Abstract
One of the main paradigms of Industry 5.0 is represented by human-robot collaboration (HRC), which aims to support humans in production processes. However, working entire shifts in close contact with a robotic system may introduce new hazards from a cognitive ergonomics perspective. This paper presents a methodological approach to monitor the evolution of the operator’s psychophysical state noninvasively in shifts of a repetitive assembly process, focusing on stress, mental workload, and fatigue. Through the use of non-invasive biosensors, it is possible to obtain objective information, even in real time, on the operator’s cognitive load and stress in a naturalistic manner (i.e., without interrupting or hindering the process). In the HRC setting, recognition of the operator’s psychophysical state is the first step in supporting his or her well-being and can provide clues to improve collaboration. The proposed method was applied to a case study aimed at comparing shifts performed both manually and with a cobot of a repetitive assembly process. The results showed significant differences in terms of process performance evolution and psychophysical state of the operator. In particular, the presence of the cobot resulted in fewer process failures, stress and cognitive load especially in the first phase of the work shift. The case study analyzed also showed the adequacy of noninvasively collected physiological data in providing important information on the evolution of the operator’s stress, cognitive load, and fatigue.
Similar content being viewed by others
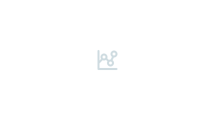
Avoid common mistakes on your manuscript.
1 Introduction
Human-robot collaboration (HRC) has spread as a new approach whereby humans and collaborative robots (also called cobots) work together in a shared environment. By sharing space, time and goals, HRC may leverage the strengths of both parties to achieve better results productivity and quality performances [1,2,3]. In manufacturing, collaborative robotics mainly finds application in assembly processes. In a collaborative assembly process, tasks are allocated between humans and cobots, working symbiotically to assemble a product [4, 5]. The main issues present in an assembly process involve not only physical ergonomics and operator safety, but also cognitive aspects. Optimization of cognitive ergonomics may enable reduction of errors, improvement of performance and facilitation of operators’ decision-making process [6]. Collaborative robotics has the potential to provide not only physical but also cognitive support to the operator.
Extensive research has shown that HRC encompasses a wide variety of dimensions, including human factor [7]. Fatigue and stress are gaining increasing attention as they may result in poor performances of operators in manufacturing processes, as well as impacting their well-being. For this reason, it is important to be able to monitor the psychophysical state of operators by obtaining information noninvasively and without hindering their work [8]. In addition, the implementation of cobots may improve both quality of the production system and humans’ well-being, but to date the study of the effects of HRC in repetitive assembly processes has received limited attention [9, 10].
This paper aims to explore the evolution of the psychophysical state (focusing on stress, cognitive load, and fatigue) and performance of operators during continuous interaction with a collaborative robot in a repetitive assembly process. In addition, a naturalistic and noninvasive approach to gather information about the operator’s psychophysical state is also emphasized.
An experimental setting involving 4 h assembly work-shifts of a case-study product (i.e., a tile cutter) was designed and implement. The assembly work-shifts were performed both manually and with the support of a cobot, in order to highlight potential effects of HRC. Three main aspects were analyzed:
-
i.
Number and type of process failures, to address the evolution of process quality and humans’ performance over time.
-
ii.
Physiological responses (i.e., electrodermal activity and heart rate variability) collected noninvasively, to measure stress, cognitive load, and fatigue experienced in prolonged repetitive assembly processes.
-
iii.
Relationship between process failures and physiological responses, to explore the influence of process failures on operator psychophysical state.
This paper is organized as follows. Section 2 presents a literature review on HRC in industrial contexts, providing also details on the use of physiological data. In Sect. 3 the experimental methodology proposed and adopted is described in detail. Section 4 shows the main results, analyzing process failures and physiological responses. The main findings are summarized and discussed in Sect. 5. Finally, Sect. 6 contains conclusions and future works.
2 Literature review
HRC has received considerable attention in recent years. HRC involves humans and robots sharing the same workspace and time in order to pursue a common goal and work simultaneously [11]. The great potential of this technology lies in the possibility to combine the dexterity of the human operator with the repeatability and precision of the robot [1, 2]. However, the coexistence of humans and cobots in the same workspace has raised several safety concerns thus leading to a wide variety of methodologies to improve it [12,13,14]. In this regard, ISO standards (i.e., ISO 10218-2:2011 [15] and ISO/TS 15066:2016 [16]) were also established to ensure safe operations in human-robot collaboration tasks. The main safety hazards involved in HRC include physical contact and collision, pinch points and trapping, robot speed and force. The most commonly used strategies to mitigate these hazards involve limiting robot speed and force, implementing proximity sensors or vision systems to avoid collisions, or integrating corrective actions systems that lead to collision avoidance without stopping the robot operation [17, 18].
Safety represents only one of the variables that can impact the efficiency of a collaborative assembly process. Gervasi et al. [7] introduced a framework summarizing several critical dimensions to be considered in evaluating HRC (i.e., autonomy, information exchange, team organization, adaptivity and training, task, human factors, ethics and cybersecurity). A multidimensional approach is also followed by Hoffman [19] who proposed a methodology for assessing HRC fluency by making use of a set of objective metrics (human idle time, robot idle time, concurrent activity) and other subjective metrics related to human perception of the cobot. Kokotinis et al. [20] recently proposed a tool for evaluating HRC quality, based on metrics related to human (e.g., safety, ergonomics, and trust), robot (e.g., autonomy, manipulation ability, and costs), and interaction (e.g., interaction ability, workspace and time sharing).
