Abstract
Introduction
Spontaneous reporting of suspected adverse drug reactions is key for efficient post-marketing safety surveillance. To increase usability and accessibility of reporting tools, the Web-Recognising Adverse Drug Reactions (WEB-RADR) consortium developed a smartphone application (app) based on a simplified reporting form.
Objective
The objective of this study was to evaluate the characteristics, quality and contribution to signals of reports submitted via the WEB-RADR app.
Methods
The app was launched in the UK, the Netherlands and Croatia between July 2015 and May 2016. Spontaneous reports submitted until September 2016 with a single reporter were included. For each country, app reports and reports received through conventional means in the same time period were compared to identify characteristic features. A random subset of reports was assessed for clinical quality and completeness. The contribution to signal detection was assessed by a descriptive analysis.
Results
Higher proportions of app reports were submitted by patients in the UK (28 vs. 18%) and Croatia (32 vs. 7%); both p < 0.01. In the Netherlands, the difference was small (60 vs. 57%; p = 0.5). The proportion of female patients and the median patient ages in app reports submitted by patients were similar to the reference. The proportion of reports of at least moderate quality was high in both samples (app: 78–85%, reference: 78–98%), for all countries. App reports contributed to detecting eight potential safety signals at the national level, four of which were eventually signalled.
Conclusion
The WEB-RADR app offers a new route of spontaneous reporting that shows promise in attracting reports from patients and that could become an important tool in the future. Patient demographics are similar to conventional routes, report quality is sufficient despite a simplified reporting form, and app reports show potential in contributing to signal detection.
Similar content being viewed by others
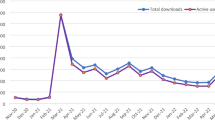
Avoid common mistakes on your manuscript.
Reports on suspected adverse drug reactions received through the WEB-RADR mobile application (app) are of adequate quality for signal detection, despite a simplified reporting form. |
The characteristics of the app reports are similar to those received through conventional routes and show promise in attracting reports directly from patients. |
The sample of app reports used in this study is limited, and this study should be repeated once a larger volume of app reports has been obtained. |
1 Introduction
In spontaneous reporting systems, cases of suspected adverse drug reactions (ADRs) are reported with the aim to detect signals. A signal can be either a new unknown adverse reaction or a new aspect of an already known association [1]. Spontaneous reporting systems receive reports from different sources and through different reporting means. These systems have many advantages, such as operating for all drugs during their whole life cycle and being an affordable method for detection of serious and rare ADRs. Spontaneous reporting has led to the identification and verification of many unexpected and serious ADRs [2,3,4]. Potential disadvantages of spontaneous reporting are that no incidences can be calculated due to reporting bias and a lack of information on the number of exposed patients [5]. Underreporting may make it difficult to reach conclusions regarding relative risks, and may hamper the detection of safety signals. There are various potential reasons for underreporting, such as lack of time, different care priorities, uncertainty about the drug causing the ADR, inaccessible reporting forms, lack of awareness of the requirements for reporting and lack of knowledge regarding the purpose of spontaneous reporting systems. Well-known and non-serious ADRs are less likely to be reported [6, 7].
Current technologies lead to new opportunities for ADR reporting. The number of people using a smartphone or tablet computer for health-related reasons is high, especially among those who are young, have a high level of education, a high income and with self-reported excellent health [8]. These technologies, including mobile applications (apps), may serve as new methods to collect spontaneous ADR reports. Healthcare professionals and patients view apps as accessible tools that may increase the reporting of ADRs. However, these tools need to be usable, visually attractive and available in the languages of the intended users [9]. A previous study indicates that most patients prefer an app, and experience apps as efficient and realistic methods of reporting vaccine adverse events [10]. Lack of time is mentioned as an influencing factor in ADR reporting [9], and a study in USA used the Medwatcher app to report ADRs concerning the medical device Essure® to the US Food and Drug Administration [11]. It showed that an average app report takes 11 min to complete compared with 40 min by traditional reporting. The study also found these app reports to be of a high average quality as measured by the vigiGrade completeness score, [12] with an average of 0.8 (range 0–1). For a report to be of most use for signal detection purposes, it needs to contain high-quality clinical information [13], which is needed for a good causality assessment. However, little is known concerning the characteristics, quality and the contribution to signal detection of spontaneous ADR reports submitted via an app compared with conventional routes. Consequently, the aim of this study was to evaluate the characteristics, quality and contribution to signal detection of spontaneous reports via an accessible app.
