Abstract
Epithelial ovarian cancer (EOC) is the most lethal gynecologic malignancy in North America. Current therapeutic regimens are ineffective against advanced EOC. A better understanding of the molecular mechanisms that regulate the biology of EOC will be a critical step toward developing more efficacious therapies against EOC. Herein, we demonstrate that elevated expression of transcription factor ZIC2 was associated with lower survival of EOC patients. Knockout of endogenous ZIC2 in EOC cells attenuated the tumorigenic phenotypes associated with both bulk and cancer stem cells in vitro and in vivo, indicating a pro-tumorigenic role of ZIC2 in EOC. On the other hand, however, overexpression of ZIC2 in EOC cells that do not express endogenous ZIC2 promoted cell migration and sphere formation, but inhibited cell growth and colony formation in vitro and tumor growth in vivo, indicating that the role for ZIC2 in EOC is context dependent. Our transcriptomic analysis showed that ZIC2-regulated genes were involved in multiple biological processes and signaling pathways associated with tumor progression. In conclusion, our findings reveal a context-dependent role for ZIC2 in regulating tumorigenic phenotypes in EOC, providing evidence that ZIC2 can be a potential therapeutic target for EOCs that express a high level of ZIC2.
Similar content being viewed by others
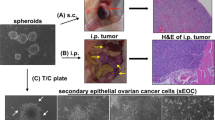
Introduction
Epithelial ovarian cancer (EOC), comprising approximately 90% of ovarian cancer, is the most lethal gynecologic malignancy and the fifth leading cause of cancer death in women in North America [1]. Current therapeutic regimens against advanced EOCs often fail due to the recurrence of the disease, driven at least in part by tumor heterogeneity [2, 3], resulting in approximately 30% of the five-year survival rate [4]. For example, there exists within ovarian cancers a population of cells with stem cell-like characteristics (cancer stem cells, CSCs) that are more chemoresistant and tumorigenic than non-CSC counterparts (bulk cells) [5, 6]. CSCs have the ability to self-renew and differentiate into different lineages of cells, giving rise to heterogeneous tumors [7, 8]. Molecular mechanisms by which CSCs arise are associated with genomic instability leading to differing mutational profiles and epigenetic alterations that support the emergence of phenotypically diverse cells during tumor progression [9]. A better understanding of the molecular mechanisms that regulate the biology of both CSC and bulk populations, as well as the phenotypic plasticity (transition between CSCs and bulk cells) will be a critical step towards developing more efficacious therapies against EOC.
The zinc finger of the cerebellum (Zic) family comprises five members (Zic1-5) that function as transcription factors and co-factors and are critical for patterning and morphogenesis during embryonic development [10, 11]. Accumulating evidence indicates that elevated ZIC2 expression in a variety of human cancers is associated with poor survival of patients and promotes growth, survival, metastasis, and tumorigenicity of cancer cells [12,13,14,15,16,17,18,19]. On the other hand, ZIC2 is downregulated and plays a tumor suppressing role in breast cancer [20]. Recent studies demonstrate that ZIC2 is a key factor in regulating self-renewal in normal and cancer stem cell populations and determines cell fate by associating with the nucleosome remodeling and deacetylase (NuRD) complex or with the nucleosome remodeling factor (NURF) complex at the enhancers or promoters of stem cell-associated genes [17, 21, 22]. Similar to many developmentally regulated transcription factors, the functions of ZIC2 are context dependent. For example, a recent study demonstrated that ZIC2 maintains a constant level of expression in the developing cerebellum yet manifests dynamic changes in DNA binding engendered by alterations in chromatin accessibility that occur as cells alter their fate [23]. Hence, ZIC2 could have varying functions within a heterogeneous ovarian tumor, wherein numerous epigenetic states (e.g., CSCs and bulk proliferative cells) are present. To date, there is only one published study on ZIC2 in EOC, which showed that higher ZIC2 expression was associated with poor patient survival and that siRNA knockdown of ZIC2 reduced the growth of OVCAR8 cells (an EOC cell line) [14]. However, a comprehensive study is necessary to delineate the function of ZIC2 in this highly heterogeneous cancer.
In this study, we demonstrate that ZIC2 expression is associated with poor overall and post-progression survival of EOC patients. Functionally, ZIC2 is required for maintaining tumorigenic phenotypes in EOC cells that express endogenous ZIC2, including proliferation, colony formation, migration, and stemness of CSCs in vitro and tumor growth in vivo. Overexpression of ZIC2 in the EOC cells that express little endogenous ZIC2 leads to variable effects on tumorigenic phenotypes that are either consistent with or contradictory to the effects of knockout of endogenous ZIC2. Transcriptomic analysis showed that ZIC2 is a pivotal regulator of the genes that are involved in various biological processes in EOC. Collectively, our findings suggest that ZIC2 regulates tumorigenic phenotypes in EOC in a context-dependent manner. Accordingly, ZIC2 can be a potential therapeutic target for the treatment of EOCs that express a high level of ZIC2.
Results
ZIC2 expression is associated with poor survival in EOC patients
To investigate the role of ZIC2 in EOC, we first analyzed the expression of ZIC2 in EOC and its association with the outcome of EOC patients. Our analysis using the Kaplan–Meier Plotter (kmplot.com) showed that high mRNA levels of ZIC2 were associated with poor overall survival and post-progression survival in EOC patients, but not with progression-free survival (Fig. 1A), which is consistent with the published study [14]. Furthermore, analysis using the Gene Expression Profiling Interactive Analysis 2 (GEPIA2) showed that ZIC2 mRNA levels were more variable and higher in EOC compared to normal tissues (Fig. 1B). Immunoblotting showed that ZIC2 was expressed at variable levels in EOC cell lines (Fig. 1C), which is consistent with the ZIC2 mRNA levels in the EOC cell lines in the Cancer Cell line Encyclopedia (CCLE) database (Supplementary Fig. S1), but not expressed in immortalized ovarian surface epithelial (IOSE386) and fallopian tube cell lines (FT189) (Fig. 1C). IF showed that ZIC2 was localized in the nuclei of SKOV3 and OVCAR8 cells, which was absent in ZIC2 KO cells (Fig. 1D), confirming the specificity of the ZIC2 staining. Taken together, our results show that higher ZIC2 expression is associated with poorer outcomes of EOC patients, and that ZIC2 is expressed at variable levels in EOC cell lines.
A Overall, progression-free, and post-progression survival curves were generated using the Kaplan–Meier Plotter for EOC patients with low (bottom 75%) versus high (top 25%) ZIC2 mRNA expression. B ZIC2 mRNA levels were higher in ovarian cancer tumors compared with normal ovarian tissues as determined using the GEPIA2. C ZIC2 protein level in immortalized ovarian epithelial cells (IOSE386), immortalized fallopian tube epithelial cells (FT189) and EOC cell lines was examined using immunoblotting. The arrowhead indicates the ZIC2 band. The top band is non-specific. β-actin was the loading control. D Immunofluorescence showed that ZIC2 was localized in the nuclei of SKOV3 and OVCAR8 cells. Nuclei were stained with DAPI. Scale bar: 10 µm.