In the field of HRC evaluation, there is a growing interest in the analysis of cognitive aspects and affective states involved, with special attention to stress and mental workload [9, 21, 22]. To enhance quality, Ahmed et al. [23] highlighted the importance of controlling both human and process parameters and their influence on assembly quality. A similar approach was followed by Quenehen et al. [24] who assessed quality of different human-robot collaboration modes through an energy expenditure model taking into account economic and ergonomic indicators. Some classic metrics used in case studies are based on participants’ performance, such as the number of errors, occurrence of conflicts, task completion rate, and response time [25]. Another method is to obtain subjective feedback through questionnaires and surveys, mainly focused on reporting perceived workload, anxiety, pleasantness, situation awareness, and so on. Some of the most widely used tools in this area include the System Usability Scale (SUS) [26], Self-Assessment Manikin (SAM), NASA-TLX [27], Subjective Workload Assessment Technique (SWAT) [28], and Instantaneous Self-Assessment of workload (ISA) [29]. Compared to performance-based metrics, the use of questionnaires or surveys is particularly suitable in evaluating the interaction with a robot in the case of tasks with a qualitative nature and rather limited duration [30]. However, a limitation is their use in contexts where there is interest in analyzing the evolution of the user experience in more complex and longer duration tasks [31]. In fact, in these cases, obtaining subjective feedback would imply task interruption, which can potentially undermine the naturalistic setting of an experiment. One way to circumnavigate this obstacle is to collect and analyze physiological data from users.
2.1 Physiological data analysis in HRC
In recent years, the exploitation of physiological data to obtain objective information concerning cognitive activity and affective state of users involved in HRC has received increasing attention. [8, 32]. Khamaisi et al. [33] developed a comprehensive approach to identify potential stressful situation for workers, combining questionnaires, operators’ feedback and the analysis of psycho-physiological responses. Similarly, Gervasi et al. [34] explored how different robot configurations may impact user experience collecting also physiological response.
It is possible to derive information about a user’s psychophysical state through the analysis of physiological parameters such as heart rate [35, 36], blood pressure [37], skin conductance [38, 39], respiratory rate [40], pupil dilation [41], electroencephalography (EEG) [42], and electromyography (EMG) [30].
The collection of physiological data is accomplished using biosensors that are usually applied to humans. Especially in manufacturing, the implementation of noninvasive wearable biosensors proves to be crucial in order to obtain information about the operator’s state [31]. This would also allow to not hinder the operator during dynamic processes and to maintain as naturalistic as possible the data collection setting.
Heart activity and electrodermal activity (EDA) are among the physiological parameters most commonly used in HRC to monitor cognitive activity and stress and with high potential for real-world application in manufacturing settings [8]. This potential stems mainly from (i) the widespread of noninvasive wearable biosensors (e.g., wristbands) for monitoring heart activity and EDA, (ii) a not-particularly high difficulty in analyzing and interpreting their signals, and (iii) a relatively low implementation cost.
Heart monitoring is a common method used in fitness and working contexts for assessing user experience [43]. It is quite easy and cheap to implement and can be performed by electrocardiography (ECG) or photopletismography (PPG) (Fig. 1). Heart rate is regulated by sympathetic and parasympathetic inputs and can vary according to the body’s physical needs [44]. It is also modulated by numerous factors, such as stress, psychological state, physical activity, medications, hormonal status, and illness. Heart rate variability (HRV) represents the variation in the time interval between heartbeats (called R-R intervals) and has been used in several studies to objectively assess psychological stress [45]. HRV is reported to be an index of the influence of both the parasympathetic nervous system (PSNS) and the sympathetic nervous systems (SNS). PSNS is referred to as the part of the nervous system responsible for “rest and digest” activities, i.e., responsible for the internal functions when resting and relaxing [46]. PSNS has opposite functions to those of the SNS, basically undoing the work of SNS after a stressful situation. SNS is the division of the autonomic nervous system that mediates the neuronal and hormonal response to stress and arousal, commonly known as the “fight or flight” response [47].
Regarding heart activity, SNS stimulation leads to an increase in blood pressure and heart rate, while PSNS stimulation leads to a decrease in them. In healthy individuals, high resting HRV indicates balanced activation of the SNS and the PSNS [44]. This variability, indeed, reflets the ability of the cardiovascular system to rapidly adjust and cope with uncertainty and sudden environmental changes [45]. During acute stress, activity of the SNS increases and the PSNS is suppressed, leading to an imbalance that leads to a lower HRV. Upon dissipation of the stress source, HRV returns to the initial state of balanced activation.
EDA, sometimes also known as galvanic skin response or skin conductance, refers to the measurement of the continuous changes in the electrical conductance (i.e., capacity to conduct a flow of electrical current) of the skin in response to sweat secretion by the eccrine sweat glands [48].
Example of electrocardiography (ECG) or photopletismography (PPG) signals with R-R intervals [49]
The eccrine sweat glands (Fig. 2a) have a secretory segment (located in the skin hypodermis) innervated by sudomotor fibers connected to the SNS. Upon activation of the SNS, the sweat gland duct fills with sweat, which in turn increases skin conductance due to ions contained in sweat. Therefore, EDA is considered a marker of SNS activity, which is related to stress and arousal [48].
Changes in EDA can be decomposed in short term (phasic) or relatively long lasting (tonic) (Fig. 2b) [50]. Phasic component reflects rapid changes in the EDA within a few seconds, called skin conductance responses (SCRs), resulting from an underlying sympathetic reaction. These reactions can be induced by external stimuli, e.g., stressors. Therefore, their analysis can provide information on the level of arousal and stress. Tonic component, also called skin conductance level (SCL), corresponds to slow changes in the EDA resulting from the tonic activity of sympathetic innervation of the eccrine sweat gland. Prolonged stress and increase of cognitive load are typically associated to a slow increase of EDA, i.e., an increase of SCL.
3 Methodology
In this section, a methodology is proposed to noninvasively study the user’s experience and psychophysical state (i.e., stress, cognitive load, and fatigue) during shifts in an assembly process. In order to investigate also the potential support of a cobot in repetitive assembly processes, 4 h shifts of a tile cutter assembly process were designed and implemented at the “Politecnico di Torino” at Mind4Lab [52]. The study involved twelve participants (six males and six females) between the ages of 20 and 25 who had no prior experience with cobots.
