB1, SERPINE1). It’s worth noting that the compound with the highest degree value was caffeic acid (degree = 4). The target with the highest degree value was NFKB1 (degree = 30). The KEGG pathway with the highest degree value was chagas disease (American trypanosomiasis) (hsa05142, degree = 3). However, the NF-kappa B signaling pathway (hsa04064) with the smallest p-value and q-value will be analyzed as an important pathway (Fig. 7).
Discussion
TCM, a complex mixed system with multiple ingredients and multiple targets, has traditionally used to prevent and treat various cardiovascular diseases (CVDs) for a long time [54,55,56]. Although DHI can effectively cure UA, its pharmacological mechanism of action remains unclear. Consequently, in the present study, a pharmacology network method was executed to identify bioactive compounds, potential targets and the pathways modulated by these compounds in DHI treatment of UA.
Based on module and network topology analysis, the four potential hub targets were found: TNF, TLR4, NFKB1 and SERPINE1. The studies of Biasucci et al. and Huang et al. showed that patients with UA have significantly increased proinflammatory cytokines compared to healthy individuals [57, 58]. Notably, this enhanced inflammatory activity appears to be not only an apparent phenomenon, but may also be related to the pathogenesis of UA. For example, proinflammatory cytokines such as TNF-α may enhance thrombus activity by increasing the expression of monocyte/macrophage tissue factor, as well as cause plaque instability by enhancing apoptosis and degradation of matrix metalloproteinases in atherosclerotic plaques [59,60,61]. More and more studies have shown that TLR4 was one of the important factors leading to the inflammatory process of atherosclerosis, intimal hyperplasia and accelerated formation of atherosclerotic plaque [62, 63]. Meanwhile, a previous study demonstrated that TLR4 were more frequently expressed in classical monocytes of UA patients than control group individuals [64]. In addition, binding of the ligand to the extracellular domain of TLR4 triggers the production of pro-inflammatory cytokines such as TNF-α and interleukin 6 (IL-6) [65]. Earlier studies from this laboratory demonstrated that nuclear factor NF-kappa-B p105 subunit (NFKB1, p50/p105), one of the five subunits of NF-κB, widely implicated in many biological processes such as immunity, inflammation, cell growth, differentiation, apoptosis and tumorigenesis [66, 67]. Importantly, Jin et al. shown that NFKB1 gene mutant is significantly connected with the severity of coronary artery in ACS patients [68]. What’s more, Lanfear et al. indicated that SERPINE1-668delG genotypes have been associated with risk of myocardial infarction [69]. Overall, based on the series of results, this study preliminarily hypothesized that DHI can treat UA by regulating proinflammatory cytokines, NFKB1 and SERPINE1.
To understand potential biological mechanism of DHI against UA, GO and KEGG functional enrichment analysis were applied. Through the KEGG pathway analysis (p-value< 0.05 and q-value< 0.05), we recognized 78 UA-related signaling pathways, NF-kappa B, TNF, complement and coagulation cascades, and toll-like receptor signaling pathway et al. Accordingly, these pathways may be involved in the progress of UA. Based on p-value and q-value, we choose NF-kappa B signaling pathway as most candidate signal for further study. Furthermore, nuclear factor kappa-B (NF-κB), which is a key transcription factor, may play a pivotal role in plaque instability by promoting the cascade expression of procoagulant genes and the regulation of pro-inflammatory genes in response to various stimuli [70, 71]. More importantly, NF-κB transcriptionally activates interferon, interleukins, TNF-α and adhesion molecules [72]. Activated NF-κB had been shown to reside in monocytes/macrophages, endothelial cells and smooth muscle cells in human atherosclerotic vessels, and is enhanced in coronary plaques with UA patients [73]. Notably, deficiency of NFKB1 (p50) had been demonstrated that it plays a regulatory role in NF-κB activity, leading to more inflammatory atherosclerotic lesions in low-density lipoprotein receptor (LDL-R)−/− mice [74]. C-reactive protein (CRP) and amyloid A protein are elevated in patients with UA and can predict the occurrence of subsequent unstable coronary artery events [75]. Other studies suggested that at least some of the deleterious effects of CRP in promoting plaque instability may be mediated through activation of the NF-κB signaling pathway [76]. To conclude, the results suggest that DHI may produce therapeutic effects by regulating NF-κB signaling pathway.
