Abstract
Background
Expression quantitative trait loci (eQTL) studies aim to understand the influence of genetic variants on gene expression. The colocalization of eQTL mapping and GWAS strategy could help identify essential candidate genes and causal DNA variants vital to complex traits in human and many farm animals. However, eQTL mapping has not been conducted in ducks. It is desirable to know whether eQTLs within GWAS signals contributed to duck economic traits.
Results
In this study, we conducted an eQTL analysis using publicly available RNA sequencing data from 820 samples, focusing on liver, muscle, blood, adipose, ovary, spleen, and lung tissues. We identified 113,374 cis-eQTLs for 12,266 genes, a substantial fraction 39.1% of which were discovered in at least two tissues. The cis-eQTLs of blood were less conserved across tissues, while cis-eQTLs from any tissue exhibit a strong sharing pattern to liver tissue. Colocalization between cis-eQTLs and genome-wide association studies (GWAS) of 50 traits uncovered new associations between gene expression and potential loci influencing growth and carcass traits. SRSF4, GSS, and IGF2BP1 in liver, NDUFC2 in muscle, ELF3 in adipose, and RUNDC1 in blood could serve as the candidate genes for duck growth and carcass traits.
Conclusions
Our findings highlight substantial differences in genetic regulation of gene expression across duck primary tissues, shedding light on potential mechanisms through which candidate genes may impact growth and carcass traits. Furthermore, this availability of eQTL data offers a valuable resource for deciphering further genetic association signals that may arise from ongoing extensive endeavors aimed at enhancing duck production traits.
Similar content being viewed by others
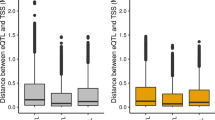
Background
Ducks are frequently raised as poultry for the consumption of their meat and eggs by humans. Duck meat is commonly acknowledged for its rich flavor, high amino acid and polyunsaturated fatty acid content, and comparatively low-fat levels [1, 2]. Breeders consider traits related to growth and body weight composition to be the most critical in broiler ducks [3]. Meat production and growth traits are influenced by numerous polygenic QTLs, with each QTL making a small contribution to the trait [4, 5]. To overcome the challenge, genome-wide associated studies (GWAS) have been widely used to identify genetic loci affecting complex traits such as growth rate and meat production. Currently, several candidate loci associated with economic traits have been identified [6, 7]. For example, the variants of IGF2BP1 determined the variation in body size and feed efficiency [8]. However, how to mechanistically decipher these candidate loci contributing to agronomic traits in ducks is a challenge. Nowadays, with the development of molecular phenotypic measurements, we can elucidate the genetic architecture of complex traits using these molecular phenotypes, such as gene expression information [9, 10].
Analyzing expression quantitative trait loci (eQTLs) is the most effective approach for evaluating how sequence variants influence gene expression within their native genomic and cellular environments [11]. This approach has been extensively documented in the series of Genotype-Tissue Expression (GTEx) project [12,13,14] in human. Currently, several eQTL studies have been conducted on farm animals, including cattle [15], pigs [16], sheep [17], and chicken [18]. For example, they observed that the production traits related to eQTLs also affect the expression of MGST1 [19] and SLC37A1 [20] in dairy cattle. However, eQTL analysis has not been conducted in ducks. Therefore, it is desirable to know whether eQTLs within GWAS signals contributed to duck economic traits.
In this study, we hypothesized that the colocalization of eQTL mapping and GWAS strategy could help identify essential candidate genes and causal DNA variants vital to growth and carcass traits. We integrated GWAS results of duck carcass and growth traits with the transcriptomes from seven tissues to prioritize genes and variants that influence duck economic traits through transcriptome. This work provided a duck cis-eQTL catalog consisting of 820 samples from seven tissues. The study results provide valuable resources for understanding the genetic effects on the transcriptome and also suggest the underlying molecular mechanisms of potentially causal functional variants in duck economic traits.
Methods
RNA-seq analysis
We downloaded 820 RNA-Seq datasets from Sequence Read Archive (SRA; https://www.ncbi.nlm.nih.gov/sra) and BIGD (https://bigd.big.ac.cn/bioproject/; Table S1). The adaptor and low-quality reads were trimmed and adaptor by Trimmomatic v0.39 [21]. The clean reads were mapped to the duck reference genome (ZJU1.0, https://www.ncbi.nlm.nih.gov/datasets/genome/GCF_015476345.1/) using STAR aligner [22]. To quantify gene expression, we used the mapped reads to calculate the gene expression levels using transcript per million (TPM) by StringTie [23] based on the annotation of RefSeq (GCF_015476345.1). A gene was considered expressed if it had a TPM threshold of ≥ 0.1 in at least 20% of the samples [15].
Genotype imputation
PCR duplicates of STAR alignments were marked using MarkDuplicates in Picard (http://broadinstitute.github.io/picard/). We splited reads into exon segments and trimmed any sequences overhanging into the intronic regions using SplitNCigarReads modules of GATK v4.2.6.1 [24]. Then, we recalibrated base quality scores based on known genomic variants using BaseRecalibrator and ApplyBQSR modules of GATK. Then, we carried out joint-calling of all GVCF samples using the GenotypeGVCFs module of the GATK tool. The low-quality variants were filtered out using –filter-expression “FS > 30.0 & QD < 2.0”. We also check the variants consistency of different tissues from the same individual in PRJNA419583 study. The average of consistency was 99.6% (Table S2). The variants were imputed to DNA sequence variants level based on a multiple-breed reference panel consisting of 2215 Pekin ducks and 289 public individuals from Shaoxing ducks (n = 166) and wide mallards (n = 123) by Beagle 5.4 [25]. We removed variants with dosage R-squared (DR2) of less than 0.8, genotype call rates of less than 90%, minor allele frequency (MAF) of less than 0.05 or variants located outside of autosomes using PLINK v1.90 [26]. On average, we retained 1,112,867 autosomal variants with average DR2 of 0.92 for eQTL mapping.
