Abstract
Background
Wilms’ tumor (WT) is the most common renal tumor in childhood. Pyroptosis, a type of inflammation-characterized and immune-related programmed cell death, has been extensively studied in multiple tumors. In the current study, we aim to construct a pyroptosis-related gene signature for predicting the prognosis of Wilms’ tumor.
Methods
We acquired RNA-seq data from TARGET kidney tumor projects for constructing a gene signature, and snRNA-seq data from GEO database for validating signature-constructing genes. Pyroptosis-related genes (PRGs) were collected from three online databases. We constructed the gene signature by Lasso Cox regression and then established a nomogram. Underlying mechanisms by which gene signature is related to overall survival states of patients were explored by immune cell infiltration analysis, differential expression analysis, and functional enrichment analysis.
Results
A pyroptosis-related gene signature was constructed with 14 PRGs, which has a moderate to high predicting capacity with 1-, 3-, and 5-year area under the curve (AUC) values of 0.78, 0.80, and 0.83, respectively. A prognosis-predicting nomogram was established by gender, stage, and risk score. Tumor-infiltrating immune cells were quantified by seven algorithms, and the expression of CD8( +) T cells, B cells, Th2 cells, dendritic cells, and type 2 macrophages are positively or negatively correlated with risk score. Two single nuclear RNA-seq samples of different histology were harnessed for validation. The distribution of signature genes was identified in various cell types.
Conclusions
We have established a pyroptosis-related 14-gene signature in WT. Moreover, the inherent roles of immune cells (CD8( +) T cells, B cells, Th2 cells, dendritic cells, and type 2 macrophages), functions of differentially expressed genes (tissue/organ development and intercellular communication), and status of signaling pathways (proteoglycans in cancer, signaling pathways regulating pluripotent of stem cells, and Wnt signaling pathway) have been elucidated, which might be employed as therapeutic targets in the future.
Similar content being viewed by others
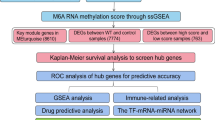
Introduction
Wilms’ tumor (WT) is the most common renal tumor and the second most common malignant abdominal tumor in childhood. The incidence of WT in general population is 0.5–7.5 per million and is lower in high-income areas [1]. Current treatment strategy for WT is based on genetic markers, histology, stage, and other risk factors, which spare children with low-risk tumors from intensive treatment and intensify treatment for children with high-risk tumors [2]. Outcomes and long-term survival have improved over the decades [1, 3].
WTs are divided into two histologies: the favorable histology (FH) and the unfavorable histology (UH) which includes anaplastic histology (AH), clear cell sarcoma of the kidney (CCSK), and malignant rhabdoid tumor (MRT) [4]. Despite the advances in multi-disciplinary treatment and risk-based management of WT, the current prognoses of patients with unfavorable histology remain dismal [5].
Pyroptosis is a type of gasdermin-mediated, inflammation-characterized, and immune-related programmed cell death, which has received increasing attention due to its association with immune response in neoplastic and non-neoplastic diseases [6]. The role of pyroptosis has been extensively elucidated in cardiovascular diseases, nervous system disorders, psychiatric disorders, infection diseases, periodontal diseases, etc. [7,8,9,10,11]. Further, pyroptosis has been proven to be closely related to the biological behavior of multiple tumors [12].
The tumor microenvironment (TME) consists of miscellaneous cell types, such as cancer cells, cancer stem cells, immune cells, stromal cells, and vascular endothelial cells. Tumor-infiltrating immune cells are found generic in tumor tissues with complex tumor-antagonizing or tumor-promoting functions, which surprisingly can affect the hallmarks of the tumor [13]. Quantification methods of these cells are divided into two categories: methods of enrichment on marker genes and methods leveraging the deconvolution algorithm [14, 15]. Each of these methods has a property to allow intra- or inter-sample comparison. We used several authoritative methods in current research.
The RNA-sequencing technique has been extensively employed to identify transcriptome profiles of various tumors [16], based on which several gene signatures have been established for the prognosis of WT [17, 18]. We have previously constructed a ferroptosis-related lncRNA signature in WT [17]. To the best of our knowledge, no signature based on pyroptosis for WT was reported. Here, we established a pyroptosis-related gene signature from the RNA-seq data of WT and constructed a nomogram with the signature and clinical variables. Immune infiltration characteristics of the signature were then identified. We further used snRNA-seq data for validation of our signature. The overall workflow is presented in Fig. 1.
Material and methods
Acquisition of gene expression profiles and case information
RNA-seq data (counts value) of 132 tissue samples derived from WT patients were downloaded from Xena datasets (https://xena.ucsc.edu/), which originally came from the TARGET kidney tumor projects (https://ocg.cancer.gov/programs/target) [19]. Of the 132 samples, 126 were from tumor tissues and six were from normal tissues. Supplemental clinical and survival information was acquired from the same source. Preprocessing of expression matrices was completed before further analysis. Briefly, the logarithmed count values downloaded from Xena datasets were transformed by taking anti-logarithms. Genes with counts equal to zero in all the samples were eliminated and a processed counts matrix was obtained, which was used for differential expression analysis. The processed counts data was transformed to transcript per million (TPM) [20], which was used for other subsequent analyses.
Identification of prognosis-associated pyroptosis-related genes
Pyroptosis-related genes (PRGs) were obtained from three online databases, among which were Gene Ontology Biological Process database (http://geneontology.org/) [21, 22], Molecular Signatures Database (MSigDB) (https://www.gsea-msigdb.org/gsea/index.jsp) [23, 24], and GeneCards database (https://www.genecards.org/) [25]. The union of genes was considered PRGs. The PRGs were then filtered by univariate Cox analysis (uniCox) of overall survival (OS) with R package survival (version 3.5–5, https://github.com/therneau/survival), in which PRGs with p-values less than 0.05 were selected as prognosis-associated PRGs.
Generation of a pyroptosis-related gene signature
The prognosis-associated PRGs were further admitted into Lasso Cox regression analysis with R package glmnet (version 4.1–7, https://glmnet.stanford.edu/) [26]. The function “cv.glmnet” was run for cross-validation and returned a lambda sequence, among which the value of lambda that gave a minimum mean cross-validated error (cvm) was used to extract model coefficients for each PRG. Only PRG with a non-zero coefficient was included in constructing a prognostic gene signature which was then established with eligible PRGs and their coefficients. After, the risk score was calculated for each sample as the following formula: Risk score = Σ Coefficient (PRGs) × Expression (PRGs). Further, samples were divided into high- and low-risk groups by the median risk score. The signature was then verified by plotting Kaplan–Meier (K-M) curve and time-dependent receiver operating characteristic (ROC) curves of 1, 3, and 5 years.
Construction of a prognosis-predicting model
Univariate Cox regression was applied to risk scores and four clinical variables including gender, age, histology of the tumor, and stage to test the predicting capacity of each variable for OS of patients. Only variables with p-values < 0.05 were included into Multivariate Cox regression (multiCox). Multivariate Cox regression method was then applied to these variables, based on which a nomogram was further constructed for the prediction of 1-, 3-, and 5-year overall survival states. And the model was validated by C-index, calibration curves, and decision curve analysis (DCA). Uni- and multi-Cox regression was performed by R package survival (version 3.5–5, https://github.com/therneau/survival) and the nomogram was constructed by R package rms (version 6.7–0, https://hbiostat.org/r/rms/).