A more detailed description of the methodology and assembly process will follow in the next sections.
3.1 Case study: tile cutter assembly process
Participants were asked to assemble repetitively a tile cutter. Each participant performed the assembly process in two 4 h sessions, one in a manual setting (Manual) and the other in conjunction with a cobot (HRC). The order of the two modalities was randomized for each participant. In HRC modality, the UR3e cobot [53] was used. Participants were briefed on the cobot’s actions and automatic emergency stop on impact (i.e., power and force limiting safety system). Figure 3a and b show the final assembled product and the components, respectively. Figure 4 shows the assembly workstation with the UR3e cobot and supports for the assembly. Table 1 contains the list of the operations for both the HRC and Manual modalities and shows the agent performing them. The assembly of the tile cutter can be broken down into 4 macro-phases:
-
1.
The assembly of the base holders.
-
2.
The assembly of the cutting mechanism.
-
3.
The insertion of rail rods into the cutting mechanism and the joining with the base.
-
4.
Completion of the tile cutter screwing the handle.
The assembly of a tile cutter lasted about 240s, leading to the production of about 60 products in a 4 h shift. Overall, considering all the participants who joined the experimental campaign, a total of 720 tile cutter were assembled.
a Tile cutter and b components of the tile cutter with their tags [52]
3.2 Process failures
In order to investigate the evolution of human operator’s performance over time and the contextual learning rate, the occurrence and type of process failures caused by humans were collected. In this regard, Table 2 contains a taxonomy of possible human process failures for the assembly process under analysis. Four main macro-groups of human process failures were identified:
-
Incorrect part selection (HF1): the operator selects a component, screw, nut, or washer that is not required to perform the subsequent task.
-
Incorrect part positioning (HF2): the operator positions a component, screw, nut, or washer in an unsuitable way, making it difficult or even impossible to perform the following task.
-
Incorrect part assemblies (HF3): the operator incorrectly assembles different components.
-
Part droppings (HF4): the operator accidentally drops a component, screw, nut, washer, or tool.
To investigate potential differences between manual and collaborative assemblies in terms of learning rate, the learning curve of human process failures will be analyzed. Learning curves have been widely addressed in manufacturing-related literature. Different learning models were proposed over the years, and one of the most common is the power law learning curve [54, 55]. In this study, a power law learning model will be implemented to investigate the evolution of process failures caused by humans in both modalities. The aforementioned learning model can be expressed as follows:
where \(a\) and \(b\) are the model coefficients and represent the starting performance and learning rate, respectively, while \(c\) represents the asymptotic steady-state [56, 57]. In this paper, it was assumed \(c=0\), since: (i) there are no external conditions preventing the operator from reaching the 0-failure scenario, (ii) the number of trials is limited, leading to the well-known Wright’s model [58]. In our case, the lower \(b\) is, the faster the learning and consequently the achievement of zero failures. \(Y\) is the response variable, i.e., the process failures. \(Trial\) represents the number of trials performed by the operator. The R package “stats” [59, 60] was used for fitting learning models thought nonlinear least squares [61] and calculating confidence intervals for the coefficient estimates.
In the HRC setting, the occurrence of robot failures was tracked. Possible types of failures of the cobot include missed or incorrect grasping of a component, dropping a component during movement, colliding with objects or operator, and system errors/blocks. This kind of information may provide clues to the operator’s user experience in the HRC, and their relationship to physiological responses will be explored.
3.3 Physiological signal collection
The noninvasive biosensor Empatica E4 [62] was used to collect data on various physiological responses of participants. Physiological data were then analysed to investigate the evolution of stress, fatigue and cognitive exertion of human operators performing repetitive assembly processes. The device records two types of physiological information: EDA data at 4 Hz and heart rate data through PPG at 64 Hz. From PPG, R-R intervals were obtained, and the Root Mean Square of Successive Differences of R-R intervals (RMSSD) was used as HRV index due to its common use as stress and fatigue indicator [63].
“Ledalab”, a MATLAB-based software, was used to process EDA data. Through Continuous Decomposition Analysis (CDA) [64], the EDA signal was decomposed into phasic and tonic activity signals. Through the analysis of tonic activity (i.e., SCL), information on cognitive load evolution can be obtained. In contrast, through the analysis of the phasic activity signal, skin conductance responses (SCRs) (i.e., amplitude changes from the SCL to a peak of the response) can be identified providing information on arousal and stress.
In this study, for each trial, average SCR, SCL and RMSSD were computed to investigate the evolution of stress, cognitive load, and fatigue [65, 66].
3.4 Experimental procedure
At the beginning, each group of participants was briefed about the objectives and the detailed procedure of the experimental study. Secondly, participants were conducted to their work-area where they were given detailed information of the assembly process to perform. Meanwhile, the participant in charge of assembly was equipped with the Empatica E4 on the left wrist and a 15 min wait period was observed to ensure an appropriate adherence of the electrodes, so as to acquire reliable EDA data. Next, the participant was asked to relax and stand still to record 2 min of “baseline”, i.e., the physiological signals at rest. Next, the repetitive assembly process was randomly selected in one of the two modalities (i.e., Manual or HRC) and began for a 4 h work-shift. A 10 min break was also scheduled after 2 h of work, in order to simulate real-life working conditions. The other two participants monitored the whole process by recording process failures and product defects. At the end of the 4 h work-shift, general unstructured feedbacks on the experiment were collected. In the second shift, the same procedure was followed for the remaining modality (i.e., HRC or Manual). Therefore, each group of participants performed the 4 h work shift in both modalities with random order.
4 Results
In this section, the obtained experimental results are presented.