In this study, GO enrichment analysis was adopted to statistically analyze the modules. These potential targets (such as TNF, TLR4, NFKB1) were highly connected with regulation of coagulation, hemostasis, cell membrane region, platelet alpha granule, peptidase activity and cofactor binding. Therefore, the results suggest that DHI treats UA by participating in these BP, CC and MF.
Molecular docking analysis simulation provided a visual interpretation of the interaction between key compounds and their potential protein targets. For example, rosmarinic acid small molecule mainly forms 9 hydrogen bonds with GLN-102, PRO-100, SER-99, TYR-115 and ARG-103 residues on TNF. Rosmarinic acid, which is considered one of the most important polyphenols, has several pharmacological effects: anti-oxidant, inhibition of oxidative stress, anti-inflammatory, anti-cancer and immunomodulatory [77, 78]. Besides, it has been reported that rosmarinic acid may inhibit the expression of NF-κB promoter-related genes. Especially, TNF-α-induced NF-κB activation can be inhibited by rosmarinic acid [79]. Overall, it was speculated that the main compositions of DHI may play a significant role in the treatment of UA through hub targets in these top-ranking signaling pathways. However, some limitations of our study should be considered. For instance, the results are only based on screening already known chemical constituents of DHI, related targets, and signaling pathways from literatures and existing databases. Consequently, more in-depth researches are required for characterization of the underlying mechanisms.
Conclusion
Via the method of network pharmacology and molecular docking, 12 ingredients of DHI and 27 putative known therapeutic targets were collected and explored the underlying mechanism of DHI in treatment of UA. Then, DHI exerted treatment effects on UA by regulating 4 hub targets: TNF, TLR4, NFKB1 and SERPINE1. Based on the results of GO and KEGG pathway enrichment analysis, we found that these hub targets ameliorated UA by participating in regulating coagulation, hemostasis, peptidase activity, signal transduction and immune system. In conclusion, the results of the study preliminarily predicted the related underlying mechanism of DHI against UA, proving that the characteristics of multi-target synergy. However, animal experiments, molecular biological experiments and clinical investigations should be performed to verify the mechanism of DHI against UA in future studies.
Availability of data and materials
All data obtained or analyzed during this study are available from published article and supplementary materials. The datasets during the current study are available from the corresponding author upon reasonable request.
Abbreviations
- ACS:
-
Acute coronary syndromes
- ADP:
-
Adenosine diphosphate
- BATMAN-TCM:
-
Bioinformatics analysis tool for molecular mechanism of Traditional Chinese Medicine
- BP:
-
Biological processes
- CA12:
-
Carbonic anhydrase 12
- CABG:
-
Coronary artery bypass grafting
- CC:
-
Cellular component
- CNKI:
-
China National Knowledge Infrastructure Database
- CRP:
-
C-reactive protein
- CVDs:
-
Cardiovascular diseases
- DHI:
-
Danhong Injection
- DigSee:
-
Disease Gene Search Engine with Evidence Sentences
- DS:
-
Dan Shen
- F3:
-
Tissue factor
- FGA:
-
Fibrinogen alpha chain
- FGG:
-
Fibrinogen gamma chain
- GO:
-
Gene Ontology
- HH:
-
Hong Hua
- IL-6:
-
Interleukin 6
- KEGG:
