Abstract
A major reason for the lack of effective therapeutics and a deep biological understanding of complex diseases, which are thought to result from a complex interaction between genetic and environmental risk factors, is the paucity of relevant experimental models. This review describes a novel experimental approach that allows the study of the functional effects of disease-associated risk in complex disease by combining genome wide association studies (GWAS) and genome–scale epigenetic data to prioritize disease-associated risk variants with efficient gene editing technologies in human pluripotent stem cells (hPSCs). As a proof of principle, we recently used such a genetically precisely controlled experimental system to identify a common Parkinson’s disease-associated risk variant in a non-coding distal enhancer element that alters the binding of transcription factors and regulates the expression of α-synuclein (SNCA), a key gene implicated in the pathogenesis of Parkinson’s disease.
You have full access to this open access chapter, Download chapter PDF
Similar content being viewed by others
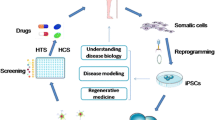
Introduction
One of the main challenges to understanding the onset and progression of human disease is to develop effective model systems that combine known genetic elements with disease-associated phenotypic readouts. The identification of genes linked to familial forms of diseases such as cystic fibrosis, sickle cell anemia or monogenetic forms of neurodegenerative disorders has fundamentally changed our understanding of many diseases and provided vital clues into the underlying pathogenesis (Botstein and Risch 2003; Altshuler et al. 2008; McClellan and King 2010). Detailed knowledge of disease-causing mutations and genes allows the establishment of reliable and disease-relevant cellular and animal models and facilitates the systematic analysis of molecular and cellular disease mechanisms and the development and validation of novel and effective therapeutic approaches.
In contrast to such predominantly rare and monogenic disorders, the majority of the most common medical conditions, such as obesity, heart disease, diabetes, autoimmune disease or sporadic neurodegenerative disease, have no well-defined genetic etiology and do not follow Mendelian inheritance patterns. Population genetics suggest that such sporadic or polygenic diseases result from a complex interaction between multiple genetic and non-genetic, lifestyle and environmental risk factors (Botstein and Risch 2003; Altshuler et al. 2008). The complexity and our limited knowledge of the underlying genetic component have largely prevented the generation of genetically defined disease models. The paucity of disease-relevant experimental systems represents one of the major reasons for our limited biological understanding of complex diseases and an almost complete lack of disease-modifying effective therapeutics.
In the following, we will summarize recent progress in genetics and developmental and molecular biology, which may provide a solution for generating disease-relevant in vitro models for complex disease. By combining human pluripotent stem cell (hPSC)-technology with genome editing and genome-scale epigenetic and genome-wide association studies (GWAS) data to identify disease-associated risk variants, we will provide a blueprint to create genetically defined experimental model systems that allow the functional analysis of disease-associated risk variants. As a proof of principle, we describe how we applied this approach to sporadic Parkinson’s disease and identified a common risk variant in a non-coding distal enhancer element that regulates the expression of SNCA, a key gene implicated in the pathogenesis of Parkinson’s disease (Soldner et al. 2016).
Induced Pluripotent Stem Cells to Model Complex Diseases
The ability to reprogram somatic cells into human induced pluripotent stem cells (hiPSCs) has opened the intriguing possibility of studying complex human disease in a cell culture dish (Takahashi and Yamanaka 2006; Takahashi et al. 2007; Yu et al. 2007). Following in vitro differentiation, patient-derived hiPSCs provide access to large amounts of human disease-relevant cells that carry all the genetic alterations involved in disease development (Saha and Jaenisch 2009; Soldner and Jaenisch 2012; Takahashi and Yamanaka 2013; Yu et al. 2013). Without precise knowledge of the underlying genetics, such patient-derived cells, therefore, allow the generation of relevant cellular model systems based on disease-associated genetic elements. This approach has already been used to model a range of primarily monogenetic diseases, including neurodegenerative diseases such as Alzheimer’s disease, Parkinson’s disease and amyotrophic lateral sclerosis (ALS; Cooper et al. 2012; Israel et al. 2012; Reinhardt et al. 2013; Alami et al. 2014; Wainger et al. 2014; Young et al. 2015). Despite the unprecedented potential and excitement of this approach, it became apparent that individual hiPSC lines, independent of disease status or genotype, displayed highly variable biological properties in vitro, such as the propensity to differentiate into functional cell types (Bock et al. 2011; Boulting et al. 2011; Soldner and Jaenisch 2012; Nishizawa et al. 2016). This observation significantly limits their value to identify robust disease-associated phenotypes by simply comparing patient-derived cells with unrelated controls. This system-immanent variability has proven to be particular challenging in the context of age-related diseases including neurodegenerative diseases such as Alzheimer’s and Parkinson’s disease, considering that disease-associated phenotypes typically progress slowly over many years in patients, which suggests that expected in vitro phenotypes would be rather mild and subtle. The reasons for the observed cell-to-cell differences include genetic background variations, genetic and epigenetic changes resulting from reprogramming and extended maintenance of hiPSCs and the lack of robust in vitro differentiation protocols (Soldner and Jaenisch 2012; Liang and Zhang 2013).
Some of the above-described limitations have been overcome by improved reprogramming and culture conditions (Warren et al. 2010; Hou et al. 2013), directed differentiation approaches including transcription factor-induced reprogramming (Zhang et al. 2013), insertion of cell type-specific fluorescent marker proteins to monitor differentiation (Di Giorgio et al. 2008; Hockemeyer et al. 2009, 2011; Chambers et al. 2012; Mica et al. 2013) or by consortium-size experiments to significantly increase the number of independent experimental samples (The HD iPSC Consortium 2012). However, variable genetic backgrounds between patient-derived and control cells remain an unresolved major limitation of the current hiPSC approach, due to the well-established influence of uncharacterized genetic modifiers on disease development and progression in patients and, accordingly, on disease-associated phenotypes in vitro.
Gene Editing to Generate Genetically Controlled Disease Models
The recent progress in gene editing technologies by using engineered nucleases such as meganucleases, zinc finger nucleases (ZFNs), transcription activator-like effector-based nucleases (TALEN) and the CRISPR/Cas9 system is thought to provide an elegant solution to control for differences in genetic background (Soldner et al. 2011; Soldner and Jaenisch 2012; Hockemeyer and Jaenisch 2016). In particular, the simplicity and ease of the CRISPR/Cas9 system to efficiently modify the genome in human cells, even at multiple loci simultaneously, allow us to engineer genetically controlled hPSC lines that differ only at known genetic disease-causing variants (Jinek et al. 2012, 2013; Cong et al. 2013; Mali et al. 2013).
As a proof of principle, we recently used ZFNs to either seamlessly correct Parkinson’s disease-associated mutations in the SNCA gene in patient-derived hiPSCs or to insert similar variants into wild-type human embryonic stem cells (hESCs; Soldner et al. 2011). Such isogenic pairs of hPSC lines provided an experimental system with a controlled genetic background in which the engineered disease-associated risk variants were the only experimental variables. Analyzing disease-associated phenotypes in this genetically controlled system allowed identification of nitrosative stress, accumulation of endoplasmic reticulum (ER)-associated degradation substrates, and ER stress as early Parkinson’s disease-associated pathological phenotypes (Chung et al. 2013). A further study revealed that nitrosative and oxidative stress result in S-nitrosilation of the transcription factor MEF2C and inhibition of the MEF2C-PGC1α transcriptional network contributing to mitochondrial dysfunction and apoptotic neuronal cell death (Ryan et al. 2013). By combining this monogenic disease model with disease-associated environmental stressors, the experiments further provide new mechanistic insight into gene-environmental (GxE) interaction in the pathogenesis of Parkinson’s disease (Ryan et al. 2013). Notably, both studies relying on a genetically controlled in vivo model identified novel therapeutic targets and small molecules that reversed the observed pathological phenotypes in neurons, which are currently perused as novel therapeutics for Parkinson’s disease (Chung et al. 2013; Ryan et al. 2013). The above-described approach clearly overcomes many of the limitations of the current hiPSC technology. Due to the simplicity of the CRISPR/Cas9 system to efficiently edit the genome in hiPSCs, the use of isogenic cell lines is becoming the gold standard for analyzing disease-associated phenotypes in vitro (Reinhardt et al. 2013; Kiskinis et al. 2014; Paquet et al. 2016). However, such an approach seems currently limited to monogenetic diseases in which the disease-causing genetic alterations are well established and the expected disease-associated phenotypes display robust and highly penetrant effects.
