Abstract
In Ethiopia, the Ribb River is one of the tributaries of the Lake Tana sub-basin. Temperature, precipitation, and streamflow would all be affected by climate change in the Ribb watershed. As a result of the disruption of regular hydrological processes, these climate changes have an impact on water resources. The goal of this study was to look into the effects of climate change on the Ribb watershed’s hydro-climatic characteristics. The forecasted climatic data for rainfall and temperature (minimum and maximum) came from the CORDEX (Coordinated Regional Climate Downscaling Experiments) Africa database. Climate change consequences were investigated using RCP 4.5 emission scenarios for the 2021–2060 time range, compared to the 1985–2005 baselines. The observed precipitation and temperature data were used to adjust for bias. The simulation of stream flow was carried out using the semi-distributed and physically based soil and water assessment tool (SWAT). From 1997 to 2003, the model was calibrated, and from 2004 to 2007, it was validated. To determine the trend of the climate variables, trend test analyses were performed on the various time series data. In all of the experiments conducted, the trend test revealed that historical and forecast precipitation recording stations showed statistically negligible trends for all critical values. At a level of 0.05, the historical and prospective maximum and minimum temperature data revealed increasing patterns. In general, the results demonstrated that meteorological conditions cause the flow to decrease over the season. As a result, climate change will have an impact on the Ribb watersheds water resources.
Similar content being viewed by others
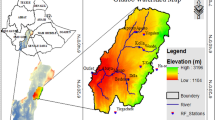
Avoid common mistakes on your manuscript.
Introduction
Climate change, which is caused by an increase in greenhouse gas emissions and other radiative trace gases in the atmosphere, has been the focus of a large number of scientific studies during the last two decades (Lineman et al. 2015; Xu et al. 2005). The widespread belief that global climate change has serious consequences for the environment, ecosystems, water supplies, and nearly every element of human life has prompted this study (Gan et al. 2016). At the global level, the influence of global climate change on water supplies is the most important study agenda (IPCC 2007; Tenagashaw and Andualem 2022). Climate change is the most pressing global concern, particularly for Africans, as it increases the frequency of extreme weather events seen around the world (Kotir 2011; Mirza 2003). By disrupting hydrological processes in basins, climate change has a profound impact on water resources (Malede et al. 2022a). Climate change has impacted the timing, magnitudes, and patterns of stream flows, according to Jiang et al. (2007), resulting in an increase in flood damage to agricultural land, property, and human life.
The Intergovernmental Panel on Climate Change (IPCC) confirms that global climate change has both beneficial and negative effects on the natural and social environment (Malede et al. 2022b). According to the findings of the IPCC, emerging countries such as Ethiopia would be more vulnerable to climate change. Ethiopia’s climate has altered significantly during the previous five decades, according to evidence. According to previous study, there has been a considerable temperature variation and trend shift throughout time. Annual minimum temperatures, averaged over 40 sites from 1951 to 2005 and shown as temperature departures from the mean, exhibited a lot of variety (NAPA 2007). Over the course of those 55 years, the country has had both warm and cold years, with recent years being the warmest in comparison with previous decades.
Increasing demand conflicts such as irrigation, domestic, and hydropower of the river basin as the appropriate unit of analysis to meet the difficulties facing water resource management necessitate proper planning and management of water resources in the basin. Climate change has an impact on hydro-climatic variables that support water demand in the watershed. The primary goal of this study was to evaluate the impact of climate change on the hydro-climatic variables of the Ribb watershed, which have a substantial impact on the multi-purpose water resource development and river basin management.
Materials and methods
Description of the study area
This research was carried out in one of Lake Tana’s contributing watersheds. The Ribb watershed is located in the eastern parts of the Lake, primarily in Farta Wereda, with a minor portion of it encompassing the Ebinat wereda of the South Gonder Zone in Amhara Region. The river Ribb drains to the eastern part of Lake Tana. The study watershed was taken upstream of the dam headwork with a drainage area of 685 km2. Geographically, the watershed is located between 37° 56′ 10″ and 38° 14′ 33″ longitude, and 11° 42′ 46″ and 12° 6′ 25″ latitude. The study area’s topography spans from 1863 to 4092 m (Fig. 1).