ZIC2 knockout attenuates tumorigenic phenotypes of EOC cells
Immunoblotting and the CCLE database analysis showed that SKOV3 cells expressed the highest level of ZIC2 among the cell lines we examined (Fig. 1C and Supplementary Fig. 1). COV318 and TYK-NU cells expressed a higher level of ZIC2 than OVCAR8 cells in immunoblotting (Fig. 1C), however, we found that they grew very slowly in culture. OVCAR8 cells expressed the third highest ZIC2 mRNA in the CCLE database among the ovarian cancer cell lines we examined (Supplementary Fig. S1), expressed a reasonable level of ZIC2 in immunoblotting (Fig. 1C), and were easy to grow in culture. Therefore, we used SKOV3 and OVCAR8 to generate CRISPR/Cas9 knockout models for the loss of function experiments in this study. ZIC2 knockout was confirmed by immunoblotting (Fig. 2A), IF (Fig. 1D), and genomic DNA sequencing (Supplementary Table S3). Neutral red uptake assays showed that ZIC2 knockout decreased cell growth in SKOV3 and OVCAR8 cells compared to their respective WT cells (Fig. 2B). Clonogenic assays showed that ZIC2 knockout decreased the number of SKOV3 colonies, however, the size of KO colonies appeared to be larger than WT colonies in SKOV3 cells compared to the WT cells (Fig. 2C). On the other hand, ZIC2 knockout decreased the size, but not the number, of OVCAR8 colonies compared to the WT cells (Fig. 2C). Soft agar assays showed that ZIC2 knockout reduced the number and size of SKOV3 colonies, but it only reduced the size, but not the number, of OVCAR8 colonies (Fig. 2D). In addition, ZIC2 knockout decreased migration of SKOV3 and OVCAR8 cells (Fig. 2E). Finally, subcutaneous xenograft experiments showed that ZIC2 knockout decreased growth, weight, and size of SKOV3 and OVCAR8 tumors in mice (Fig. 2F–I). We observed that SKOV3 WT cells formed palpable tumors as early as 7 days after injection and the WT tumors grew rapidly afterwards, whereas SKOV3 KO cells did not form measurable tumors until 19 days post-injection and the ZIC2 KO tumors grew slowly and were much smaller than the WT tumors when harvested at day 39 (Fig. 2F). IHC showed that Ki67-positive (a proliferation marker) cells were reduced in the KO tumors compared to the WT tumors (Fig. 2G). Knockout of ZIC2 also decreased the growth of OVCAR8 tumors, although the effect was less pronounced compared to SKOV3 tumors (Fig. 2H). Because of the irregular shape of the OVCAR8 tumors, we could not accurately determine the volume of the live tumors. Hence, we measured the tumors after they were harvested. As shown in Fig. 2H, ZIC2 KO OVCAR8 cells formed smaller tumors in mice compared to ZIC2 WT cells as determined by the volume and weight of the tumors. Similar to SKOV3 tumors, Ki67-positive cells were reduced in ZIC2 KO OVCAR8 tumors compared to WT OVCAR8 tumors (Fig. 2I). Taken together, our results suggest that ZIC2 is required to maintain the tumorigenic phenotypes in ZIC2-expressing EOC cell lines.
A Immunoblotting confirmed ZIC2 knockout in SKOV3 and OVCAR8 cells. The arrowhead indicates the ZIC2 band. The top band is non-specific. β-actin was the loading control. B Neutral red uptake assays showed that ZIC2 knockout reduced the growth of SKOV3 cells (mean ± SD, four independent experiments) and OVCAR8 cells (mean ± SD, three independent experiments). C Clonogenic assays showed that ZIC2 knockout decreased the number of SKOV3 colonies (mean ± SD, four independent experiments) and the size of OVCAR8 colonies (mean ± SD, three independent experiments). D Soft agar assays showed that ZIC2 knockout decreased the number and size of SKOV3 colonies and decreased the size of OVCAR8 colonies. Data are the mean ± SD of three independent experiments. Scale bar: 100 µm. E Transwell migration assays showed that ZIC2 knockout reduced migration of SKOV3 and OVCAR8 cells. Data are the mean ± SD of three independent experiments. Scale bar: 100 μm. F Subcutaneous xenograft model showed that ZIC2 knockout reduced growth, weight, and size of SKOV3 tumors (n = 8 for WT and n = 10 for KO tumors). Scale bar: 10 mm. G Immunohistochemistry showed that ZIC2 knockout reduced the number of Ki67-positive cells. The histogram showed the percentage of Ki67-positive (Ki67+) cells per field (average of 6 random fields). Scale bar: 100 μm. *Significant differences (P < 0.05). H The subcutaneous xenograft model showed that ZIC2 knockout reduced the growth, weight, and size of OVCAR8 tumors (n = 7 for WT and n = 7 for KO tumors). I Immunohistochemistry showed that ZIC2 knockout reduced the number of Ki67-positive cells. The histogram showed the percentage of Ki67-positive (Ki67+) cells per field (average of 6 random fields). Scale bar: 100 μm. Data are the mean ± SD. *Significant differences (P < 0.05). WT: ZIC2 wild type,KO; knockout of ZIC2.
ZIC2 knockout reduces cancer stem cell-like phenotypes in EOC cells
To determine whether ZIC2 regulates CSCs in EOC, we performed sphere formation assays, which measure self-renewal of CSCs, and found that ZIC2 knockout reduced the number and size of SKOV3 and OVCAR8 spheres (Fig. 3A), suggesting that ZIC2 is an important regulator of CSCs in SKOV3 and OVCAR8 cells. Because ALDH high population (ALDHhigh) has been shown to be enriched for ovarian CSCs [24,25,26,27], we sorted ALDHhigh and ALDHlow cells from SKOV3 and OVCAR8 cells using the ALDEFLUORTM assays and compared their sphere-forming ability. As shown in Fig. 3B, ALDHhigh cells isolated from SKOV3 cells, but not those from OVCAR8 cells, formed more spheres than ALDHlow cells, suggesting that ALDHhigh cells in SKOV3, but not in OVCAR8, are more stem cell-like. Consistently, ALDEFLUORTM assays showed that ZIC2 knockout reduced the ALDHhigh population in SKOV3 (from 11.6% to 1.9%) cells (Fig. 3C). ALDEFLUORTM assays showed that the ALDHhigh population was very low in OVCAR8 cells and it was further decreased in ZIC2 KO cells (from 1.6% to 0.7%) (Fig. 3C).
A Sphere formation assays showed that ZIC2 knockout decreased the number and size of SKOV3 and OVCAR8 spheres. Scale bar: 200 µm. Sphere sizes were measured using ImageJ and expressed as sphere areas. Data are the mean ± SD of three independent experiments. B Sphere formation assays showed that ALDHhigh (ALDH+) cells formed more spheres in SKOV3 cells, but not in OVCAR8 cells, compared to ALDHlow (ALDH−) cells. Data are the mean ± SD of three independent experiments. C ALDEFLUORTM assays showed that ZIC2 knockout reduced the percentage of ALDHhigh (ALDH+) cells in SKOV3 cells (mean ± SD, seven independent experiments) and OVCAR8 cells (mean ± SD, three independent experiments). D In vitro differentiation assays showed that ZIC2 was required to maintain the ALDHhigh population in SKOV3 cells. The images show the data from one experiment. E The histogram shows the average of the percentage of ALDHhigh (ALDH+) cells from two independent experiments. F Immunohistochemistry showed that SKOV3 KO tumors contained fewer ALDH1A1-positive cells compared to WT tumors. Scale bar: 100 μm. Histogram showed the percentage of ALDH1A1-positive (ALDH1A1+) cells per field (average of 6 random fields) *Significant differences (P < 0.05).