Functional Role of GWAS-Identified Risk Variants in Complex Disease
Translating the concept of engineering genetically controlled model systems to complex disease seems daunting and will require a detailed understanding of the underlying genetic component. GWAS and genome-scale next generation sequencing (NGS) approaches have significantly advanced our understanding of the genetic basis of complex disease. GWAS in particular have identified numerous common single-nucleotide polymorphisms (SNPs) associated with human traits and diseases, pinpointing the genomic loci and genes thought to play important roles in the pathophysiology of the respective diseases (Botstein and Risch 2003; Altshuler et al. 2008; McClellan and King 2010).
However, the interpretation of this permanently increasing amount of data is limited by the fact that disease-associated SNPs only statistically correlate with the underlying disease and the vast majority of risk variants have no established biological relevance to disease or clinical utility for prognosis or treatment (Altshuler et al. 2008; McClellan and King 2010). Any SNP in linkage disequilibrium (LD) with a GWAS-identified risk variant is equally likely to be causative for the risk to develop a specific disease. It has therefore been difficult to distinguish variants that are functional and disease-relevant from those that are in LD and thus only mark the underlying haplotype containing the functional variant. Advancing from genetic association to causal biologic processes has been challenging for two additional reasons. First, the majority of disease-associated genetic variants fall into the non-coding part of the genome, which impedes any functional analysis through simple transgenic overexpression or disruption in established cell lines or any analysis in non-human model systems due to the limited conservation of non-coding elements between species. Second, the prevailing hypothesis about the heritability of complex diseases suggests that multiple common or potentially rare SNPs cooperatively contribute to the risk of developing a specific disease; however, each individual risk variant will have only a small or at most medium-size additive or multiplicative effect on disease phenotypes (Gibson 2012). Indeed, disease-associated genetic variants are also prevalent in the healthy population, although with lower frequency, and the majority of carriers of risk SNPs do not develop a disease, implying that individual risk variants are not sufficient to cause disease-associated phenotypes. Consequently, only very few risk variants have been functionally linked to specific diseases, such as a common polymorphism at the 1q13 locus, which alters the expression of the SORT1 gene and is correlated with both plasma low-density lipoprotein cholesterol (LDL-C) and myocardial infarction (Musunuru et al. 2010).
Under the assumption that specific risk haplotypes contribute through dysregulation of the same molecular pathways to disease risk, a current approach suggests that we stratify patient-derived hiPSCs according to specific genetic risk variants rather than according to disease status. This approach may be sufficient in some cases to reduce the genetic heterogeneity based on known disease haplotypes and to reveal previously masked disease-associated phenotypes. Indeed, this approach was successfully used to dissect the function of a common Alzheimer’s disease-associated non-coding genetic variant in the 5′ region of the SORL1 (sortilin related receptor 1; Young et al. 2015). However, the main limitation of this approach remains the uncontrolled effect of additional genetic modifiers and the inability to identify the specific causative sequence variant that is required for further functional analysis.
Epigenomic Signatures to Prioritize GWAS-Identified Risk Variants
Cis-acting effects of genetic variants on gene expression have been proposed to be a major factor for phenotypic variation of complex traits and disease susceptibility (Schadt et al. 2003; Morley et al. 2004; Cheung et al. 2005, 2010; Lee and Young 2013; GTEx Consortium 2015). The widespread availability of cell- and tissue-specific transcriptome-wide expression data along with the corresponding genotyping data has greatly facilitated the identification of expression quantitative trait loci (eQTLs; GTEx Consortium 2015). Although able to detect statistical correlation between specific risk variants and gene expression, this approach entails limitations that are comparable to traditional GWAS in identifying the functional risk variants. Recent genome-scale epigenetic studies such as the ENCODE (ENCODE Project Consortium 2012) and Roadmap Epigenomics project (Roadmap Epigenomics Consortium 2015) have allowed us to reliably identify and catalogue regulatory elements in a cell type-, tissue- and in some cases disease-specific manner. These studies specifically have highlighted the enrichment of GWAS-identified risk variants in regulatory DNA elements specific to tissues and cell types (Ernst et al. 2011; Degner et al. 2012; Maurano et al. 2012; Hnisz et al. 2013; Trynka et al. 2013; Farh et al. 2014; Pasquali et al. 2014; Ripke et al. 2014) affected by the respective diseases. These results suggest that disease-associated risk variants may affect gene regulation by modifying the function of tissue-specific regulatory elements. In particular, distal enhancer elements that are bound by key transcription factors (TFs) and known to precisely control spatial and temporal gene expression during embryonic development and tissue homeostasis in a cell type-specific manner (Ward and Kellis 2012; Lee and Young 2013; Farh et al. 2014; Ripke et al. 2014; Wamstad et al. 2014) are found to be enriched for GWAS variants in many complex diseases.
A number of recent studies have correlated changes in TF binding in enhancer regions with sequence-specific, heritable changes in chromatin state and gene regulation (Kasowski et al. 2013; Kilpinen et al. 2013; McVicker et al. 2013), thus providing a molecular mechanism for how individual sequence variants contribute to the development of complex diseases. Recent progress in defining TF binding specificities using high throughput SELEX and chromatin immunoprecipitation sequencing (ChIP-seq) approaches has largely increased our understanding of sequence-specific TF binding in the genome and significantly improved our ability to analyze or predict TF binding on a genome-wide scale (Jolma et al. 2013, 2015). Based on the rapidly increasing availability of epigenetic data, mapping of GWAS-identified variants to TF binding sites within tissue-specific enhancer elements has been proposed as a valuable approach to prioritize and identify functional and disease-relevant risk variants (Ward and Kellis 2012; Rivera and Ren 2013; Claussnitzer et al. 2014; Wamstad et al. 2014). Indeed, such integration of GWAS with epigenetic signatures for heart-specific enhancers allowed for the identification of novel functional risk variants for cardiac phenotypes (Wang et al. 2016). Likewise, a similar approach identified an obesity-associated risk variant in the FTO locus, which alters early adipose differentiation by disrupting a TF binding site at a pre-adipocyte-specific enhancer (Claussnitzer et al. 2015).
The 3-dimensional (3D) organization of the genome is thought to contribute to the regulation of gene expression (Bickmore 2013; de Graaf and van Steensel 2013; de Laat and Duboule 2013). The recent development of chromosome conformation capture techniques (“3C” and genome-wide 3C-based methods; Dekker et al. 2002, 2013) or cohesin chromatin interaction analysis by paired-end tag sequencing (ChIA-PET; Dowen et al. 2014) allow us to determine long-range chromatin interactions such as cell type-specific promoter-enhancer interaction. These analyses suggest that active enhancer elements are bound by transcription factors and loop over long distances to contact target genes to regulate transcription. An emerging model suggests promoter-enhancer interactions typically only occur within megabase-sized topological-associated domains (TAD; Dixon et al. 2012; Nora et al. 2012), as defined by high DNA interaction frequency based on genome-wide chromosome capture data or within such TADs in insulated neighborhoods restricted by cohesin-associated CTCF-CTCF loops (Handoko et al. 2011; DeMare et al. 2013; Dowen et al. 2014; Rao et al. 2014; Ji et al. 2016). Notably, there is mounting evidence that changes in 3D structure, potentially through sequence-specific disruption of CTCF interaction, might contribute to disease development (Ji et al. 2016). Integrating datasets of cell type-specific changes in enhancer-promoter interactions and information about the 3D structure of the genome will further help us to assign disease-associated risk variants in enhancer sequences to target genes and provide supporting evidence to identify functional disease-associated risk variants and deregulated target genes.