4.1 Process failures
Figure 5 shows the evolution of the average number across participants of human process failures for both Manual and HRC settings. Slightly more failures were present in the Manual setting, although there was some overlap between the curves. Figure 6 shows the comparison of the fitted learning curves with the power law model for each modality. The learning curve for the manual modality was found to be above that of the HRC modality, highlighting the tendency to observe more process failures in the Manual setting. This phenomenon provides clues on the cognitive support of the cobot for the operator during assembly. In fact, participant feedback revealed that the cobot, indirectly with its operations, helped the operator in remembering the various assembly steps, thus making fewer mistakes.
Table 3 contains the parameter estimates of the power-law learning curves. It can be seen that the two learning curves have a rather similar learning rate (HRC: \(b\) = − 0.3889; Manual: \(b\) = − 0.3558), while the initial value of average process failures is higher in the manual setting (HRC: \(a\) = 0.9398; Manual: \(a\) = 1.1444). However, it was not possible to conclude that the difference was statistically significant since the 95% confidence intervals overlap.
Figure 7 shows the evolution of average across participants human process failures categorized into incorrect part selections (HF1), incorrect part positionings (HF2), incorrect part assemblies (HF3), and part droppings (HF4). No significant differences emerged between Manual and HRC modalities, except in incorrect part positionings. Observing the curves, more incorrect part positionings can be noted in the Manual setting. Several failures of this kind were observed during the assembly of the cutting mechanism. One of the main difficulties encountered by the participants was to correctly position the components of the cutting mechanism. Since the cobot always presented and held the C2 component in the same way, it was easier for the operator to remember how to correctly position the other components. This effect is also reflected by the coefficients of the learning curves (Table 4), where the starting performance was better in the HRC modality (HRC: \(a\) = 0.3375; Manual: \(a\) = 0.7172). In addition, this difference was statistically significant as there was no overlap between the confidence intervals. The learning rate in the Manual setting had a slightly higher magnitude (HRC: \(b\) = − 0.6073; Manual: \(b\) = − 0.5834) to compensate for the higher initial gap, however, the difference with the HRC setting was not significant when observing the confidence intervals.
4.2 Physiological response
In order to compare physiological response, data were standardized for each participant as physiological signals may be influenced by personal characteristics. The following formula was used to obtain the z-scores:
where \({z}_{ij}\) and \({x}_{ij}\) are respectively the i-th z-score and observation of participant j, \({\overline{x}}_{j}\) the sample mean for participant j, and \({s}_{j}\) the sample standard deviation for participant j. To check for significant differences between the Manual and HRC setting the paired t-test was implemented, as the normality assumption was not rejected by the Shaipiro-Wilks [67] test for each response variable.
Figure 8a shows the evolution of the average SCR across participants over the work shift, comparing the Manual and HRC settings. It can be noted that in general the Manual setting higher values were observed, implying higher stress for participants. This difference was also found to be highly significant by the paired t-test (p < 0.001). Observing the trend of the average SCR, the Manual setting shows a decreasing trend while the HRC setting an increasing one. Initially in the Manual setting the stress was quite high, mainly due to a learning phase of the task, while in the HRC setting it tended to be lower. This was due to the cobot providing cognitive support to the participants, helping them to more easily remember the assembly steps and how to join certain parts through its operations. The observed difference in terms of stress resulted highly significant in the first half of the shift (p < 0.001). However, after about half of the shift, the difference between the two settings narrowed until it was no longer significant (p = 0.428). The increase in stress in the HRC setting may have been caused by the fact that the cobot began to be perceived also as a limitation for the operator in the second part of the shift. Several participants wished that cobot could be faster as they had to wait for the cobot concluding its operations to continue with the process, consequently reducing the control of the task time.
Figure 8b contains the evolution of the average SCL across participants over the trials for both the Manual and HRC settings. Overall, in the Manual setting slightly higher values were observed, revealing a significant difference by the paired t-test (p = 0.0155). It is interesting to note a different behavior of the average SCL between the first and second part of the shift. In the first part, significantly higher SCL values were observed in the Manual setting (p < 0.001), highlighting a greater prolonged use of cognitive resources due mainly to having to learn the assembly process. Interestingly, significantly lower values were observed in the HRC setting in this phase, providing additional evidence of the cognitive support of the cobot. However, as the trials progressed, the average SCL tended to increase in the HRC setting, slightly exceeding the values in the Manual setting in the second part of the shift (p = 0.0223). This phenomenon points to a gradual increase in prolonged cognitive stress mainly due to having to adjust to the pace of the cobot when faster or simultaneous actions would have been welcome once the operator became familiar with the assembly process.
Figure 9 shows the evolution of the average RMSSD across the trials, comparing the HRC and Manual settings. Both curves show an increasing trend, indicating a gradual reduction in initial stress mainly resulting from the learning phase of the task. In addition, the two curves tend to overlap considerably across trials, implying no significant difference between the two modalities in terms of HRV by the paired t-test (p = 0.071). Even focusing only on the first and second part of the shift, no significant difference emerged (p = 0.092 and p = 0.370 respectively), although a slight decrease in the average RMSSD can be noted toward the end of the shift for the HRC setting.
4.3 Relationship between process failures and physiological response
In this section, the relationships between process failures and physiological response are explored. Figure 10 shows the Pearson’s coefficients (ρ) obtained for both Manual and HRC settings, as well as the significance level of each coefficient obtained through a t-test. A high magnitude of Pearson’s coefficient indicates a strong correlation between the two variables, while if the value is close to zero the correlation is very low.
In the Manual setting, it can be seen that significant correlations emerged only with EDA. The occurrence of failures by humans generally resulted in a consistent increase in SCR (ρ = 0.56) (Fig. 11). This means that process failures caused rather rapid sympathetic reactions, leading to increased stress on the part of the operator. Observing the correlations with the different types of human failures, incorrect positioning (HF2) and assembly (HF3) contributed significantly to the increase in SCR (ρ = 0.46 and ρ = 0.28, respectively). Incorrect selection also resulted in an increase in SCR, although it was not found significant. This effect is related to the realization of having commit a process failure, which can cause a rapid reaction (i.e., sympathetic activation) in the operator, thereby increasing his/her stress. The occurrence of failures by humans resulted also in a slight significant increase in SCL (ρ = 0.31), i.e., an increase in the cognitive load. Looking at the correlations between the different types of human failures, the incorrect assembly (HF3) was the one that most affected SCL (ρ = 0.22).