Our IF results showed that ZIC2 was expressed in all SKOV3 cells (Fig. 1D), however, ALDEFLUORTM assays showed that only 11.6% of the cells were ALDHhigh (Fig. 3C), suggesting that ZIC2 expression alone is not sufficient to generate CSC cells. To determine whether ZIC2 is required to maintain the CSCs in SKOV3 cells, we sorted ALDHhigh cells from SKOV3 WT and ZIC2 KO cells using the ALDEFLUORTM assays and applied them to in vitro differentiation assays where they were cultured under adherent conditions for up to three days to determine the rate of differentiation of ALDHhigh cells with and without ZIC2. In the first experiment, after three days of culture, 87.5% of the ALDHhigh cells were retained in ZIC2 WT cells (Fig. 3D, top), whereas only 0.7% of the ALDHhigh cells were retained in ZIC2 KO cells (Fig. 3D, bottom). This result was confirmed by the second experiment, indicating that ZIC2 is required to maintain the CSCs in EOC cells. The average of results from the two experiments was shown in Fig. 3E. In addition, IHC showed a decrease in ALDH1A1-positive cells in the xenografted SKOV3 KO tumors compared to SKOV3 WT tumors (Fig. 3F). Because our results showed that ALDHhigh is not a CSC marker for OVCAR8 cells (Fig. 3B), we wanted to determine whether CD133high (CD133+), another CSC marker in EOC [28], can be a CSC marker for OVCAR8. However, sphere formation assays showed that CD133high cells isolated from SKOV3 and OVCAR8 cells did not form more spheres than CD133low cells (Supplementary Fig. S2), suggesting that CD133high is not enriched for CSCs in these two cell lines. Taken together, our results demonstrate a critical role for ZIC2 in maintaining the CSC population in EOC cells.
ZIC2 overexpression causes variable effects on tumorigenic phenotypes in EOC cells
Having shown that ZIC2 knockout mitigated tumorigenic phenotypes, we wanted to determine whether overexpression of ZIC2 in EOC cell lines that do not express endogenous ZIC2 would promote tumorigenic phenotypes. ZIC2 overexpression in A2780 and OVCAR3 cells was confirmed by immunoblotting (Fig. 4A). Unexpectedly, ZIC2 overexpression caused inconsistent effects on tumorigenic phenotypes. Neutral red uptake assays showed that ZIC2 overexpression decreased the growth of OVCAR3 and A2780 cells (Fig. 4B). Clonogenic assays showed that overexpression of ZIC2 increased the number, without affecting the size of OVCAR3 colonies, but decreased the number and size of A2780 clones (Fig. 4C). Furthermore, soft agar assays showed that overexpression of ZIC2 decreased the number of A2780 colonies in soft agar (Fig. 4D). Of note, OVCAR3 cells did not form colonies in soft agar. Transwell migration assays showed that ZIC2 overexpression increased migration in both A2780 and OVCAR3 cells (Fig. 4E). Finally, two independent subcutaneous xenograft experiments showed that overexpression of ZIC2 led to formation of smaller OVCAR3 tumors in mice (Fig. 4F–H and Supplementary Fig. S3). IHC showed that the ZIC2 overexpression did not change percentage of Ki67-positive cells in these tumors (Fig. 4I). Taken together, our results show that overexpression of ZIC2 in EOC cells that express little endogenous ZIC2 causes variable biological effects in a cell type- and context-dependent manner.
A ZIC2 overexpression in A2780 and OVCAR3 cells was confirmed by immunoblotting using an anti-FLAG antibody. β-actin was the loading control. B Neutral red uptake assays showed that ZIC2 overexpression decreased the growth of OVCAR3 and A2780 cells. C Clonogenic assays showed that ZIC2 overexpression increased the number of OVCAR3 colonies but decreased the number and size of A2780 colonies. D Soft agar assays showed that overexpression of ZIC2 reduced the number, but not the size of A2780 colonies. Scale bar: 200 μm. E Transwell migration assays showed that overexpression of ZIC2 increased the migration of OVCAR3 and A2780 cells. Scale bar: 100 μm. For A–E, data are mean ± SD of three independent experiments. *Significantly different (P < 0.05). F–H The subcutaneous xenograft model showed that ZIC2 overexpression reduced the growth, weight, and size of OVCAR3 tumors (n = 8 for EV and OE tumors). Scale bar: 10 mm. I Immunohistochemistry showed no change in the percentage of Ki67-positive cells in EV and OE tumors. Scale bar: 100 μm. The histogram showed the percentage of Ki67-positive (Ki-67+) cells per field (average of 6 random fields). *Significant differences (P < 0.05). EV empty vector, OE ZIC2 overexpression.
ZIC2 overexpression enhances the sphere-forming capacity in EOC cells
We then examined the effect of ZIC2 overexpression on the CSC phenotypes in EOC cells. Sphere formation assays showed that ALDHhigh cells isolated from OVCAR3 and A2780 cells formed more spheres relative to their respective ALDHlow cells, suggesting that ALDHhigh cells are more CSC-like in these two cell lines (Fig. 5A). ZIC2 overexpression increased the number of OVCAR3 and A2780 spheres, without significantly affecting the sphere sizes (Fig. 5B), which was consistent with an increase of the ALDHhigh cells from 2.2% to 7.1% in OVCAR3 cells and from 0.3% to 1.3% in A2780 cells (Fig. 5C). We then wanted to determine whether overexpression of ZIC2 will help maintaining the ALDHhigh population in EOC cells. We used A2780 cells for the differentiation assays because ALDHhigh cells can be readily enriched in A2780 cells when cultured in sphere-forming medium for 7 days (comparing the percentage of ALDHhigh cells on day 0 in Fig. 5D with that in Fig. 5C). In vitro differentiation assays showed that ZIC2 overexpression significantly slowed the loss of ALDHhigh cells when cultured in adherent conditions in regular culture medium (Fig. 5D, E), indicating that ZIC2 is required to maintain the ALDHhigh cells in A2780 cells. However, IHC showed that ZIC2 overexpression did not significantly change ALDH1A1 protein levels and the number of ALDH1A1-positive cells in OVCAR3 tumors (Fig. 5F). We also examined the effect of ZIC2 overexpression on the CD133high population. Sphere formation assays showed that CD133high cells formed more spheres in OVCAR3, but not in A2780 cells, relative to CD133low cells (Supplementary Fig. S4A), suggesting that CD133high is a CSC marker for OVCAR3 cells, but not for A2780 cells. ZIC2 overexpression increased CD133high cells in OVCAR3 from 3.1% to 7.0% (Supplementary Figs. S4B, C). Taken together, our results show that ZIC2 overexpression increases the number of CSC-like cells in EOC cells.
A Sphere formation assays showed that ALDHhigh cells are more capable of forming spheres compared to ALDHlow cells in OVCAR3 and A2780 cells. B Sphere formation assays showed that ZIC2 overexpression increased the number, but not the size, of OVCAR3 and A2780 spheres. Scale Bar: 200 µm. For A, B, data are the mean ± SD of three independent experiments. C ALDEFLUORTM assays showed that ZIC2 overexpression increased the percentage of ALDHhigh (ALDH+) cells in OVCAR3 cells (mean ± SD, three independent experiments) and A2780 cells (mean ± SD, five independent experiments). D In vitro differentiation assays showed that ZIC2 was required to maintain ALDHhigh (ALDH+) cells in A2780 cells. The images showed the data from one experiment. E The histogram showed the average percentage of ALDHhigh (ALDH+) cells from three independent experiments. Data are the mean ± SD of three independent experiments. F Immunohistochemistry showed that ZIC2 overexpression did not change in the percentage of ALDH1A1-positive cells in OVCAR3 tumors. The histogram showed the percentage of ALDH1A1-positive (ALDH1A1+) cells per field (average of 6 random fields). Scale bar: 100 μm. *Significant differences (P < 0.05).