Functional Analysis of Parkinson’s Disease-Associated Risk Variants
As a proof of principle, we describe below how we recently applied the above-elucidated approach to sporadic Parkinson’s disease as a prototypical complex disorder, to identify common risk variants in non-coding distal enhancer elements that functionally modulate the risk to develop the disease (Soldner et al. 2016). Parkinson’s disease is the second most common chronic progressive neurodegenerative disease, with a prevalence of more than 1% in the population over the age of 60. Although the discovery of genes linked to rare Mendelian forms of PD such as SNCA, LRRK2, PARKIN, PINK1 and DJ1 has provided insight into the molecular and cellular pathogenesis of the disease (Gasser et al. 2011; Singleton et al. 2013), the etiology leading to neuronal cell loss is largely unknown. Importantly, over 90% of Parkinson’s cases do not show Mendelian inheritance patterns; however, substantial clustering of cases within families suggests that sporadic, late age of onset Parkinson’s disease results from a complex interaction between genetic risk alleles and environmental factors. A recent GWAS meta-analysis has identified 26 genomic loci containing risk variants for sporadic Parkinson’s disease (Nalls et al. 2014); however, as for the majority of neurodegenerative disorders, little mechanistic insight is available on how specific sequence variations contribute to disease development and progression.
Identification of Parkinson’s Disease-Associated Risk Variants in Brain-Specific Enhancer Elements
A recent analysis of Histone H3 acetylated at lysine 27 (H3K27ac)-marked regions in the post-mortem adult brain suggests a significant enrichment of Parkinson’s disease-associated risk SNPs within distal enhancer elements (Vermunt et al. 2014). This finding supports the hypothesis that sequence-specific changes in enhancer function and deregulated transcription of linked genes mediate the risk to develop the disease. A number of specific epigenetic modifications, such as p300, mono-methylation of Histone H3 at lysine 4 (H3K4me1), H3K27ac and DNase I hypersensitive sites (DHSs) have been established as surrogate marks to reliably identify candidate enhancer sequences (Visel et al. 2009, 2013; Creyghton et al. 2010; Rada-Iglesias et al. 2011; Maurano et al. 2012). Thus, to identify specific candidate risk variants in distal enhancers, we intersected Parkinson’s disease-associated risk SNPs (Nalls et al. 2014) with publicly available epigenetic data (Roadmap Epigenomics Consortium 2015). This analysis allowed us to compile a list of risk variants ranked by the overlap of active enhancer elements. Interestingly, many of the top-ranked risk variants were located to the SNCA locus. Because changes in TF binding are thought to be the major mediator of SNP-specific changes in gene expression (Kasowski et al. 2013; Kilpinen et al. 2013; McVicker et al. 2013) we incorporated this idea to further prioritize the risk variants in enhancers by analyzing predicted TF binding for known TF binding specificities comparing both alternative genotypes for each Parkinson’s disease-associated SNP. This analysis highlighted the Parkinson’s disease-associated SNP rs356168 in an enhancer in intron-4 of SNCA as the risk variant with the highest number of genotype-dependent differential TF binding in the SNCA locus. The functional relevance of this enhancer was further supported by chromosome conformation capture data, which indicate a physical interaction (looping) between the enhancer and the promoter region of SNCA that is thought to be necessary for the cis-acting effects on gene expression (Vermunt et al. 2014).
It is well established that SNCA plays a central role in the pathogenesis of Parkinson’s disease. Point mutations in SNCA were the first genetic variants linked to familial forms of Parkinson’s disease, and the SNCA protein is the major component of Lewy bodies and Lewy neuritis, which are considered the pathological hallmark of familial and sporadic Parkinson’s disease (Gasser et al. 2011; Singleton et al. 2013). In addition, the SNCA locus represents one of the strongest Parkinson’s disease-associated GWAS hits (Nalls et al. 2014). Notably, multiplication of the entire SNCA locus was identified as causal for a rare autosomal-dominant form of Parkinson’s disease, indicating that a moderate increase of wild-type SNCA expression (1.5 times in the case of genomic duplications) is sufficient to cause an autosomal-dominant form of Parkinson’s disease (Singleton et al. 2003; Miller et al. 2004; Devine et al. 2011; Kim et al. 2012). This observation is highly suggestive of a molecular mechanism by which risk variants in the SNCA locus modify the risk to develop Parkinson’s disease by slightly modulating the expression of SNCA. This clear link between SNCA expression and the development of Parkinson’s disease in the context of genomic amplification therefore provides a good rationale for gene expression as a disease-relevant phenotypic readout to connect genetic variation to disease risk (Devine et al. 2011). Indeed, the first indication that the SNCA locus may contain risk alleles that modulate SNCA expression came from the identification of SNCA-Rep1, a complex polymorphic microsatellite repeat region approximately 10 kb upstream of the transcription start site. Multiple candidate gene association studies suggested that individuals who are homozygous for a shorter, “protective” repeat region (Rep1-257 or Rep1-259) have a significantly lower risk of developing Parkinson’s disease compared to individuals carrying the longer forms (Rep1-261 or Rep1-263; Kruger et al. 1999; Maraganore et al. 2006). Several functional studies, including the analysis of transgenic mice carrying different human SNCA-Rep1 alleles (Chiba-Falek et al. 2005; Cronin et al. 2009), suggested an “enhancer-like” function of the microsatellite repeat element based on the cis-regulatory correlation between the SNCA-Rep1 repeat length and SNCA expression.
Allele-Specific Gene Expression as a Robust Read-Out to Analyze Cis-Regulatory Effects
As explained in detail above, one of the major limitations of using hPSC-derived somatic cells to model disease in vitro is the considerable variability of the biological properties between individual cell lines. As for SNCA, a gene known to be variable between neuronal cell types such as astrocytes, oligodendrocytes and neurons and to be regulated during development and terminal differentiation, cellular heterogeneity and incomplete maturation significantly interfere with the detection of subtle differences in gene expression between distinct risk-genotypes or patient compared to control cells, respectively. Indeed, individual in vitro differentiation experiments from genetically identical sub-clones resulted in up to fourfold differences in SNCA expression (Soldner et al. 2016). To address this problem, we recently described an experimental approach that is based on determining the effect of individual regulatory elements on the transcription of the cis-regulated gene by analyzing allele-specific gene expression (Soldner et al. 2016). The deletion of just a single copy (heterozygous) of a candidate regulatory element or its exchange with an alternative disease-associated element affects only the gene expression of the cis-regulated gene on the same allele while maintaining the expression of the other, homologous allele, unaltered. Consequently, allele-specific gene expression would be biased towards lower or higher expression of the cis-regulated allele depending on the introduced genetic modification. Because expression is measured as the ratio between two individual alleles in every cell, this analysis is expected to be largely independent of cell homogeneity and can be applied to heterogeneous cell populations. In this respect, the non-targeted SNCA allele allows for a simple normalization and serves as internal control across isogenic samples.