2 Methods
2.1 Data Collection
2.1.1 WEB-RADR app
The Web-Recognising Adverse Drug Reactions (WEB-RADR) project [14] developed an app for spontaneous reporting of ADRs, based on a simplified reporting form. This WEB-RADR app was used to collect the app reports used in this study. The form contained questions about the patient, the suspected drug(s), ADR(s), and medical history and is fully compatible with the ICH-E2B(R2) format, which is a current standard for the electronic transfer of ADR reports [15]. In designing the app, we tried to create a reporting form that would be easy to use and quick to complete on a mobile device. By reducing the number of structured fields, and allowing for more free text, the number of questions to answer was drastically reduced. Additionally, by letting the answer on a previous question trigger the next one, the burden on the reporter was further reduced. For example, in the conventional web form, several separate questions are asked regarding the ADR, whereas in the app, these questions can be answered more freely in one single text field. This type of simplification is used throughout the form. Besides the reporting functionality, the app also provided drug safety information [9]. Users were able to create a list of medications on which to receive news from the national competent authority (NCA), and to view numbers of reports received by the NCA.
The app was launched in three countries: UK (July 2015), Netherlands (January 2016) and Croatia (May 2016). The app was promoted through social media in the UK, and through a press release and information on the Lareb website in the Netherlands. In Croatia, the app was introduced in a press conference and received national media attention.
2.1.2 Data Extraction and Processing
The data collection period was between the launch date of the app in each country and 1 September, 2016. All reports within the time period for each country were extracted from VigiBase, the World Health Organization global database of individual case safety reports [16]. The data were filtered to meet the following criteria: (1) report type “spontaneous” to exclude study reports; (2) a single reporter that is either a patient or healthcare professional; and (3) the earliest available version of all reports to avoid introducing a bias owing to differing follow-up procedures. Reporter type “patient” was defined as the category “consumer/non-health professional”, and reporter type “healthcare professional” as “physician”, “pharmacist” or “other health professional” in the ICH-E2B reporting standard [15]. Reports from market authorisation holders and automatically generated reports from external sources such as poison control centres were excluded because these types of reports could not be collected through the WEB-RADR app. A reference sample of reports from conventional sources was collected for each of the three countries during the same period that app reports were collected for that country. These reports consist of approximately 95% electronic reports for the Netherlands and the UK, and about 30% for Croatia. The other reports were received on paper. The questions on the paper forms and electronic forms are close to identical to meet the ICH-E2B reporting standard [15]. The reference sample was extracted from VigiBase together with the app reports and adhered to the same criteria.
2.2 Analysis
2.2.1 Characteristics of Reports
2.2.1.1 vigiPoint Features in the Full Dataset
vigiPoint [17] was used on the full dataset for the comparison between the reports received through the app and the reference sample. vigiPoint automatically highlights key features in a user-defined case series compared to a reference sample using log-odds ratios (ORs) to identify statistically significant differences between the case series and the reference sample in various pre-defined stratifications such as patient age, sex, co-reported drugs, co-reported ADRs, drug indication, type of reporter and geographical location. A key feature is defined as having a log OR005 > 0.5 or a log OR995 < − 0.5, where log OR005 and log OR995 are the lower and upper limits of the 99% credibility interval of the log OR, respectively. Requiring that the credible interval is at least 0.5 above or below zero ensures that the key feature, apart from being statistically significant, also deviates enough to be considered a characteristic feature of the case series. The vigiPoint analysis was performed using all extracted reports and was done for each country separately.