Quantification of tumor-infiltrating immune cells
Three categories of immune cell infiltration analysis were performed among tumor samples. First, the ESTIMATE algorithm was employed to estimate tumor purity with R package estimate (version 1.0.13/r21, https://R-Forge.R-project.org/projects/estimate/) [27]. Second, three marker-gene-based approaches including ssGSEA, xCell, and MCP-counter algorithm were used to calculate enrichment scores of various immune cell types by R packages GSVA (version 1.50.1, https://github.com/rcastelo/GSVA) [28], xCell (version 1.1.0, https://github.com/dviraran/xCell) [29], and MCPcounter (version 1.2.0, https://github.com/ebecht/MCPcounter) [30], respectively. Last, three deconvolution-based approaches which include quanTIseq, CIBERSORT, and CIBERSORT abs. mode were employed to compute relative or absolute fractions of different immune cell types with R packages quantiseqr (version 1.8.0, https://bioconductor.org/packages/quantiseqr) [31] and immunedeconv (version 2.0.3, https://github.com/omnideconv/immunedeconv) [14], respectively. Intra- or inter-sample comparisons were performed in accordance with the characteristic of each algorithm [15].
Identification of differentially expressed genes and functional enrichment analysis
Differentially expressed genes (DEGs) were identified between high- and low-risk groups by R package limma (version 3.56.2, https://bioinf.wehi.edu.au/limma/) [32]. The Benjamini-Hochberg’s method was used to adjust p-values for multiple comparisons [33]. Cut-off threshold was set as |log2FC|> 1 and adjusted p-value < 0.05. Function and pathway enrichment analysis of Gene Ontology (GO) database and Kyoto Encyclopedia of Genes and Genomes (KEGG) database were performed by R package clusterProfiler (version 4.8.1, https://www.liebertpub.com/doi/full/10.1089/omi.2011.0118) [34].
Validation of pyroptosis-related gene signature by snRNA-seq data
Single nuclear RNA-seq (snRNA-seq) data of two WT samples (GSM6025607, and GSM6025616) were gained from GEO database (https://www.ncbi.nlm.nih.gov/geo/). The two snRNA-seq samples are of two different histological types (GSM6025607 was the anaplastic type whereas GSM6025616 was the favorable type) and were analyzed by R package Seurat (version 4.3.0.1, https://github.com/satijalab/seurat) [35]. The number of genes in each cell and the fraction of mitochondrial genes were detected. Cells with unique feature counts of 200–4000 and mitochondrial counts < 5% were sustained. After, the snRNA-seq data was normalized by method “LogNormalize”. Further, principal component analysis (PCA) and t-distributed statistical neighbor embedding (tSNE) were performed to accomplish dimensional reduction. Based on the marker genes, clusters were annotated by results of authoritative literature [36].
Statistical analysis and data visualization
All statistical analyses were conducted by R (version 4.1.2). Comparative analysis for quantitative data between groups was performed by Wilcoxon rank test and for qualitative data was performed by Chi-square test. Linear correlation analysis between variables was performed by Spearman correlation analysis. P-value threshold was set as 0.05. Data visualization processes were conducted with R, except a Venn diagram was processed by Python (version 3.10.2).
Results
Prognosis-associated PRGs were identified by uniCox regression
A union gene list of 265 genes was exhibited in Fig. 2A and Table S1, among which were not only protein-coding genes but also genes that do not code for proteins. All of them were included in univariate Cox analysis (Table S2), after which 16 genes were kept for further research. The hazard ratio (HR) and p-value of each gene were presented in Fig. 2B. The differential expression levels of these genes between tumors and normal tissues were exhibited in Fig. 2C.
Identification of prognosis-associated PRGs. A The Venn diagram of PRGs from Gene Ontology Biological Process database, Molecular Signatures Database, and GeneCards database. B The forest plot of prognosis-associated PRGs. C The heatmap of gene expression levels (Z-score scaled) of prognosis-associated PRGs in tumor and normal tissues. HR, hazard ratio; HR.95L, lower 95% hazard ratio; HR.95H, higher 95% hazard ratio
A Pyroptosis-related gene signature was constructed by Lasso Cox regression
The model-fitting and cross-validation processes were exhibited in Fig. 3A, B. The selected value of lambda that gave a minimum mean cross-validated error (cvm) was marked by the left dotted line in Fig. 3B. 16 candidate PRGs were analyzed by Lasso Cox regression and 14 PRGs were eligible to construct a prognostic gene signature. Signature-constructing genes and their coefficients were listed in Table 1. Among tumor patients, the correlations between risk scores and OS states were presented in Fig. 3C. K-M curves showed that patients in the low-risk group have better survival states than patients in the high-risk group (Fig. 3D).
Generation of a pyroptosis-related gene signature. A, B The lasso Cox regression profile of 14 prognosis-associated PRGs. C The distribution of risk scores between OS states of patients. D The K-M curve between high- and low-risk groups. E, F The distribution of risk scores and OS states among patients. G The time-dependent ROC curves at 1, 3, and 5 years. H The heatmap of gene expression levels (Z-score scaled) of prognosis-associated PRGs between high- and low-risk groups. OS, overall survival; ROC, receiver operating characteristic; AUC, area under the curve
The distribution of risk scores, OS states, and signature genes’ expression was presented in Fig. 3E-G. To confirm the independent predicting power of the gene signature, time ROC curves were plotted (Fig. 3H). And the signature was proved to have a moderate to high predicting capacity with 1-, 3-, and 5-year area under the curve (AUC) values of 0.78, 0.80, and 0.83, respectively.
A prognosis-predicting model was constructed with 3 variables
Despite our best efforts, only four clinical variables with high-quality information were extracted, including gender, age, histology of the tumor, and stage (Table 2). Univariate Cox regression analysis was employed to risk score and four clinical variables (Fig. 4A, Table S3). Gender, stage, and risk score were further included into multivariate Cox regression analysis (Fig. 4B, Table S4). A nomogram based on multiCox was exhibited in Fig. 4C. The C-index was 0.758, which indicated a moderate predicting efficiency of the model. The model showed good calibration with the diagonal (Fig. 4D). Moreover, DCA curves showed good benefits in prediction (Fig. 4E-G).
Construction of a prognosis-predicting model. A, B The forest plots of uni- and multi-Cox regression for risk score and clinical variables. C The nomogram is constructed by gender, stage, and risk score. D The calibration plot at 1, 3, and 5 years. E–G The DCA curves at 1, 3, and 5 years. HR, hazard ratio; HR.95L, lower 95% hazard ratio; HR.95H, higher 95% hazard ratio; OS, overall survival; DCA, decision curve analysis
Tumor-infiltrating immune cells were quantified by multiple algorithms
Various infiltrating immune cells exert complex functions and impact on biological behavior of tumors. Stromal cells are also thought to be significant for tumor growth [13]. Stromal Score, Immune Score, ESTIMATE Score, and Tumor Purity of each sample were evaluated. The Stromal Scores were significantly higher in low-risk group than those in high-risk group (Fig. 5A). However, no statistical difference was sighted in the Immune Scores (Figure S1A). The ESTIMATE Scores and Tumor Purities indicated that tumors in high-risk group had higher tumor purity (Fig. 5B, C), which might be dominantly caused by Stromal Scores.