The upper watershed has three major dominant soils namely chromic luvisol, lithic leptosol and haplic luvisol. The slope of the watershed ranges from 0.08 to 44.44 degrees. The dominant type of land cover is cultivated followed by grassland (Fig. 1).
The climate of the Ribb watershed is marked by a rainy season from May to September, with monthly rainfall varying from 65 mm in May to 411 mm in July. Mean annual precipitation is about 1400 mm in the upper part and about 1200 mm in the lower part of the study area. Temperature variations throughout the year are minor (19 °C in December to 23 °C in May), with maximum and minimum temperatures of 28.5 °C and 8.5 °C, respectively (Ribb detail design documents 2007).
Dataset
For this study, the daily stream flow of a river was obtained from the Ethiopian Ministry of Water, Irrigation, and Electricity (MoWIE) for the dam site, and meteorological data (rainfall, temperature, relative humidity, wind speed, and sunshine hour) were collected from the Amhara National Metrological Agency for meteorological stations located inside and around the watershed (ANMA). The DEM with a resolution of 12.5 × 12.5 m was downloaded from the Alaska website and used to define the watershed’s physical and hydrological properties (slope, flow accumulation, flow direction, and stream network). The classified land use map of the study area by Andualem et al. (2018) for the year 2018 was used for this study. The soil map was collected from Ethiopian Ministry of Water, Irrigation, and Electricity (MoWIE) and used as an input for SWAT.
It is a global initiative to aid climate change effect and adaptation research by making a relatively fine-scale climate projection readily available to users. The CORDEX-Africa database was used to collect downscaled rainfall and temperature (minimum and maximum) predicted climatic data from the CMIP5 simulation for the period 1951–2100. (Kim et al. 2014; Hewitson et al. 2012). During this research, the Representative Concentration Pathway RCP 4.5 projection scenario was used. Since the RCPs are consistent with a wide range of possible changes in future, anthropogenic GHG emissions are intended to represent their atmospheric concentrations. RCP 4.5 is described by the IPCC as an intermediate scenario and emissions in RCP 4.5 peak around 2040, then decline. RCP 4.5 is better for analyzing climate change impacts on hydrology because it represents the reality in the Lake Tana sub-basin.
Data analysis
Climatic data analysis
A double mass curve was used to assess the consistency of rainfall data from individual stations. The Pettit test was used to determine the homogeneity of each station’s annual precipitation data.
Using the recorded observed data, bias correction was used to correct the downscaled precipitation and temperature. The power transformation method is the most popular and straightforward way of precipitation adjustment (Ahmed et al. 2013; Smitha, et al. 2018). The statistical variance of precipitation time series is adjusted using power transformation, a nonlinear correction approach with the exponential form Xb. The coefficient of variation (CV) of the corrected simulations Xb and the CV of the observed values are equalized to estimate the parameter b. Each daily precipitation amount P is turned into a corrected P* using Eq. 1 in this nonlinear correction.
where P* is corrected precipitation, P is uncorrected precipitation, a and b are transformer parameters.
Because temperature is known to be approximately regularly distributed, power transformation cannot be used to adjust it. When a normally distributed dataset is corrected with an influence power-law function, the result is a dataset that is not normally distributed (Li et al. 2014). Temperature correction entailed regulating the mean and variance of the temperature data via shifting and scaling. T*, the corrected daily temperature, was calculated as follows:
where Tobs is observed daily temperature, \({T}_{\mathrm{rcp}}\) is rcp daily temperature, \(\sigma\) is standard deviation and \({T}^{*}\) is corrected daily temperature.
Trend analysis
Recently assessing the status of environmental circumstances, as well as detecting changes in environmental conditions, has influenced by statistical trend assessment approaches (Esterby 1993).
Mann–Kendal test
The Mann–Kendal test was developed to discover monotonic (increasing or decreasing) patterns and to determine whether or not they are statistically significant (Birsan et al. 2005).