Cis-eQTL mapping
Gene expression values were normalized across samples using the inverse normal transformation. To account for population effects, we incorporated principal components (PCs) of genotype into the eQTL analyses. The number of PCs selected for the analysis was determined by testing their significance using EIGENSTRAT v6.1.4 (https://alkesgroup.broadinstitute.org/EIGENSOFT/). We ultimately decided to use the first three PCs for the eQTL analysis, as the test was not significant (P-value = 0.061) when more than three PCs were included (Table S3). Additionally, in order to address hidden batch effects and other sources of technical or biological variation, we estimated latent covariates for gene expression levels in each tissue using the Probabilistic Estimation of Expression Residuals (PEER) [27]. We retained 15 PEER confounding variables because the posterior variances of factor weights were nearly at their minimum values (Fig. S1). For blood tissue, we also conducted cis-eQTLs using 20 PEER confounding variables (https://github.com/WentaoCai/Duck_eQTL_results), we identified 1029 eGenes with 20 peer variables compared to 1040 eGenes using 15 peer variables. The number of overlapped eGenes was 952 (Fig. S2). We defined potential cis-eQTLs as variants located within a 1 Mb proximity ups or downstream of the transcription start sites (TSSs) of genes [28]. The cis-eQTLs mapping was conducted using a nominal P-value threshold that corresponded to FDR ≤ 0.05 for each gene by fastQTL [29]. The scripts of data analysis can be accessed on https://wentaocai.github.io/eQTL-analysis/. The gene lists were subjected to GO and KEGG analyses using DAVID with a significance threshold set at a P-value ≤ 0.05 [30]. We estimated the replication rate of cis-eQTL across different tissues using π1 statistic of the qvalue R package [31].
GWAS and Colocalization
The phenotype of 50 growth and carcass traits were collected from 941 Pekin XMallard F2 individuals. The summary of phenotype records can be found in Table S4. The genotypes were conducted using resequencing technology on Illumina HiSeq X Ten, which was described in our previous study [8]. After mapping, call SNPs, and quality control, 13,064,619 variants for 941 individuals were obtained for GWAS. The phenotype was adjusted for covariates, including sex, feed room, and the first three PCs of genotypes. We used a linear mixed model for the association test of each SNP using the GCTA -mlma [32].
where y is adjusted phenotype; X is a vector of genotypes of a variant at the locus tested; β is the effect size of the variant; u is a vector of random polygenic effects ~ N (0, \({{\varvec{G}}{\varvec{\sigma}}}_{{\varvec{g}}}^{2}\)), where G is genomic relationship matrix constructed from all variants; ε is a vector of residual errors.
We conducted the colocalization analysis of GWAS variants and eQTLs using Coloc R package [33]. The variants with P-value < 5 × 10−5 and their neighboring ± 50 Kb extracted from GWAS summaries of 50 growth and carcass traits were obtained for colocalization analysis [34]. GWAS signals and eQTLs were considered colocalizing if a posterior probability (H4) of the shared signal was > 0.4 [35].
Results
Transcriptome profile of duck primary tissues
We obtained 22.5 billion clean reads from a total of 820 publicly available RNA-seq samples, including seven tissues (251 liver, 184 muscle, 120 blood, 71 adipose, 87 ovary, 66 spleen, and 41 lung) from 17 breeds (Table S1). Pekin ducks accounted for the largest proportion of samples (36.2%), indicative of their extensive economic significance worldwide. Approximately 88.9% of the total reads were successfully mapped to the reference genome (Table S1). Under the expression threshold of TPM ≥ 0.1 in at least 20% of the samples, 16,485 (65.7% of total genes) genes were expressed across tissues. Using PCA analysis, we can successfully distinguish samples according to different tissues and reconstruct the relationships between these tissues based on their expression levels (Fig. 1A). The expression profiles accurately reflected tissue types (Fig. 1B), which reaffirmed the high quality of these profiles and underscores their suitability for our subsequent analysis. We called variants from RNA-Seq samples and imputed each genotype using a reference population of 2,504 ducks. When applying PCA clustering to imputed genotypes, as anticipated, the samples exhibited clustering patterns corresponding to their respective breeds (Fig. 1C).
Principal component analysis (PCA) and hierarchical clustering of samples. A Sample clustering (n = 820) using PCA based on gene expression levels. B Hierarchical clustering of 820 samples using gene expression levels, sample clustering is affected by both tissues and breeds. C PCA of samples (n = 820) based on imputed genotypes
Genetic effects on gene expression
Considering all tissues, our analysis detected a total of 113,374 cis-eQTLs associated with 12,266 genes. These genes represent approximately 54.8% of all autosomally expressed genes. The numbers of eGene (gene with significant cis-eQTLs) for liver, muscle, blood, ovary, adipose, spleen, and lung were 7,301, 5,706, 1,040, 2,390, 989, 368, and 775, respectively (Table 1). The most notable cis-eQTLs detected in the liver were found to influence the expression of TIMMDC1, a gene crucial for the assembly of the membrane arm of mitochondrial Complex I (Fig. 2A). The most significant cis-eQTLs of muscle affected expression of PHOSPHO2, involved in dephosphorylation (Fig. 2B). The Manhattan plots of cis-eQTLs for other tissues are shown in Figs. S3, S4, S5, S6 and S7. The cis-eQTL results of seven tissues are accessed on https://github.com/WentaoCai/Duck_eQTL_results.