ZIC2 regulates genes associated with a variety of biological processes in EOC cells
To elucidate the mechanisms underlying the biological functions of ZIC2, we performed RNA-sequencing (RNA-seq) to characterize the transcriptome regulated by ZIC2 in SKOV3 and OVCAR3 cells. In SKOV3 cells, ZIC2 knockout altered the expression of 6,565 genes (FDR < 0.05) (Supplementary Fig. S5A–C), whereas in OVCAR3 cells, ZIC2 overexpression altered the expression of 10,498 genes (FDR < 0.05) (Supplementary Fig. S5D–F), confirming that ZIC2 is a pivotal regulator of gene expression in EOC cells. The top 50 differentially expressed genes ranked by FDR in SKOV3 and OVCAR3 cells were shown in the heatmaps (Fig. 6A). Gene Set Enrichment Analysis (GSEA) showed that ZIC2 regulated a variety of biological processes and signaling pathways (Fig. 6B). The enriched gene sets (from oncogenic signatures and hallmark gene set collections) common to both SKOV3 KO and OVCAR3 OE models are shown in Fig. 6C (KRAS.600_UP.V1_up, BMI1_DN-MEL18_DN.V1_UP, E2F3_UP.V1_UP, and HALLMARK_WNT_BETA_CATENIN_SIGNALING). By comparing the genes altered in the SKOV3 KO and OVCAR3 OE models, we classified ZIC2-regulated genes into three categories: (i) commonly regulated genes whose regulation by ZIC2 is consistent between KO and OE models; (ii) oppositely regulated genes whose regulation by ZIC2 is opposite between KO and OE models; and (iii) independently regulated genes that are regulated by ZIC2 in KO or OE model, but not in both. The expression and proportion of these three classes of genes were variable in the oncogenic signatures and hallmarks that are commonly enriched by ZIC2 in the KO and OE models (Fig. 6D).
A Cluster heatmap of top 50 differentially expressed genes (FDR < 0.05) related to ZIC2 knockout in SKOV3 cells and ZIC2 overexpression in OVCAR3 cells. B GSEA showed the enrichment of gene signatures in SKOV3 WT samples (n = 6) compared with ZIC2 KO samples (n = 6) and OVCAR3 EV samples (n = 6) compared to the OE samples (n = 6) from the RNA-seq analysis. C GSEA enrichment plots showed four gene signatures shared between SKOV3 KO and OVCAR3 OE models. D Rain-cloud plots showed the expression of three categories of genes (i.e., commonly, oppositely, and independently regulated genes) in four gene signatures in SKOV3 KO and OVCAR3 OE models. The percentage of the genes in the respective gene signatures is shown.
We next selected for validation a subset of downregulated genes in SKOV3 KO cells from the RNA-seq analysis that are involved in a variety of biological processes: CSC (ALDH1A1, LIN28B and POSTN), cell cycle (CCND2), EMT (ZEB2), signaling kinases (MAPK4), invasion and metastasis (MMP3 and POSTN), and mRNA binding (IGF2BP1 and LIN28B). RT-qPCR confirmed that all these genes were downregulated in SKOV3 cells when ZIC2 was knocked out (Fig. 7A). Immunoblotting confirmed that ZIC2 knockout decreased the protein level of ALDH1A1, MMP3 and IGF2BP1 (IMP1) in SKOV3 cells (Fig. 7B) and SKOV3 tumors (Fig. 7C). While cyclin D2 was hardly detectable in SKOV3 cells cultured in vitro (Fig. 7B), it was readily detected in SKOV3 WT tumors, but not in ZIC2 KO tumors (Fig. 7C). In OVCAR3 cells, however, at the mRNA level, ZIC2 increased the expression of ALDH1A1, MMP3, LIN28B, and ZEB2, had no effect on the expression of CCND2 and MAPK4, but decreased the expression of IGF2BP1 and POSTN in OVCAR3 cells, confirming that these genes are commonly, oppositely, or independently regulated by ZIC2 in SKOV3 ZIC2 KO and OVCAR3 ZIC2 OE cells (Fig. 7D). At the protein level, ZIC2 overexpression increased the expression of ALDH1A1, but decreased the expression of cyclin D2, MMP3 and IMP1 (IGF2BP1) in OVCAR3 cells or in OVCAR3 tumors (Fig. 7E, F). Taken together, our transcriptomic study showed that ZIC2 regulates multiple biological processes and signaling pathways in a context-dependent manner in EOC.
A Downregulated genes identified by RNA-seq in SKOV3 ZIC2 KO cells were validated at the mRNA level using RT-qPCR. B, C Downregulated genes identified by RNA-seq in SKOV3 ZIC2 KO cells were examined at the protein level using immunoblotting in SKOV3 cells and tumors, respectively. For A and B, data are the mean ± SD of three independent experiments. For C, data are mean ± SD of 7 SKOV3 WT tumors and 5 SKOV3 ZIC2 KO tumors. D Downregulated genes identified by RNA-seq in SKOV3 ZIC2 KO cells were examined in OVCAR3 cells (EV vs. OE) using RT-qPCR. Data are the mean ± SD of five independent experiments. E, F Downregulated genes identified by RNA-seq in SKOV3 ZIC2 KO cells were examined at the protein level using immunoblotting in OVCAR3 cells (EV vs. OE) and OVCAR3 tumors (EV vs. OE), respectively. For E, data are the mean ± SD of three independent experiments. For F, data are the mean ± SD of 6 OVCAR3 EV and 6 OVCAR3 OE tumors. *Significant differences (P < 0.05).
Discussion
Dysregulation of transcription factors contributes to multiple hallmarks of cancer and transcription factors have become promising druggable targets for cancer treatment [29]. In this study, we demonstrate that high expression of transcription factor ZIC2 is associated with poor overall and post-progression survival of EOC patients. Our results from the ZIC2 knockout model showed that ZIC2 is required to maintain the tumorigenic phenotypes associated with both bulk cells and CSCs in EOC cells expressing endogenous ZIC2, suggesting that ZIC2 can be a potential therapeutic target to treat the EOCs that express a high level of ZIC2.
Our findings indicate that the role of ZIC2 in EOC is context-dependent, which is manifested by the variable effects of ZIC2 we observed between different cell lines and experimental models. The context-dependent functions of ZIC2 could be due to the variable chromatin accessibility to the ZIC2 target genes caused by epigenetic heterogeneity and/or the availability of ZIC2 interacting proteins (e.g., transcription factors and co-factors) in each cell line. In contrast to the ZIC2 dependency observed in EOC cell lines that express endogenous ZIC2, overexpression of exogenous ZIC2 in EOC cell lines that have evolved and adapted to survive without ZIC2 may confer certain advantages but can also be detrimental to the cells. There is evidence that ZIC2 acts both as a transcription factor and as a co-factor to regulate gene transcription [12, 30]. We propose that whereas the endogenous ZIC2 is a critical factor to maintain or promote tumorigenic phenotypes in EOC cells, overexpressed ZIC2 might compete with other transcription factors for recruiting co-factors or compete with other co-factors for binding to transcription factors. This may consequently disrupt the expression of genes required for vital biological processes and the well-established homeostasis, which could explain the conflicting effects of ZIC2 observed in the knockout and overexpression models (Fig. 8). In keeping with this, overexpression of ZIC2 in ZIC2 KO SKOV3 cells did not affect cell proliferation and colony formation, as determined by the neutral red uptake and clonogenic assays, but did result in slightly increased sphere-forming ability (Supplementary Fig. S6). Re-expression of a gene may not necessarily fully restore the functions of the deleted gene in the knockout model, particularly when the factor alters cell fate, as expected of ZIC2 [17, 21, 22]. For example, long term exposure to TGF-β results in a stable mesenchymal phenotype through epigenetic mechanisms, and it cannot be reverted into the epithelial state by simply withdrawing TGF-β [31, 32]. We believe that a similar phenomenon may explain the inability of ZIC2 overexpression to fully rescue the effects of ZIC2 knockout.