2.2.1.2 Reports Submitted by Patients
The difference in the proportion of patient reports between the app and reference sample was compared for each country separately, using the χ2 test. P values < 0.01 were considered statistically significant. For the subset of reports from patients, patient age and sex were compared between the app and the reference sample for each country separately, using the Mann–Whitney U test and the χ2 test, respectively. P values < 0.01 were considered statistically significant. Because the vigiPoint analysis includes both patient and healthcare professional reports, and the Mann–Whitney U test and the χ2 test use different approaches in measuring statistical differences, the results between the vigiPoint and the two traditional tests are not identical.
2.2.2 Quality of Reports
2.2.2.1 Clinical Documentation Tool
To measure and compare the clinical quality, a subsample of a maximum of 100 app reports and a corresponding number of reference reports were selected randomly for each country by the Uppsala Monitoring Centre using VigiBase. The randomisation was performed using a fixed ratio between reports sent by healthcare professionals and reports sent by patients, based on the country-specific proportion in the app reports. App reports and reference reports were manually scored by blinded assessors, separately for each country, with the clinical documentation assessment tool ClinDoc [13]. The assessors used the report version stored in their national database. Clinical quality was divided into three categories: well (≥ 75%), moderately (46–74%) or poorly (≤ 45%) documented reports. The proportion of reports of at least moderate clinical quality was compared between app reports and reference reports, using a χ2 test. A p value < 0.01 was considered statistically significant.
2.2.2.2 vigiGrade Completeness Score
The manually assessed reports were also subjected to an automated evaluation of quality using vigiGrade [12]. The vigiGrade completeness score measures how complete the information on the report is, based on a selection of ICH-E2B fields sent from the national databases to VigiBase. Each report field carries a weight depending on the field’s importance in regard to causality assessment. The score ranges between zero and one, where zero represents a report with all considered fields empty, and one represents a fully complete report.
2.2.3 Duplication Rate
With the introduction of an additional reporting route, report duplication is a potential concern. To identify possible duplicate reports both within each sample and between the app and reference samples, an automatic algorithm called vigiMatch was used. vigiMatch uses a probabilistic hit-miss model, by scoring a report pair while taking into account the amount of matching data. If the calculated total score reaches above a pre-set threshold, the report pair is flagged as a suspected duplicate [18, 19].
Each report within the dataset that is flagged as a suspected duplicate will be counted, regardless whether its suspected duplicate is within the dataset used in this study or not. For example, if one report is flagged as a suspected duplicate, but its pair entered VigiBase before or after the dates defined for the datasets in this study (until January 2017), it is counted as one suspected duplicate. However, if its duplicate pair is within the study sample, both will be counted as both are flagged as suspected duplicates. Note that the countries perform some duplicate detection in their own database before submitting reports to VigiBase.
2.2.4 Prospective Contribution of App Reports to Signal Detection
The contribution of app reports to signal detection can highlight the potential value of app reports. This was quantified by counting how many drug–event combinations, analysed potential signals and issued signals that included any app reports. The following signal detection processes were investigated: (1) NCAs in the three countries and (2) Uppsala Monitoring Centre.
2.2.4.1 Identifying Signals in National Competent Authorities
In the Netherlands and Croatia, case-by-case review is performed to identify signals. The Netherlands is also performing disproportionality analysis as a supportive measure on the reports that are not assessed manually, mostly those submitted by pharmaceutical companies. In the UK, drug–event combinations of potential interest are flagged for assessment based on pre-defined criteria. These criteria include both statistical methods to identify drug–event combinations that are disproportionately present in the database and rule-based approaches to highlight populations or events of particular interest and particularly serious cases. Signal assessors then review the drug–event combinations. When identifying a potential signal, these are discussed at a signal detection meeting where it is decided whether further action is required.
2.2.4.2 Identifying Signals at the Uppsala Monitoring Centre
At the Uppsala Monitoring Centre, the first step for signal detection is performed in a signal detection sprint, in which initial assessments of drug–event combinations take place with a pre-defined set of filters (usually at the report and drug–event combination level with a specific theme). After filtering, drug–event combinations are ordered using vigiRank [20]. Drug–event combinations are assessed manually in order of decreasing vigiRank score, and a subset of drug–event combinations are taken further for an in-depth manual assessment.