Manhattan plot of cis-eQTLs. A The Manhattan plot illustrates the nominal P-value (y-axis) for all cis-eQTLs in liver (left). Points colored dark red or pink represents significant cis-eQTLs, whereas those in black or gray were SNPs that did not reach a significant threshold. The expression of TIMMDC1 with three genotypes in liver (right). B The Manhattan plot shows the nominal P-value (y-axis) for all cis-eQTLs in muscle (left). The expression of PHOSPHO2 with three genotypes in muscle (right)
Character of cis-eQTLs
Consistent with previous work in humans [36], most of the significant duck cis-eQTLs clustered around the TSS of target genes (Fig. 3A). An average of 45.3% of significant cis-eQTLs fell within 100 kb around the TSS. We found an enrichment of low P values closer to TSSs, showing that cis-eQTLs are more likely to be located within this distance (Fig. 3B and Fig. S8). Cis-eGenes of most tissues exhibited significantly higher expression levels compared to non-eGenes (Wilcoxon test, Bonferroni adjusted P-value < 0.05), with the exception of lung and spleen tissue (Fig. 3C). To assess the sharing patterns of cis-eQTLs between tissues, we computed the π1 statistics for each pair of tissues. We observed that cis-eQTLs in blood exhibited lower conservation across different tissues., while cis-eQTLs from any tissue exhibited a strong sharing pattern to liver tissue (Fig. 3D). We detected that any two tissues shared 39.1% of eGenes (Fig. 3E), and 10.7% of eVariants (Fig. S9). The 244 eGenes shared by at least four tissues were involved in basic metabolic pathways, such as nucleoplasm, mitochondrion, cytosol, and ATP binding (Table S5). Interestingly, we detected that the expression of THNSL1, SF3B6, PPIL3, PHOSPHO2, LOC119713243, and LOC119717646 were affected by cis-eQTLs in almost all tissues except for spleen. On average, 10.7% of cis-eQTLs were involved in at least two eGenes.
The characters of cis-eQTLs. A The density of top significant cis-eQTLs for each tested gene around TSS. B The P-value distribution of top significant cis-eQTLs for each tested gene in liver. C The expression of eGenes of cis-eQTL compared to non-eGenes. The significant P-value were calculated using Wilcoxon test. D Pairwise sharing patterns of cis-eQTL (π1 value) across tissues. E The overlaps of eGenes across tissues
Colocalization of cis-eQTLs and GWAS loci
We conducted GWAS between genomic variants and 50 growth and carcass traits in F2 ducks (Table S4). We identified a total of 2,136 unique variants that showed significant association (P < 5 × 10–8) with 47 agronomic traits, of which, 1,039 variants were associated with at least two traits (Table S6). We obtained the variants with P-value < 5 × 10−5 and their neighboring ± 50 Kb for colocalization analysis. We observed that the eQTLs for 49 eGenes in seven tissues were colocalized with 41 traits, resulting in a total of 94 tissue-gene-trait pairs (Fig. 4, Table 2, Fig. S10, and Table S5). In liver, we detected that eQTLs of SRSF4 (serine and arginine rich splicing factor 4) were colocalized with GWAS signals of jejunum length and total intestine length (Fig. 5A). The top GWAS signal of jejunum length significantly affecting the expression of SRSF4. The eQTLs of GSS (glutathione synthetase) were colocalized with GWAS signals of shank length (Fig. 5B). There was a strong LD (r2 = 1) between the top eQTLs signal and the top GWAS signal. The top GWAS signal of shank length is also significantly associated with the expression of GSS. Previously, we detected the variants located IGF2BP1 gene affecting the carcass traits and body size [8]. Here, we confirmed these GWAS signals can affect the phenotype of eviscerated weight, heart weight, neck length, and skeleton weight through the change of IGF2BP1 expression (Fig. 5C and Table S7).
The colocalized results between cis-eQTLs and GWAS signals. A Manhattan plot illustrates the colocalization results (H4 > 0.4) between liver eQTLs and GWAS signals. The x-axis represents the P-value of lead eQTLs (points) across traits in liver. The right labels are colocalized SNP-gene-trait pairs. The different categories of tissues are distinguished by colors (B) Manhattan plot illustrates the colocalization results (H4 > 0.4) between muscle cis-eQTLs and GWAS signals
Examples of colocalized results between eQTLs and GWAS signals. A The colocalization between jejunum length GWAS and SRSF4 eQTLs of liver. The colors of the variants are determined based on their linkage disequilibrium values with the most significant variant. B The colocalization between shank length GWAS and GSS eQTLs of liver. C The colocalization between eviscerated weight GWAS and IGFBP1 eQTLs of liver. D The colocalization between BW28 (body weight at 28 days) GWAS and NDUFC2 eQTLs of muscle
In muscle tissue, the eQTLs of NDUFC2 (NADH: ubiquinone oxidoreductase subunit C2) were colocalized with BW28 (body weight at 28 days; Fig. 5D). The top significant GWAS signals for BW28 and the top significant eQTLs of NDUFC2 were the same. It appeared that there were two independent loci in eQTL signals of liver GSS and muscle NDUFC2 (Fig. 5B and D). To confirm whether the eQTL signals were derived from the same causative variant, we repeated cis-eQTL mapping using the top eQTL signal of GSS or NDUFC2 as a covariate. No significant signals were detected using this conditional analysis (Fig. S11), indicating the eQTL signals of liver GSS and muscle NDUFC2 arise from one causative variant. The eQTLs of LOC113840004 were colocalized with 20 traits, implying this uncharacterized gene would be an important functional gene in ducks. In other tissues, we observed the eQTLs of EIF3 (E74 like ETS transcription factor 3) in adipose were associated with the GWAS of BW56 body weight at 56 days (Table S7). The eQTLs of RUNDC1 (RUN domain containing) in blood were detected colocalized with the GWAS of ileum length. The eQTLs of IPPK (inositol-pentakisphosphate 2-kinase) in ovary were detected colocalized with the GWAS of neck weight percentage. Overall, the colocalization analysis enhanced our ability to identify potentially causal genes and gain a deeper understanding of the genetic underpinnings of complex traits in ducks.