-
Kyoto Encyclopedia of Genes and Genomes
- LDL-R:
-
Low-density lipoprotein receptor
- LY96:
-
Lymphocyte antigen 96
- MCODE:
-
Molecular Complex Detection
- MF:
-
Molecular function
- NFKB1:
-
Nuclear factor NF-kappa-B p105 subunit
- NF-κB:
-
Nuclear factor kappa-B
- NSTEMI:
-
Non–ST-segment elevation myocardial infarction
- OMIM:
-
Online Mendelian Inheritance in Man
- PDB:
-
Protein Data Bank
- PPI:
-
Protein-protein interaction
- SERPINE1:
-
Plasminogen activator inhibitor 1
- SMILES:
-
Simplified molecular input line entry specification
- STEMI:
-
ST-segment elevation myocardial infarction
- TCM:
-
Traditional Chinese Medicine
- TLR4:
-
Toll-like receptor 4
- TNF:
-
Tumor necrosis factor
- TTD:
-
Therapeutic Target Database
- UA:
-
Unstable angina
References
Benjamin EJ, Muntner P, Alonso A, Bittencourt MS, Callaway CW, Carson AP, Chamberlain AM, Chang AR, Cheng S, Das SR, Delling FN, Djousse L, MSV E, Ferguson JF, Fornage M, Jordan LC, Khan SS, Kissela BM, Knutson KL, Kwan TW, Lackland DT, Lewis TT, Lichtman JH, Longenecker CT, Loop MS, Lutsey PL, Martin SS, Matsushita K, Moran AE, Mussolino ME, O'Flaherty M, Pandey A, Perak AM, Rosamond WD, Roth GA, UKA S, Satou GM, Schroeder EB, Shah SH, Spartano NL, Stokes A, Tirschwell DL, Tsao CW, Turakhia MP, LB VW, Wilkins JT, Wong SS, Virani SS. American Heart Association Council on Epidemiology and Prevention Statistics Committee and Stroke Statistics Subcommittee. Heart disease and stroke statistics-2019 update: a report from the American Heart Association. Circulation. 2019;139(10):e56–e528.
Xu S, Xu Y, Liu P, Zhang S, Liu H, Slavin S, Kumar S, Koroleva M, Luo J, Wu X, Rahman A, Pelisek J, Jo H, Si S, Miller CL, Jin ZG. The novel coronary artery disease risk gene JCAD/KIAA1462 promotes endothelial dysfunction and atherosclerosis. Eur Heart J. 2019;40(29):2398–408.
Yeghiazarians Y, Braunstein JB, Askari A, Stone PH. Unstable angina pectoris. N Engl J Med. 2000;342(2):101–14.
Giannitsis E, Biener M, Hund H, Mueller-Hennessen M, Vafaie M, Gandowitz J, Riedle C, Löhr J, Katus HA, Stoyanov KM. Management and outcomes of patients with unstable angina with undetectable, normal, or intermediate hsTnT levels. Clin Res Cardiol. 2019. https://doi.org/10.1007/s00392-019-01529-4.
Sandoval Y, Apple FS, Smith SW. High-sensitivity cardiac troponin assays and unstable angina. Eur Heart J Acute Cardiovasc Care. 2018;7(2):120–8.
Braunwald E, Morrow DA. Unstable angina: is it time for a requiem? Circulation. 2013;127(24):2452–7.
Chakhtoura EY, Shamoon FE, Haft JI, Obiedzinski GR, Cohen AJ, Watson RM. Comparison of platelet activation in unstable and stable angina pectoris and correlation with coronary angiographic findings. Am J Cardiol. 2000;86(8):835–9.
Peng R, Yang X, Liang X. Nicorandil effects on platelet function, Hs-CRP, MMP-9 and myocardial antioxidation in patients with unstable angina. Exp Ther Med. 2019;18(4):3095–9.
McNeely CA, Brown DL. Upcoding, fraud, and the stubborn persistence of unstable angina. JAMA Intern Med. 2019;179(2):261–3.
Kumar A, Cannon CP. Acute coronary syndromes: diagnosis and management, part I. Mayo Clin Proc. 2009;84(10):917–38.
Yusuf S, Zhao F, Mehta SR, Chrolavicius S, Tognoni G, Fox KK. Clopidogrel in unstable angina to prevent recurrent events trial investigators. Effects of clopidogrel in addition to aspirin in patients with acute coronary syndromes without ST-segment elevation. N Engl J Med. 2001;345(7):494–502.