We propose that ZIC2 can act as a transcription factor (A, C) or a co-factor (B, D) in EOC. A, B when endogenous ZIC2 is knocked out from EOC cells, the expression of the genes regulated by ZIC2 as a transcription factor (A) or as a co-activator (B) will be abolished or impaired. C, D when ZIC2 is overexpressed in ZIC2-negative EOC cells, it will increase the expression of ZIC2-regulated genes as a transcription factor or a co-activator, and meanwhile, it could also disrupt the expression of the genes regulated by other transcription factors through competing for the co-activators (C) or through competing for binding to the transcription factors with other co-activators (D). Consequently, deletion of endogenous ZIC2 and overexpression of ZIC2 in ZIC2-negative cells may have similar and opposing effects depending on the contextual environment in the cells as shown in this study. TF transcription factor.
Given the variable effects of ZIC2 knockout in SKOV3 and overexpression in OVCAR3 cells, we hypothesized that ZIC2 regulates distinct transcriptomes in SKOV3 and OVCAR3 cells. RNA-seq data showed that ZIC2 regulates the expression of thousands of genes in both cell lines, indicating that ZIC2 is a pivotal regulator of gene expression in EOC. Analysis of the transcriptomic data showed that ZIC2-regulated genes are involved in multiple biological processes and signaling pathways that are associated with tumor progression. Four gene signatures are commonly enriched in ZIC2 knockout and overexpression models. However, the expression and proportions of ZIC2-regulated genes (e.g., the commonly, oppositely, and independently regulated genes) within the four gene signatures are different between ZIC2 knockout and overexpression models, reflecting the varying effects of ZIC2 observed in the two models.
ZIC2 has been shown to promote tumorigenic phenotypes by activating several signaling pathways in human cancers [15, 30, 33]. For example, ZIC2 promotes PAK4 expression and cell growth through activation of the Raf/MEK/ERK pathway in hepatocellular carcinoma [15] and promotes the self-renewal of CSCs via activation of the PI3K/AKT pathway in nasopharyngeal carcinoma [33]. Our transcriptomic analysis suggests that ZIC2 might be involved in KRAS, BMI and MEL18 related, E2F3-related, and Wnt/β-catenin signaling in EOC, all of which are well known to be closely associated with cancer aggressiveness. This is the first comprehensive analysis of the ZIC2-regulated transcriptome in cancer, providing a clue towards a better understanding of the biological functions of ZIC2 in EOC.
CSCs are a small subpopulation of cells that can self-renew and differentiate into different lineages of cells to give rise to heterogeneous tumors and are more resistant to therapies [7, 8]. Thus, more efficacious treatment should be developed to target CSCs based on an improved understanding of the molecular mechanisms that govern the biology of CSCs. In this study, we demonstrate that ZIC2 is required for maintaining the CSC populations by limiting their differentiation in both ZIC2 knockout and overexpression models, indicating that ZIC2 is a critical regulator of CSCs in EOC. Our findings are in line with the reported role of ZIC2 in regulating normal stem cells and CSCs in liver cancer [17, 21, 22] and suggest that ZIC2 could be a novel therapeutic target to reduce the CSCs in EOC.
There are limitations to this study. First, the detailed molecular mechanisms underlying the context-dependent functions of ZIC2 need to be further explored, which will involve genome-wide analysis of the epigenetic landscapes and chromatin accessibility in the EOC cells using chromatin immunoprecipitation sequencing (ChIP-seq) and the assay for transposase-accessible chromatin with sequencing (ATAC-seq), as well as ZIC2-interactome using Mass-spectrometry and proximity-dependent biotin identification (BioID). Secondly, in this study we examined the effect of ZIC2 knockout and overexpression on tumor growth in mice by injecting a large number of SKOV3 and OVCAR3 cells into mice. However, given the critical role of ZIC2 in maintaining the CSC populations demonstrated by others and us, it would be important to determine the effect of ZIC2 on the CSC frequency and tumorigenicity of EOC cells by performing in vivo limiting dilution assay. Furthermore, our results showed that overexpression of ZIC2 increased sphere formation and ALDHhigh population in OVCAR3 cells in vitro, but inhibited cell growth in vitro and tumor growth in vivo. It is likely that the effect of overexpressed ZIC2 on CSCs is overridden by its growth inhibitory effect, however, this needs to be further investigated.
In conclusion, this study demonstrates that ZIC2 expression is associated with poor outcomes in EOC patients and that ZIC2 plays a pro-tumorigenic role in EOCs that express endogenous ZIC2 by regulating the expression of genes involved in a variety of biological processes, suggesting that ZIC2 may be a therapeutic target for the treatment of EOC expressing high levels of ZIC2. Loss of function mutations of ZIC2 are not lethal, and transcription factors are becoming increasingly targetable for cancer therapy [34,35,36,37]. Hence, ZIC2 may be an attractive and well-tolerated target for the treatment of EOC. A better understanding of the context-dependent functions of ZIC2 would help develop strategies for targeting ZIC2 in EOC.
Materials and methods
Kaplan–Meier analysis and GEPIA 2 analysis
The association between ZIC2 expression and overall survival, progression-free survival, and post-progression survival of high grade serous EOC patients was analyzed using Kaplan–Meier Plot (kmplot.com). The Kaplan–Meier Plotter compiled the ovarian cancer cohorts with transcriptome level gene expression data and clinical follow-up from public repositories into an integrated database [38]. For the analysis, ZIC2-high was defined by upper quartile (top 25%); follow-up threshold was set to 60 months; and “Stage” was set to “2 + 3 + 4”. P values were generated automatically using the Kaplan–Meier method with the log-rank test. The differences in ZIC2 expression in normal versus EOC tissues were analyzed using the Gene Expression Profiling Interactive Analysis 2 (GEPIA2), which used the database from the TCGA and GTEx projects [39]. Specifically, we used the “Expression DIY” function to compare the expression level of ZIC2 mRNA in normal ovarian tissues and ovarian cancer and chose “Box Plot” to generate the box plot.
Cell lines
SKOV3 (ATCC) and A2780 (gift from Dr. Benjamin Tsang, Ottawa Hospital Research Institute) were cultured in DMEM/Hams’ F12 medium supplemented with 5% FBS. OVCAR8 (gift from Dr. Trevor Shepherd, University of Western Ontario) and OVCAR3 (ATCC) were cultured in RMPI-1640 medium supplemented with 10% FBS. All cell lines were identified by short tandem repeat (STR) genotyping analysis and monitored for mycoplasma using the Universal Mycoplasma Test Kit (ATCC 30-1012KTM, ATCC). All culture media were supplemented with penicillin (100 U/mL) and streptomycin (100 μg/mL). Information on other cell lines is listed in Supplementary Table S1.
ZIC2 knockout with CRISPR/Cas9
ZIC2 in SKOV3 and OVCAR8 cells was knocked out using the All_in_one_CRISPR/Cas9_LacZ plasmid (Cat#74293, Addgene) by inserting a g-Block fragment containing a ZIC2-specific guide RNA (gRNA). The DNA sequences of its guide RNAs (gRNA) targeting the human ZIC2 gene are: gRNA #1: AGTAGGAGCCAACGTG (forward strand: 5′–3′); gRNA #2: CGCGCCCGGGTTGAGCTTGA (reverse strand: 3′–5′). After transfection with plasmids containing sgRNA1 and sgRNA2, mCherry-positive cells were sorted into 96-well plates at a density of 1 cell/well. Clones derived from the single cells were screened by immunoblotting for ZIC2 expression and confirmed by Sanger sequencing of genomic DNA. Clones proven to be ZIC2 intact and have lost ZIC2 expression were used as wild-type (WT) and knockout (KO) clones, respectively. Three WT or KO clones were maintained separately and pooled at a 1:1:1 ratio to generate a mix of WT and KO clones for the experiments, which were defined as WT and KO cells, respectively. All ZIC2 KO experiments were performed using the mix of three WT vs. the mix of three KO clones.