Discussion
In this study, we created a comprehensive catalog of eQTLs across multiple tissues, further expanding the list of candidate genes and potential variants affecting important agronomic traits in ducks.
Consistent with other species [36, 37], a high density of signals was observed near the TSSs of their respective genes. The majority of eQTLs appear to be tissue-specific, suggesting intricate and distinct genetic mechanisms governing gene expression across different tissues. The functional annotation of tissue-shared eGenes revealed that genetic variants more prominently and frequently impact genes related to immune and metabolic functions, which is consistent with previous findings in human [38] and cattle [37]. Similar to cattle [37], these cis-eGenes in duck were found to exhibit higher expression levels than non-eGenes in most tissues.
The colocalization analysis between GWAS signals and cis-eQTLs in the seven tissues facilitates the identification of causal genes for association signals that were previously unresolved. Several GWAS signals were observed to colocalize with cis-eQTLs. SRSF4 belongs to the family of Arginine-Serine-rich (SR) proteins, which play a crucial role in constitutive splicing and also regulate alternative splicing [39]. Like other SRSFs, SRSF4 shuttles between the nucleus and cytoplasm, and mediate mRNA regulation, including export, stability, and translation [40, 41]. SRSF4 is associated with human colon adenocarcinomas [42]. SRSF4 has a relatively high expression in the liver, stomach, and intestine [43]. The upstream variants of SRSF4 have significantly regulated the expression of SRSF4 in the liver for both cattle [15] and pigs [16]. GSS is responsible for catalyzing the condensation of gamma-glutamylcysteine and glycine to produce glutathione (GSH) [44]. GSH is essential for a multitude of processes, such as safeguarding cells from oxidative damage, facilitating amino acid transport, detoxifying foreign compounds, preserving protein sulfhydryl groups in a reduced state, and serving as a cofactor for several enzymes [45]. The mutations of GSS are associated with body height in humans [46, 47]. The length of the shank directly determines a poultry's height. The top GWAS signal of shank length is also significantly associated with the expression of GSS, which implies that GSS could be a candidate gene for the shank length and body height of the duck. IGF2BP1 belongs to the insulin-like growth factor 2 mRNA-binding protein family and plays a role in RNA transport, cell proliferation, differentiation, and metabolism by regulating the mRNA localization, stability, and translation of specific target genes. [48, 49]. IGF2BP1 is a well-known candidate gene for body size in ducks [8], chickens [50], and humans [51]. Here, we confirmed the variants of IGF2BP1 can regulate the duck body size by changing the IGF2BP1 expression in liver.
NDUFC2 is a subunit of the mitochondrial membrane respiratory chain NADH dehydrogenase (Complex I) [52]. A deficiency in NDUFC2 results in cellular-level mitochondrial dysfunction and increased oxidative stress [53]. NDUFC2 appears to be downregulated in the skeletal muscle cells of individuals with insulin resistance and is linked to insulin secretion in vivo [54, 55]. The mutations of NDUFC2 are associated with body mass index [56]. Both BW28 GWAS and NDUFC2 eQTLs shared the same of top significant variant, implying NDUFC2 may act as a candidate gene for duck body weight. ELF3, an important member of the E74-like transcription factor family, involves in inflammatory response [57] and adipogenic differentiation [58]. Leptin is a potent pro-inflammatory and pro-catabolic factor, and its downstream actions are mediated by ELF3 [59]. The mutations near ELF3 were associated with body mass index in humans [60]. The eQTLs of ELF3 in adipose were associated with BW56. RUNDC1 is an inhibitor of the tumor suppressor p53 [61], which can negatively modulate autophagy by blocking fusion between autophagosomes and lysosomes [62]. The eQTLs of RUNDC1 in blood were associated with ileum length. We can hypothesize that RUNDC1 is a candidate gene for ileum length. These results are crucial for gaining a deeper understanding of the molecular mechanisms underlying specific traits by considering gene expression and the functional characteristics of genes associated with these traits.
Although our study released thousands of eGenes by analyzing public RNA-seq data, there are still some limitations. For example, some tissues have limited sample sizes, resulting in the detection of a small number of eGenes in several tissues, such as spleen, lung, and adipose. The potential biases in variant detection, population stratification resulting from the inclusion of data from multiple breeds, and confounding factors stemming from diverse experimental designs may not have been completely resolved when using publicly available RNA-seq data. In addition, the variety and sample size of our multiple-breed reference panel for genotype imputation remain limited, which may introduce biases in the genotype results. This study serves as a pioneering investigation into the field of duck eQTLs. The summary statistics of duck eQTLs are still unavailable, which impedes a more detailed investigation of colocalized signals and thus an evaluation of possible pleiotropic effects. Our analysis of GWAS signals within the context of duck eQTLs serves as a preliminary foundation for future investigations in this field.
Conclusions
The interpretation of genetic mechanisms underlying complex traits based on the molecular phenotype of primary tissues in ducks was rare. Through the integration of eQTL and GWAS data, we have constructed a molecular QTL map in ducks, which aids in unraveling genetic association signals by identifying candidate genes, such as SRSF4, GSS, IGF2BP1, NDUFC2, ELF3, and RUNDC1. Moreover, these newly identified cis-eQTLs and candidate genes will enhance the accuracy of genomic prediction and contribute to the benefit of genetic improvement programs in duck breeding.