Functional Analysis of Parkinson’s-Associated Risk Variants
To analyze allele-specific expression, we developed a robust, sensitive and highly quantitative reverse transcription polymerase chain reaction (qRT-PCR) assay based on the detection of a heterozygous SNP in the 3′UTR of SNCA. Using CRISPR/Cas9 genome editing, we generated an allelic series of isogeneic cell lines by either heterozygous deletion of the entire microsatellite repeat region (thought to have the most pronounced effect on SNCA expression) or insertion of SNCA-Rep1 elements with all of the repeat length alleles (Rep1-257, Rep1-259, Rep1-261 and Rep1-263) that are present in the normal population. Using allele-specific expression as readout, we showed that neither the deletion of the microsatellite repeat SNCA-Rep1 element nor its exchange for the shorter or longer repeat length risk alleles affected the cis-regulated expression of the linked SNCA allele, suggesting that this element has no clear role in SNCA regulation. This result conflicts with previous studies that supported an “enhancer-like” cis-regulatory effect of SNCA-Rep1 on the expression of SNCA. It is possible that difficulties in controlling the experimental variables of the transgenic mouse (Cronin et al. 2009) or neuroblastoma cell system (Chiba-Falek et al. 2005) used in the functional analyses, species-specific differences of non-coding regulatory elements or the variability in analyzing human post-mortem brain tissue (Fuchs et al. 2008; Dumitriu et al. 2012) affected the validity of these conclusions. However, because in vitro differentiated cells allow only for the analysis of early events, due to the limited time in culture, we cannot completely exclude an effect of the SNCA-Rep1 element at later time points or only in combination with additional environmental factors.
In contrast to SNCA-Rep1, the CRISPR/Cas9-mediated exchange of Parkinson’s disease-associated alleles spanning an enhancer element in the fourth intron that carries two risk SNPs (rs356168 and rs3756054) showed a significant effect on allele-specific expression of SNCA (Fig. 1; Soldner et al. 2016). When the protective A-allele at SNP rs356168 was exchanged for the risk-associated G-allele, the expression of the cis-regulated SNCA allele was increased by 6–18%. In contrast, the exchange of the adjacent risk SNP rs3756054 showed no effect on allele-specific SNCA expression, suggesting that this variant only reaches genome-wide significance in GWAS because this variant is in LD with the functional risk-modifying SNP (Fig. 1). Given that a 1.5-fold increase in SNCA expression is sufficient to cause a familial autosomal-dominant form of Parkinson’s disease, these data support the notion that a modest life-long increase of SNCA expression may represent the molecular cause of increased risk to develop Parkinson’s disease of individuals carrying the G-allele at this risk variant. Moreover, an expression quantitative trait loci (eQTL) analysis of SNCA expression in post-mortem adult brain samples suggested that a similar sequence-specific modest increase in SNCA expression occurs within the human population, further substantiating a functional role of the risk variant rs356168 in Parkinson’s disease (Soldner et al. 2016). This subtle effect on the expression of a disease-relevant gene is consistent with the hypothesis that small effect size of common genetic risk variants contributes to the heritability of sporadic diseases.
Proposed model describing the effect of multiple Parkinson’s disease (PD)-associated risk variants on SNCA expression (modified from Soldner et al. 2016). The schematic illustrates the genomic organization of the SNCA locus, including the PD-associated risk variants SNCA-Rep1 and the risk SNPs rs356168 and rs3756045, both located in a distal enhancer element in the fourth intron of SNCA. The analysis described in Soldner et al. (2016) suggests that the brain-specific transcription factors (TF) EMX2 and NKX6-1 show sequence-dependent binding at rs356168 with preference for the A-allele. The efficient TF binding in carriers of the protective A-allele results in a suppressed distal enhancer element and, consequently, in reduced expression of SNCA associated with reduced risk to develop PD. In contrast, the reduced TF binding in carriers of the PD risk-associated G-allele at this variant leads to a more active distal enhancer, resulting in increased expression of SNCA associated with an increased risk to develop PD. Notably, neither the repeat length of SNCA-Rep1 nor the PD-risk variant at rs3756054 significantly affects SNCA expression, suggesting that these elements are in linkage disequilibrium (LD) with other functional risk-modifying variants
To gain insight into the molecular basis of how risk variants affect target gene expression, we analyzed TF binding data and identified two brain-specific TFs, EMX2 and NKX6-1, that bind to the enhancer element at the risk variant. Further analysis for sequence-specific binding indicated that both TFs, EMX2 and NKX6-1 preferentially bind to the protective, lower SNCA expressing A-allele at rs356168 (Fig. 1). These results suggest a model in which the sequence-dependent binding of these TFs at a distal enhancer element represses enhancer activity and thus modulate SNCA expression. Indeed, ectopic overexpression of both TFs in neurons reduced SNCA expression (Soldner et al. 2016), consistent with previous data in mouse models demonstrating their role as repressors of enhancer function (Ligon 2003; Schisler et al. 2005; Schaffer et al. 2010; Mariani et al. 2012). Thus, our data provide a molecular link between GWAS-identified risk SNP-dependent changes in TF binding at a distal enhancer element, altered expression of SNCA and the risk to develop sporadic Parkinson’s disease (Fig. 1). EMX2 and NKX6-1 may physically interact and function in a complex to suppress enhancer activity. However, expression analysis indicated that the two TFs are only expressed in a subset of neurons and are primarily not co-expressed in the same cell, suggesting that they may function at the same enhancer element in different cell types. TF-specific usage of identical regulatory elements in distinct cell populations might be a possible explanation for the selective vulnerability of distinct neuronal populations, as observed in Parkinson’s disease.
Mechanistic Study of Sporadic Diseases: Conclusions
As outlined in this review, a major challenge of modeling sporadic diseases in the culture dish is the system-immanent variability in differentiating hESCs or hiPSCs to functional cells. The variability is caused by genetic background differences between patient-derived hiPSCs and cells derived from control individuals as well as the inconsistency of most protocols to generate homogeneous cultures of differentiated cells. These issues complicate, if not exclude, the use of gene expression level as a valid functional readout to define the molecular mechanisms of candidate disease risk variant, which are expected to only subtly alter the transcription of the downstream gene. As our analysis of the SNCA-associated risk variants demonstrates, two experimental strategies allow us to overcome these limitations: (1) the use of CRISPR/Cas9-mediated gene editing for generating disease-relevant and control lines that differ exclusively at the risk variant and (2) the development of an allele-specific assay that allows the robust detection of small differences in disease risk-associated gene expression, an assay that is independent of cell heterogeneity and extent of differentiation.