The trend test was applied to a time series Xi, which is ranked from \(I \, = \, 1, \, 2 \ldots n - 1\), and a time series Xj, which is ranked from \(j = i + 1, \, 2 \ldots \, n\). Each data point Xi was used as a benchmark against which the rest of the data points Xj were measured:
where Xi and Xj are the annual values for years I and j, respectively (j I The statistic 'S' is essentially normally distributed with the mean when the number of observations is more than 10 (n ≥ 10), and E(S) becomes 0. (Kendall 1975). The variance of statistics is calculated as follows:
where n is the number of observations and ti are the ties of the sample time series. The test statistics Z is computed as follows:
where Z follows standard a normal distribution, a positive Z and a negative Z depict an upward and downward trend for the period, respectively.
Simple linear regression
A statistical approach with one explanatory variable is simple linear regression toward the mean. It is concerned with two-dimensional sample points with one experimental variable and one variable, and it seeks out the most accurate linear function (a non-vertical straight line) to predict variable values as a function of the experimental variable. One predictor is given the result variable (Zou et al. 2003).
A line with a slope and a y-intercept is described in this way. In general, such a relationship may not hold for the mainly unknown population of independent and dependent variable values. The underlying relationship (linear regression model) between yi and xi containing this error factor i can be expressed as follows:
Student t test
Under the null hypothesis, the t test is a statistical hypothesis test in which the test statistic follows a Student’s t-distribution. When the test statistic has a conventional distribution and the value of a scaling term within the test statistic is questioned, a t test is used. The test statistics follow a Student’s t-distribution when the scaling term is unknown and is substituted by an estimate based on the data. The t test is commonly used to examine whether the means of two sets of knowledge are significantly different. Z could be affected by the choice theory. The magnitude tends to be larger when the choice hypothesis is true, whereas S is a scaling parameter that allows the distribution of t to be (Zimmerman 1987).
where Xav is the sample mean from a sample \(X1,X2, \ldots ,Xn\), of size n, s is the standard error of the mean, σ is the estimate of the standard deviation of the population, and μ is the population mean.
Stream flow forecasting
Forecasting stream flow at various time intervals (hourly, daily, monthly, or yearly) is critical for supplying data (sediment and flow into reservoirs) and ensuring the proper operation of a water resources system (Musa 2013). Forecasting stream flow is critical in the case of multifunctional reservoirs, which are critical to the operation of flood control reservoir systems. Various models are used around the world to forecast stream flow (Liang et al. 2018). The Arc SWAT model was used to simulate river flow under current and future climate change scenarios in this study.
Watershed delineation, HRU definition, meteorological write-up, and stream flow simulation are all required steps in the SWAT model. Arc SWAT was used to demarcate the sub-basin and watershed. To establish the hydrologic parameters for each land use and soil type simulated in each sub-watershed, the SWAT model requires land use/cover and soil data. SWAT can be used to model a single watershed or a network of hydrologically connected watersheds (Di Luzio et al. 2002). The SUFI-2 optimization approach was utilized to calibrate and validate the simulated streamflow output using the SWAT-CUP (calibration and uncertainty program). In each iteration, different parameters were modified until the best correlation between simulated and observed flow was found (Arnold et al. 2012). The model was calibrated between 1997 and 2003 and then validated between 2004 and 2007. The year 1997 was labeled as a “warm” year. The Upper Ribb watershed’s average observed streamflow data were used to calibrate the system on monthly time increments. The Percept BIAS (PBIAS), Nash and Sutcliffe coefficient of efficiency (NSE), and coefficient determination (R2) assessment criteria were used to assess the SWAT model’s effectiveness.
Results and discussion
Bias correction for baseline period
The bias-corrected downscaled RCM output from the fifth phase of the Coupled Model Intercomparison Project (CMIP5), which was downscaled over the Africa-CORDEX domain by a regional climate model, was used to investigate the effects of climate change and variability on stream flow (CCLM). The SWAT model was used to simulate the stream flow using observed meteorological data and RCM results. The bias in the RCMs dataset was corrected using power transformation, shifting, and scaling processes. Observed data from 1985 to 2005 were utilized to rectify the bias in the different grids’ historical RCM data. The twelve-month power transformation constants were used to correct the long-run RCP 4.5 data for 2021–2040 and 2041–2060.