He S, Guo H, Zhao T, Meng Y, Chen R, Ren J, Pan L, Fan G, Jiang M, Qin G, Zhu Y, Gao X. A defined combination of four active principles from the Danhong injection is necessary and sufficient to accelerate EPC-mediated vascular repair and local angiogenesis. Front Pharmacol. 2019;10:1080.
Cyranoski D. Why Chinese medicine is heading for clinics around the world. Nature. 2018;561(7724):448–50.
Zhao T, Chang L, Zhang B, Lu M, Wang X, Orgah JO, Wang Y, Tian X, Yang J, Fan G, Zhang B, Zhu Y. Specific combination of salvianolic acids as core active ingredients of Danhong injection for treatment of arterial thrombosis and its derived dry gangrene. Front Pharmacol. 2017;8:361.
Li SN, Li P, Liu WH, Shang JJ, Qiu SL, Zhou MX, Liu HX. Danhong injection enhances angiogenesis after myocardial infarction by activating MiR-126/ERK/VEGF pathway. Biomed Pharmacother. 2019;120:109538.
Xu W, Zhang Y, Yu Y, Li B, Liu J, Wang P, Wu H, Liu Q, Wei Z, Xiao H, Wang Z. Dose-dependent target diversion of Danhong injection on the Glu-GLT-1/Gly-GlyRα dynamic balance module of cerebral ischemia. Pharmacol Res. 2018;135:80–8.
Li C, Yang J, Tong X, Zhao C, He Y, Wan H. Precursor ion scan enhanced rapid identification of the chemical constituents of Danhong injection by liquid chromatography–tandem mass spectrometry: an integrated strategy. J Chromatogr A. 1602;2019:378–85.
Li JP, Liu Y, Guo JM, Shang EX, Zhu ZH, Zhu KY, Tang YP, Zhao BC, Tang ZS, Duan JA. A comprehensive strategy to evaluate compatible stability of chinese medicine injection and infusion solutions based on chemical analysis and bioactivity assay. Front Pharmacol. 2017;8:833.
Zou JB, Zhang XF, Wang J, Wang F, Cheng JX, Yang FY, Song X, Wang Y, Liang YL, Shi YJ. The therapeutic efficacy of Danhong injection combined with percutaneous coronary intervention in acute coronary syndrome: a systematic review and meta-analysis. Front Pharmacol. 2018;9:550.
Zhang X, Wang H, Chang Y, Wang Y, Lei X, Fu S, Zhang J. An overview of meta-analyses of Danhong injection for unstable angina. Evid Based Complement Alternat Med. 2015;2015:358028.
Li S, Zhang B, Jiang D, Wei Y, Zhang N. Herb network construction and co-module analysis for uncovering the combination rule of traditional Chinese herbal formulae. BMC Bioinformatics. 2010;11(Suppl 11):S6.
Song Y, Wang H, Pan Y, Liu T. Investigating the multi-target pharmacological mechanism of Hedyotis diffusa Willd acting on prostate Cancer: a network pharmacology approach. Biomolecules. 2019;9(10):E591.
Li S, Zhang B. Traditional Chinese medicine network pharmacology: theory, methodology and application. Chin J Nat Med. 2013;11(2):110–20.
Kim S, Thiessen PA, Bolton EE, Chen J, Fu G, Gindulyte A, Han L, He J, He S, Shoemaker BA, Wang J, Yu B, Zhang J, Bryant SH. PubChem substance and compound databases. Nucleic Acids Res. 2016;44(D1):D1202–13.
Evans DA. History of the Harvard ChemDraw project. Angew Chem Int Ed Engl. 2014;53(42):11140–5.
Nickel J, Gohlke BO, Erehman J, Banerjee P, Rong WW, Goede A, Dunkel M, Preissner R. SuperPred: update on drug classification and target prediction. Nucleic Acids Res. 2014;42:W26–31.
Gfeller D, Grosdidier A, Wirth M, Daina A, Michielin O, Zoete V. SwissTargetPrediction: a web server for target prediction of bioactive small molecules. Nucleic Acids Res. 2014;42:W32–8.