2.2.5 Contribution of App Reported Drug–Event Combinations at the European Medicines Agency
Reports from the app are not distinguished in EudraVigilance (operated by the European Medicines Agency on behalf of the European Union medicines regulatory network) and therefore no prospective analysis of the contribution to signal detection could be performed. Drug–event combinations were identified for the reports received via the app, and the Proportional Reporting Ratio [21] was calculated based on all EudraVigilance reports to identify signals of disproportionate reporting. A distinction was made between products authorised through the single ‘centralised’ procedure for the European Union (using a European Medicines Agency internal signal detection tracking table) and products authorised through other procedures (using the European Issues Tracking Tool, EPITT). It was investigated how many of these drug–event combinations would have qualified as a validated signal including those that were identified historically.
3 Results
3.1 Data Collection
The resulting number of app reports collected was 144 for the UK, 106 for the Netherlands and 37 for Croatia. The number of reports in the reference sample, collected during the same time period, was 22,582 for the UK, 5779 for the Netherlands and 307 for Croatia.
3.2 Analysis
3.2.1 Characteristics of Reports
3.2.1.1 vigiPoint Features in the Full Dataset
vigiPoint identified key features for all three countries. The full lists are available in the Electronic Supplementary Material. The results for reporter type, patient sex and age are visualised in Figs. 1, 2 and 3. In the UK, app reports more frequently originated from pharmacists, while the reporter type “other health professional” was reported less frequently. In the Netherlands, there were no detected vigiPoint features for reporter type. In Croatia, app reports more frequently originated from patients, while physicians reported less frequently. For patient sex and age, there were no detected vigiPoint features for any of the three countries.
Distributions of reporter type for the full dataset as calculated by vigiPoint, presented for each country separately. Each bar represents the proportion among the application (app) reports and the vertical lines represent the corresponding proportion in the reference sample. If the difference between the two samples is large enough to be considered a vigiPoint feature, the bar and line are coloured yellow and red if the app sample has a higher proportion of reports, and coloured light blue and dark blue for the opposite scenario
Distributions of reported patient sex for the full dataset as calculated by vigiPoint, presented for each country separately. Each bar represents the proportion among the application (app) reports and the vertical lines represent the corresponding proportion in the reference sample. If the difference between the two samples is large enough to be considered a vigiPoint feature, the bar and line are coloured yellow and red if the app sample has a higher proportion of reports, and coloured light blue and dark blue for the opposite scenario
Distributions of reported patient ages for the full dataset as calculated by vigiPoint, presented for each country separately. Each bar represents the proportion among the application (app) reports and the vertical lines represent the corresponding proportion in the reference sample. If the difference between the two samples is large enough to be considered a vigiPoint feature, the bar and line are coloured yellow and red if the app sample has a higher proportion of reports, and coloured light blue and dark blue for the opposite scenario
3.2.1.2 Reports Submitted by Patients
In the app sample (n = 287), 116 (40%) were patient reports. For the UK and Croatia, there was a significantly (p < 0.01) larger proportion of patient reports in the app sample (UK = 28%, Croatia = 32%), compared with the reference sample (UK = 18%, Croatia = 7%). For the Netherlands, there was no significant difference between the proportions of patient reports in the app sample (60%), compared with the reference sample (57%).
There was no significant difference (p ≥ 0.16 for all countries) in the distributions of patient sex in patient reports for the app sample, compared with the reference sample (Table 1). There was no significant difference in patient ages for patient reports sent in via the app (median ages: UK = 42.5 years, the Netherlands = 47.5 years, Croatia = 38.0 years) compared with reference reports (median ages: UK = 44.0 years, the Netherlands = 45.0 years, Croatia = 36.0 years), p ≥ 0.17 for all countries.
3.2.2 Quality of Reports
The resulting randomised datasets used for the quality assessments contained 100 app reports and 100 reference reports each for the UK and the Netherlands. For Croatia, 37 app reports and 68 reference reports were included because they had not received 100 app reports and the reference sample did not contain a sufficient number of patient reports.