Estimation of tumor purity and quantification of tumor-infiltrating immune cells by marker-gene-based approaches. A The estimation of Stromal Scores, ESTIMATE Scores, and Tumor Purity of tumors. D The heatmap of enrichment scores (ES) of immune infiltrating cells by marker-gene-based approaches. E-J The correlation plots of ES of Th2 cells, CD8 + T cells, and B cells with risk scores
The results of marker-gene-based immune cells infiltration analyses were exhibited in heatmap (Fig. 5D). The enrichment scores (ES) of three immune cell types (Th2 cells, CD8( +) T cells, and B cells) were statistically significant between groups in more than one algorithm. The correlation between ES of these three cell types and risk score is shown in Fig. 5E-J. Except for Th2 cells in ssGSEA algorithm, 5 scatter plots showed a low or negligible positive correlation between ES and risk score.
The absolute fractions of immune infiltrating cells were quantified by quanTIseq algorithm (Fig. 6A, B). Immune cells took up a minority of tumor tissue, while “other” cells occupied the majority. Among the immune cell types, CD4 + T cells had a predominantly quantity advantage over other immune cells, while macrophages type 1 had a minute quantity in both groups. Between high- and low-risk groups, three types of immune cells (dendritic cells, macrophages type 2, and CD8( +) T cells) were statistically different in absolute fractions. The correlations of fractions of these three cell types with risk score were shown in Fig. 6C-E, of which CD8( +) T cells had a low or negligible positive correlation with risk score, while macrophages type 2 and dendritic cells had a low or negligible negative correlation with risk score. The correlations among cell types in quanTIseq algorithm were exhibited in Figure S1B.
Quantification of tumor-infiltrating immune cells by deconvolution-based approaches. A, B The differences of absolute fractions of immune infiltrating cells between high- and low-risk groups by quanTIseq algorithm. C-E The correlation plots of absolute fractions of dendritic cells, macrophages type 2, and CD8 + T cells with risk score. F The fraction of each type of immune infiltrating cells in all immune cells by CIBERSORT. G The estimated absolute fractions of immune infiltrating cells. *P value < 0.05, **P value < 0.01, ***P value < 0.001
The fraction of each type of immune infiltrating cells in all immune cells was evaluated by CIBERSORT, as is shown in Fig. 6F. Further, CIBERSORT abs. mode was applied and estimated absolute fractions of each immune cell type were exhibited in Fig. 6G, in which the fraction differences of three immune cell types (resting CD4 + T memory cells, resting NK cells, and macrophages type 2) were statistically significant.
Differential expression and functional enrichment analysis
DEGs between the two groups were visualized in Fig. 7A. Results of functional enrichment analyses for GO and KEGG databases for DEGs were shown in Fig. 7B, C. Overall, enrichment for biological process (BP), cellular component (CC), and molecular function (MF) mainly focused on tissue/organ development and intercellular communication. Of note, enrichment for pathways in KEGG database indicated that DEGs were mainly enriched in proteoglycans in cancer, signaling pathways regulating pluripotent of stem cells, and Wnt signaling pathway, which were highly related to tumor invasion and metastasis [37,38,39,40,41,42,43].
Differential expression and functional enrichment analysis between high- and low-risk groups. A The valcano plot of DEGs. B, C Function and pathway enrichment analysis of GO and KEGG database. GO, gene ontology; KEGG, Kyoto encyclopedia of genes and genomes. p.adjust, adjusted p-value; FC, fold change
Validation of pyroptosis-related gene signature by snRNA-seq data
Favorable histology and anaplastic histology are two distinct groups of WT. The snRNA-seq data of samples from each histologic type were analyzed respectively. Consistent with the annotation method of an authoritative research, clusters of the two samples were annotated in Fig. 8A, C. The sample of favorable histology had clusters of intermediate population-like cancer cells, ureteric bud-like cancer cells, fibroblasts-like cancer cells, nephron epithelial cells, proliferating T cells, and mononuclear phagocytes. The sample of anaplastic histology had clusters of ureteric bud-like cancer cells, primitive vesicle-like cancer cells, endothelium-like cancer cells, mesangial cells, proliferating T cells, and mononuclear phagocytes.
Validation of pyroptosis-related gene signature by snRNA-seq data. A, B The annotation of clusters and distribution of signature genes in the sample of favorable histology. C, D The annotation of clusters and distribution of signature genes in the sample of anaplastic histology. tSNE, t-distributed stochastic neighbor embedding
Of the 14 signature genes, NAIP and TP63 were considered marker genes for mononuclear phagocytes and ureteric bud-like cancer cells respectively in the sample of favorable histology. In the sample of anaplastic histology, GLMN and NAIP were considered marker genes for primitive vesicle-like cancer cells and endothelium-like cancer cells, respectively.
The distribution of 14 signature genes in each cell type and all cells was exhibited in Fig. 8B, D, and Figure S1C, D. The signature genes’ expression was relatively low in ureteric bud-like cancer cells, fibroblasts-like cancer cells, and endothelium-like cancer cells in the two samples. Of note, NLRP6 and NAIP had the highest expression while GSDMD and TP63 had the lowest expression in both the samples.
Discussion
Wilms’ tumor is an embryonal malignancy derived from nephrogenic blastemal cells in nephrogenic rests [2]. Current treatment strategy for WT from Children’s Oncology Group (COG) is based on traditional risk factors, including histology, stage, age, tumor weight, response to therapy, and loss of heterozygosity at 1p and 16q [44]. These prognostic factors are derived from elaborate clinical trials and have been guiding operative therapy, chemotherapy, and radiotherapy of WT. Here, we have screened out gender and stage as prognostic clinical factors in WT. To acquire a more precise risk-classifying approach, exploring specific patterns of gene expression profiles in tumor tissue is necessary.
Characterized by inflammation, pyroptosis is a more intense pattern of cell death compared with apoptosis, leading to pore formation on cell membrane, chromatin fragmentation, cell swelling, and osmotic lysis of the cell [6]. Exact molecular relationship between pyroptosis and tumors’ bio-behavior remains unclear, but studies have found that enhancement of pyroptosis in tumors leads to inhibition of tumor progression [45,46,47,48,49]. To the best of our knowledge, few studies have elucidated the role of pyroptosis in WT.
In this study, we have established a pyroptosis-associated gene signature with 14 PRGs, among which CARD8, GSDMD, TP63, TFAP2A, IRF2, and PCSK9 were negatively correlated with risk score, while NAIP, NLRP6, SDHB, MIR30C1, APIP, CASP9, MIR103A1, and GLMN were positively correlated with risk score.