Availability of data and materials
All eQTL results are available on GitHub with the identifier https://github.com/WentaoCai/Duck_eQTL_results. All the genotype data of duck F2 population have been deposited in the Sequence Read Archive (https://www.ncbi.nlm.nih.gov/sra) with the accession numbers PRJNA471401 and PRJNA450892. Other results were provided in the Additional file. The scripts used for data processing and analyses are available on the website through the following link, https://wentaocai.github.io/eQTL-analysis/.
Abbreviations
- eQTLs:
-
Expression quantitative trait loci
- FDR:
-
False discovery rate
- GO:
-
Gene Ontology
- GTEx:
-
The Genotype-Tissue Expression
- GWAS:
-
Genome-wide associated studies
- KEGG:
-
Kyoto Encyclopedia of Genes and Genomes
- LD:
-
Linkage disequilibrium
- PCs:
-
Principal components
- PCA:
-
Principal component analysis
- PEER:
-
Probabilistic estimation of expression residuals
- SNPs:
-
Single nucleotide polymorphisms
- TPM:
-
Transcripts per million
- TSS:
-
Transcription start site
References
OnbaŞIlar EE, YalÇIn S. Fattening performance and meat quality of Pekin ducks under different rearing systems. Worlds Poult Sci J. 2018;74(1):61–8. https://doi.org/10.1017/S004393391700099X.
Shin D-M, Kim YJ, Choi Y-S, Kim B-K, Han SG. Duck fat: Physicochemical characteristics, health effects, and food utilizations. LWT. 2023;188:115435. https://doi.org/10.1016/j.lwt.2023.115435.
Szwaczkowski T, Grzech M, Borowska A, Wencek E, Wolc A. Maternal genetic effects on body weight and breast morphological traits in duck population under selection. Arch Anim Breed. 2010;53(5):600–8. https://doi.org/10.5194/aab-53-600-2010.
Andersson L, Georges M. Domestic-animal genomics: deciphering the genetics of complex traits. Nat Rev Genet. 2004;5(3):202–12. https://doi.org/10.1038/nrg1294.
Gao Y, Zhang R, Hu X, Li N. Application of genomic technologies to the improvement of meat quality of farm animals. Meat Sci. 2007;77(1):36–45. https://doi.org/10.1016/j.meatsci.2007.03.026.
Xi Y, Wu Q, Zeng Y, Qi J, Li J, He H, et al. Identification of the genetic basis of the duck growth rate in multiple growth stages using genome-wide association analysis. BMC Genomics. 2023;24(1):285. https://doi.org/10.1186/s12864-023-09302-8.
Hu ZL, Park CA, Reecy JM. Building a livestock genetic and genomic information knowledgebase through integrative developments of Animal QTLdb and CorrDB. Nucleic Acids Res. 2019;47(D1):D701–10. https://doi.org/10.1093/nar/gky1084.
Zhou Z, Li M, Cheng H, Fan W, Yuan Z, Gao Q, et al. An intercross population study reveals genes associated with body size and plumage color in ducks. Nat Commun. 2018;9(1):2648. https://doi.org/10.1038/s41467-018-04868-4.
Chen W, Wang W, Peng M, Gong L, Gao Y, Wan J, et al. Comparative and parallel genome-wide association studies for metabolic and agronomic traits in cereals. Nat Commun. 2016;7(1):12767. https://doi.org/10.1038/ncomms12767.
Peng S, Deyssenroth MA, Di Narzo AF, Cheng H, Zhang Z, Lambertini L, et al. Genetic regulation of the placental transcriptome underlies birth weight and risk of childhood obesity. PLoS Genet. 2018;14(12):e1007799. https://doi.org/10.1371/journal.pgen.1007799.
Albert FW, Kruglyak L. The role of regulatory variation in complex traits and disease. Nat Rev Genet. 2015;16(4):197–212. https://doi.org/10.1038/nrg3891.
Melé M, Ferreira PG, Reverter F, DeLuca DS, Monlong J, Sammeth M, et al. The human transcriptome across tissues and individuals. Science. 2015;348(6235):660–5. https://doi.org/10.1126/science.aaa0355.
Aguet F, Brown AA, Castel SE, Davis JR, He Y, Jo B, et al. Genetic effects on gene expression across human tissues. Nature. 2017;550(7675):204–13. https://doi.org/10.1038/nature24277.
Consortium G. The GTEx Consortium atlas of genetic regulatory effects across human tissues. Science. 2020;369(6509):1318–30. https://doi.org/10.1126/science.aaz1776.
Liu S, Gao Y, Canela-Xandri O, Wang S, Yu Y, Cai W, et al. A multi-tissue atlas of regulatory variants in cattle. Nat Genet. 2022;54(9):1438–47. https://doi.org/10.1038/s41588-022-01153-5.
Consortium TF-P. A compendium of genetic regulatory effects across pig tissues. bioRxiv. 2022:2022.11.11.516073. https://doi.org/10.1101/2022.11.11.516073.
Yuan Z, Sunduimijid B, Xiang R, Behrendt R, Knight MI, Mason BA, et al. Expression quantitative trait loci in sheep liver and muscle contribute to variations in meat traits. Genet Sel Evol. 2021;53(1):8. https://doi.org/10.1186/s12711-021-00602-9.
Guan D, Bai Z, Zhu X, Zhong C, Hou Y, Consortium TC, et al. The ChickenGTEx pilot analysis: a reference of regulatory variants across 28 chicken tissues. bioRxiv. 2023:2023.06.27.546670. https://doi.org/10.1101/2023.06.27.546670.