3.2.2.1 Clinical Documentation Tool
For all countries, the proportion of reports of at least moderate clinical quality was high for both the app and reference sample, but overall lower for the app sample (UK = 83%; the Netherlands = 85%; Croatia = 78%) compared with the reference sample (UK = 92%, p = 0.08; the Netherlands = 98%, p < 0.01; Croatia = 78%, p = 1.0) (Fig. 4).
3.2.2.2 vigiGrade Completeness Score
For all countries, the vigiGrade completeness score was high for both the app and reference samples, but overall lower for the app samples (Fig. 5).
3.2.3 Duplication Rate
The fractions of duplicate reports among the app reports were similar or lower than among the reference reports. For the UK, 1.4% of the app reports were suspected duplicates compared with 1.8% of the reference reports. For the Netherlands, the proportions of suspected duplicates were 0.0% for the app reports and 0.5% for the reference reports. For Croatia, these percentages were 2.7% and 0.0%, respectively.
3.2.4 Prospective Contribution of app Reports to Signal Detection
In the national signal detection processes in the three countries, app reports contributed to the analysis of eight potential safety signals resulting in four issued signals (Table 2). A potential safety signal is defined as a drug–event combination that has undergone an initial manual assessment and is in need of a more in-depth review. No app reports contributed to signals raised through VigiBase. Note that this analysis was conducted in the national databases at a later date than the main data extraction described in Sect. 2.1.2, hence the number of app reports is higher.
3.2.5 ‘Relevance’ of App Reported Drug–Event Combinations at the European Medicines Agency
In the analysis from the European Medicines Agency, it was shown that 19 drug–event combinations reported through the app had been validated signals in the past (Table 3).
4 Discussion
Current technologies lead to new opportunities for ADR reporting. Within the WEB-RADR project, an app for two-way safety communication and spontaneous reporting of ADRs was developed. We evaluated the characteristics and quality of spontaneous reports submitted via this app in the UK, the Netherlands and Croatia, and compared them with conventional reports. Our results indicate no differences in patient demographics, and they display a higher proportion of patient reporting through the app. Further, despite the use of a simplified reporting form, the app reports were of sufficient quality, albeit slightly lower than in the conventional reports. The usefulness of the app reports is further supported by the finding that they contributed to eight potential safety issues at the national level, four of which were eventually signalled.
A higher proportion of patients reported via the app than via conventional means in the UK and Croatia, whereas in the Netherlands, the difference was small and non-significant. A possible explanation for this difference across the countries is that the UK used social media to promote the app, and that this way of promotion might have attracted more patients than healthcare professionals to the app. Croatia managed to gain national media attention for the app, which also might have increased uptake and reporting by the general public. In the Netherlands, the promotion consisted of a press release and information on the website. Another possible explanation might be that in the Netherlands, the proportion of patient reports in the reference sample is high (in 2016, 55% of all reports that were sent directly to Lareb were from patients). This high percentage of patient reporting might make it more difficult to see trends of increased reporting in such a limited sample of app reports.
The proportion of reports of at least moderate quality was high in both groups, for all countries, but overall lower for app reports. The reason for this could be that the app reporting form has been simplified to make reporting faster and easier. The standard reporting forms used in the three countries are more extensive, which facilitates good-quality reports as recognised by the methods used in this study, but may also form a barrier for reporting. The results from this study are encouraging because a simplified reporting form still yields reports of comparable quality for signal detection purposes.
When introducing a new reporting route, one concern would be the potential for increased duplicate reports being received. However, using probabilistic record matching, the study shows that app reports do not contribute to more potential duplicates than reports from conventional means.
Although there were limited differences between app reports and reference reports in each national setting, there were clear differences when comparing the different national reporting systems to each other. Although not the subject of this article, differences in national reporting systems are expected because of a range of issues including awareness levels, cultural differences, differing healthcare settings, promotional tactics and system maturity. Approaches to data collection also differ between individual NCAs, reflecting differing national and global requirements. There may be value in future work to further characterise these differences and reduce unnecessary inconsistencies across the global pharmacovigilance network.
In reports with high quality, a more reliable causality assessment can be performed, and cases with high quality are therefore more valuable from a signal detection point of view. However, the usefulness of a report cannot be entirely determined on the basis of its quality alone, its contribution to signal detection also needs to be investigated to be useful for pharmacovigilance purposes.