Precipitation
In comparison with linear scaling, gamma distribution, and local intensity scaling, Fang et al. (2015) found that power transformation and quintile mapping are the best strategies for bias correction of precipitation. Similarly, Teutschbein and Seibert (2012) found that, when compared to linear scaling, delta change, and local intensity scaling, power transformation and distribution mapping are the best strategies for bias correction of precipitation. As a result, for this study, the power method for bias correction of precipitation was adopted.
The precipitation data bias was corrected using a power transformation, which corrects the coefficient of variation (CV) as well as the mean. Iterating until the CV and mean of the corrected daily precipitation match the CV of the observed daily precipitation yielded the values of the power transformation constants for each month. RCP and observed rainfall data showed good agreement in the power transformation correction. Before the bias is addressed, the RCP data are overestimated in comparison with the observed data (Fig. 2).
Temperature
The RCP minimum and maximum temperature values were corrected using the shifting and scaling procedures. For the baseline period, the projected maximum and minimum temperatures exhibited good agreement between observed and bias-corrected values (Fig. 3).
Trend analysis of climate data
The Ribb watershed has just two climatic stations that are used to study future climate change in the watershed: Grid Gp11221 (near Amed Ber metrological station) and Grid Gp113221 (near Ibnat metrological station). The Amedber observed station was used to correct climate station Gp112221, while the Ibnat station was used to correct climate station Gp113221. The Mann–Kendall, Linear regression, and Student’s t test trend analysis methods were used to analyze the trend test of climate data for each climatic station.
In Mann–Kendall and linear regression tests, the historical precipitation record at Gp112221 station revealed no statistically significant trend at a = 0.1. The student's t-test, on the other hand, revealed a significant trend at a = 0.1. Using Mann–Kendall and linear regression, the future forecasted precipitation data trend test of Gp112221 station revealed a statistically significant and decreasing trend at a = 0.1 (Table 1). On the other hand, GP113221 showed an non-significant trend (Table 2).
Gp112221’s historical and projected maximum and lowest temperature data were subjected to a trend test (Table 3). At a = 0.05, the data exhibited an increasingly substantial trend, according to the trend test results. Similarly, the forecasted temperature data revealed an upward trend. This demonstrated that global warming resulted in an increase in temperature, which had a substantial impact on precipitation and other components of the hydrologic cycle.
At a = 0.01, both the future minimum and maximum temperature data indicated a statistically significant and upward trend. There was no statistically significant trend in the record minimum and maximum temperatures at the Gp113221 station (Table 4).
In all types of testing, the historical and forecasted precipitation record station exhibited no statistically significant trend at all key values. At a = 0.05, the maximum and minimum temperature data from the past and future revealed a growing trend. This suggested that global warming resulted in an increase in temperature, which had a substantial impact on precipitation and other components of the Ribb watershed’s hydrologic cycle. Ayalew et al. (2021) and Malede et al. (2022a, b) finding demonstrates that although the temperature is rising, the amount of precipitation is decreasing. According to Addisu et al. (2015) and Tenagashaw and Andualem (2022), the mean, maximum, and minimum temperature in Lake Tana Sub-basin showed a general increasing trend, whereas rainfall amount showed a general declining trend.
Stream flow modeling
Sensitivity analysis
The sensitive and significant stream flow parameters (Table 5) were identified using the SWAT-CUP (Calibration and uncertainty program) model.
CN2, SOL K, CH K2 SLP, and SOL BD were determined to be the 1st to 5th most sensitive flow metrics in the Upper Ribb watershed, respectively (Table 5). ESCO, SOIL-AWC, EPCO, CN2, and ALPHA BF, on the other hand, were identified as the first five sensitive flow parameters by Andualem et al. (2020a). T-STAT and p values were used to determine the parameter’s level of sensitivity and significance. Parameters having a high absolute T-STAT value are regarded as sensitive, whereas those with a P value near 0 are regarded as very significant.
Stream flow calibration and validation
The calibration of simulated flow was done using Addis Zemen gauging station observed flow data and the identified sensitive flow parameters. The calibration period covered the years 1998–2003, with the first year of simulation (1997) serving as a “warm-up” period. Calibration was used to determine the fitting values of stream flow parameters (Table 6).
