Abstract
This study explores the determinants of Bitcoin’s price from 2010 to 2018. This study applies Generalized Autoregressive Conditional Heteroskedastic model to investigate the Bitcoin datasets. The experimental results find the Bitcoin price has positive relationship to the exchange rates (USD/Euro, USD/GBP, USD/CHF and Euro/GBP), the DAX and the Nikkei 225, while a negative relationship with the Fed funds rate, the FTSE 100, and the USD index. Especially, Bitcoin price is significantly affected by the Fed funds rate, followed by the Euro/GBP rate, the USD/GBP rate and the West Texas Intermediate price. This study also executes the decision tree and support vector machine techniques to predict the trend of Bitcoin price. The machine learning approach could be a more suitable methodology than traditional statistics for predicting the Bitcoin price.

Similar content being viewed by others
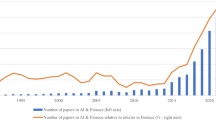
Notes
Banks could borrow money for short periods (typically overnight) to make up transitory cash shortfalls. The interest rate that is paid on these borrowed reserves is called the federal funds rate.
References
Anders, N. (2014). The troublesome history of the Bitcoin exchange mtgox. https://anders.io/the-troublesome-history-of-the-bitcoin-exchange-mtgox/
Baur, D. G., Dimpfl, T., & Kuck, K. (2018). Bitcoin, gold and the US dollar—A replication and extension. Finance Research Letters, 25, 103–110.
Bouri, E., Molnár, P., Azzi, G., Roubaud, D., & Hagfors, L. I. (2017). On the hedge and safe haven properties of Bitcoin: Is it really more than a diversifier? Finance Research Letters, 20, 192–198.
Brière, M., Oosterlinck, K., & Szafarz, A. (2015). Virtual currency tangible return: Portfolio divesification with Bitcoin. Journal of Asset Management, 16(6), 365–373.
Chiang, H. S., Sangaiah, A. K., Chen, M. Y., & Liu, J. Y. (2020). A novel artificial bee colony optimization algorithm with SVM for bio-inspired software-defined networking. International Journal of Parallel Programming, 48, 310–328.
Conti, M., Kumar, E. S., Lal, C., & Ruj, S. (2018). A survey on security and privacy issues of bitcoin. IEEE Communications Surveys & Tutorials, 20(4), 3416–3452.
Damodaran, A. (2017). The Bitcoin bom asset, currency, commodity or collectible? http://aswathdamodaran.blogspot.com/2017/10/the-bitcoin-boom-asset-currency.html.
Daştan, İ., & Gürler, C. (2016). Factors affecting the adoption of mobile payment systems: An empirical analysis. Emerging Markets Journal, 6(1), 17–24.
Dyhrberg, A. H. (2016). Bitcoin, gold and the dollar—A GARCH volatility analysis. Finance Research Letters, 16, 85–92.
Franklin, M. (2016). A profile of BitCoin currency: An exploratory study. International Journal of Business & Economics Perspectives, 11(1), 80–92.
Fujibuchi, W., & Kato, T. (2007). Classification of heterogeneous microarray data by maximum entropy kernel. BMC Bioinformatics, 8(267), 1–10.
Grinberg, R. (2012). Bitcoin : An innovative alternative digital currency. Hastings Science and Technology Law Journal, 4, 159–208.
Harish, N., Mandal, S., Rao, S., & Patil, S. G. (2015). Particle swarm optimization based support vector machine for damage level prediction of non-reshaped berm breakwater. Applied Soft Computing, 27, 313–321.
Harper, J. (2013). What is the value of Bitcoin. CATO At Liberty, https://www.cato.org/blog/what-value-bitcoin.
Hsu, C.W., Chang, C.C., & Lin, C.J. (2010). A practical guide to support vector classification, National Taiwan University. https://www.csie.ntu.edu.tw/~cjlin/papers/guide/guide.pdf
Ju, L., Lu, T., & Tu, Z. (2016). Capital flight and Bitcoin regulation. International Review of Finance, 16(3), 445–455.
Nakamoto, S. (2009). Bitcoin: a peer-to-peer electronic cash system. https://bitcoin.org/bitcoin.pdf.
Plassaras, N. A. (2013). Regulating digital currencies: Bringing Bitcoin within the reach of the IMF. Chicago Journal of International Law, 14, 377–407.
Quinlan, J. R. (1993). Programs for machine learning. San Fransisco: Morgan Kaufmann.
Sebtosheikh, M. A., & Salehi, A. (2015). Lithology prediction by support vector classifiers using inverted seismic Attributes data and petrophysical Logs as a new approach and Investigation of training data set size effect on its performance in a heterogeneous carbonate reservoir. Journal of Petroleum Science and Engineering, 134, 143–149.
Takaishi, T., & Adachi, T. (2018). Taylor effect in Bitcoin time series. Economics Letters, 172, 5–7.
Tsukerman, M. (2015). The block is hot a survey of the state of Bitcoin regulation and suggestions for the future. Berkeley Technology Law Journal, 30(385), 1127–1170.
Urquhart, A. (2016). The inefficiency of Bitcoin. Economics Letters, 148, 80–82.
Wu, C. Y., & Pandey, V. K. (2014). The value of Bitcoin in enhancing the efficiency of an investor’s portfolio. Journal of Financial Planning, 27(9), 44–52.
Zhu, Y., Dickinson, D., & Li, J. (2017). Analysis on the influence factors of Bitcoin’s price based on VEC model. Financial Innovation, 3(1), 1–13.
Author information
Authors and Affiliations
Corresponding author
Additional information
Publisher's Note
Springer Nature remains neutral with regard to jurisdictional claims in published maps and institutional affiliations.
Rights and permissions
About this article
Cite this article
Chen, TH., Chen, MY. & Du, GT. The Determinants of Bitcoin’s Price: Utilization of GARCH and Machine Learning Approaches. Comput Econ 57, 267–280 (2021). https://doi.org/10.1007/s10614-020-10057-7
Accepted:
Published:
Issue Date:
DOI: https://doi.org/10.1007/s10614-020-10057-7