In fact, once realized the incorrect assembly of a component, figuring out how to remedy requires a certain amount of mental resources that is reflected in the increase of SCL.
In the HRC setting, no significant correlations emerged between process failures and physiological responses (Figs. 10 and 11). Neither process failures resulting from the cobot seemed affect the users’ physiological response. This fact suggests that in the HRC setting the observed increases in stress, cognitive load, and fatigue may not be attributable to process failures, but may arise, for example, from the process itself. Thus, the presence of the cobot could mitigate the negative effects on the operator due to process failures. From previous sections, it should be noted that significantly lower mean SCR and mean SCL were observed in the HRC setting than in the Manual one, especially in the learning phase in which the greatest number of failures were found. These results put even more emphasis on the cognitive support of the cobot in processes and suggests that the physiological response trends observed for the HRC setting can be attributed to a natural process of operator fatigue during the shift.
5 Discussion
In the case study analyzed, concerning a repetitive assembly process, interesting differences emerged in terms of process failures and physiological responses of the operator between the Manual and HRC setting.
Analysis of the evolution of human process failures revealed highly significant differences between the two settings. In particular, there were generally significantly fewer failures in the HRC setting, especially in the learning phase. From the detailed analysis of the types of human failures, it was found that the cobot provided significant support from the cognitive point of view especially in avoiding incorrect part positionings. In fact, the participants’ feedback showed that the cobot’s actions helped to remember the various assembly steps especially at the beginning. In addition, the fact that the cobot always handed the components in the same way created less uncertainty about the correct positioning and assembly of the components. From a production rate perspective, Manual and HRC settings were comparable.
Regarding the psychophysical state of operators, the information obtained from EDA proved most useful in bringing out significant differences between the Manual and HRC settings. The presence of the cobot resulted in significantly lower values of SCR and SCL, especially in the first part of the work shift. Initially, indeed, in the Manual setting the average SCR was quite high, which highlights a distinctly high stress resulting mainly from learning the task. In contrast, significantly lower values were observed in the HRC setting, highlighting greater relaxation on the behalf of the operator in the learning phase. These results further corroborate the cognitive support role of the cobot in manufacturing assembly processes. As the trials progressed, there was a gradual increase in SCR in the HRC setting, although reaching values in the average range and similar to those found in the Manual setting. In contrast, a fairly significant increase was shown in the SCL, leading to values higher than the Manual setting toward the end of the shift. This phenomenon also emerged in the RMSSD analysis, although it was not significant. The increased demand for cognitive resources at this stage is due to a process of fatigue and slight frustration of the participants. In fact, toward the end of the shift several participants would have liked to be even more efficient desiring the cobot to be faster in performing its operations. This feedback shows the need to allow operators to customize and adapt HRC according to their needs in order to ensure an optimal and fully profitable experience with such technology.
Finally, relating process failures and physiological responses revealed further interesting differences between the HRC and Manual setting. In the Manual setting, the occurrence of human failures resulted in a negative effect on the psychophysical state of the operator, translating into a consistent increase in SCR (i.e., stress) and a slight increase in SCL (i.e., cognitive load). Surprisingly, these effects were not significant in the HRC setting. This result suggests that the presence of the cobot may also help to contain potential negative effects on the operator from process failures. In addition, the observed stress and cognitive load may therefore result from other factors, such as the configuration of the interaction with the cobot [34].
Other assistive technologies, such as augmented reality (AR) or artificial intelligence (AI) based vision systems, can also provide cognitive support to the human operator, contributing to the reduction of failures in the context of assembly processes. These tools are particularly suitable for guiding the operator in real time, however they can burden mental load of operators. Collaborative robots provide both physical and implicit cognitive support to the operator, and the integration of other assistive technologies can enable the provision of more information. However, having to manage and process additional information can increase the operator’s mental load especially on prolonged processes. Further investigation of this topic in terms of user experience is needed.
Finally, implementation of HRC systems can provide a favorable return of investment (ROI) over time, particularly when they lead to substantial reductions in quality errors and defects, increased production efficiency, and improved product consistency [68]. However, it is important to note the ROI is highly dependent on the application [69]. Form a human-centered perspective, the implementation of HRC can relieve operators from repetitive or highly physically demanding tasks. This would allow operators to focus on more complex, value-added tasks that require problem-solving and decision-making skills, potentially leading to productivity gains and greater satisfaction.
6 Conclusions
This paper aimed to propose a methodology to obtain information on the performance and psychophysical state of operators in a continuous and noninvasively during entire shifts in an HRC production process. An implementation of this methodology was shown in a case study of a repetitive assembly process of a tile cutter, aimed at comparing shifts performed in Manual and HRC modality in terms of physiological response and process failure evolution.
In terms of performance, the use of the cobot implied a significant reduction in human failures, such as incorrect part positionings or assemblies, highlighting the cognitive support of the cobot. This role was further investigated and confirmed through the analysis of physiological responses, which showed a significant reduction in stress and cognitive load especially in the first half of the work shift. By also relating process failures to physiological responses, it was observed that in the Manual setting the occurrence of failures resulted in a significant increase in stress and operator cognitive load. However, these relationships did not emerge significant in the HRC setting, suggesting that the observed physiological responses may result from other factors, such as the configuration of the interaction with the cobot. This result contributes to the need to develop collaborative robotic systems that can meet operator preferences in order to fully exploit the supportive role of this technology.
Future work will focus on exploring and implementing other noninvasive biosensors to assess their added value on understanding the operator’s state. In addition, future work will also focus on developing systems that can process real-time information about the operator’s state to support the operator in personalizing HRC.