ZIC2-FLAG overexpression
HEK293T cells were transfected with a lentiviral plasmid pLenti-ZIC2-C-Myc-DDK-P2A-Puro (Cat#RC220798L3, Origene) or an empty vector plasmid pLenti-C-mGFP-P2A-Puro (Cat#PS100093V, Origene), a packaging plasmid psPAX2 (#12260, Addgene), and an envelope plasmid pMD2.G (#12259, Addgene) using Fugene HD (Promega) transfection reagent in a 1:2:2 molar ratio. Three days post-transfection, the virus-containing medium was collected, filtered, and used to infect A2780s and OVCAR3 cells. After infection, cells were selected with puromycin to obtain stable ZIC2-overexpression (OE) and empty vector control (EV) cells.
Immunoblotting
Cells were lysed with RIPA buffer and immunoblotting was carried out as previously described [40]. Primary antibodies were purchased from Abcam [ZIC2 (ab150404) and cyclin D2 (ab207604)], Cell Signaling Technology [IMP1 (#84821), MMP3 (#14351), ALDH1A1 (#54135), Ki-67 (#9449)], and Sigma-Aldrich [β-actin (A5441) and FLAG M2 (F3165)]. The secondary antibodies are IRDyde 800CW anti-rabbit (926-32211, LI-COR Bioscience) or anti-mouse (926-32210, LICOR Bioscience) secondary antibody. Images of immunoblotting were obtained and quantified using the LI-COR Odyssey Imaging system (LICOR Bioscience).
Immunofluorescence microscopy
ZIC2 expression and intracellular localization in EOC cells were examined by immunofluorescence (IF) as previously described [40]. Fixed cells grown on the coverslips were immunostained with anti-ZIC2 antibody at a 1:1000 dilution, followed by Alexa Fluor® 594 anti-rabbit secondary antibodies (R37119, Invitrogen) at a 1:400 dilution. DAPI staining (1 μg/mL) was used to detect nuclei. Coverslips were mounted onto slides and images were captured using a Zeiss LSM 710 confocal microscope (ZEISS) at 20X magnification and processed using the Zeiss ZEN software.
Immunohistochemistry (IHC)
IHC of formalin-fixed and paraffin-embedded patient-derived xenografts (PDX-550 and PDX-899) and xenografted SKOV3 and OVCAR3 tumors were performed as described previously [41]. The concentration of the antibodies was 1:1,000, 1:500, and 1:1,000 for ZIC2, Ki-67, and ALDH1A1, respectively. Images of tumor sections were acquired on an Aperio digital pathology slide scanner (Leica Microsystems).
Neutral red uptake assay
The neutral red uptake assay was performed as previously described [42]. SKOV3, OVCAR8, and A2780 cells at 1000 cells/well and OVCAR3 cells at 2000 cells/well were seeded in 96-well plates for the assay and cultured in the incubator for 1, 3, 5, and 7 days. Cell growth was expressed as the fold change relative to day 1.
Clonogenic assay
The clonogenic assay was conducted as previously described [42]. Cells were seeded in 6-well plates at 200 cells/well and cultured for 10 days. Colonies were visualized by crystal violet staining and counted. The size of colonies was measured using ImageJ software with the parameter “Threshold” set to “Auto” and other settings to default.
Soft agar assay
5000 cells in 0.35% top agar were layered above an agar base (0.7%) in 6-well plates and cultured for 2 to 3 weeks. Colonies were visualized with crystal violet. Colonies containing more than 50 cells in 18 random regions were counted under a light microscope (EVOS FL, AMG), and the size of the colonies was measured using ImageJ software as described above.
Transwell migration assay
The transwell migration assay was conducted as previously described [40]. 5 × 104 cells in serum-free medium were added to the top chamber placed in a 24-well plate and allowed to migrate towards the medium containing 10% FBS in the lower chamber for 24 h, and then fixed and stained with crystal violet. The unmigrated cells on the upper side of the membrane were wiped off with a cotton swab. The number of migrated cells was counted under a light microscope.
Subcutaneous xenograft models
SKOV3 WT and ZIC2 KO cells (2 × 106), OVCAR8 WT and ZIC2 KO cells (2 × 106), or OVCAR3 EV and OVCAR3 ZIC2 OE cells (1 × 107) were mixed with an equal volume of Matrigel and injected subcutaneously into both flanks of female NOD scid gamma (NSG) mice. Tumor growth was measured weekly with a digital caliper. Tumor volume was calculated using the formula of (length × width2)/2. Once the largest tumors reached 1 cm3, all mice were euthanized. Tumors were collected, weighed, and processed for subsequent analysis. The animal experiments were conducted with the approval of the University of Alberta Animal Care and Use Committee (AUP000004444), Edmonton, Canada, in accordance with guidelines from the Canadian Council for Animal Care.
ALDEFLUORTM assay
The percentage of ALDHhigh (aldehyde dehydrogenase/ALDH+) cells was determined by ALDEFLUORTM assay using the ALDEFLUORTM assay kit (01700, STEMCELL Technologies). Briefly, 5 µL of activated FITC-conjugated ALDEFLUORTM reagent was added to 1 mL of ALDEFLUORTM buffer containing 1 × 106 cells, mixed, immediately dispensed at an equal volume into ALDH negative control tubes containing ALDH inhibitor N,N-diethylaminobenzaldehyde (DEAB) and ALDH sample tubes, and then incubated in a 37 °C water bath for 40 min. Afterwards, cells were centrifuged and resuspended in 0.5 mL ALDEFLUORTM buffer for flow cytometry or fluorescence-activated cell sorting (FACS). The cells treated with the ALDH inhibitor (DEAB) were used as ALDH-negative control cells to set up gating for sorting ALDHhigh cells. Flow cytometry was performed on a BD LSRFortessa X-20 analyzer (BD Biosciences), and FACS was performed on a BD FACSAriaTM III (BD Biosciences). Data were collected and analyzed by FlowJo 10.4 (TreeStar).
Sphere formation assay
Cells were processed into single cells and seeded at various density (200, 150, 120, 100, 75, 50, 25, 20, 10, 5, 2, 1 cells per well) onto the ultra-low attachment 96-well plates and cultured in DMEM/F12 (SKOV3 and A2780) or RPMI-1640 (OVCAR8 and OVCAR3) medium supplemented with 10 ng/mL EGF (R&D System, cat#236-EG-01M), 10 ng/mL FGF (R&D System, cat#3718-GMP), and 1X B-27 supplement (Invitrogen cat# 17504044) for 11 days. The formed spheres were counted under a light microscope and images were captured using an EVOS FL microscope. Sphere size was measured using ImageJ software as described for the clonogenic assay.
In vitro differentiation assay
For SKOV3 cells, ALDHhigh cells isolated from SKOV3 WT cells and ZIC2 KO cells using the ALDEFLUORTM assay kit were cultured under regular adherent conditions for 0, 1, 2, and 3 days. The percentage of ALDHhigh cells in these cells at each time point was then determined by flow cytometry using the ALDEFLUORTM assay kit. For A2780 cells, ALDHhigh cells in A2780 EV and A2780 ZIC OE cells were first enriched in sphere-forming conditions and then isolated by FACS using the ALDEFLUORTM assay kit and used for the differentiation assay as described above.