Caspase recruitment domain family member 8 (CARD8) is an inflammasome sensor, which ultimately activates GSDMD and inflammatory cytokines, leading to pyroptosis [50]. Gasdermin D (GSDMD) is the main executioner of pyroptosis, and is considered as a tumor suppressor [51, 52]. It has been deeply studied in pyroptotic cell death. Transcription factor AP-2 alpha (TFAP2A) and interferon regulatory factor 2 (IRF2) were proven to transcriptionally induce GSDMD by binding to its promoter, which subsequently induces pyroptosis [53, 54]. The role of proprotein convertase subtilisin/kexin type 9 (PCSK9) in pyroptosis has been clarified in cardiomyocytes and vascular endothelial cells [55, 56]. These five genes are pyroptosis-executive or pyroptosis-promoting, and in the sight of the tumor-suppressing role of pyroptosis, these are reasonable to be negatively correlated with risk score.
APAF1 interacting protein (APIP) is validated to inhibit pyroptosis and apoptosis [57, 58]. Glomulin, FKBP Associated Protein (GLMN) is a negative regulator of pyroptosis via regulating cIAP-mediated inflammasome activation [59, 60]. These two genes have the pyroptosis-inhibiting effect, which is easy to explain the positive correlations with risk score.
NLR family apoptosis inhibitory protein (NAIP) is necessary for inflammasome assembly which subsequently cleaves caspase-1 and leads to pyroptosis [61]. Like CARD8, NLR family pyrin domain containing 6 (NLRP6) is also an inflammasome sensor that mediates inflammasome activation and promotes recruitment of effector proinflammatory caspases [62]. Overexpression of succinate dehydrogenase complex iron sulfur subunit B (SDHB) has been proven to enhance pyroptosis in vascular endothelial cells [63]. Caspase 9 (CASP9) has the ability to cleave and activate caspase-3, which subsequently activates Gasdermin E (GSDME) [64]. These four genes are pyroptosis-executive or pyroptosis-promoting genes. Providing the negative correlation of pyroptosis with tumor progression, the four genes are supposed to be negatively correlated with risk score, which contradicts our observations.
Here are possible explanations. First, the predominant role of pyroptosis in the bio-behavior of tumors is tumor suppressor, but the adverse effect may exist in certain tumor microenvironments. Second, except for pyroptosis, the functions of genes are most likely to relate to multiple biological processes. Such as NAIP can also act as an anti-apoptotic protein by inhibiting caspase-3, and caspase-7 [65, 66], which may promote tumorigenesis and tumor invasion. Third, few studies have explored the exact roles of certain genes (NLRP6 and SDHB) in WT. Although NLRP6 was reported to serve as a tumor suppressor in colorectal cancer, hepatocellular carcinoma, and gastric cancer [67,68,69], it was reported to restore immune evasion and radio-resistance in glioma through ASC/caspase-1/IL-1β axis [70]. The SDHB gene encodes the iron-sulfur protein subunit of the succinate dehydrogenase enzyme complex which plays a critical role in respiratory electron transport and tricarboxylic acid cycle [71]. Higher gene expression of SDHB may provide more energy for tumor cells in WT. The roles of these genes in WT need further investigation. Last but not the least, genes may have complex interactions with the tumor microenvironment. For example, CASP9 inhibition triggers immunogenic cell death, increases tumor-intrinsic innate sensing, and induces remarkable anti-tumor effects in chemotherapy-induced anti-tumor immunity [72]. Higher gene expression of CASP9 may exhibit less immunogenic cell death and worse prognosis in WT. The above-mentioned theoretical deductions need further experiment-based validation.
Tumor protein p63 (TP63) has two isoforms, in which TAp63 is thought of as a tumor suppressor, while ΔNp63 is considered as an oncogene [73]. In the sample set of our study, TAp63 may be functionally predominant over ΔNp63. MicroRNA 30c-1 (MIR30C1) is known to inhibit the progression of prostate cancer and the invasion of melanoma [74, 75]. MicroRNA 103a-1 (MIR103A1) was found to have dual effects on different tumor types [76,77,78]. In our results of bioinformatics analysis, MIR103A1 is most likely to negatively regulate the progression of WT.
Solid tumor tissue does not only have cancer cells but also immune inflammatory cells, endothelial cells, etc. Tumor-promoting and tumor-antagonizing immune cells exist simultaneously in tumors [13]. In our observations of immune cell infiltration, the expression of CD8( +) T cells, B cells, and Th2 cells are positively correlated with risk score, while the expression of dendritic cells and type 2 macrophages are negatively correlated with risk score.
Dendritic cells can either suppress tumor progression or drive tolerance in the TME [79]. As for our results, dendritic cells are most likely to play a tumor suppressor role in WT. B cells are considered to have dual effects in the TME, and Th2 cells are the intrinsic helper for B cells [80]. They are both positively related to risk score in our research, based on which we can assume that the tumor-promoting effect overweighs the tumor-suppressing effect.
CD8( +) T cells, also called cytotoxic lymphocytes (CTLs), have long been known to relate to better survival in tumors [81,82,83]. But in our results, it is positively related to risk score. Type 2 macrophages are known to be tumor-promoting [84, 85], which contradicts our observations, too. There are several explanations. First, it is the enrichment scores or fractions of immune cells that stand for expression levels, but not the real quantity of them. Second, despite our best efforts, only one dataset in TARGET database was included in our research, and no validating dataset of good quality was harnessed. Thereby, the sample number of tumors is only 126, which may be too small to get completely correct results. Third, it may be reasonable to assume that the more invasive the tumor is, the more activation of immune system to fight against it. Based on this assumption, the role of immune infiltrating cells in WT is more of a defensive reactor than a prognostic indicator.
Hence, the distinct immune cells infiltration landscape between high- and low-risk groups was mainly made up of CD8( +) T cells, B cells, Th2 cells, dendritic cells, and type 2 macrophages, which were validated by various algorithms.
WT classically consists of three elements: blastemal, stromal, and epithelial tubules, which are normal components of developing kidneys. Less commonly, skeletal muscle, cartilage, osteoid, or adipose tissue can also be found in WT [2, 4]. In our study, GO BP analysis showed DEGs between two groups were mainly enriched in tissue/organ development, the top five of which were pattern specification process, muscle tissue development, connective tissue development, cartilage development, and ossification. These observation indicates that the extent of development of embryonal components in WT is correlated with tumor progression and prognostic risk. KEGG analysis showed pathway enrichment in signaling pathways regulating pluripotency of stem cells, proteoglycans in cancer, and Wnt signaling pathway. WT has similar components to fetal kidneys, where pluripotent stem cells (PSCs) play a key role in tissue formation and organ development. Our results suggest the signaling pathway that regulates PSCs is correlated with tumorigenesis of WT. Proteoglycans (PGs) and the Wnt signaling pathway have been extensively studied in multiple cancers, both of which are closely related to tumor progress and invasion [37,38,39,40,41,42,43].
Therefore, the extent of development of embryonal components in WT together with the status of proteoglycans and Wnt signaling pathway might be intermediate contributors to tumor progression.
Single-cell RNA-seq (scRNA-seq) and single-nucleus RNA-seq (snRNA-seq) have received increasing attention over the years. Both techniques have remarkable advantages over bulk RNA-seq. We have employed a recently published snRNA-seq dataset of WT to validate our gene signature. In accordance with a former authoritative study [36], cell clusters are similar to normal constituents in fetal kidneys, which is a distinct characteristic of WT. Combined with the results of GO/KEGG analysis, we might infer that the capacity of tumor cells to generate various renal cells isn’t lost or is required in WT.