Liu Z, Guo F, Wang Y, Li C, Zhang X, Li H, Diao L, Gu J, Wang W, Li D, He F. BATMAN-TCM: a bioinformatics analysis tool for molecular mechANism of traditional Chinese medicine. Sci Rep. 2016;6:21146.
Liu J, Li Y, Zhang Y, Huo M, Sun X, Xu Z, Tan N, Du K, Wang Y, Zhang J, Wang W. A network pharmacology approach to explore the mechanisms of Qishen granules in heart failure. Med Sci Monit. 2019;25:7735–45.
Kim J, So S, Lee HJ, Park JC, Kim JJ, Lee H. DigSee: disease gene search engine with evidence sentences (version cancer). Nucleic Acids Res. 2013;41:W510–7.
Piñero J, Bravo À, Queralt-Rosinach N, Gutiérrez-Sacristán A, Deu-Pons J, Centeno E, García-García J, Sanz F, Furlong LI. DisGeNET: a comprehensive platform integrating information on human disease-associated genes and variants. Nucleic Acids Res. 2017;45(D1):D833–9.
Amberger JS, Bocchini CA, Schiettecatte F, Scott AF, Hamosh A. OMIM. Org: online Mendelian inheritance in man (OMIM®), an online catalog of human genes and genetic disorders. Nucleic Acids Res. 2015;43:D789–98.
Yang H, Qin C, Li YH, Tao L, Zhou J, Yu CY, Xu F, Chen Z, Zhu F, Chen YZ. Therapeutic target database update 2016: enriched resource for bench to clinical drug target and targeted pathway information. Nucleic Acids Res. 2016;44(D1):D1069–74.
Szklarczyk D, Morris JH, Cook H, Kuhn M, Wyder S, Simonovic M, Santos A, Doncheva NT, Roth A, Bork P, Jensen LJ, von Mering C. The STRING database in 2017: quality-controlled protein–protein association networks, made broadly accessible. Nucleic Acids Res. 2017;45(D1):D362–8.
Franz M, Lopes CT, Huck G, Dong Y, Sumer O, Bader GD. Cytoscape.Js: a graph theory library for visualisation and analysis. Bioinformatics. 2016;32(2):309–11.
Jeong H, Mason SP, Barabási AL, Oltvai ZN. Lethality and centrality in protein networks. Nature. 2001;411(6833):41–2.
Li S. Network systems underlying traditional Chinese medicine syndrome and herb formula. Curr Bioinforma. 2009;4(3):188–96.
Newman MEJ. A measure of betweenness centrality based on random walks. Soc Networks. 2005;27(1):39–54.
Liu X, Wu J, Zhang D, Wang K, Duan X, Zhang X. A network pharmacology approach to uncover the multiple mechanisms of hedyotis diffusa willd on colorectal cancer. Evid Based Complement Alternat Med. 2018;2018:6517034.
Liu X, Wu J, Zhang D, Wang K, Duan X, Meng Z, Zhang X. Network pharmacology-based approach to investigate the mechanisms of hedyotis diffusa willd in the treatment of gastric cancer. Evid Based Complement Alternat Med. 2018;2018:7802639.
Sabidussi G. The centrality index of a graph. Psychometrika. 1966;31(4):581–603.
Bader GD, Hogue CWV. An automated method for finding molecular complexes in large protein interaction networks. BMC bioinformatics. 2003;4(1):2.
Feng H, Gu ZY, Li Q, Liu QH, Yang XY, Zhang JJ. Identification of significant genes with poor prognosis in ovarian cancer via bioinformatical analysis. J ovarian res. 2019;12(1):35.
Huber W, Carey VJ, Gentleman R, Anders S, Carlson M, Carvalho BS, Bravo HC, Davis S, Gatto L, Girke T, Gottardo R, Hahne F, Hansen KD, Irizarry RA, Lawrence M, Love MI, MacDonald J, Obenchain V, Oleś AK, Pagès H, Reyes A, Shannon P, Smyth GK, Tenenbaum D, Waldron L, Morgan M. Orchestrating high-throughput genomic analysis with bioconductor. Nat Methods. 2015;12(2):115–21.