In this study, the contribution of app reports to signal detection was investigated. For the NCAs and the Uppsala Monitoring Centre, this was conducted in a prospective manner, i.e., the contribution to signal detection was measured from the first day of the app report until the last day of the data collection period. The European Medicines Agency analysis examined the ‘relevance’ of app reported drug–event combinations by comparing them to validated signals in the past. Through national signal detection, eight potential safety issues with at least one app report were found. At the time of this analysis, no app reports had contributed to signals raised by the Uppsala Monitoring Centre from the analysis of VigiBase. The most likely explanation for this is the tiny proportion of incoming VigiBase reports that originate from the app. This makes the app reports very unlikely to even be considered among the relatively limited number of drug–event combinations that remain after statistical prioritisation and other relevance filtering have been applied, not to mention among those few drug–event combinations that eventually become signals. Nineteen drug–event combinations reported through the app correspond to already validated signals at the European Medicines Agency.
Analysis from this study suggested that the app reports can contribute to signal detection, and consequently that they are a valuable asset alongside other reporting mechanisms. Users of the app in the Netherlands are primarily new reporters that have not reported by conventional means. Out of the 52 first app reports, 43 came from new reporters and nine from previous reporters, based on the reporters e-mail address [22]. The fact that we obtained these reports through the app suggests that the reporter considered it the most convenient way of submitting it.
Because of the nature of analysis of spontaneous cases and differing signal detection methodologies, it cannot be determined whether a signal may have undergone evaluation irrespective of the inclusion of reports received via the app. We have examined whether app reports led to regulatory discussions about a potential safety issue, using the Good Pharmacovigilance Practice definition of a signal [23]. However, even if no regulatory action was deemed to be required, reports received via the app can contribute to evidence, which warrants further investigation.
4.1 Strengths and Limitations of the Study
The study used an identical data collection period for app and reference reports, and in this sample all app reports received were included. This means that if there are certain time-dependent reporting biases such as for example media attention to a certain ADR, the chance would be equal that this would be reported through either the app or through conventional means and not create a bias in the sample.
Another strength of the study is that the quality of the reports has been measured with two different methods. With vigiGrade, the technical completeness of the reports was evaluated and with the ClinDoc, the clinical quality was manually evaluated, making sure that two parameters representing the quality of the reports were included in the analysis.
A limitation of the study is the relatively low number of reports received through the app (n = 287). Because of the low number of reports, it is difficult to say how generalisable the results are. With a larger sample size, it would have been possible to draw firmer conclusions as to the characteristics of the reports and their contribution to signal detection. One specific concern is that insufficient numbers of reports were collected, particularly for Croatia, to statistically detect true differences between the two sources of reports. It is therefore important to continue promoting the use of the app and after more reports are collected, to redo this analysis to see if the conclusions drawn from this study are still valid. The low number of app reports in this study is not surprising because this is a new route of reporting and it will need time to become an integral part of the promotion of ADR reporting.
Clearly, app reports did not contribute to every signal investigated since the launch. However, the fact that they contributed to a number of signal evaluations, in the limited time since the apps were launched, shows their value as an additional reporting route amongst the range of pharmacovigilance tools that might be used to gather information on the safety of medicines. Of course, we cannot assess whether the reports would have been received by other routes had the apps not been available. However, the fact that the app was the preferred route of reporting for those individuals indicates that for at least part of the reporting community, the availability of reporting via mobile apps is helpful in the communication of suspected side effects.
5 Conclusion
New reporting tools such as the WEB-RADR app offer a complementary route of spontaneous reporting. Patient demographics are similar to conventional reporting routes, and report quality is sufficient despite a simplified reporting form.
The addition of new reporting methods is important to attract more reporters. Although the app report sample was small, the data show that the app has managed to attract patients as reporters to a larger relative proportion than conventional reporting routes, and that the app reports show potential in contributing to finding new signals.