The model performed well in the flow calibration, with R2 and NSE values of 0.73. The flow parameters were validated between 2004 and 2007, and the findings revealed that the model performed well, with NSE, R2, and PBIAS values of 0.5, 0.72, and 47, respectively (Figs. 4 and 5). Because of poor data quality and a small number of years of data utilized for validation, the validation value of NSE was determined to be lower than the calibration value.
Similar research in the Ribb watershed yielded positive results. R2 and NSE values of 0.9 and 0.84 were found by Andualem et al. (2020a, b). As a result, the findings can be applied to water resource management and other applications.
Trend Analysis of streamflow data
The trend analysis of historic and predicted stream flow data from the future climate and simulation results were determined using different types of tests.
Using a trend test, the historic and expected future flow trends were analyzed, and it was found to be satisfactory at 95 and 90% level accuracy. However, with 99 percent accuracy, it revealed a big change. As a result, the forecasted stream flow data might be used to improve water resource management and development (Table 7 and 8).
Climate change impact on stream flow
The current global warming has had a considerable impact on weather factors. Changes in weather variables cause the water circulation system to vary.
The forecasted stream flow results revealed a decreasing trend in stream flow in the Upper Ribb watershed, indicating that sustainable water resource management is required (Figs. 6 and 7). The decline in stream flow in the Upper Ribb watershed could result in a major fall in the Ribb reservoir’s water volume. The decrease in water storage in Ribb reservoir will reduce the amount of land that can be irrigated, lowering agricultural productivity. According to Ayalew et al. (2021), based on the RCP4.5 and RCP8.5 scenarios, the mean annual stream flow might potentially decline from 42.7 to 40.24 m3/s and from 42.78 to 37.58 m3/s, respectively.
Conclusion
Climate change had a substantial impact on the hydro-climatic variables of a watershed. The evaluation of the changes in hydro-climatic variables is critical for sustainable water resources and environmental management. Downscaling climate data from CORDEX-Africa using RCP 4.5 emission scenarios revealed the potential implications of global climate change. Different bias correction approaches were used to adjust the downscaled climatic data (power transformation for precipitation data, and shifting and scaling method for temperature data). For the studied period, the precipitation data indicated a negligible trend. Temperature trends, on the other hand, revealed a significant upward tendency. Variability in rainfall and temperature patterns was seen in global climate change scenarios over the catchment. Using a semi-distributed physically based SWAT model, this study attempted to simulate the effects of temperature change on stream flow and other parameters. The observed stream flow data were used to calibrate and validate the simulated stream flow results. Percent bias (PBIAS), Nash–Sutcliffe simulation efficiency (NSE), and coefficient of determination were used to evaluate the model’s performance (R2). With a Percent bias (PBIAS) value of 5.4, Nash–Sutcliffe simulation efficiency (NSE) value of 0.73, and coefficient of determination (R2) value of 0.73, the calibration method revealed a satisfactory agreement between observed and simulated stream flow. The results of the study showed that in the long-run, stream flow declines during as a result of changes in climate variables such as temperature and evapotranspiration. As a result, future water resource development and management projects should address the effects of climate change in order to ensure the resource’s long-term use. The multi-purpose Ribb reservoir operation must also be adjusted depending on changes in hydro-climatic variables caused by climate change and their impact on reservoir volume.
Data availability
The data will be available upon request.