Thus, the snRNA-seq dataset has facilitated the validation of signature-establishing genes and has supported the renal lineage-generating ability of tumor cells in WT.
The tumor microenvironment has complex gene interactions and cell cross-talks, which form latent networks. Identifying key modules and hub genes by weighted gene co-expression network analysis might provide a deeper understanding of the roles of signature-constructing genes and tumor-infiltrating immune cells like another study [86], which needs future exploration.
Several limitations are supposed to be acknowledged. First, as mentioned before, only one bulk RNA-seq dataset of good quality was used in our research, which might not draw completely correct conclusions. Second, due to the particularities of constituents of WT, the annotation process for each cluster in snRNA-seq analysis was mainly based on one literature of authority, which might lack rigor. Last, compared with clinical factors, there is still a long way to go to perform risk classification based on tumor transcriptome.
The current study is mainly based on retrospective data analysis and in silico experiments. Further validation is essential to confirm the exact roles of signature-constructing genes and tumor-infiltrating immune cells. For future investigation, we aim to perform gene-overexpression or knockdown in WT cell lines and observe changes in proliferation, migration, invasion, and other malignant behavior in vitro and in vivo. Moreover, a long-term follow-up of patient cohorts grouped by the gene signature will provide validation for the current study.
Conclusions
Here, we have established a pyroptosis-related gene signature of moderate to high predicting capacity with 14 PRGs and have constructed a prognostic-predicting nomogram with risk score and two clinical variables. Moreover, immune cell infiltration analysis, differential expression analysis, and functional enrichment analysis were performed to further explore underlying mechanisms contributing to the differences in survival states. Our research provides insights into the role of pyroptosis and possible therapeutic targets in Wilms’ tumor.
Availability of data and materials
The datasets analyzed during the current study are available in the Xena datasets (https://xena.ucsc.edu/) and GEO database (https://www.ncbi.nlm.nih.gov/geo/).
Abbreviations
- WT:
-
Wilms’ tumor
- PRGs:
-
Pyroptosis-related genes
- AUC:
-
Area under the curve
- FH:
-
Favorable histology
- UH:
-
Unfavorable histology
- AH:
-
Anaplastic histology
- CCSK:
-
Clear cell sarcoma of the kidney
- MRT:
-
Malignant rhabdoid tumor
- TME:
-
Tumor microenvironment
- snRNA-seq:
-
Single nuclear RNA-seq
- MSigDB:
-
Molecular Signatures Database
- OS:
-
Overall survival
- K-M:
-
Kaplan–Meier
- ROC:
-
Receiver operating characteristic
- DCA:
-
Decision curve analysis
- DEGs:
-
Differentially expressed genes
- GO:
-
Gene Ontology
- KEGG:
-
Kyoto Encyclopedia of Genes and Genomes
- PCA:
-
Principal component analysis
- tSNE:
-
T-distributed statistical neighbor embedding
- HR:
-
Hazard ratio
- ES:
-
Enrichment score
- BP:
-
Biological process
- CC:
-
Cellular component
- MF:
-
Molecular function
- COG:
-
Children’s Oncology Group
- CARD8:
-
Caspase recruitment domain family member 8
- GSDMD:
-
Gasdermin D
- TFAP2A:
-
Transcription factor AP-2 alpha
- IRF2:
-
Interferon regulatory factor 2
- PCSK9:
-
Proprotein convertase subtilisin/kexin type 9
- APIP:
-
APAF1 interacting protein
- GLMN:
-
Glomulin, FKBP Associated Protein
- NAIP:
-
NLR family apoptosis inhibitory protein
- NLRP6:
-
NLR family pyrin domain containing 6
- SDHB:
-
Succinate dehydrogenase complex iron sulfur subunit B
- CASP9:
-
Caspase 9
- GSDME:
-
Gasdermin E
- TP63:
-
Tumor protein p63
- MIR30C1:
-
MicroRNA 30c-1
- MIR103A1:
-
MicroRNA 103a-1
- CTLs:
-
Cytotoxic lymphocytes
- PSCs:
-
Pluripotent stem cells
- PGs:
-
Proteoglycans
- scRNA-seq:
-
Single-cell RNA-seq
- DAWT:
-
Diffuse anaplasia Wilms’ tumor
- FHWT:
-
Favorable histology Wilms’ tumor
References
Spreafico F, Fernandez CV, Brok J, Nakata K, Vujanic G, Geller JI, Gessler M, Maschietto M, Behjati S, Polanco A, et al. Wilms tumour. Nat Rev Dis Primers. 2021;7(1):75.
Moch H, Cubilla AL, Humphrey PA, Reuter VE, Ulbright TM. The 2016 WHO classification of tumours of the urinary system and male genital organs—part A: renal, penile, and testicular tumours. Eur Urol. 2016;70(1):93–105.
Ekenze SO, Okafor OC, Obasi AA, Okafor DC, Nnabugwu II. Wilms tumor in Africa: a systematic review of management challenges and outcome in two decades (2000–2019). Pediatr Blood Cancer. 2020;67(11):e28695.
Ehrlich PF, Shamberger RC. Chapter 30 - Wilms' Tumor. In: Pediatric Surgery (Seventh Edition). Edited by Coran AG. Philadelphia: Mosby; 2012. p. 423–440.
Dome JS, Graf N, Geller JI, Fernandez CV, Mullen EA, Spreafico F, Van den Heuvel-Eibrink M, Pritchard-Jones K. Advances in Wilms tumor treatment and biology: progress through international collaboration. J Clin Oncol. 2015;33(27):2999–3007.
Yu P, Zhang X, Liu N, Tang L, Peng C, Chen X. Pyroptosis: mechanisms and diseases. Signal Transduct Target Ther. 2021;6(1):128.
Zhaolin Z, Guohua L, Shiyuan W, Zuo W. Role of pyroptosis in cardiovascular disease. Cell Prolif. 2019;52(2):e12563.
Zhang X, Zhang Y, Li R, Zhu L, Fu B, Yan T. Salidroside ameliorates Parkinson’s disease by inhibiting NLRP3-dependent pyroptosis. Aging (Albany NY). 2020;12(10):9405–26.
Sordi MB, Magini RS, Panahipour L, Gruber R. Pyroptosis-mediated periodontal disease. Int J Mol Sci. 2021;23(1):372.
Li Y, Song W, Tong Y, Zhang X, Zhao J, Gao X, Yong J, Wang H. Isoliquiritin ameliorates depression by suppressing NLRP3-mediated pyroptosis via miRNA-27a/SYK/NF-kappaB axis. J Neuroinflammation. 2021;18(1):1.
Gao YL, Zhai JH, Chai YF. Recent advances in the molecular mechanisms underlying pyroptosis in sepsis. Mediators Inflamm. 2018;2018:5823823.
Fang Y, Tian S, Pan Y, Li W, Wang Q, Tang Y, Yu T, Wu X, Shi Y, Ma P, Shu Y. Pyroptosis: a new frontier in cancer. Biomed Pharmacother. 2020;121:109595.
Hanahan D, Weinberg RA. Hallmarks of cancer: the next generation. Cell. 2011;144(5):646–74.