Littlejohn MD, Tiplady K, Fink TA, Lehnert K, Lopdell T, Johnson T, et al. Sequence-based association analysis reveals an MGST1 eQTL with pleiotropic effects on bovine milk composition. Sci Rep. 2016;6(1):25376. https://doi.org/10.1038/srep25376.
Kemper KE, Littlejohn MD, Lopdell T, Hayes BJ, Bennett LE, Williams RP, et al. Leveraging genetically simple traits to identify small-effect variants for complex phenotypes. BMC Genom. 2016;17(1):858. https://doi.org/10.1186/s12864-016-3175-3.
Bolger AM, Lohse M, Usadel B. Trimmomatic: a flexible trimmer for Illumina sequence data. Bioinformatics. 2014;30(15):2114–20. https://doi.org/10.1093/bioinformatics/btu170.
Kim D, Paggi JM, Park C, Bennett C, Salzberg SL. Graph-based genome alignment and genotyping with HISAT2 and HISAT-genotype. Nat Biotechnol. 2019;37(8):907–15. https://doi.org/10.1038/s41587-019-0201-4.
Pertea M, Pertea GM, Antonescu CM, Chang T-C, Mendell JT, Salzberg SL. StringTie enables improved reconstruction of a transcriptome from RNA-seq reads. Nat Biotechnol. 2015;33(3):290–5. https://doi.org/10.1038/nbt.3122.
Poplin R, Ruano-Rubio V, DePristo MA, Fennell TJ, Carneiro MO, Auwera GAVd, et al. Scaling accurate genetic variant discovery to tens of thousands of samples. bioRxiv. 2018:201178. https://doi.org/10.1101/201178.
Browning BL, Tian X, Zhou Y, Browning SR. Fast two-stage phasing of large-scale sequence data. Am J Hum Genet. 2021;108(10):1880–90. https://doi.org/10.1016/j.ajhg.2021.08.005.
Purcell S, Neale B, Todd-Brown K, Thomas L, Ferreira MAR, Bender D, et al. PLINK: a tool set for whole-genome association and population-based linkage analyses. Am J Hum Genet. 2007;81(3):559–75. https://doi.org/10.1086/519795.
Stegle O, Parts L, Piipari M, Winn J, Durbin R. Using probabilistic estimation of expression residuals (PEER) to obtain increased power and interpretability of gene expression analyses. Nat Protoc. 2012;7(3):500–7. https://doi.org/10.1038/nprot.2011.457.
Brown AA, Fernandez-Tajes JJ, Hong MG, Brorsson CA, Koivula RW, Davtian D, et al. Genetic analysis of blood molecular phenotypes reveals common properties in the regulatory networks affecting complex traits. Nat Commun. 2023;14(1):5062. https://doi.org/10.1038/s41467-023-40569-3.
Ongen H, Buil A, Brown AA, Dermitzakis ET, Delaneau O. Fast and efficient QTL mapper for thousands of molecular phenotypes. Bioinformatics. 2016;32(10):1479–85.
Beißbarth T, Speed TP. GOstat: find statistically overrepresented gene ontologies within a group of genes. Bioinformatics. 2004;20(9):1464–5. https://doi.org/10.1093/bioinformatics/bth088.
Storey JD, Tibshirani R. Statistical significance for genomewide studies. Proc Natl Acad Sci U S A. 2003;100(16):9440–5. https://doi.org/10.1073/pnas.1530509100.
Yang J, Lee SH, Goddard ME, Visscher PM. GCTA: a tool for genome-wide complex trait analysis. Am J Hum Genet. 2011;88(1):76–82. https://doi.org/10.1016/j.ajhg.2010.11.011.
Giambartolomei C, Vukcevic D, Schadt EE, Franke L, Hingorani AD, Wallace C, et al. Bayesian test for colocalisation between pairs of genetic association studies using summary statistics. PLoS Genet. 2014;10(5):e1004383. https://doi.org/10.1371/journal.pgen.1004383.
Teng J, Gao Y, Yin H, Bai Z, Liu S, Zeng H, et al. A compendium of genetic regulatory effects across pig tissues. Nat Genet. 2024;56(1):112–23. https://doi.org/10.1038/s41588-023-01585-7.
Guo Y, Daghlas I, Gormley P, Giulianini F, Ridker PM, Mora S, et al. Phenotypic and genotypic associations between migraine and lipoprotein subfractions. Neurology. 2021;97(22):e2223–35. https://doi.org/10.1212/wnl.0000000000012919.
Consortium G. Genetic effects on gene expression across human tissues. Nature. 2017;550(7675):204–13. https://doi.org/10.1038/nature24277.
Cai W, Zhang Y, Chang T, Wang Z, Zhu B, Chen Y, et al. The eQTL colocalization and transcriptome-wide association study identify potentially causal genes responsible for economic traits in Simmental beef cattle. J Anim Sci Biotechnol. 2023;14(1):78. https://doi.org/10.1186/s40104-023-00876-7.
Fagny M, Paulson JN, Kuijjer ML, Sonawane AR, Chen C-Y, Lopes-Ramos CM, et al. Exploring regulation in tissues with eQTL networks. Proc Natl Acad Sci. 2017;114(37):E7841–50. https://doi.org/10.1073/pnas.1707375114.
Tranell A, Fenyö EM, Schwartz S. Serine- and Arginine-rich Proteins 55 and 75 (SRp55 and SRp75) Induce Production of HIV-1 vpr mRNA by Inhibiting the 5′-Splice Site of Exon 3. J Biol Chem. 2010;285(41):31537–47. https://doi.org/10.1074/jbc.M109.077453.
Sapra AK, Änkö M-L, Grishina I, Lorenz M, Pabis M, Poser I, et al. SR Protein Family Members Display Diverse Activities in the Formation of Nascent and Mature mRNPs In Vivo. Mol Cell. 2009;34(2):179–90. https://doi.org/10.1016/j.molcel.2009.02.031.