References
Council for International Organizations of Medical Sciences. Practical aspects of signal detection in pharmacovigilance: report of CIOMS Working Group VIII. Geneva: Council for International Organizations of Medical Sciences; 2010.
Ishiguro C, Hall M, Neyarapally GA, Dal Pan G. Post-market drug safety evidence sources: an analysis of FDA drug safety communications. Pharmacoepidemiol Drug Saf. 2012;21(10):1134–6. https://doi.org/10.1002/pds.3317.
Pacurariu AC, Coloma PM, van Haren A, Genov G, Sturkenboom MCJM, Straus SMJM. A description of signals during the first 18 months of the EMA Pharmacovigilance Risk Assessment Committee. Drug Saf. 2014;37(12):1059–66. https://doi.org/10.1007/s40264-014-0240-1.
Raine JM. Risk management: a European regulatory view. In: Mann RD, Andrews EB, editors. Pharmacovigilance. 2nd ed. Chichester: Wiley; 2007. p. 553–8.
Mann RD, Andrews EB. Introduction. In: Mann RD, Andrews EB, editors. Pharmacovigilance. 2nd ed. Chichester: Wiley; 2007. p. 1–11.
Lopez-Gonzalez E, Herdeiro MT, Figueiras A. Determinants of under-reporting of adverse drug reactions. Drug Saf. 2009;32(1):19–31. https://doi.org/10.2165/00002018-200932010-00002.
Hazell L, Shakir SAW. Under-reporting of adverse drug reactions. Drug Saf. 2006;29(5):385–96. https://doi.org/10.2165/00002018-200629050-00003.
Carroll JK, Moorhead A, Bond R, LeBlanc WG, Petrella RJ, Fiscella K. Who uses mobile phone health apps and does use matter? A secondary data analytics approach. J Med Internet Res. 2017;19(4):e125. https://doi.org/10.2196/jmir.5604.
de Vries ST, Wong L, Sutcliffe A, Houÿez F, Ruiz CL, Mol PGM. Factors influencing the use of a mobile app for reporting adverse drug reactions and receiving safety information: a qualitative study. Drug Saf. 2017;40(5):443–55. https://doi.org/10.1007/s40264-016-0494-x.
Wilson K, Atkinson KM, Westeinde J, Bell C, Marty K, Fergusson D, et al. An evaluation of the feasibility and usability of a proof of concept mobile app for adverse event reporting post influenza vaccination. Hum Vaccin Immunother. 2016;12(7):1738–48. https://doi.org/10.1080/21645515.2016.1152434.
Bahk CY, Goshgarian M, Donahue K, Freifeld CC, Menone CM, Pierce CE, et al. Increasing patient engagement in pharmacovigilance through online community outreach and mobile reporting applications: an analysis of adverse event reporting for the Essure device in the US. Pharm Med. 2015;29(6):331–40. https://doi.org/10.1007/s40290-015-0106-6.
Bergvall T, Norén GN, Lindquist M. vigiGrade: A tool to identify well-documented individual case reports and highlight systematic data quality issues. Drug Saf. 2014;37(1):65–77. https://doi.org/10.1007/s40264-013-0131-x.
Oosterhuis I, Rolfes L, Ekhart C, Muller-Hansma A, Härmark L. First experiences with a tool to measure the level of clinical information present in adverse drug reaction reports. Expert Opin Drug Saf. 2017:1–5. https://doi.org/10.1080/14740338.2018.1400008.
Ghosh R, Lewis D. Aims and approaches of Web-RADR: a consortium ensuring reliable ADR reporting via mobile devices and new insights from social media. Expert Opin Drug Saf. 2015;14(12):1845–53. https://doi.org/10.1517/14740338.2015.1096342.
ICH Expert Working Group. Maintenance of the ICH guideline on clinical safety data management: data elements for transmission of individual case safety reports E2B(R2). 2001. Available from: http://www.ich.org/fileadmin/Public_Web_Site/ICH_Products/Guidelines/Efficacy/E2B/Step4/E2B_R2__Guideline.pdf. Accessed 17 Aug 2017.
Lindquist M. VigiBase, the WHO Global ICSR database system: basic facts. Ther Innov Regul Sci. 2008;42(5):409–19. https://doi.org/10.1177/009286150804200501.