Sturm G, Finotello F, Petitprez F, Zhang JD, Baumbach J, Fridman WH, List M, Aneichyk T. Comprehensive evaluation of transcriptome-based cell-type quantification methods for immuno-oncology. Bioinformatics. 2019;35(14):i436–45.
Finotello F, Trajanoski Z. Quantifying tumor-infiltrating immune cells from transcriptomics data. Cancer Immunol Immunother. 2018;67(7):1031–40.
Hong M, Tao S, Zhang L, Diao LT, Huang X, Huang S, Xie SJ, Xiao ZD, Zhang H. RNA sequencing: new technologies and applications in cancer research. J Hematol Oncol. 2020;13(1):166.
Liu H, Zhang M, Zhang T, Shi M, Lu W, Yang S, Cui Q, Li Z. Identification of a ferroptosis-related lncRNA signature with prognosis for Wilms tumor. Transl Pediatr. 2021;10(10):2418–31.
He L, Wang X, Jin Y, Xu W, Lyu J, Guan Y, Wu J, Han S, Liu G. A Prognostic Nomogram for Predicting Overall Survival in Pediatric Wilms Tumor Based on an Autophagy-related Gene Signature. Combinatorial Chemistry & High Throughput Screening. 2022;25(8):1385–97.
Gadd S, Huff V, Walz AL, Ooms AHAG, Armstrong AE, Gerhard DS, Smith MA, Auvil JMG, Meerzaman D, Chen Q-R, et al. A Children’s oncology group and TARGET initiative exploring the genetic landscape of Wilms tumor. Nat Genet. 2017;49(10):1487–94.
Li B, Dewey CN. RSEM: accurate transcript quantification from RNA-Seq data with or without a reference genome. BMC Bioinformatics. 2011;12:323.
Ashburner M, Ball CA, Blake JA, Botstein D, Butler H, Cherry JM, Davis AP, Dolinski K, Dwight SS, Eppig JT, et al. Gene ontology: tool for the unification of biology. The Gene Ontology Consortium. Nat Genet. 2000;25(1):25–9.
Aleksander SA, Balhoff J, Carbon S, Cherry JM, Drabkin HJ, Ebert D, Feuermann M, Gaudet P, Harris NL, Hill DP, et al. The gene ontology knowledgebase in 2023. Genetics. 2023;224(1):iyad031.
Subramanian A, Tamayo P, Mootha VK, Mukherjee S, Ebert BL, Gillette MA, Paulovich A, Pomeroy SL, Golub TR, Lander ES, Mesirov JP. Gene set enrichment analysis: a knowledge-based approach for interpreting genome-wide expression profiles. Proc Natl Acad Sci USA. 2005;102(43):15545–50.
Liberzon A, Subramanian A, Pinchback R, Thorvaldsdóttir H, Tamayo P, Mesirov JP. Molecular signatures database (MSigDB) 3.0. Bioinformatics (Oxford, England). 2011;27(12):1739–40.
Stelzer G, Rosen N, Plaschkes I, Zimmerman S, Twik M, Fishilevich S, Stein TI, Nudel R, Lieder I, Mazor Y, et al. The GeneCards suite: from gene data mining to disease genome sequence analyses. Curr Protoc Bioinformatics. 2016;54:1.30.1-1.30.33.
Friedman J, Hastie T, Tibshirani R. Regularization paths for generalized linear models via coordinate descent. J Stat Softw. 2010;33(1):1–22.
Yoshihara K, Kim H, Verhaak RG. estimate: estimate of stromal and immune cells in malignant tumor tissues from expression data. 2016.
Hänzelmann S, Castelo R, Guinney J. GSVA: gene set variation analysis for microarray and RNA-seq data. BMC Bioinformatics. 2013;14:7.
Aran D. Cell-type enrichment analysis of bulk transcriptomes using xCell. Methods Mol Biol. 2020;2120:263–76.
Becht E, Giraldo NA, Lacroix L, Buttard B, Elarouci N, Petitprez F, Selves J, Laurent-Puig P, Sautès-Fridman C, Fridman WH, de Reyniès A. Estimating the population abundance of tissue-infiltrating immune and stromal cell populations using gene expression. Genome Biol. 2016;17(1):218.
Finotello F, Mayer C, Plattner C, Laschober G, Rieder D, Hackl H, Krogsdam A, Loncova Z, Posch W, Wilflingseder D, et al. Molecular and pharmacological modulators of the tumor immune contexture revealed by deconvolution of RNA-seq data. Genome Med. 2019;11(1):34.
Ritchie ME, Phipson B, Wu D, Hu Y, Law CW, Shi W, Smyth GK. limma powers differential expression analyses for RNA-sequencing and microarray studies. Nucleic Acids Res. 2015;43(7):e47.
Benjamini Y, Hochberg Y. Controlling the false discovery rate: a practical and powerful approach to multiple testing. J Roy Stat Soc: Ser B (Methodol). 1995;57(1):289–300.
Yu G, Wang L-G, Han Y, He Q-Y. clusterProfiler: an R package for comparing biological themes among gene clusters. OMICS. 2012;16(5):284–7.
Hao Y, Stuart T, Kowalski MH, Choudhary S, Hoffman P, Hartman A, Srivastava A, Molla G, Madad S, Fernandez-Granda C, Satija R. Dictionary learning for integrative, multimodal and scalable single-cell analysis. Nat Biotechnol. 2024;42(2):293–304.
Young MD, Mitchell TJ, Vieira Braga FA, Tran MGB, Stewart BJ, Ferdinand JR, Collord G, Botting RA, Popescu DM, Loudon KW, et al. Single-cell transcriptomes from human kidneys reveal the cellular identity of renal tumors. Science. 2018;361(6402):594–9.
Zhang Y, Wang X. Targeting the Wnt/beta-catenin signaling pathway in cancer. J Hematol Oncol. 2020;13(1):165.
Xu X, Zhang M, Xu F, Jiang S. Wnt signaling in breast cancer: biological mechanisms, challenges and opportunities. Mol Cancer. 2020;19(1):165.
Sofeu Feugaing DD, Gotte M, Viola M. More than matrix: the multifaceted role of decorin in cancer. Eur J Cell Biol. 2013;92(1):1–11.
Parsons MJ, Tammela T, Dow LE. WNT as a driver and dependency in cancer. Cancer Discov. 2021;11(10):2413–29.
Derksen PWB, Keehnen RMJ, Evers LM, van Oers MHJ, Spaargaren M, Pals ST. Cell surface proteoglycan syndecan-1 mediates hepatocyte growth factor binding and promotes Met signaling in multiple myeloma. Blood. 2002;99(4):1405–10.
Bourguignon LY, Zhu H, Chu A, Iida N, Zhang L, Hung MC. Interaction between the adhesion receptor, CD44, and the oncogene product, p185HER2, promotes human ovarian tumor cell activation. J Biol Chem. 1997;272(44):27913–8.
Bourguignon LY, Gilad E, Rothman K, Peyrollier K. Hyaluronan-CD44 interaction with IQGAP1 promotes Cdc42 and ERK signaling, leading to actin binding, Elk-1/estrogen receptor transcriptional activation, and ovarian cancer progression. J Biol Chem. 2005;280(12):11961–72.