Masaki S, Kabuto T, Suzuki K, Kataoka N. Multiple nuclear localization sequences in SRSF4 protein. Genes Cells. 2020;25(5):327–33. https://doi.org/10.1111/gtc.12756.
Ghigna C, Moroni M, Porta C, Riva S, Biamonti G. Altered expression of heterogenous nuclear ribonucleoproteins and SR factors in human colon adenocarcinomas. Cancer Res. 1998;58(24):5818–24.
Consortium TF-P, Teng J, Gao Y, Yin H, Bai Z, Liu S, et al. A compendium of genetic regulatory effects across pig tissues. bioRxiv. 2022:2022.11.11.516073. https://doi.org/10.1101/2022.11.11.516073.
Njålsson R, Norgren S. Physiological and pathological aspects of GSH metabolism. Acta Paediatr. 2005;94(2):132–7. https://doi.org/10.1111/j.1651-2227.2005.tb01878.x.
Polekhina G, Board PG, Gali RR, Rossjohn J, Parker MW. Molecular basis of glutathione synthetase deficiency and a rare gene permutation event. Embo j. 1999;18(12):3204–13. https://doi.org/10.1093/emboj/18.12.3204.
Sollis E, Mosaku A, Abid A, Buniello A, Cerezo M, Gil L, et al. The NHGRI-EBI GWAS Catalog: knowledgebase and deposition resource. Nucleic Acids Res. 2023;51(D1):D977–85. https://doi.org/10.1093/nar/gkac1010.
Tachmazidou I, Süveges D, Min JL, Ritchie GRS, Steinberg J, Walter K, et al. Whole-Genome Sequencing Coupled to Imputation Discovers Genetic Signals for Anthropometric Traits. Am J Hum Genet. 2017;100(6):865–84. https://doi.org/10.1016/j.ajhg.2017.04.014.
Stöhr N, Köhn M, Lederer M, Glass M, Reinke C, Singer RH, et al. IGF2BP1 promotes cell migration by regulating MK5 and PTEN signaling. Genes Dev. 2012;26(2):176–89. https://doi.org/10.1101/gad.177642.111.
Müller S, Glaß M, Singh AK, Haase J, Bley N, Fuchs T, et al. IGF2BP1 promotes SRF-dependent transcription in cancer in a m6A- and miRNA-dependent manner. Nucleic Acids Res. 2018;47(1):375–90. https://doi.org/10.1093/nar/gky1012.
Wang K, Hu H, Tian Y, Li J, Scheben A, Zhang C, et al. The chicken pan-genome reveals gene content variation and a promoter region deletion in IGF2BP1 affecting body size. Mol Biol Evol. 2021;38(11):5066–81. https://doi.org/10.1093/molbev/msab231.
Yengo L, Vedantam S, Marouli E, Sidorenko J, Bartell E, Sakaue S, et al. A saturated map of common genetic variants associated with human height. Nature. 2022;610(7933):704–12. https://doi.org/10.1038/s41586-022-05275-y.
Gershoni M, Levin L, Ovadia O, Toiw Y, Shani N, Dadon S, et al. Disrupting mitochondrial-nuclear coevolution affects OXPHOS complex I integrity and impacts human health. Genome Biol Evol. 2014;6(10):2665–80. https://doi.org/10.1093/gbe/evu208.
Raffa S, Scrofani C, Valente S, Micaloni A, Forte M, Bianchi F, et al. In vitro characterization of mitochondrial function and structure in rat and human cells with a deficiency of the NADH: ubiquinone oxidoreductase Ndufc2 subunit. Hum Mol Genet. 2017;26(23):4541–55. https://doi.org/10.1093/hmg/ddx333.
Rubattu S, Di Castro S, Schulz H, Geurts AM, Cotugno M, Bianchi F, et al. Ndufc2 Gene Inhibition Is Associated With Mitochondrial Dysfunction and Increased Stroke Susceptibility in an Animal Model of Complex Human Disease. J Am Heart Assoc. 2016;5(2). https://doi.org/10.1161/jaha.115.002701.
Olsson AH, Rönn T, Ladenvall C, Parikh H, Isomaa B, Groop L, et al. Two common genetic variants near nuclear-encoded OXPHOS genes are associated with insulin secretion in vivo. Eur J Endocrinol. 2011;164(5):765–71. https://doi.org/10.1530/eje-10-0995.
Cho H-W, Jin H-S, Eom Y-B. Association between non-Caucasian-specific ASCC1 gene polymorphism and osteoporosis and obesity in Korean postmenopausal women. J Bone Miner Metab. 2020;38(6):868–77. https://doi.org/10.1007/s00774-020-01120-2.
Grall F, Gu X, Tan L, Cho JY, Inan MS, Pettit AR, et al. Responses to the proinflammatory cytokines interleukin-1 and tumor necrosis factor alpha in cells derived from rheumatoid synovium and other joint tissues involve nuclear factor kappaB-mediated induction of the Ets transcription factor ESE-1. Arthritis Rheum. 2003;48(5):1249–60. https://doi.org/10.1002/art.10942.
Wang S, Chen L, Xu B. ELF3-induced miR-182 inhibits adipogenic differentiation in Graves’ orbitopathy by targeting thyrotropin receptor. Cent Eur J Immunol. 2022;47(4):308–22. https://doi.org/10.5114/ceji.2022.124087.
Conde J, Otero M, Scotece M, Abella V, Gómez R, López V, et al. E74-Like Factor (ELF3) and Leptin, a Novel Loop Between Obesity and Inflammation Perpetuating a Pro-Catabolic State in Cartilage. Cell Physiol Biochem. 2018;45(6):2401–10. https://doi.org/10.1159/000488227.