Data availability
The datasets generated and analysed during this study are not currently publicly available.
Abbreviations
- ECG:
-
Electrocardiogram
- EDA:
-
Electrodermal activity
- EEG:
-
Electroencephalography
- HRC:
-
Human-robot collaboration
- HRV:
-
Heart rate variability
- PPG:
-
Photopletismogram
- PSNS:
-
Parasympathetic nervous system
- SCL:
-
Skin conductance level
- SCR:
-
Skin conductance response
- SNS:
-
Sympathetic nervous system
References
Bauer A, Wollherr D, Buss M (2008) Human–robot collaboration: a survey. Int J Humanoid Robot 05:47–66. https://doi.org/10.1142/S0219843608001303
Villani V, Pini F, Leali F, Secchi C (2018) Survey on human–robot collaboration in industrial settings: safety, intuitive interfaces and applications. Mechatronics 55:248–266. https://doi.org/10.1016/j.mechatronics.2018.02.009
Colgate JE, Wannasuphoprasit W, Peshkin MA (1996) : Cobots: robots for collaboration with human operators. In: Proceedings of the 1996 ASME international mechanical engineering congress and exposition. pp. 433–439
Wang L, Gao R, Váncza J, Krüger J, Wang XV, Makris S, Chryssolouris G (2019) Symbiotic human-robot collaborative assembly. CIRP Ann 68:701–726. https://doi.org/10.1016/j.cirp.2019.05.002
Lippi M, Marino A (2021) Human multi-robot physical interaction: a distributed framework. J Intell Robot Syst 101:35. https://doi.org/10.1007/s10846-020-01277-y
Brolin A, Thorvald P, Case K (2017) Experimental study of cognitive aspects affecting human performance in manual assembly. Prod Manuf Res 5:141–163. https://doi.org/10.1080/21693277.2017.1374893
Gervasi R, Mastrogiacomo L, Franceschini F (2020) A conceptual framework to evaluate human-robot collaboration. Int J Adv Manuf Technol 108:841–865. https://doi.org/10.1007/s00170-020-05363-1
Gervasi R, Barravecchia F, Mastrogiacomo L, Franceschini F (2023) Applications of affective computing in human-robot interaction: state-of-art and challenges for manufacturing. Proc Inst Mech Eng Part B J Eng Manuf 237:815–832. https://doi.org/10.1177/09544054221121888
Gualtieri L, Fraboni F, De Marchi M, Rauch E (2022) Development and evaluation of design guidelines for cognitive ergonomics in human-robot collaborative assembly systems. Appl Ergon 104:103807. https://doi.org/10.1016/j.apergo.2022.103807
Gervasi R, Capponi M, Mastrogiacomo L, Franceschini F (2023) Manual assembly and human–robot collaboration in repetitive assembly processes: a structured comparison based on human-centered performances. Int J Adv Manuf Technol. https://doi.org/10.1007/s00170-023-11197-4
Krüger J, Lien TK, Verl A (2009) Cooperation of human and machines in assembly lines. CIRP Ann 58:628–646. https://doi.org/10.1016/j.cirp.2009.09.009
Zanchettin AM, Ceriani NM, Rocco P, Ding H, Matthias B (2016) Safety in human-robot collaborative manufacturing environments: metrics and control. IEEE Trans Autom Sci Eng 13:882–893. https://doi.org/10.1109/TASE.2015.2412256
Robla-Gómez S, Becerra VM, Llata JR, González-Sarabia E, Torre-Ferrero C, Pérez-Oria J (2017) Working together: a review on safe human-robot collaboration in industrial environments. IEEE Access 5:26754–26773. https://doi.org/10.1109/ACCESS.2017.2773127
Liu H, Qu D, Xu F, Du Z, Jia K, Song J, Liu M (2022) Real-time and efficient collision avoidance planning approach for safe human-robot interaction. J Intell Robot Syst 105:93. https://doi.org/10.1007/s10846-022-01687-0
ISO 10218-2:2011 (2011) Robots and robotic devices — safety requirements for industrial robots — part 2: robot systems and integration. Presented at the, Geneva, CH
ISO/TS 15066 (2016) :: Robots and robotic devices — collaborative robots. In: ISO., Geneva, CH (2016)
Michalos G, Makris S, Tsarouchi P, Guasch T, Kontovrakis D, Chryssolouris G (2015) Design considerations for safe human-robot collaborative workplaces. Procedia CIRP 37:248–253. https://doi.org/10.1016/j.procir.2015.08.014
Tsarouchi P, Makris S, Chryssolouris G (2016) Human–robot interaction review and challenges on task planning and programming. Int J Comput Integr Manuf 29:916–931. https://doi.org/10.1080/0951192X.2015.1130251
Hoffman G (2019) Evaluating fluency in human–robot collaboration. IEEE Trans Hum Mach Syst 49:209–218. https://doi.org/10.1109/THMS.2019.2904558
Kokotinis G, Michalos G, Arkouli Z, Makris S (2023) On the quantification of human-robot collaboration quality. Int J Comput Integr Manuf. https://doi.org/10.1080/0951192X.2023.2189304
Gervasi R, Mastrogiacomo L, Franceschini F (2023) An experimental focus on learning effect and interaction quality in human–robot collaboration. Prod Eng 17:355–380. https://doi.org/10.1007/s11740-023-01188-5
Faber M, Mertens A, Schlick CM (2017) Cognition-enhanced assembly sequence planning for ergonomic and productive human–robot collaboration in self-optimizing assembly cells. Prod Eng 11:145–154. https://doi.org/10.1007/s11740-017-0732-9
Ahmed A, Guozhu J, Salam A, Nawaz MK (2019) : Reliability and quality control approach for collaborative assembly process. In: Proceedings of 2019 16th international bhurban conference on applied sciences and technology, IBCAST 2019. pp. 210–217
Quenehen A, Thiery S, Klement N, Roucoules L, Gibaru O (2020) Assembly process design: performance evaluation under Ergonomics consideration using several robot collaboration modes. IFIP Adv Inf Commun Technol 592 IFIP:477–484. https://doi.org/10.1007/978-3-030-57997-5_55