Dome JS, Fernandez CV, Mullen EA, Kalapurakal JA, Geller JI, Huff V, Gratias EJ, Dix DB, Ehrlich PF, Khanna G, et al. Children’s Oncology Group’s 2013 blueprint for research: renal tumors. Pediatr Blood Cancer. 2013;60(6):994–1000.
Zhou Y, Kong Y, Jiang M, Kuang L, Wan J, Liu S, Zhang Q, Yu K, Li N, Le A, Zhang Z. Curcumin activates NLRC4, AIM2, and IFI16 inflammasomes and induces pyroptosis by up-regulated ISG3 transcript factor in acute myeloid leukemia cell lines. Cancer Biol Ther. 2022;23(1):328–35.
Xia J, Chu C, Li W, Chen H, Xie W, Cheng R, Hu K, Li X. Mitochondrial protein UCP1 inhibits the malignant behaviors of triple-negative breast cancer through activation of mitophagy and pyroptosis. Int J Biol Sci. 2022;18(7):2949–61.
Shi Y, Cao H, Liu Z, Xi L, Dong C. Echinacoside induces mitochondria-mediated pyroptosis through Raf/MEK/ERK signaling in non-small cell lung cancer cells. J Immunol Res. 2022;2022:3351268.
Sala R, Rioja-Blanco E, Serna N, Sanchez-Garcia L, Alamo P, Alba-Castellon L, Casanova I, Lopez-Pousa A, Unzueta U, Cespedes MV, et al. GSDMD-dependent pyroptotic induction by a multivalent CXCR4-targeted nanotoxin blocks colorectal cancer metastases. Drug Deliv. 2022;29(1):1384–97.
Ma L, Bian M, Gao H, Zhou Z, Yi W. A novel 3-acyl isoquinolin-1(2H)-one induces G2 phase arrest, apoptosis and GSDME-dependent pyroptosis in breast cancer. PLoS ONE. 2022;17(5):e0268060.
Chui AJ, Griswold AR, Taabazuing CY, Orth EL, Gai K, Rao SD, Ball DP, Hsiao JC, Bachovchin DA. Activation of the CARD8 inflammasome requires a disordered region. Cell Rep. 2020;33(2):108264.
Shi J, Zhao Y, Wang K, Shi X, Wang Y, Huang H, Zhuang Y, Cai T, Wang F, Shao F. Cleavage of GSDMD by inflammatory caspases determines pyroptotic cell death. Nature. 2015;526(7575):660–5.
Kuang S, Zheng J, Yang H, Li S, Duan S, Shen Y, Ji C, Gan J, Xu XW, Li J. Structure insight of GSDMD reveals the basis of GSDMD autoinhibition in cell pyroptosis. Proc Natl Acad Sci U S A. 2017;114(40):10642–7.
Kayagaki N, Lee BL, Stowe IB, Kornfeld OS, O’Rourke K, Mirrashidi KM, Haley B, Watanabe C, Roose-Girma M, Modrusan Z, et al. IRF2 transcriptionally induces expression for pyroptosis. Sci Signal. 2019;12(582):eaax4917.
Cao Q, Feng D, He J, Zhou L, Fan Z, Chen Y, Chen X, Jin R, Zhou G. Involvement of TFAP2A in the activation of GSDMD gene promoter in hyperoxia-induced ALI. Exp Cell Res. 2021;401(1):112521.
Zeng J, Tao J, Xi L, Wang Z, Liu L. PCSK9 mediates the oxidative low-density lipoprotein-induced pyroptosis of vascular endothelial cells via the UQCRC1/ROS pathway. Int J Mol Med. 2021;47(4):53.
Wang X, Li X, Liu S, Brickell AN, Zhang J, Wu Z, Zhou S, Ding Z. PCSK9 regulates pyroptosis via mtDNA damage in chronic myocardial ischemia. Basic Res Cardiol. 2020;115(6):66.
Ko DC, Gamazon ER, Shukla KP, Pfuetzner RA, Whittington D, Holden TD, Brittnacher MJ, Fong C, Radey M, Ogohara C, et al. Functional genetic screen of human diversity reveals that a methionine salvage enzyme regulates inflammatory cell death. Proc Natl Acad Sci U S A. 2012;109(35):E2343-2352.
Kang W, Hong SH, Lee HM, Kim NY, Lim YC, Le le TM, Lim B, Kim HC, Kim TY, Ashida H, et al. Structural and biochemical basis for the inhibition of cell death by APIP, a methionine salvage enzyme. Proc Natl Acad Sci U S A. 2014;111(1):E54-61.
Suzuki S, Suzuki T, Mimuro H, Mizushima T, Sasakawa C. Shigella hijacks the glomulin-cIAPs-inflammasome axis to promote inflammation. EMBO Rep. 2018;19(1):89–101.
Suzuki S, Mimuro H, Kim M, Ogawa M, Ashida H, Toyotome T, Franchi L, Suzuki M, Sanada T, Suzuki T, et al. Shigella IpaH7.8 E3 ubiquitin ligase targets glomulin and activates inflammasomes to demolish macrophages. Proc Natl Acad Sci U S A. 2014;111(40):E4254-4263.
Duncan JA, Canna SW. The NLRC4 inflammasome. Immunol Rev. 2018;281(1):115–23.
Shen C, Lu A, Xie WJ, Ruan J, Negro R, Egelman EH, Fu TM, Wu H. Molecular mechanism for NLRP6 inflammasome assembly and activation. Proc Natl Acad Sci U S A. 2019;116(6):2052–7.
Wu P, Chen J, Chen J, Tao J, Wu S, Xu G, Wang Z, Wei D, Yin W. Trimethylamine N-oxide promotes apoE(-/-) mice atherosclerosis by inducing vascular endothelial cell pyroptosis via the SDHB/ROS pathway. J Cell Physiol. 2020;235(10):6582–91.
Zhou B, Zhang JY, Liu XS, Chen HZ, Ai YL, Cheng K, Sun RY, Zhou D, Han J, Wu Q. Tom20 senses iron-activated ROS signaling to promote melanoma cell pyroptosis. Cell Res. 2018;28(12):1171–85.
Karimpour S, Davoodi J, Ghahremani MH. Integrity of ATP binding site is essential for effective inhibition of the intrinsic apoptosis pathway by NAIP. Biochem Biophys Res Commun. 2011;407(1):158–62.
Dziarmaga A, Hueber PA, Iglesias D, Hache N, Jeffs A, Gendron N, Mackenzie A, Eccles M, Goodyer P. Neuronal apoptosis inhibitory protein is expressed in developing kidney and is regulated by PAX2. Am J Physiol Renal Physiol. 2006;291(4):F913-920.
Wang Q, Wang C, Chen J. NLRP6, decreased in gastric cancer, suppresses tumorigenicity of gastric cancer cells. Cancer Manag Res. 2018;10:6431–44.
Gao X, Wang W-X, Zhang X-L. A novel pyroptosis risk model composed of NLRP6 effectively predicts the prognosis of hepatocellular carcinoma patients. Cancer Med. 2023;12(1):808–23.