References
Alami NH, Smith RB, Carrasco MA, Williams LA, Winborn CS, Han SSW, Kiskinis E, Winborn B, Freibaum BD, Kanagaraj A, Clare AJ, Badders NM, Bilican B, Chaum E, Chandran S, Shaw CE, Eggan KC, Maniatis T, Taylor JP (2014) Axonal transport of TDP-43 mRNA granules is impaired by ALS-causing mutations. Neuron 81:536–543
Altshuler D, Daly MJ, Lander ES (2008) Genetic mapping in human disease. Science 322:881–888
Bickmore WA (2013) The spatial organization of the human genome. Annu Rev Genomics Hum Genet 14:67–84
Bock C, Kiskinis E, Verstappen G, Gu H, Boulting G, Smith ZD, Ziller M, Croft GF, Amoroso MW, Oakley DH, Gnirke A, Eggan K, Meissner A (2011) Reference maps of human ES and iPS cell variation enable high-throughput characterization of pluripotent cell lines. Cell 144:439–452
Botstein D, Risch N (2003) Discovering genotypes underlying human phenotypes: past successes for mendelian disease, future approaches for complex disease. Nat Genet 33(Suppl):228–237
Boulting GL, Kiskinis E, Croft GF, Amoroso MW, Oakley DH, Wainger BJ, Williams DJ, Kahler DJ, Yamaki M, Davidow L, Rodolfa CT, Dimos JT, Mikkilineni S, MacDermott AB, Woolf CJ, Henderson CE, Wichterle H, Eggan K (2011) A functionally characterized test set of human induced pluripotent stem cells. Nat Biotechnol 29:279–286
Chambers SM, Qi Y, Mica Y, Lee G, Zhang X-J, Niu L, Bilsland J, Cao L, Stevens E, Whiting P, Shi S-H, Studer L (2012) Combined small-molecule inhibition accelerates developmental timing and converts human pluripotent stem cells into nociceptors. Nat Biotechnol 30:715–720
Cheung VG, Spielman RS, Ewens KG, Weber TM, Morley M, Burdick JT (2005) Mapping determinants of human gene expression by regional and genome-wide association. Nature 437:1365–1369
Cheung VG, Nayak RR, Wang IX, Elwyn S, Cousins SM, Morley M, Spielman RS (2010) Polymorphic cis- and trans-regulation of human gene expression. PLoS Biol 8:e1000480
Chiba-Falek O, Kowalak JA, Smulson ME, Nussbaum RL (2005) Regulation of alpha-synuclein expression by poly (ADP ribose) polymerase-1 (PARP-1) binding to the NACP-Rep1 polymorphic site upstream of the SNCA gene. Am J Hum Genet 76:478–492
Chung CY, Khurana V, Auluck PK, Tardiff DF, Mazzulli JR, Soldner F, Baru V, Lou Y, Freyzon Y, Cho S, Mungenast AE, Muffat J, Mitalipova M, Pluth MD, Jui NT, Schüle B, Lippard SJ, Tsai LH, Krainc D, Buchwald SL, Jaenisch R, Lindquist S (2013) Identification and rescue of α-synuclein toxicity in Parkinson patient-derived neurons. Science 342:983–987
Claussnitzer M, Dankel SN, Klocke B, Grallert H, Glunk V, Berulava T, Lee H, Oskolkov N, Fadista J, Ehlers K, Wahl S, Hoffmann C, Qian K, Rönn T, Riess H, Müller-Nurasyid M, Bretschneider N, Schroeder T, Skurk T, Horsthemke B, DIAGRAM+Consortium, Spieler D, Klingenspor M, Seifert M, Kern MJ, Mejhert N, Dahlman I, Hansson O, Hauck SM, Blüher M, Arner P, Groop L, Illig T, Suhre K, Hsu YH, Mellgren G, Hauner H, Laumen H (2014) Leveraging cross-species transcription factor binding site patterns: from diabetes risk loci to disease mechanisms. Cell 156:343–358
Claussnitzer M, Dankel SN, Kim K-H, Quon G, Meuleman W, Haugen C, Glunk V, Sousa IS, Beaudry JL, Puviindran V, Abdennur NA, Liu J, Svensson P-A, Hsu Y-H, Drucker DJ, Mellgren G, Hui C-C, Hauner H, Kellis M (2015) FTO obesity variant circuitry and adipocyte browning in humans. N Engl J Med 373:895–907
Cong L, Ran FA, Cox D, Lin S, Barretto R, Habib N, Hsu PD, Wu X, Jiang W, Marraffini LA, Zhang F (2013) Multiplex genome engineering using CRISPR/Cas systems. Science 339:819–823
Cooper O, Seo H, Andrabi S, Guardia-Laguarta C, Graziotto J, Sundberg M, McLean JR, Carrillo-Reid L, Xie Z, Osborn T, Hargus G, Deleidi M, Lawson T, Bogetofte H, Perez-Torres E, Clark L, Moskowitz C, Mazzulli J, Chen L, Volpicelli-Daley L, Romero N, Jiang H, Uitti RJ, Huang Z, Opala G, Scarffe LA, Dawson VL, Klein C, Feng J, Ross OA, Trojanowski JQ, Lee VM, Marder K, Surmeier DJ, Wszolek ZK, Przedborski S, Krainc D, Dawson TM, Isacson O (2012) Pharmacological rescue of mitochondrial deficits in iPSC-derived neural cells from patients with familial Parkinson’s disease. Sci Transl Med 4:141ra90–141ra90
Creyghton MP, Cheng AW, Welstead GG, Kooistra T, Carey BW, Steine EJ, Hanna J, Lodato MA, Frampton GM, Sharp PA, Boyer LA, Young RA, Jaenisch R (2010) Histone H3K27ac separates active from poised enhancers and predicts developmental state. Proc Natl Acad Sci USA 107:21931–21936
Cronin KD, Ge D, Manninger P, Linnertz C, Rossoshek A, Orrison BM, Bernard DJ, El-Agnaf OMA, Schlossmacher MG, Nussbaum RL, Chiba-Falek O (2009) Expansion of the Parkinson disease-associated SNCA-Rep1 allele upregulates human -synuclein in transgenic mouse brain. Hum Mol Genet 18:3274–3285
de Graaf CA, van Steensel B (2013) Chromatin organization: form to function. Curr Opin Genet Dev 23:185–190
de Laat W, Duboule D (2013) Topology of mammalian developmental enhancers and their regulatory landscapes. Nature 502:499–506
Degner JF, Pai AA, Pique-Regi R, Veyrieras J-B, Gaffney DJ, Pickrell JK, De Leon S, Michelini K, Lewellen N, Crawford GE, Stephens M, Gilad Y, Pritchard JK (2012) DNase I sensitivity QTLs are a major determinant of human expression variation. Nature 482:390–394
Dekker J, Rippe K, Dekker M, Kleckner N (2002) Capturing chromosome conformation. Science 295:1306–1311
Dekker J, Marti-Renom MA, Mirny LA (2013) Exploring the three-dimensional organization of genomes: interpreting chromatin interaction data. Nat Rev Genet 14:390–403
DeMare LE, Leng J, Cotney J, Reilly SK, Yin J, Sarro R, Noonan JP (2013) The genomic landscape of cohesin-associated chromatin interactions. Genome Res 23:1224–1234
Devine MJ, Gwinn K, Singleton A, Hardy J (2011) Parkinson’s disease and α-synuclein expression. Mov Disord 26:2160–2168
Di Giorgio F, Boulting G, Bobrowicz S, Eggan K (2008) Human embryonic stem cell-derived motor neurons are sensitive to the toxic effect of glial cells carrying an ALS-causing mutation. Cell Stem Cell 3:637–648
Dixon JR, Selvaraj S, Yue F, Kim A, Li Y, Shen Y, Hu M, Liu JS, Ren B (2012) Topological domains in mammalian genomes identified by analysis of chromatin interactions. Nature 485:376–380
Dowen JM, Fan ZP, Hnisz D, Ren G, Abraham BJ, Zhang LN, Weintraub AS, Schuijers J, Lee TI, Zhao K, Young RA (2014) Control of cell identity genes occurs in insulated neighborhoods in mammalian chromosomes. Cell 159:374–387
Dumitriu A, Moser C, Hadzi TC, Williamson SL, Pacheco CD, Hendricks AE, Latourelle JC, Wilk JB, DeStefano AL, Myers RH (2012) Postmortem interval influences α-synuclein expression in Parkinson disease brain. Park Dis 2012:1–8