The Gene Ontology Consortium. Expansion of the gene ontology knowledgebase and resources. Nucleic Acids Res. 2017;45(D1):D331–8.
Kanehisa M, Goto S. KEGG: Kyoto encyclopedia of genes and genomes. Nucleic Acids Res. 2000;28(1):27–30.
Morris GM, Huey R, Lindstrom W, Sanner MF, Belew RK, Goodsell DS, Olson AJ. AutoDock4 and AutoDockTools4: automated docking with selective receptor flexibility. J Comput Chem. 2009;30(16):2785–91.
Goodsell DS, Zardecki C, Di Costanzo L, Duarte JM, Hudson BP, Persikova I, Segura J, Shao C, Voigt M, Westbrook JD, Young JY, Burley SK. RCSB protein data Bank: enabling biomedical research and drug discovery. Protein Sci. 2019. https://doi.org/10.1002/pro.3730.
Trott O, Olson AJ. AutoDock Vina: improving the speed and accuracy of docking with a new scoring function, efficient optimization, and multithreading. J Comput Chem. 2010;31(2):455–61.
Yuan S, Chan HCS, Filipek S, Vogel H. PyMOL and Inkscape bridge the data and the data visualization. Structure. 2016;24(12):2041–2.
Viegas DJ, Edwards TG, Bloom DC, Abreu PA. Virtual screening identified compounds that bind to cyclin dependent kinase 2 and prevent herpes simplex virus type 1 replication and reactivation in neurons. Antivir Res. 2019;172:104621.
Xu LL, Shang ZP, Bo T, Sun L, Guo QL, Qiao X, Ye M. Rapid quantitation and identification of the chemical constituents in Danhong Injection by liquid chromatography coupled with orbitrap mass spectrometry. J Chromatogr A. 2019;1606:460378.
Zuo H, Zhang Q, Su S, Chen Q, Yang F, Hu Y. A network pharmacology-based approach to analyse potential targets of traditional herbal formulas: an example of Yu ping Feng decoction. Sci Rep. 2018;8(1):11418.
Shu Z, Wu T, Shahen M, Guo Z, Shu J, Wang HE, Shar AH, Farag MR, Alagawany M, El-Hack MEA, Liu C. System-pharmacology dissection of traditional Chinese herbs SINI decoction for treatment of cardiovascular diseases. An Acad Bras Cienc. 2019;91(3):e20180424.
Hao DC, Xiao PG. Impact of drug metabolism/pharmacokinetics and their relevance upon traditional medicine-based cardiovascular drug research. Curr Drug Metab. 2019;20(7):556–74.
Hao P, Jiang F, Cheng J, Ma L, Zhang Y, Zhao Y. Traditional Chinese medicine for cardiovascular disease: evidence and potential mechanisms. J Am Coll Cardiol. 2017;69(24):2952–66.
Biasucci LM, Liuzzo G, Fantuzzi G, Caligiuri G, Rebuzzi AG, Ginnetti F, Dinarello CA, Maseri A. Increasing levels of interleukin (IL)-1Ra and IL-6 during the first 2 days of hospitalization in unstable angina are associated with increased risk of in-hospital coronary events. Circulation. 1999;99(16):2079–84.
Huang D, Yang CZ, Yao L, Wang Y, Liao YH, Huang K. Activation and overexpression of PARP-1 in circulating mononuclear cells promote TNF-α and IL-6 expression in patients with unstable angina. Arch Med Res. 2008;39(8):775–84.
Tousoulis D, Antoniades C, Nikolopoulou A, Koniari K, Vasiliadou C, Marinou K, Koumallos N, Papageorgiou N, Stefanadi E, Siasos G, Stefanadis C. Interaction between cytokines and sCD40L in patients with stable and unstable coronary syndromes. Eur J Clin Investig. 2007;37(8):623–8.