Chen GY, Liu M, Wang F, Bertin J, Núñez G. A functional role for Nlrp6 in intestinal inflammation and tumorigenesis. J Immunol. 2011;186(12):7187–94.
Yu Y, Cao F, Xiong Y, Zhou H. SP1 transcriptionally activates NLRP6 inflammasome and induces immune evasion and radioresistance in glioma cells. Int Immunopharmacol. 2021;98:107858.
Du Z, Zhou X, Lai Y, Xu J, Zhang Y, Zhou S, Feng Z, Yu L, Tang Y, Wang W, et al. Structure of the human respiratory complex II. Proc Natl Acad Sci USA. 2023;120(18):e2216713120.
Li J, Han X, Sun M, Li W, Yang G, Chen H, Guo B, Li J, Li X, Wang H. Caspase-9 inhibition triggers Hsp90-based chemotherapy-mediated tumor intrinsic innate sensing and enhances antitumor immunity. J Immunother Cancer. 2023;11(12):e007625.
Wu G, Nomoto S, Hoque MO, Dracheva T, Osada M, Lee C-CR, Dong SM, Guo Z, Benoit N, Cohen Y, et al. DeltaNp63alpha and TAp63alpha regulate transcription of genes with distinct biological functions in cancer and development. Cancer Res. 2003;63(10):2351–7.
Kordaß T, Weber CEM, Eisel D, Pane AA, Osen W, Eichmüller SB. miR-193b and miR-30c-1 inhibit, whereas miR-576-5p enhances melanoma cell invasion. Oncotarget. 2018;9(65):32507–22.
Chen W, Yao G, Zhou K. miR-103a-2-5p/miR-30c-1-3p inhibits the progression of prostate cancer resistance to androgen ablation therapy via targeting androgen receptor variant 7. J Cell Biochem. 2019;120(8):14055–64.
Zhang J, Lu Q, Pang H, Zhang M, Wei W. MiR-103a-3p aggravates renal cell carcinoma by targeting TMEM33. Am J Transl Res. 2021;13(11):12694–703.
Sun Z, Zhang Q, Yuan W, Li X, Chen C, Guo Y, Shao B, Dang Q, Zhou Q, Wang Q, et al. MiR-103a-3p promotes tumour glycolysis in colorectal cancer via hippo/YAP1/HIF1A axis. J Exp Clin Cancer Res. 2020;39(1):250.
Ge J, Mao L, Xu W, Fang W, Wang N, Ye D, Dong Z, Guan H, Guan C. miR-103a-3p suppresses cell proliferation and invasion by targeting tumor protein D52 in prostate cancer. J Invest Surg. 2021;34(9):984–92.
Wculek SK, Cueto FJ, Mujal AM, Melero I, Krummel MF, Sancho D. Dendritic cells in cancer immunology and immunotherapy. Nat Rev Immunol. 2020;20(1):7–24.
Yuen GJ, Demissie E, Pillai S. B lymphocytes and cancer: a love-hate relationship. Trends Cancer. 2016;2(12):747–57.
van der Leun AM, Thommen DS, Schumacher TN. CD8(+) T cell states in human cancer: insights from single-cell analysis. Nat Rev Cancer. 2020;20(4):218–32.
Raskov H, Orhan A, Christensen JP, Gogenur I. Cytotoxic CD8(+) T cells in cancer and cancer immunotherapy. Br J Cancer. 2021;124(2):359–67.
Barnes TA, Amir E. HYPE or HOPE: the prognostic value of infiltrating immune cells in cancer. Br J Cancer. 2017;117(4):451–60.
Wu K, Lin K, Li X, Yuan X, Xu P, Ni P, Xu D. Redefining tumor-associated macrophage subpopulations and functions in the tumor microenvironment. Front Immunol. 2020;11:1731.
Chen Y, Zhang S, Wang Q, Zhang X. Tumor-recruited M2 macrophages promote gastric and breast cancer metastasis via M2 macrophage-secreted CHI3L1 protein. J Hematol Oncol. 2017;10(1):36.
Zhang X, Feng H, Li Z, Li D, Liu S, Huang H, Li M. Application of weighted gene co-expression network analysis to identify key modules and hub genes in oral squamous cell carcinoma tumorigenesis. Onco Targets Ther. 2018;11:6001–21.
Acknowledgements
We are grateful to Xena datasets, TARGET kidney tumor projects, and GEO database for their diligent work.
Funding
This study was supported by the Key Research and Development Plan of Heilongjiang Province (no. 2022ZX06C08), and the National Natural Science Foundation of China (no. 81871837).
Author information
Authors and Affiliations
Contributions
Conception and design: Yujun Guo, Wenjun Lu, Zhaozhu Li Administrative support: Zhaozhu Li, Qingbo Cui, Shulong Yang Provision of study materials or patients: Ze’nan Zhang, Hengchen Liu, Aodan Zhang Collection and assembly of data: Tingting Zhang, Yang Wu, Xiangqi Li Data analysis and interpretation: Yujun Guo, Wenjun Lu Manuscript writing: All authors Final approval of manuscript: All authors.
Corresponding authors
Ethics declarations
Ethics approval and consent to participate
All datasets were obtained from online public databases, therefore, no additional ethics approval and consent to participate is needed.
Consent for publication
Not applicable.
Competing interests
The authors declare no competing interests.
Additional information
Publisher’s Note
Springer Nature remains neutral with regard to jurisdictional claims in published maps and institutional affiliations.
Supplementary Information
Additional file 1: Figure S1.
(A) The estimation of Immune Scores of tumors. (B) The correlation heatmap of immune infiltrating cells by quanTIseq algorithm. (C-D) The distribution of signature genes in each cluster in the samples of favorable histology and anaplastic histology, respectively. tSNE, t-distributed stochastic neighbor embedding.
Additional file 2: Table S1.
The list of PRGs from three online databases. Table S2. The prognostic capacities of PRGs by univariate Cox regression. Table S3. The prognostic capacities of risk score and clinical variables by univariate Cox regression. Table S4. The prognostic capacities of risk score and clinical variables by multivariate Cox regression.
Rights and permissions
Open Access This article is licensed under a Creative Commons Attribution 4.0 International License, which permits use, sharing, adaptation, distribution and reproduction in any medium or format, as long as you give appropriate credit to the original author(s) and the source, provide a link to the Creative Commons licence, and indicate if changes were made. The images or other third party material in this article are included in the article's Creative Commons licence, unless indicated otherwise in a credit line to the material. If material is not included in the article's Creative Commons licence and your intended use is not permitted by statutory regulation or exceeds the permitted use, you will need to obtain permission directly from the copyright holder. To view a copy of this licence, visit http://creativecommons.org/licenses/by/4.0/. The Creative Commons Public Domain Dedication waiver (http://creativecommons.org/publicdomain/zero/1.0/) applies to the data made available in this article, unless otherwise stated in a credit line to the data.
About this article
Cite this article
Guo, Y., Lu, W., Zhang, Z. et al. A novel pyroptosis-related gene signature exhibits distinct immune cells infiltration landscape in Wilms’ tumor. BMC Pediatr 24, 279 (2024). https://doi.org/10.1186/s12887-024-04731-0
Received:
Accepted:
Published:
DOI: https://doi.org/10.1186/s12887-024-04731-0