RNA-sequencing and data analysis
RNAs isolated using the Qiagen RNeasy Mini Kit (74104, Qiagen) were submitted to McGill University and the Quebec Centre for Genomic Innovation for cDNA library construction and high-throughput sequencing. Briefly, RNA samples were collected from the mix of three WT vs. the mix of three KO clones, as well as OVCAR3 EV vs. OVCAR3 ZIC2 OE cells, in six independent experiments to generate a total of 6 biological replicates for each cell type. RNA quality and quantity were measured by an Agilent bioanalyzer, and NEB mRNA stranded libraries were prepared and subsequently sequenced by Illumina HiSeq for SKOV3 samples and NovaSeq for OVCAR3 samples. The quality of the raw sequencing data was analyzed using FastQC (Andrews S. (2010). FastQC: a quality control tool for high throughput sequence data (available online at: http://www.bioinformatics.babraham.ac.uk/projects/fastqc) and adapter sequences were removed using Skewer [43]. STAR (a rapid RNA-seq read mapper) [44] was used to align reads to the GRCh38/hg38 human reference genome using Gencode gene annotations and gene-based read counts were obtained with FeatureCounts [45]. The read counts were then used for pairwise differential gene expression analysis (SKOV3 WT vs. SKOV3 KO cells, and OVCAR3 EV vs. OVCAR3 OE cells) using the R package, edgeR [46]. Differentially expressed genes with a false discovery rate (FDR) < 0.05 were considered significant. Raw RNA-seq files and read count data from SKOV3 WT vs KO and OVCAR3 EV vs. OE models were deposited in the Gene Expression Omnibus (GEO) database as GSE227395.
Gene set enrichment analysis
Gene set enrichment analysis (GSEA) was performed using GSEA 4.1.0 software. The source selection for the Gene set collections is as follows: hallmark gene sets (h.all.v7.4.symbols.gmt), ontology gene sets (biological processes-c5.go.bp.v7.4.symbols.gmt), signaling pathways (curated gene sets-canonical pathways-c2.cp.v7.4.symbols.gmt), and oncogenic signature gene sets (c6.all.v7.4.symbols.gmt). Differentially expressed genes with FDR < 0.05 were included in GSEA, and all enriched gene sets with FDR < 0.25 were considered significant according to the use guide of GSEA).
RNA isolation and reverse transcription-quantitative PCR (RT-qPCR)
RNA isolation and RT-qPCR were performed as previously described [40]. PCR primer sequences are listed in Supplementary Table S2.
Statistical analysis
All experiments in this study were repeated independently at least three times unless otherwise noted. Statistical significance analysis was performed using GraphPad 6.0 (Prism). Statistical significance between the two groups was determined by the two-tailed unpaired t-test, defined as P < 0.05.
Data availability
RNA-sequencing data have been deposited in NCBI’s Gene Expression Omnibus (GEO) database and are accessible through GEO Series accession number GSE227395.
References
Siegel RL, Miller KD, Fuchs HE, Jemal A. Cancer statistics, 2022. CA Cancer J Clin. 2022;72:7–33.
Testa U, Petrucci E, Pasquini L, Castelli G, Pelosi E. Ovarian cancers: genetic abnormalities, tumor heterogeneity and progression, clonal evolution and cancer stem cells. Medicines. 2018;5:16.
Kroeger PT Jr, Drapkin R. Pathogenesis and heterogeneity of ovarian cancer. Curr Opin Obstet Gynecol. 2017;29:26–34.
Bowtell DD, Bohm S, Ahmed AA, Aspuria PJ, Bast RC Jr, Beral V, et al. Rethinking ovarian cancer II: reducing mortality from high-grade serous ovarian cancer. Nat Rev Cancer. 2015;15:668–79.
Zong X, Nephew KP. Ovarian cancer stem cells: role in metastasis and opportunity for therapeutic targeting. Cancers. 2019;11:934.
Terraneo N, Jacob F, Dubrovska A, Grunberg J. Novel therapeutic strategies for ovarian cancer stem cells. Front Oncol. 2020;10:319.
Shackleton M, Quintana E, Fearon ER, Morrison SJ. Heterogeneity in cancer: cancer stem cells versus clonal evolution. Cell. 2009;138:822–9.
Tang DG. Understanding cancer stem cell heterogeneity and plasticity. Cell Res. 2012;22:457–72.
Berdasco M, Esteller M. Aberrant epigenetic landscape in cancer: how cellular identity goes awry. Dev Cell. 2010;19:698–711.
Houtmeyers R, Souopgui J, Tejpar S, Arkell R. The ZIC gene family encodes multi-functional proteins essential for patterning and morphogenesis. Cell Mol Life Sci. 2013;70:3791–811.
Ali RG, Bellchambers HM, Arkell RM. Zinc fingers of the cerebellum (Zic): transcription factors and co-factors. Int J Biochem Cell Biol. 2012;44:2065–8.
Chan DW, Liu VW, Leung LY, Yao KM, Chan KK, Cheung AN, et al. Zic2 synergistically enhances Hedgehog signalling through nuclear retention of Gli1 in cervical cancer cells. J Pathol. 2011;225:525–34.
Sakuma K, Kasamatsu A, Yamatoji M, Yamano Y, Fushimi K, Iyoda M, et al. Expression status of Zic family member 2 as a prognostic marker for oral squamous cell carcinoma. J Cancer Res Clin Oncol. 2010;136:553–9.
Marchini S, Poynor E, Barakat RR, Clivio L, Cinquini M, Fruscio R, et al. The zinc finger gene ZIC2 has features of an oncogene and its overexpression correlates strongly with the clinical course of epithelial ovarian cancer. Clin Cancer Res. 2012;18:4313–24.
Lu SX, Zhang CZ, Luo RZ, Wang CH, Liu LL, Fu J, et al. Zic2 promotes tumor growth and metastasis via PAK4 in hepatocellular carcinoma. Cancer Lett. 2017;402:71–80.
Inaguma S, Ito H, Riku M, Ikeda H, Kasai K. Addiction of pancreatic cancer cells to zinc-finger transcription factor ZIC2. Oncotarget. 2015;6:28257–68.
Zhu P, Wang Y, He L, Huang G, Du Y, Zhang G, et al. ZIC2-dependent OCT4 activation drives self-renewal of human liver cancer stem cells. J Clin Investig. 2015;125:3795–808.
Xiao P, Meng Q, Liu Q, Lang Q, Yin Z, Li G, et al. IGF2BP1-mediated N6-methyladenosine modification promotes intrahepatic cholangiocarcinoma progression. Cancer Lett. 2023;557:216075.
Liu F, Shi Z, Bao W, Zheng J, Chen K, Lin Z, et al. ZIC2 promotes colorectal cancer growth and metastasis through the TGF-beta signaling pathway. Exp Cell Res. 2022;415:113118.
Liu ZH, Chen ML, Zhang Q, Zhang Y, An X, Luo YL, et al. ZIC2 is downregulated and represses tumor growth via the regulation of STAT3 in breast cancer. Int J Cancer. 2020;147:505–18.
Luo Z, Gao X, Lin C, Smith ER, Marshall SA, Swanson SK, et al. Zic2 is an enhancer-binding factor required for embryonic stem cell specification. Mol Cell. 2015;57:685–94.
Matsuda K, Mikami T, Oki S, Iida H, Andrabi M, Boss JM, et al. ChIP-seq analysis of genomic binding regions of five major transcription factors highlights a central role for ZIC2 in the mouse epiblast stem cell gene regulatory network. Development. 2017;144:1948–58.