ENCODE Project Consortium (2012) An integrated encyclopedia of DNA elements in the human genome. Nature 489:57–74
Ernst J, Kheradpour P, Mikkelsen TS, Shoresh N, Ward LD, Epstein CB, Zhang X, Wang L, Issner R, Coyne M, Ku M, Durham T, Kellis M, Bernstein BE (2011) Mapping and analysis of chromatin state dynamics in nine human cell types. Nature 473:43–49
Farh KK, Marson A, Zhu J, Kleinewietfeld M, Housley WJ, Beik S, Shoresh N, Whitton H, Ryan RJ, Shishkin AA, Hatan M, Carrasco-Alfonso MJ, Mayer D, Luckey CJ, Patsopoulos NA, De Jager PL, Kuchroo VK, Epstein CB, Daly MJ, Hafler DA, Bernstein BE (2014) Genetic and epigenetic fine mapping of causal autoimmune disease variants. Nature 518:337–343
Fuchs J, Tichopad A, Golub Y, Munz M, Schweitzer K, Wolf B, Berg D, Mueller J, Gasser T (2008) Genetic variability in the SNCA gene influences alpha-synuclein levels in the blood and brain. FASEB J 22:1327–1334
Gasser T, Hardy J, Mizuno Y (2011) Milestones in PD genetics. Mov Disord 26:1042–1048
Gibson G (2012) Rare and common variants: twenty arguments. Nat Rev Genet 13:135–145
GTEx Consortium (2015) Human genomics. The Genotype-Tissue Expression (GTEx) pilot analysis: multitissue gene regulation in humans. Science 348:648–660
Handoko L, Xu H, Li G, Ngan CY, Chew E, Schnapp M, Lee CW, Ye C, Ping JL, Mulawadi F, Wong E, Sheng J, Zhang Y, Poh T, Chan CS, Kunarso G, Shahab A, Bourque G, Cacheux-Rataboul V, Sung WK, Ruan Y, Wei CL (2011) CTCF-mediated functional chromatin interactome in pluripotent cells. Nat Genet 43:630–638
Hnisz D, Abraham BJ, Lee TI, Lau A, Saint-André V, Sigova AA, Hoke HA, Young RA (2013) Super-enhancers in the control of cell identity and disease. Cell 155:934–947
Hockemeyer D, Jaenisch R (2016) Induced pluripotent stem cells meet genome editing. Cell Stem Cell 18:573–586
Hockemeyer D, Soldner F, Beard C, Gao Q, Mitalipova M, Dekelver RC, Katibah GE, Amora R, Boydston EA, Zeitler B, Meng X, Miller JC, Zhang L, Rebar EJ, Gregory PD, Urnov FD, Jaenisch R (2009) Efficient targeting of expressed and silent genes in human ESCs and iPSCs using zinc-finger nucleases. Nat Biotechnol 27:851–857
Hockemeyer D, Wang H, Kiani S, Lai CS, Gao Q, Cassady JP, Cost GJ, Zhang L, Santiago Y, Miller JC, Zeitler B, Cherone JM, Meng X, Hinkley SJ, Rebar EJ, Gregory PD, Urnov FD, Jaenisch R (2011) Genetic engineering of human pluripotent cells using TALE nucleases. Nat Biotechnol 29:731–734
Hou P, Li Y, Zhang X, Liu C, Guan J, Li H, Zhao T, Ye J, Yang W, Liu K, Ge J, Xu J, Zhang Q, Zhao Y, Deng H (2013) Pluripotent stem cells induced from mouse somatic cells by small-molecule compounds. Science 341:651–654
Israel MA, Yuan SH, Bardy C, Reyna SM, Mu Y, Herrera C, Hefferan MP, Van Gorp S, Nazor KL, Boscolo FS, Carson CT, Laurent LC, Marsala M, Gage FH, Remes AM, Koo EH, Goldstein LSB (2012) Probing sporadic and familial Alzheimer’s disease using induced pluripotent stem cells. Nature 482:216–220
Ji X, Dadon DB, Powell BE, Fan ZP, Borges-Rivera D, Shachar S, Weintraub AS, Hnisz D, Pegoraro G, Lee TI, Misteli T, Jaenisch R, Young RA (2016) 3D chromosome regulatory landscape of human pluripotent cells. Cell Stem Cell 18:262–275
Jinek M, Chylinski K, Fonfara I, Hauer M, Doudna JA, Charpentier E (2012) A programmable dual-RNA-guided DNA endonuclease in adaptive bacterial immunity. Science 337:816–821
Jinek M, East A, Cheng A, Lin S, Ma E, Doudna J (2013) RNA-programmed genome editing in human cells. Elife 2:e00471
Jolma A, Yan J, Whitington T, Toivonen J, Nitta KR, Rastas P, Morgunova E, Enge M, Taipale M, Wei G, Palin K, Vaquerizas JM, Vincentelli R, Luscombe NM, Hughes TR, Lemaire P, Ukkonen E, Kivioja T, Taipale J (2013) DNA-binding specificities of human transcription factors. Cell 152:327–339
Jolma A, Yin Y, Nitta KR, Dave K, Popov A, Taipale M, Enge M, Kivioja T, Morgunova E, Taipale J (2015) DNA-dependent formation of transcription factor pairs alters their binding specificity. Nature 527:384–388
Kasowski M, Kyriazopoulou-Panagiotopoulou S, Grubert F, Zaugg JB, Kundaje A, Liu Y, Boyle AP, Zhang QC, Zakharia F, Spacek DV, Li J, Xie D, Olarerin-George A, Steinmetz LM, Hogenesch JB, Kellis M, Batzoglou S, Snyder M (2013) Extensive variation in chromatin states across humans. Science 342:750–752
Kilpinen H, Waszak SM, Gschwind AR, Raghav SK, Witwicki RM, Orioli A, Migliavacca E, Wiederkehr M, Gutierrez-Arcelus M, Panousis NI, Yurovsky A, Lappalainen T, Romano-Palumbo L, Planchon A, Bielser D, Bryois J, Padioleau I, Udin G, Thurnheer S, Hacker D, Core LJ, Lis JT, Hernandez N, Reymond A, Deplancke B, Dermitzakis ET (2013) Coordinated effects of sequence variation on DNA binding, chromatin structure, and transcription. Science 342:744–747
Kim HJ, Jeon BS, Yoon MY, Park SS (2012) Increased expression of alpha-synuclein by SNCA duplication is associated with resistance to toxic stimuli. J Mol Neurosci 47:249–255
Kiskinis E et al (2014) Pathways disrupted in human ALS motor neurons identified through genetic correction of mutant SOD1. Cell Stem Cell 14:781–795
Kruger R, Vieira-Saecker A, Kuhn W, Berg D, Muller T, Kuhnl N, Fuchs G, Storch A, Hungs M, Woitalla D, Przuntek H, Epplen J, Schols L, Riess O (1999) Increased susceptibility to sporadic Parkinson’s disease by a certain combined alpha-synuclein/apolipoprotein E genotype. Ann Neurol 45:611–617
Lee TI, Young RA (2013) Transcriptional regulation and its misregulation in disease. Cell 152:1237–1251
Liang G, Zhang Y (2013) Genetic and epigenetic variations in iPSCs: potential causes and implications for application. Cell Stem Cell 13:149–159
Ligon KL (2003) Loss of Emx2 function leads to ectopic expression of Wnt1 in the developing telencephalon and cortical dysplasia. Development 130:2275–2287
Mali P, Yang L, Esvelt KM, Aach J, Guell M, DiCarlo JE, Norville JE, Church GM (2013) RNA-guided human genome engineering via Cas9. Science 339:823–826
Maraganore DM, de Andrade M, Elbaz A, Farrer MJ, Ioannidis JP, Krüger R, Rocca WA, Schneider NK, Lesnick TG, Lincoln SJ, Hulihan MM, Aasly JO, Ashizawa T, Chartier-Harlin MC, Checkoway H, Ferrarese C, Hadjigeorgiou G, Hattori N, Kawakami H, Lambert JC, Lynch T, Mellick GD, Papapetropoulos S, Parsian A, Quattrone A, Riess O, Tan EK, Broeckhoven V (2006) Collaborative analysis of α-synuclein gene promoter variability and Parkinson disease. JAMA 296:661–670
Mariani J, Favaro R, Lancini C, Vaccari G, Ferri AL, Bertolini J, Tonoli D, Latorre E, Caccia R, Ronchi A, Ottolenghi S, Miyagi S, Okuda A, Zappavigna V, Nicolis SK (2012) Emx2 is a dose-dependent negative regulator of Sox2 telencephalic enhancers. Nucl Acids Res 40:6461–6476