Sullivan GW, Sarembock IJ, Linden J. The role of inflammation in vascular diseases. J Leukoc Biol. 2000;67(5):591–602.
Waehre T, Halvorsen B, Damås JK, Yndestad A, Brosstad F, Gullestad L, Kjekshus J, Frøland SS, Aukrust P. Inflammatory imbalance between IL-10 and TNFα in unstable angina potential plaque stabilizing effects of IL-10. Eur J Clin Investig. 2002;32(11):803–10.
Candore G, Aquino A, Balistreri CR, Bulati M, Di Carlo D, Grimaldi MP, Listì F, Orlando V, Vasto S, Caruso M, Colonna-Romano G, Lio D, Caruso C. Inflammation, longevity, and cardiovascular diseases: role of polymorphisms of TLR4. Ann N Y Acad Sci. 2006;1067:282–7.
Norata GD, Garlaschelli K, Ongari M, Raselli S, Grigore L, Benvenuto F, Maggi FM, Catapano AL. Effect of the toll-like receptor 4 (TLR-4) variants on intima-media thickness and monocyte-derived macrophage response to LPS. J Intern Med. 2005;258(1):21–7.
Justo-Junior AS, Villarejos LM, Lima XTV, Nadruz W Jr, Sposito AC, Mamoni RL, Abdalla R, Fernandes JL, Oliveira RTD, Blotta MHSL. Monocytes of patients with unstable angina express high levels of chemokine and pattern-recognition receptors. Cytokine. 2019;113:61–7.
Yang J, Liu C, Zhang L, Liu Y, Guo A, Shi H, Liu X, Cheng Y. Intensive atorvastatin therapy attenuates the inflammatory responses in monocytes of patients with unstable angina undergoing percutaneous coronary intervention via peroxisome proliferator-activated receptor γ activation. Inflammation. 2015;38(4):1415–23.
Beinke S, Robinson MJ, Hugunin M, Ley SC. Lipopolysaccharide activation of the TPL-2/MEK/extracellular signal-regulated kinase mitogen-activated protein kinase cascade is regulated by IkappaB kinase-induced proteolysis of NF-kappaB1 p105. Mol Cell Biol. 2004;24(21):9658–67.
Concetti J, Wilson CL. NFKB1 and cancer: friend or foe? Cells. 2018;7(9):E133.
Jin SY, Luo JY, Li XM, Liu F, Ma YT, Gao XM, Yang YN. NFKB1 gene rs28362491 polymorphism is associated with the susceptibility of acute coronary syndrome. Biosci Rep. 2019;39(4):BSR20182292.
Lanfear DE, Jones PG, Marsh S, Cresci S, Spertus JA, McLeod HL. Connexin37 (GJA4) genotype predicts survival after an acute coronary syndrome. Am Heart J. 2007;154(3):561–6.
Collins T, Cybulsky MI. NF-κB: pivotal mediator or innocent bystander in atherogenesis? J Clin Invest. 2001;107(3):255–64.
Monaco C, Andreakos E, Kiriakidis S, Mauri C, Bicknell C, Foxwell B, Cheshire N, Paleolog E, Feldmann M. Canonical pathway of nuclear factor κB activation selectively regulates proinflammatory and prothrombotic responses in human atherosclerosis. Proc Natl Acad Sci U S A. 2004;101(15):5634–9.
Kopp E, Ghosh S. Inhibition of NF-kappa B by sodium salicylate and aspirin. Science. 1994;265(5174):956–9.
Wilson SH, Best PJ, Edwards WD, Holmes DR Jr, Carlson PJ, Celermajer DS, Lerman A. Nuclear factor-κB immunoreactivity is present in human coronary plaque and enhanced in patients with unstable angina pectoris. Atherosclerosis. 2002;160(1):147–53.
Kanters E, Gijbels MJ, van der Made I, Vergouwe MN, Heeringa P, Kraal G, Hofker MH, de Winther MP. Hematopoietic NF-κB1 deficiency results in small atherosclerotic lesions with an inflammatory phenotype. Blood. 2004;103(3):934–40.