Frank CL, Liu F, Wijayatunge R, Song L, Biegler MT, Yang MG, et al. Regulation of chromatin accessibility and Zic binding at enhancers in the developing cerebellum. Nat Neurosci. 2015;18:647–56.
Silva IA, Bai S, McLean K, Yang K, Griffith K, Thomas D, et al. Aldehyde dehydrogenase in combination with CD133 defines angiogenic ovarian cancer stem cells that portend poor patient survival. Cancer Res. 2011;71:3991–4001.
Kryczek I, Liu S, Roh M, Vatan L, Szeliga W, Wei S, et al. Expression of aldehyde dehydrogenase and CD133 defines ovarian cancer stem cells. Int J Cancer. 2012;130:29–39.
Landen CN Jr, Goodman B, Katre AA, Steg AD, Nick AM, Stone RL, et al. Targeting aldehyde dehydrogenase cancer stem cells in ovarian cancer. Mol Cancer Ther. 2010;9:3186–99.
Wang YC, Yo YT, Lee HY, Liao YP, Chao TK, Su PH, et al. ALDH1-bright epithelial ovarian cancer cells are associated with CD44 expression, drug resistance, and poor clinical outcome. Am J Pathol. 2012;180:1159–69.
Curley MD, Therrien VA, Cummings CL, Sergent PA, Koulouris CR, Friel AM, et al. CD133 expression defines a tumor initiating cell population in primary human ovarian cancer. Stem Cells. 2009;27:2875–83.
Bushweller JH. Targeting transcription factors in cancer - from undruggable to reality. Nat Rev Cancer. 2019;19:611–24.
Ishiguro A, Ideta M, Mikoshiba K, Chen DJ, Aruga J. ZIC2-dependent transcriptional regulation is mediated by DNA-dependent protein kinase, poly(ADP-ribose) polymerase, and RNA helicase A. J Biol Chem. 2007;282:9983–95.
Gregory PA, Bracken CP, Smith E, Bert AG, Wright JA, Roslan S, et al. An autocrine TGF-beta/ZEB/miR-200 signaling network regulates establishment and maintenance of epithelial-mesenchymal transition. Mol Biol Cell. 2011;22:1686–98.
Celia-Terrassa T, Bastian C, Liu DD, Ell B, Aiello NM, Wei Y, et al. Hysteresis control of epithelial-mesenchymal transition dynamics conveys a distinct program with enhanced metastatic ability. Nat Commun. 2018;9:5005.
Lv B, Li F, Liu X, Lin L. The tumor-suppressive role of microRNA-873 in nasopharyngeal carcinoma correlates with downregulation of ZIC2 and inhibition of AKT signaling pathway. Cancer Gene Therapy. 2021;28:74–88.
Yeh JE, Toniolo PA, Frank DA. Targeting transcription factors: promising new strategies for cancer therapy. Curr Opin Oncol. 2013;25:652–8.
Mapp AK, Pricer R, Sturlis S. Targeting transcription is no longer a quixotic quest. Nat Chem Biol. 2015;11:891–4.
Bhagwat AS, Vakoc CR. Targeting transcription factors in cancer. Trends Cancer. 2015;1:53–65.
Hagenbuchner J, Ausserlechner MJ. Targeting transcription factors by small compounds-current strategies and future implications. Biochem Pharmacol. 2016;107:1–13.
Gyorffy B. Discovery and ranking of the most robust prognostic biomarkers in serous ovarian cancer. Geroscience. 2023;45:1889–98.
Tang Z, Kang B, Li C, Chen T, Zhang Z. GEPIA2: an enhanced web server for large-scale expression profiling and interactive analysis. Nucleic Acids Res. 2019;47:W556–W560.
Zhou J, Jain S, Azad AK, Xu X, Yu HC, Xu Z, et al. Notch and TGFbeta form a positive regulatory loop and regulate EMT in epithelial ovarian cancer cells. Cell Signal. 2016;28:838–49.
Dieters-Castator D, Dantonio PM, Piaseczny M, Zhang G, Liu J, Kuljanin M, et al. Embryonic protein NODAL regulates the breast tumor microenvironment by reprogramming cancer-derived secretomes. Neoplasia. 2021;23:375–90.
Barghout SH, Zepeda N, Vincent K, Azad AK, Xu Z, Yang C, et al. RUNX3 contributes to carboplatin resistance in epithelial ovarian cancer cells. Gynecol Oncol. 2015;138:647–55.
Jiang H, Lei R, Ding SW, Zhu S. Skewer: a fast and accurate adapter trimmer for next-generation sequencing paired-end reads. BMC Bioinformatics. 2014;15:182.
Dobin A, Davis CA, Schlesinger F, Drenkow J, Zaleski C, Jha S, et al. STAR: ultrafast universal RNA-seq aligner. Bioinformatics. 2013;29:15–21.
Liao Y, Smyth GK, Shi W. featureCounts: an efficient general purpose program for assigning sequence reads to genomic features. Bioinformatics. 2014;30:923–30.
Robinson MD, McCarthy DJ, Smyth GK. edgeR: a Bioconductor package for differential expression analysis of digital gene expression data. Bioinformatics. 2010;26:139–40.
Acknowledgements
This research has been funded by the Alberta Women’s Health Foundation through the Women and Children’s Health Research Institute (WCHRI) to YXF; funding from the Alberta Cancer Foundation and WCHRI to L-MP. L-MP is the Sawin-Baldwin Chair in Ovarian Cancer & Dr. Anthony Noujaim Legacy Oncology Chair and the Alberta Innovates Health Solutions Translational Chair in Cancer. HC was supported by graduate studentships from the Li Ka Shing Foundation and Yau Family Foundation. LJL was supported by the Alberta Innovates MD-PhD Studentship, CIHR Doctoral Research Award and Alberta Cancer Foundation Graduate Studentship. We thank Dr. Xuejun Sun at the Cell Imaging and Flow Cytometry Facility of the Oncology Department at the University of Alberta at the Cross Cancer Institute for cell imaging and sorting and Dr. Aja Rieger in the flow cytometry facility of the University of Alberta.
Author information
Authors and Affiliations
Contributions
H. Chen: Formal analysis, investigation, methodology, validation, writing-original draft. L.J. Lee: Formal analysis and methodology. K.M. Vincent: Formal analysis. Z. Xu: Investigation. J. Liu: Investigation. G. Zhang: Investigation. L.M. Z Nakevska: Investigation. D. Smith: Investigation. C.H. Lee: Formal analysis, supervision, writing review and editing. Postovit: Conceptualization, funding acquisition, project administration, supervision, writing-review and editing. Y. Fu: Conceptualization, funding acquisition, project administration, supervision, writing-review and editing.
Corresponding authors
Ethics declarations
Competing interests
The authors declare no competing interests.
Additional information
Publisher’s note Springer Nature remains neutral with regard to jurisdictional claims in published maps and institutional affiliations.
Rights and permissions
Springer Nature or its licensor (e.g. a society or other partner) holds exclusive rights to this article under a publishing agreement with the author(s) or other rightsholder(s); author self-archiving of the accepted manuscript version of this article is solely governed by the terms of such publishing agreement and applicable law.
About this article
Cite this article
Chen, H., Lee, L.J., Vincent, K.M. et al. Transcription factor ZIC2 regulates the tumorigenic phenotypes associated with both bulk and cancer stem cells in epithelial ovarian cancer. Oncogene 43, 1688–1700 (2024). https://doi.org/10.1038/s41388-024-03026-z
Received:
Revised:
Accepted:
Published:
Issue Date:
DOI: https://doi.org/10.1038/s41388-024-03026-z
- Springer Nature Limited