Maurano MT, Humbert R, Rynes E, Thurman RE, Haugen E, Wang H, Reynolds AP, Sandstrom R, Qu H, Brody J, Shafer A, Neri F, Lee K, Kutyavin T, Stehling-Sun S, Johnson AK, Canfield TK, Giste E, Diegel M, Bates D, Hansen RS, Neph S, Sabo PJ, Heimfeld S, Raubitschek A, Ziegler S, Cotsapas C, Sotoodehnia N, Glass I, Sunyaev SR, Kaul R, Stamatoyannopoulos JA (2012) Systematic localization of common disease-associated variation in regulatory DNA. Science 337:1190–1195
McClellan J, King M-C (2010) Genetic heterogeneity in human disease. Cell 141:210–217
McVicker G, van de Geijn B, Degner JF, Cain CE, Banovich NE, Raj A, Lewellen N, Myrthil M, Gilad Y, Pritchard JK (2013) Identification of genetic variants that affect histone modifications in human cells. Science 342:747–749
Mica Y, Lee G, Chambers SM, Tomishima MJ, Studer L (2013) Modeling neural crest induction, melanocyte specification, and disease-related pigmentation defects in hESCs and patient-specific iPSCs. Cell Rep 3:1140–1152
Miller DW, Hague SM, Clarimon J, Baptista M (2004) α-Synuclein in blood and brain from familial Parkinson disease with SNCA locus triplication. Neurology 62:1835–1838
Morley M, Molony CM, Weber TM, Devlin JL, Ewens KG, Spielman RS, Cheung VG (2004) Genetic analysis of genome-wide variation in human gene expression. Nature 430:743–747
Musunuru K, Strong A, Frank-Kamenetsky M, Lee NE, Ahfeldt T, Sachs KV, Li X, Li H, Kuperwasser N, Ruda VM, Pirruccello JP, Muchmore B, Prokunina-Olsson L, Hall JL, Schadt EE, Morales CR, Lund-Katz S, Phillips MC, Wong J, Cantley W, Racie T, Ejebe KG, Orho-Melander M, Melander O, Koteliansky V, Fitzgerald K, Krauss RM, Cowan CA, Kathiresan S, Rader DJ (2010) From noncoding variant to phenotype via SORT1 at the 1p13 cholesterol locus. Nature 466:714–719
Nalls MA, Pankratz N, Lill CM, Do CB, Hernandez DG, Saad M, AL DS, Kara E, Bras J, Sharma M, Schulte C, Keller MF, Arepalli S, Letson C, Edsall C, Stefansson H, Liu X, Pliner H, Lee JH, Cheng R, International Parkinson’s Disease Genomics Consortium (IPDGC), Parkinson’s Study Group (PSG) Parkinson’s Research: The Organized GENetics Initiative (PROGENI), 23andMe, GenePD, NeuroGenetics Research Consortium (NGRC), Hussman Institute of Human Genomics (HIHG), Ashkenazi Jewish Dataset Investigator, Cohorts for Health and Aging Research in Genetic Epidemiology (CHARGE), North American Brain Expression Consortium (NABEC), United Kingdom Brain Expression Consortium (UKBEC), Greek Parkinson’s Disease Consortium, Alzheimer Genetic Analysis Group, Ikram MA, Ioannidis JP, Hadjigeorgiou GM, Bis JC, Martinez M, Perlmutter JS, Goate A, Marder K, Fiske B, Sutherland M, Xiromerisiou G, Myers RH, Clark LN, Stefansson K, Hardy JA, Heutink P, Chen H, Wood NW, Houlden H, Payami H, Brice A, Scott WK, Gasser T, Bertram L, Eriksson N, Foroud T, Singleton AB (2014) Large-scale meta-analysis of genome-wide association data identifies six new risk loci for Parkinson’s disease. Nat Genet 46:989–993
Nishizawa M, Chonabayashi K, Nomura M, Tanaka A, Nakamura M, Inagaki A, Nishikawa M, Takei I, Oishi A, Tanabe K, Ohnuki M, Yokota H, Koyanagi-Aoi M, Okita K, Watanabe A, Takaori-Kondo A, Yamanaka S, Yoshida Y (2016) Epigenetic variation between human induced pluripotent stem cell lines is an indicator of differentiation capacity. Cell Stem Cell 19:341–354
Nora EP, Lajoie BR, Schulz EG, Giorgetti L, Okamoto I, Servant N, Piolot T, van Berkum NL, Meisig J, Sedat J, Gribnau J, Barillot E, Blüthgen N, Dekker J, Heard E (2012) Spatial partitioning of the regulatory landscape of the X-inactivation centre. Nature 485:381–385
Paquet D, Kwart D, Chen A, Sproul A, Jacob S, Teo S, Olsen KM, Gregg A, Noggle S, Tessier-Lavigne M (2016) Efficient introduction of specific homozygous and heterozygous mutations using CRISPR/Cas9. Nature 533:125–129
Pasquali L, Gaulton KJ, Rodríguez-Seguí SA, Mularoni L, Miguel-Escalada I, Akerman I, Tena JJ, Morán I, Gómez-Marín C, van de Bunt M, Ponsa-Cobas J, Castro N, Nammo T, Cebola I, García-Hurtado J, Maestro MA, Pattou F, Piemonti L, Berney T, Gloyn AL, Ravassard P, Gómez-Skarmeta JL, Müller F, McCarthy MI, Ferrer J (2014) Pancreatic islet enhancer clusters enriched in type 2 diabetes risk-associated variants. Nat Genet 46:136–143
Rada-Iglesias A, Bajpai R, Swigut T, Brugmann SA, Flynn RA, Wysocka J (2011) A unique chromatin signature uncovers early developmental enhancers in humans. Nature 470:279–283
Rao SSP, Huntley MH, Durand NC, Stamenova EK, Bochkov ID, Robinson JT, Sanborn AL, Machol I, Omer AD, Lander ES, Aiden EL (2014) A 3D map of the human genome at kilobase resolution reveals principles of chromatin looping. Cell 159:1665–1680
Reinhardt P, Schmid B, Burbulla LF, Schöndorf DC, Wagner L, Glatza M, Höing S, Hargus G, Heck SA, Dhingra A, Wu G, Müller S, Brockmann K, Kluba T, Maisel M, Krüger R, Berg D, Tsytsyura Y, Thiel CS, Psathaki OE, Klingauf J, Kuhlmann T, Klewin M, Müller H, Gasser T, Schöler HR, Sterneckert J (2013) Genetic correction of a LRRK2 mutation in human iPSCs links parkinsonian neurodegeneration to ERK-dependent changes in gene expression. Cell Stem Cell 12:354–367
Ripke S, Neale BM, Corvin A, Walters JTR, Farh K-H, Holmans PA, Lee P, Bulik-Sullivan B, Collier DA, Huang H et al (2014) Biological insights from 108 schizophrenia-associated genetic loci. Nature 511:421–427
Rivera CM, Ren B (2013) Mapping human epigenomes. Cell 155:39–55
Roadmap Epigenomics Consortium (2015) Integrative analysis of 111 reference human epigenomes. Nature 518:317–330
Ryan SD, Dolatabadi N, Chan SF, Zhang X, Akhtar MW, Parker J, Soldner F, Sunico CR, Nagar S, Talantova M, Lee B, Lopez K, Nutter A, Shan B, Molokanova E, Zhang Y, Han X, Nakamura T, Masliah E, Yates JR 3rd, Nakanishi N, Andreyev AY, Okamoto S, Jaenisch R, Ambasudhan R, Lipton SA (2013) Isogenic human iPSC Parkinson’s model shows nitrosative stress-induced dysfunction in MEF2-PGC1a transcription. Cell 155:1351–1364
Saha K, Jaenisch R (2009) Technical challenges in using human induced pluripotent stem cells to model disease. Cell Stem Cell 5:584–595
Schadt EE, Monks SA, Drake TA, Lusis AJ, Che N, Colinayo V, Ruff TG, Milligan SB, Lamb JR, Cavet G, Linsley PS, Mao M, Stoughton RB, Friend SH (2003) Genetics of gene expression surveyed in maize, mouse and man. Nature 422:297–302
Schaffer AE, Freude KK, Nelson SB, Sander M (2010) Nkx6 transcription factors and Ptf1a function as antagonistic lineage determinants in multipotent pancreatic progenitors. Dev Cell 18:1022–1029
Schisler JC, Jensen PB, Taylor DG, Becker TC, Knop FK, Takekawa S, German M, Weir GC, Lu D, Mirmira RG, Newgard CB (2005) The Nkx6.1 homeodomain transcription factor suppresses glucagon expression and regulates glucose-stimulated insulin secretion in islet beta cells. Proc Natl Acad Sci USA 102:7297–7302