References
Addisu S, Selassie YG, Fissha G, Gedif B (2015) Time series trend analysis of temperature and rainfall in lake Tana Sub-basin, Ethiopia. Environ Syst Res 4(1):1–12
Ahmed KF, Wang G, Silander J, Wilson AM, Allen JM, Horton R, Anyah R (2013) Statistical downscaling and bias correction of climate model outputs for climate change impact assessment in the US northeast. Global Planet Change 100:320–332
Andualem TG, Belay G, Guadie A (2018) Land use change detection using remote sensing technology. J Earth Sci Clim Chang 9:1–6
Andualem TG, Guadie A, Belay G, Ahmad I, Dar MA (2020a) Hydrological modeling of Upper Ribb watershed, Abbay Basin, Ethiopia. Glob Nest J 22(2):158–164
Andualem TG, Malede DA, Ejigu MT (2020b) Performance evaluation of integrated multi-satellite retrieval for global precipitation measurement products over Gilgel Abay watershed, Upper Blue Nile Basin, Ethiopia. Model Earth Syst Environ 6(3):1853–1861
Arnold JG, Moriasi DN, Gassman PW, Abbaspour KC, White MJ, Srinivasan R, Santhi C, Harmel RD, van Griensven A, Van Liew MW, Kannan N, Jha MK (2012) SWAT: model use, calibration, and validation. Trans ASABE 55(4):1491–1508
Ayalew DW, Asefa T, Moges MA, Leyew SM (2021) Evaluating the potential impact of climate change on the hydrology of Ribb catchment, Lake Tana Basin, Ethiopia. J Water Clim Change 13(1):190–205
Birsan MV, Molnar P, Burlando P, Pfaundler M (2005) Streamflow trends in Switzerland. J Hydrol 314(1–4):312–329
Di Luzio M, Srinivasan R, Arnold JG (2002) Integration of watershed tools and swat model into Basins 1. JAWRA J Am Water Resour Assoc 38(4):1127–1141
Esterby SR (1993) Trend analysis methods for environmental data. Environmetrics 4(4):459–481
Fang GH, Yang J, Chen YN, Zammit C (2015) Comparing bias correction methods in downscaling meteorological variables for a hydrologic impact study in an arid area in China. Hydrol Earth Syst Sci 19(6):2547–2559. https://doi.org/10.5194/hess-19-2547-2015
Gan TY, Ito M, Hülsmann S, Qin X, Lu XX, Liong SY, Rutschman P, Disse M, Koivusalo H (2016) Possible climate change/variability and human impacts, the vulnerability of drought-prone regions, water resources, and capacity building for Africa. Hydrol Sci J 61(7):1209–1226
Gebre SL, Ludwig F (2015) Hydrological response to climate change of the upper Blue Nile River Basin: based on IPCC fifth assessment report (AR5). J Climatol Weather Forecast 3(01):1–15
Herrendörfer G, Rasch D, Feige KD (1983) Robustness of statistical methods II. Methods for the one-sample problem. Biometr J 25(4):327–343
Hewitson B, Lennard C, Nikulin G, Jones C (2012) CORDEX-Africa: a unique opportunity for science and capacity building. CLIVAR Exch 17(3):6–7
Houghton JT, Ding YDJG, Griggs DJ, Noguer M, van der Linden PJ, Dai X et al (2001) Climate change 2001: the scientific basis. The Press Syndicate of the University of Cambridge, Cambridge
IPCC (2007) Intergovernmental panel on climate change: climate change, synthesis report. Cambridge Press, Cambridge
Jiang T, Chen YD, Xu CY, Chen X, Chen X, Singh VP (2007) Comparison of hydrological impacts of climate change simulated by six hydrological models in the Dongjiang Basin, South China. J Hydrol 336(3–4):316–333
Kendall K (1975) Thin-film peeling-the elastic term. J Phys D Appl Phys 8(13):1449
Kim J, Waliser DE, Mattmann CA, Goodale CE, Hart AF, Zimdars PA, Crichton DJ, Jones C, Nikulin G, Hewitson B, Jack C, Favre A (2014) Evaluation of the CORDEX-Africa multi-RCM hindcast: systematic model errors. Clim Dyn 42(5):1189–1202
Kotir JH (2011) Climate change and variability in Sub-Saharan Africa: a review of current and future trends and impacts on agriculture and food security. Environ Dev Sustain 13(3):587–605
Li C, Sinha E, Horton DE, Diffenbaugh NS, Michalak AM (2014) Joint bias correction of temperature and precipitation in climate model simulations. J Geophys Res: at 119(23):13–153
Liang Z, Tang T, Li B, Liu T, Wang J, Hu Y (2018) Long-term streamflow forecasting using SWAT through the integration of the random forests precipitation generator: a case study of Danjiangkou Reservoir. Hydrol Res 49(5):1513–1527