Huang J, Huffman JE, Huang Y, Do Valle Í, Assimes TL, Raghavan S, et al. Genomics and phenomics of body mass index reveals a complex disease network. Nat Commun. 2022;13(1):7973. https://doi.org/10.1038/s41467-022-35553-2.
Llanos S, Efeyan A, Monsech J, Dominguez O, Serrano M. A high-throughput loss-of-function screening identifies novel p53 regulators. Cell Cycle. 2006;5(16):1880–5. https://doi.org/10.4161/cc.5.16.3140.
Zhang R, Yang Y, He C, Zhang X, Torraca V, Wang S, et al. RUNDC1 inhibits autolysosome formation and survival of zebrafish via clasping ATG14-STX17-SNAP29 complex. Cell Death Differ. 2023. https://doi.org/10.1038/s41418-023-01215-z.
Acknowledgements
We are grateful to all members of the Waterfowl Breeding and Nutrition Innovative Research Team for the F2 population phenotype data collection.
Funding
This work was supported by grants from National Key Research and Development Project of China (2023YFD1300301), China Agriculture Research System of MOF and MARA (CARS-42), the Science and Technology Innovation Project of the Chinese Academy of Agricultural Sciences (CXGC-IAS-09), Taishan Industry Leadership Talent Project of Shandong province in China (TSCY20190108), and Key Technologies Research on New Breed of Broiler Poultry by Integration of Breeding, Reproduction and Promotion (2021CXGC010805-02).
Author information
Authors and Affiliations
Contributions
S.H. and W.C. conceived and designed the study. W.C. analyzed the data and wrote the manuscript. S.H. revised the paper, J.H., Y.Z., Z.G., and Z.Z. collected samples. All authors have directly contributed to this manuscript.
Corresponding author
Ethics declarations
Ethics approval and consent to participate
Not applicable.
Consent for publication
Not applicable.
Competing interests
The authors declare no competing interests.
Additional information
Publisher’s Note
Springer Nature remains neutral with regard to jurisdictional claims in published maps and institutional affiliations.
Supplementary Information
Additional file 1: Fig. S1.
Characterization of PEER factors. Factor weight variance as a function of PEER factors computed up to 30 factors for each of seven tissues. Factor weight variances become small for tissues when the number of inferred hidden PEER factors reaches 15.
Additional file 2: Fig. S2.
The number of overlapping eGenes identified using 15 and 20 peer variables in blood tissues.
Additional file 3: Fig. S3.
The distribution of cis-eQTLs in blood.
Additional file 4: Fig. S4.
The distribution of cis-eQTLs in ovary.
Additional file 5: Fig. S5.
The distribution of cis-eQTLs in adipose.
Additional file 6: Fig. S6.
The distribution of cis-eQTLs in spleen.
Additional file 7: Fig. S7.
The distribution of cis-eQTLs in lung.
Additional file 8: Fig. S8.
The P-value distribution of top significant cis-eQTLs for each tested gene in muscle, blood, ovary, adipose, spleen and lung.
Additional file 9: Fig. S9.
The number of eVariants overlaps between tissues.
Additional file 10: Fig. S10.
The colocalized results between cis-eQTLs and GWAS signals in other five tissues. Manhattan plot illustrates the colocalization results (H4 > 0.4) between cis-eQTLs and GWAS signals. The x-axis is the P-value of lead eQTLs (points) across trait categories (colors). The right labels are colocalized SNP-gene-trait pairs.
Additional file 11: Fig. S11.
The cis-QTL signals of liver GSS and muscle NDUFC2 using conditional analysis. (A) The cis-QTL signals of liver GSS using the top eQTL signal 21:3990920 as a covariate. (B) The cis-QTL signals of muscle NDUFC2 using the top eQTL signal 21:203833042 as a covariate.
Additional file 12: Table S1.
The information for 820 RNA-seq samples.
Additional file 13: Table S2.
The consistency of SNPs calling using different RNA-seq samples from the same individuals.
Additional file 14: Table S3.
Result of principal component significance test for genotype.
Additional file 15: Table S4.
The summary of GWAS results for 50 agronomic traits.
Additional file 16: Table S5.
GO and KEEG annotation of 244 eGenes shared by at least four tissues.
Additional file 17:
Table S6. The GWAS results of significant signals with P < 5 × 10–8 for 47 traits.
Additional file 18: Table S7.
The results of GWAS-eQTL colocalization by Coloc in seven tissues.
Rights and permissions
Open Access This article is licensed under a Creative Commons Attribution 4.0 International License, which permits use, sharing, adaptation, distribution and reproduction in any medium or format, as long as you give appropriate credit to the original author(s) and the source, provide a link to the Creative Commons licence, and indicate if changes were made. The images or other third party material in this article are included in the article's Creative Commons licence, unless indicated otherwise in a credit line to the material. If material is not included in the article's Creative Commons licence and your intended use is not permitted by statutory regulation or exceeds the permitted use, you will need to obtain permission directly from the copyright holder. To view a copy of this licence, visit http://creativecommons.org/licenses/by/4.0/. The Creative Commons Public Domain Dedication waiver (http://creativecommons.org/publicdomain/zero/1.0/) applies to the data made available in this article, unless otherwise stated in a credit line to the data.
About this article
Cite this article
Cai, W., Hu, J., Zhang, Y. et al. Cis-eQTLs in seven duck tissues identify novel candidate genes for growth and carcass traits. BMC Genomics 25, 429 (2024). https://doi.org/10.1186/s12864-024-10338-7
Received:
Accepted:
Published:
DOI: https://doi.org/10.1186/s12864-024-10338-7