Singleton AB, Farrer M, Johnson J, Singleton A, Hague S, Kachergus J, Hulihan M, Peuralinna T, Dutra A, Nussbaum R, Lincoln S, Crawley A, Hanson M, Maraganore D, Adler C, Cookson MR, Muenter M, Baptista M, Miller D, Blancato J, Hardy J, Gwinn-Hardy K (2003) alpha-Synuclein locus triplication causes Parkinson’s disease. Science 302:841
Singleton AB, Farrer MJ, Bonifati V (2013) The genetics of Parkinson’s disease: progress and therapeutic implications. Mov Disord 28:14–23
Soldner F, Jaenisch R (2012) Medicine. iPSC disease modeling. Science 338:1155–1156
Soldner F, Laganière J, Cheng AW, Hockemeyer D, Gao Q, Alagappan R, Khurana V, Golbe LI, Myers RH, Lindquist S, Zhang L, Guschin D, Fong LK, BJ V, Meng X, Urnov FD, Rebar EJ, Gregory PD, Zhang HS, Jaenisch R (2011) Generation of isogenic pluripotent stem cells differing exclusively at two early onset parkinson point mutations. Cell 146:318–331
Soldner F, Stelzer Y, Shivalila CS, Abraham BJ, Latourelle JC, Barrasa MI, Goldmann J, Myers RH, Young RA, Jaenisch R (2016) Parkinson-associated risk variant in distal enhancer of α-synuclein modulates target gene expression. Nature 533:95–99
Takahashi K, Yamanaka S (2006) Induction of pluripotent stem cells from mouse embryonic and adult fibroblast cultures by defined factors. Cell 126:663–676
Takahashi K, Yamanaka S (2013) Induced pluripotent stem cells in medicine and biology. Development 140:2457–2461
Takahashi K, Tanabe K, Ohnuki M, Narita M, Ichisaka T, Tomoda K, Yamanaka S (2007) Induction of pluripotent stem cells from adult human fibroblasts by defined factors. Cell 131:861–872
The HD iPSC Consortium (2012) Induced pluripotent stem cells from patients with Huntington’s disease show CAG-repeat-expansion-associated phenotypes. Cell Stem Cell 11:264–278
Trynka G, Sandor C, Han B, Xu H, Stranger BE, Liu XS, Raychaudhuri S (2013) Chromatin marks identify critical cell types for fine mapping complex trait variants. Nat Genet 45:124–130
Vermunt MW, Reinink P, Korving J, de Bruijn E, Creyghton PM, Basak O, Geeven G, Toonen PW, Lansu N, Meunier C, van Heesch S, Clevers H, de Laat W, Cuppen E, Creyghton MP, Bank NB (2014) Large-scale identification of coregulated enhancer networks in the adult human brain. Cell Rep 9:767–779
Visel A, Taher L, Girgis H, May D, Golonzhka O, Hoch RV, McKinsey GL, Pattabiraman K, Silberberg SN, Blow MJ, Hansen DV, Nord AS, Akiyama JA, Holt A, Hosseini R, Phouanenavong S, Plajzer-Frick I, Shoukry M, Afzal V, Kaplan T, Kriegstein AR, Rubin EM, Ovcharenko I, Pennacchio LA, Rubenstein JL (2013) A high-resolution enhancer atlas of the developing telencephalon. Cell 152:895–908
Visel A, Blow MJ, Li Z, Zhang T, Akiyama JA, Holt A, Plajzer-Frick I, Shoukry M, Wright C, Chen F, Afzal V, Ren B, Rubin EM, Pennacchio LA (2009) ChIP-seq accurately predicts tissue-specific activity of enhancers. Nature 457:854–858
Wainger BJ, Kiskinis E, Mellin C, Wiskow O, Han SSW, Sandoe J, Perez NP, Williams LA, Lee S, Boulting G, Berry JD, Brown RH Jr, Cudkowicz ME, Bean BP, Eggan K, Woolf CJ (2014) Intrinsic membrane hyperexcitability of amyotrophic lateral sclerosis patient-derived motor neurons. Cell Rep 7:1–11
Wamstad JA, Wang X, Demuren OO, Boyer LA (2014) Distal enhancers: new insights into heart development and disease. Trends Cell Biol 24:294–302
Wang X, Tucker NR, Rizki G, Mills R, Krijger PH, de Wit E, Subramanian V, Bartell E, Nguyen X-X, Ye J, Leyton-Mange J, Dolmatova EV, van der Harst P, de Laat W, Ellinor PT, Newton-Cheh C, Milan DJ, Kellis M, Boyer LA (2016) Discovery and validation of sub-threshold genome-wide association study loci using epigenomic signatures. Elife 5:e10557
Ward LD, Kellis M (2012) Interpreting noncoding genetic variation in complex traits and human disease. Nat Biotechnol 30:1095–1106
Warren L, Manos PD, Ahfeldt T, Loh Y-H, Li H, Lau F, Ebina W, Mandal PK, Smith ZD, Meissner A, Daley GQ, Brack AS, Collins JJ, Cowan C, Schlaeger TM, Rossi DJ (2010) Highly efficient reprogramming to pluripotency and directed differentiation of human cells with synthetic modified mRNA. Cell Stem Cell 7:618–630
Young JE, Boulanger-Weill J, Williams DA, Woodruff G, Buen F, Revilla AC, Herrera C, Israel MA, Yuan SH, Edland SD, Goldstein LSB (2015) Elucidating molecular phenotypes caused by the SORL1 Alzheimer’s disease genetic risk factor using human induced pluripotent stem cells. Cell Stem Cell 16:373–385
Yu DX, Marchetto MC, Gage FH (2013) Perspective. Cell Stem Cell 12:678–688
Yu J, Vodyanik M, Smuga-Otto K, Antosiewicz-Bourget J, Frane J, Tian S, Nie J, Jonsdottir G, Ruotti V, Stewart R, Slukvin I, Thomson J (2007) Induced pluripotent stem cell lines derived from human somatic cells. Science 318:1917–1920
Zhang Y, Pak C, Han Y, Ahlenius H, Zhang Z, Chanda S, Marro S, Patzke C, Acuna C, Covy J, Xu W, Yang N, Danko T, Chen L, Wernig M, Südhof TC (2013) NeuroResource. Neuron 78:785–798
Acknowledgments
This work was supported by grants NS088538, MH104610 and HD045022 from the NIH.
Author information
Authors and Affiliations
Corresponding author
Editor information
Editors and Affiliations
Rights and permissions
Open Access This chapter is licensed under the terms of the Creative Commons Attribution 4.0 International License (http://creativecommons.org/licenses/by/4.0/), which permits use, sharing, adaptation, distribution and reproduction in any medium or format, as long as you give appropriate credit to the original author(s) and the source, provide a link to the Creative Commons license and indicate if changes were made.
The images or other third party material in this chapter are included in the chapter's Creative Commons license, unless indicated otherwise in a credit line to the material. If material is not included in the chapter's Creative Commons license and your intended use is not permitted by statutory regulation or exceeds the permitted use, you will need to obtain permission directly from the copyright holder.
Copyright information
© 2017 The Author(s)
About this chapter
Cite this chapter
Soldner, F., Jaenisch, R. (2017). In Vitro Modeling of Complex Neurological Diseases. In: Jaenisch, R., Zhang, F., Gage, F. (eds) Genome Editing in Neurosciences. Research and Perspectives in Neurosciences. Springer, Cham. https://doi.org/10.1007/978-3-319-60192-2_1
Download citation
DOI: https://doi.org/10.1007/978-3-319-60192-2_1
Published:
Publisher Name: Springer, Cham
Print ISBN: 978-3-319-60191-5
Online ISBN: 978-3-319-60192-2
eBook Packages: Biomedical and Life SciencesBiomedical and Life Sciences (R0)