Lineman M, Do Y, Kim JY, Joo GJ (2015) Talking about climate change and global warming. PLoS ONE 10(9):e0138996
Malede DA, Agumassie TA, Kosgei JR, Pham QB, Andualem TG (2022a) Evaluation of satellite rainfall estimates in a rugged topographical basin over south Gojjam Basin, Ethiopia. J Indian Soc Remote Sens. https://doi.org/10.1007/s12524-022-01530-x
Malede DA, Agumassie TA, Kosgei JR, Andualem TG, Diallo I (2022b) Recent approaches to climate change impacts on hydrological extremes in the Upper Blue Nile Basin, Ethiopia. Earth Syst Environ 6:669–679
Mirza MMQ (2003) Climate change and extreme weather events: can developing countries adapt? Climate Policy 3(3):233–248
Musa JJ (2013) Stochastic modeling of Shiroro River streamflow process. Am J Eng Res (AJER) 2(6):49–54
NAPA (2007) Climate change national adaptation programme of action (NAPA) of Ethiopia. National Meteorological Services Agency, Ministry of Water Resources, Federal Democratic Republic of Ethiopia, Addis Ababa
Robinson PJ (1997) Climate change and hydropower generation. Int J Climatol: J R Meteorol Soc 17(9):983–996
Setegn SG, Rayner D, Melesse AM, Dargahi B, Srinivasan R (2011) Impact of climate change on the hydro climatology of Lake Tana Basin, Ethiopia. Water Resour Res 47:W0451
Smitha PS, Narasimhan B, Sudheer KP, Annamalai H (2018) An improved bias correction method of daily rainfall data using a sliding window technique for climate change impact assessment. J Hydrol 556:100–118
Tenagashaw DY, Andualem TG (2022) Analysis and characterization of hydrological drought under future climate change using the SWAT model in Tana Sub-basin, Ethiopia. Water Conserv Sci Eng 7:131–142
Tsegaye E (2006) Regionalization of potential evapotranspiration prediction for the Blue Nile (Abbay) the River Basin, Ethiopia. MSc thesis, Arba-Minch University, Ethiopia
Teutschbein C, Seibert J (2012) Bias correction of regional climate model simulations for hydrological climatechange impact studies: review and evaluation of different methods. J Hydrol 456:12–29
Xu CY, Widén E, Halldin S (2005) Modeling hydrological consequences of climate change—progress and challenges. Adv Atmos Sci 22(6):789–797
Zimmerman DW (1987) Comparative power of Student t-test and Mann–Whitney U test for unequal sample sizes and variances. J Exp Educ 55(3):171–174
Zou KH, Tuncali K, Silverman SG (2003) Correlation and simple linear regression. Radiology 227(3):617–628
Author information
Authors and Affiliations
Corresponding author
Ethics declarations
Conflict of interest
The authors declare that they have no competing interest. The authors declare that this manuscript is our original work.
Additional information
Publisher's Note
Springer Nature remains neutral with regard to jurisdictional claims in published maps and institutional affiliations.
Rights and permissions
Open Access This article is licensed under a Creative Commons Attribution 4.0 International License, which permits use, sharing, adaptation, distribution and reproduction in any medium or format, as long as you give appropriate credit to the original author(s) and the source, provide a link to the Creative Commons licence, and indicate if changes were made. The images or other third party material in this article are included in the article's Creative Commons licence, unless indicated otherwise in a credit line to the material. If material is not included in the article's Creative Commons licence and your intended use is not permitted by statutory regulation or exceeds the permitted use, you will need to obtain permission directly from the copyright holder. To view a copy of this licence, visit http://creativecommons.org/licenses/by/4.0/.
About this article
Cite this article
Tenagashaw, D.Y., Andualem, T.G., Ayele, W.T. et al. Climate change impact on hydro-climatic variables of Ribb watershed, Tana sub-basin, Ethiopia. Appl Water Sci 13, 35 (2023). https://doi.org/10.1007/s13201-022-01842-w
Received:
Accepted:
Published:
DOI: https://doi.org/10.1007/s13201-022-01842-w