Juhlin K, Star K, Norén GN. A method for data-driven exploration to pinpoint key features in medical data and facilitate expert review. Pharmacoepidemiol Drug Saf. 2017;26(10):1256–65. https://doi.org/10.1002/pds.4285.
Norén GN, Orre R, Bate A, Edwards IR. Duplicate detection in adverse drug reaction surveillance. Data Min Knowl Discov. 2007;14:305–28. https://doi.org/10.1007/s10618-006-0052-8.
Tregunno PM, Fink DB, Fernandez-Fernandez C, Lazaro-Bengoa E, Noren GN. Performance of probabilistic method to detect duplicate individual case safety reports. Drug Saf. 2014;37(4):249–58. https://doi.org/10.1007/s40264-014-0146-y.
Caster O, Juhlin K, Watson S, Norén GN. Improved statistical signal detection in pharmacovigilance by combining multiple strength-of-evidence aspects in vigiRank. Drug Saf. 2014;37(8):617–28. https://doi.org/10.1007/s40264-014-0204-5.
European Medicines Agency. Guideline on the use of statistical signal detection methods in the EudraVigilance data analysis system, Doc. Ref. EMEA/106464/2006 rev. 1. 2008. Available from: http://www.ema.europa.eu/docs/en_GB/document_library/Regulatory_and_procedural_guideline/2009/11/WC500011434.pdf. Accessed 17 Nov 2017.
Härmark L. Launching an app for patients and healthcare professionals in the Netherlands. In: Presentation presented at the DIA EuroMeeting, 6–8 Apr 2016, Hamburg.
European Medicines Agency. Guideline on good pharmacovigilance practices (GVP). Module IX: signal management (Rev 1). 2017. Available from: http://www.ema.europa.eu/docs/en_GB/document_library/Scientific_guideline/2017/10/WC500236408.pdf. Accessed 1 Dec 2017.
Acknowledgements
We thank Dr. Sebastian Monzon for his assistance and expertise in analysing reports in EudraVigilance and Dr. Tommy Carlsson for his valuable comments on this article. The authors are indebted to the national centres that contribute data to the World Health Organization Programme for International Drug Monitoring. However, the opinions and conclusions in this study are not necessarily those of the various centres nor of the World Health Organization.
Author information
Authors and Affiliations
Corresponding author
Ethics declarations
Funding
The research leading to these results was conducted as part of the WEB-RADR consortium (http://webradr.eu), which is a public-private partnership coordinated by the Medicines and Healthcare products Regulatory Agency. The WEB-RADR project has received support from the Innovative Medicine Initiative Joint Undertaking (http://www.imi.europa.eu) under Grant Agreement No. 115632, resources of which are composed of financial contributions from the European Union’s Seventh Framework Programme (FP7/2007-2013) and European Federation of Pharmaceutical Industries and Associations companies’ in-kind contributions.
Conflict of interest
Ingrid Oosterhuis, Henric Taavola, Philip M. Tregunno, Petar Mas, Sara Gama, Victoria Newbould, Ola Caster and Linda Härmark have no conflicts of interest that are directly relevant to the content of this study.
Electronic supplementary material
Below is the link to the electronic supplementary material.
Rights and permissions
Open Access This article is distributed under the terms of the Creative Commons Attribution-NonCommercial 4.0 International License (http://creativecommons.org/licenses/by-nc/4.0/), which permits any noncommercial use, distribution, and reproduction in any medium, provided you give appropriate credit to the original author(s) and the source, provide a link to the Creative Commons license, and indicate if changes were made.
About this article
Cite this article
Oosterhuis, I., Taavola, H., Tregunno, P.M. et al. Characteristics, Quality and Contribution to Signal Detection of Spontaneous Reports of Adverse Drug Reactions Via the WEB-RADR Mobile Application: A Descriptive Cross-Sectional Study. Drug Saf 41, 969–978 (2018). https://doi.org/10.1007/s40264-018-0679-6
Published:
Issue Date:
DOI: https://doi.org/10.1007/s40264-018-0679-6