Steinfeld A, Fong T, Kaber D, Lewis M, Scholtz J, Schultz A, Goodrich M (2006) : Common metrics for human-robot interaction. In: Proceedings of the 1st ACM SIGCHI/SIGART conference on Human-robot interaction. pp. 33–40. Association for Computing Machinery, New York, NY, USA
Brooke J (1996) SUS—a quick and dirty usability scale. In: Jordan P, Thomas B, Weerdmeester B, McClelland I (eds) Usability evaluation in industry. CRC Press, London, pp 189–194
Hart SG, Staveland LE (1988) Development of NASA-TLX (task load index): results of empirical and theoretical research. In: Hancock PA, Meshkati N (eds) Advances in psychology. North-Holland, pp 139–183
Reid GB, Nygren TE (1988) The subjective workload assessment technique: a scaling procedure for measuring mental workload. In: Hancock PA, Meshkati N (eds) Advances in psychology. North-Holland, pp 185–218
Jordan CS, Brenner SD (1992) Instantaneous self-assessment of workload technique (ISA). Defence Research Agency, Portsmouth
Dehais F, Sisbot EA, Alami R, Causse M (2011) Physiological and subjective evaluation of a human–robot object hand-over task. Appl Ergon 42:785–791. https://doi.org/10.1016/j.apergo.2010.12.005
Peruzzini M, Grandi F, Pellicciari M (2017) Benchmarking of tools for user experience analysis in industry 4.0. Procedia Manuf 11:806–813. https://doi.org/10.1016/j.promfg.2017.07.182
Prati E, Peruzzini M, Pellicciari M, Raffaeli R (2021) How to include user eXperience in the design of human-robot interaction. Robot Comput Integr Manuf 68:102072. https://doi.org/10.1016/j.rcim.2020.102072
Khamaisi RK, Brunzini A, Grandi F, Peruzzini M, Pellicciari M (2022) UX assessment strategy to identify potential stressful conditions for workers. Robot Comput Integr Manuf 78:102403. https://doi.org/10.1016/j.rcim.2022.102403
Gervasi R, Aliev K, Mastrogiacomo L, Franceschini F (2022) User experience and physiological response in human-robot collaboration: a preliminary investigation. J Intell Robot Syst 106:36. https://doi.org/10.1007/s10846-022-01744-8
Dehais F, Causse M, Vachon F, Tremblay S (2012) Cognitive conflict in human–automation interactions: a psychophysiological study. Appl Ergon 43:588–595. https://doi.org/10.1016/j.apergo.2011.09.004
Kühnlenz B, Kühnlenz K (2016) Reduction of heart rate by robot trajectory profiles in cooperative HRI. In: Proceedings of ISR 2016: 47st international symposium on robotics. pp 1–6
Attarchi M, Dehghan F, Safakhah F, Nojomi M, Mohammadi S (2012) Effect of exposure to occupational noise and shift working on blood pressure in rubber manufacturing company workers. Ind Health 50:205–213. https://doi.org/10.2486/indhealth.MS1321
Kulić D, Croft E (2007) Physiological and subjective responses to articulated robot motion. Robotica 25:13–27. https://doi.org/10.1017/S0263574706002955
Arai T, Kato R, Fujita M (2010) Assessment of operator stress induced by robot collaboration in assembly. CIRP Ann 59:5–8. https://doi.org/10.1016/j.cirp.2010.03.043
Argyle EM, Marinescu A, Wilson ML, Lawson G, Sharples S (2021) Physiological indicators of task demand, fatigue, and cognition in future digital manufacturing environments. Int J Hum Comput Stud 145:102522. https://doi.org/10.1016/j.ijhcs.2020.102522
Marinescu AC, Sharples S, Ritchie AC, Sánchez López T, McDowell M, Morvan HP (2018) Physiological parameter response to variation of Mental workload. Hum Factors 60:31–56. https://doi.org/10.1177/0018720817733101
Jiang Y, Hong J, Wang W, Qu J (2016) Least squares method-based quantitative modeling on visual comfort for VDT display interface. Int J Adv Manuf Technol 84:381–391. https://doi.org/10.1007/s00170-015-8222-8
Hendrick NAS, Hedge A, Brookhuis K (2004). In: Salas E, Hal W (eds) Handbook of human factors and ergonomics methods. CRC Press, Boca Raton
Ali N, Tschenett H, Nater UM (2023) Biomarkers of stress and disease. In: Friedman HS, Markey CH (eds) Encyclopedia of mental health, 3rd edn. Academic Press, Oxford, pp 221–231
Shaffer F, Ginsberg JP (2017) An overview of heart rate variability metrics and norms. Front Public Health 5:258
Rea P (2014) Introduction to the nervous system. In: Rea P (ed) Clinical anatomy of the cranial nerves. Academic Press, San Diego, pp xv–xxix
Murison R (2016) Chap. 2—the neurobiology of stress. In: Al’Absi M, Flaten MA (eds) Neuroscience of pain, stress, and emotion. Academic Press, San Diego, pp 29–49
Boucsein W (2012) Electrodermal activity. Springer US, Boston, MA
Akhter N, Tharewal S, Gite H, Kale KV (2015) Microcontroller based RR-interval measurement using PPG signals for heart rate variability based biometric application. In: 2015 international conference on advances in computing, communications and informatics (ICACCI). pp 588–593
Regalia G, Resnati D, Tognetti S (2023) Sensors on the wrist. In: Narayan R (ed) Encyclopedia of sensors and biosensors. Elsevier, Oxford, pp 1–20
Geršak G, Drnovšek J (2020) Electrodermal activity patient simulator. PLoS ONE 15:e0228949. https://doi.org/10.1371/journal.pone.0228949
Gervasi R, Mastrogiacomo L, Franceschini F (2022) Human-robot collaboration in a repetitive assembly process: a preliminary investigation on operator’s experience and product quality outputs. Proceedings book of the 5th international conference on quality engineering and management. International conference on quality engineering and management. Braga, Portugal, pp 72–92
Universal robots : collaborative robotic automation | Cobots from universal robots, https://www.universal-robots.com/