Ritchie ME. Nuclear factor-κB is selectively and markedly activated in humans with unstable angina pectoris. Circulation. 1998;98(17):1707–13.
Liuzzo G, Santamaria M, Biasucci LM, Narducci M, Colafrancesco V, Porto A, Brugaletta S, Pinnelli M, Rizzello V, Maseri A, Crea F. Persistent activation of nuclear factor kappa-B signaling pathway in patients with unstable angina and elevated levels of C-reactive protein evidence for a direct proinflammatory effect of azide and lipopolysaccharide-free C-reactive protein on human monocytes via nuclear factor kappa-B activation. J Am Coll Cardiol. 2007;49(2):185–94.
Alagawany M, Abd El-Hack ME, Farag MR, Gopi M, Karthik K, Malik YS, Dhama K. Rosmarinic acid: modes of action, medicinal values and health benefits. Anim Health Res Rev. 2017;18(2):167–76.
Joardar S, Dewanjee S, Bhowmick S, Dua TK, Das S, Saha A, De Feo V. Rosmarinic acid attenuates cadmium-induced nephrotoxicity via inhibition of oxidative stress, apoptosis, inflammation and fibrosis. Int J Mol Sci. 2019;20(8):E2027.
Lee J, Jung E, Kim Y, Lee J, Park J, Hong S, Hyun CG, Park D, Kim YS. Rosmarinic acid as a downstream inhibitor of IKK-β in TNF-α-induced upregulation of CCL11 and CCR3. Br J Pharmacol. 2006;148(3):366–75.
Acknowledgements
Not applicable.
Funding
This work was supported by the Young Scientists Training Program of Beijing University of Chinese Medicine and the National Nature Science Foundation of China (Grant nos. 81473547 and 81673829). The funding body approved the design of the study, analysis, and interpretation of data, and publication of the manuscript.
Author information
Authors and Affiliations
Contributions
SYG and JRW conceived and designed the study; XKL and WZ provided significative suggestions on the methodology. JYZ, SSJ and ZQM collected the compounds of DHI, targets of ingredients and UA. SYL, MWN and YYL analyzed and interpreted the data of research. SYG was a major contributor in writing the manuscript. All authors read and approved the final of the manuscript.
Corresponding author
Ethics declarations
Ethics approval and consent to participate
Not applicable.
Consent for publication
Not applicable.
Competing interests
The authors declare that they have no conflicts of interests regarding the publication of this paper.
Additional information
Publisher’s Note
Springer Nature remains neutral with regard to jurisdictional claims in published maps and institutional affiliations.
Supplementary information
Additional file 1 Supplementary 1. Table S1.
The information of all ingredients of DHI.
Additional file 2 Supplementary 2. Table S2.
The information of GO enrichment analysis of DHI-UA PPI network.
Additional file 3 Supplementary 3. Table S3.
The information of GO enrichment analysis for each cluster.
Additional file 4 Supplementary 4. Table S4.
The information of KEGG pathway analysis of DHI-UA PPI network.
Additional file 5 Supplementary 5. Table S5.
The information of KEGG pathway enrichment analysis for each cluster.
Rights and permissions
Open Access This article is distributed under the terms of the Creative Commons Attribution 4.0 International License (http://creativecommons.org/licenses/by/4.0/), which permits unrestricted use, distribution, and reproduction in any medium, provided you give appropriate credit to the original author(s) and the source, provide a link to the Creative Commons license, and indicate if changes were made. The Creative Commons Public Domain Dedication waiver (http://creativecommons.org/publicdomain/zero/1.0/) applies to the data made available in this article, unless otherwise stated.
About this article
Cite this article
Guo, S., Wu, J., Zhou, W. et al. Investigating the multi-target pharmacological mechanism of danhong injection acting on unstable angina by combined network pharmacology and molecular docking. BMC Complement Med Ther 20, 66 (2020). https://doi.org/10.1186/s12906-020-2853-5
Received:
Accepted:
Published:
DOI: https://doi.org/10.1186/s12906-020-2853-5