Yelle LE (1979) The learning curve: historical review and comprehensive survey. Decis Sci 10:302–328. https://doi.org/10.1111/j.1540-5915.1979.tb00026.x
Franceschini F, Galetto M (2003) Composition laws for learning curves of industrial manufacturing processes. Int J Prod Res 41:1431–1447. https://doi.org/10.1080/1352816031000075035
Anzanello MJ, Fogliatto FS (2011) Learning curve models and applications: literature review and research directions. Int J Ind Ergon 41:573–583. https://doi.org/10.1016/j.ergon.2011.05.001
Franceschini F (2002) Learning curves and p-charts for a preliminary estimation of asymptotic performances of a manufacturing process. Total Qual Manag 13:5–12. https://doi.org/10.1080/09544120120098528
Wright TP (1936) Factors affecting the cost of airplanes. J Aeronaut Sci 3:122–128. https://doi.org/10.2514/8.155
R Core Team (2022) R: a language and environment for statistical computing. R Foundation for Statistical Computing, Vienna
R Core Team : R: the R stats package, https://stat.ethz.ch/R-manual/R-devel/library/stats/html/00Index.html
Bates DM, Watts DG (1988) Nonlinear regression analysis and its applications. John Wiley and Sons, New York, NY
Empatica (2023) E4 wristband, https://www.empatica.com/research/e4. Accessed on 20 June 2023.
Kim H-G, Cheon E-J, Bai D-S, Lee YH, Koo B-H (2018) Stress and heart rate variability: a meta-analysis and review of the literature. Psychiatry Investig 15:235–245. https://doi.org/10.30773/pi.2017.08.17
Benedek M, Kaernbach C (2010) Decomposition of skin conductance data by means of nonnegative deconvolution. Psychophysiology 47:647–658. https://doi.org/10.1111/j.1469-8986.2009.00972.x
Taelman J, Vandeput S, Spaepen A, Van Huffel S (2009) Influence of mental stress on heart rate and heart rate variability. In: Vander Sloten J, Verdonck P, Nyssen M, Haueisen J (eds) 4th European conference of the international federation for medical and biological engineering. Springer, Berlin, pp 1366–1369
Liu Y, Du S (2018) Psychological stress level detection based on electrodermal activity. Behav Brain Res 341:50–53. https://doi.org/10.1016/j.bbr.2017.12.021
Shapiro SS, Wilk MB (1965) An analysis of variance test for normality (complete samples). Biometrika 52:591–611. https://doi.org/10.1093/biomet/52.3-4.591
Papanastasiou S, Kousi N, Karagiannis P, Gkournelos C, Papavasileiou A, Dimoulas K, Baris K, Koukas S, Michalos G, Makris S (2019) Towards seamless human robot collaboration: integrating multimodal interaction. Int J Adv Manuf Technol 105:3881–3897. https://doi.org/10.1007/s00170-019-03790-3
Caiazzo C, Nestić S, Savković M (2023) A systematic classification of key performance indicators in human-robot collaboration. In: Mihić M, Jednak S, Savić G (eds) Sustainable business management and digital transformation: challenges and opportunities in the post-COVID era. Springer International Publishing, Cham, pp 479–489
Funding
Open access funding provided by Politecnico di Torino within the CRUI-CARE Agreement. This study was carried out within the MICS (Made in Italy – Circular and Sustainable) Extended Partnership and received funding from the European Union Next-GenerationEU (PIANO NAZIONALE DI RIPRESA E RESILIENZA (PNRR) – MISSIONE 4 COMPONENTE 2, INVESTIMENTO 1.3 – D.D. 1551.11-10-2022, PE00000004). This manuscript reflects only the authors’ views and opinions, neither the European Union nor the European Commission can be considered responsible for them.
Author information
Authors and Affiliations
Contributions
All authors contributed to the study conception and design. Material preparation, data collection and analysis were performed by RG and MC. The first draft of the manuscript was written by RG and MC under the supervision of LM and FF. All authors read and approved the final manuscript.
Corresponding author
Ethics declarations
Conflict of interest
The authors declare that they have no conflict of interest.
Ethical approval
The authors respect the Ethical Guidelines of the Journal.
Consent to participate
Informed consent was obtained from all individual participants included in the study.
Additional information
Publisher’s Note
Springer Nature remains neutral with regard to jurisdictional claims in published maps and institutional affiliations.
Rights and permissions
Open Access This article is licensed under a Creative Commons Attribution 4.0 International License, which permits use, sharing, adaptation, distribution and reproduction in any medium or format, as long as you give appropriate credit to the original author(s) and the source, provide a link to the Creative Commons licence, and indicate if changes were made. The images or other third party material in this article are included in the article's Creative Commons licence, unless indicated otherwise in a credit line to the material. If material is not included in the article's Creative Commons licence and your intended use is not permitted by statutory regulation or exceeds the permitted use, you will need to obtain permission directly from the copyright holder. To view a copy of this licence, visit http://creativecommons.org/licenses/by/4.0/.
About this article
Cite this article
Gervasi, R., Capponi, M., Mastrogiacomo, L. et al. Analyzing psychophysical state and cognitive performance in human-robot collaboration for repetitive assembly processes. Prod. Eng. Res. Devel. 18, 19–33 (2024). https://doi.org/10.1007/s11740-023-01230-6
Received:
Accepted:
Published:
Issue Date:
DOI: https://doi.org/10.1007/s11740-023-01230-6