Abstract
Human–Robot Collaboration (HRC) represents an innovative solution able to enhance quality and adaptability of production processes. However, to fully exploit the benefits of HRC, human factors must be also taken into account. A novel experimental setting involving a repetitive assembly process is presented to investigate the effects of prolonged HRC on user experience and performance. Each participant was involved in two 4-h shifts: a manual assembly setting and a HRC one. The response variables collected in the study included self-reported affective state, perceived body discomfort, perceived workload, physiological signals for stress (i.e., heart rate variability and electrodermal activity), process and product defectiveness. Experimental results showed less upper limb exertion in the HRC setting, emphasizing the contribution of cobots in improving physical ergonomics in repetitive processes. Furthermore, results showed reduced mental effort, stress, and fewer process defects in the HRC setting, highlighting how collaborative robotics can improve process quality by supporting operators from a cognitive point of view in repetitive processes.
Similar content being viewed by others
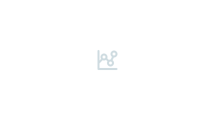
Avoid common mistakes on your manuscript.
1 Introduction
The recent paradigm of Industry 5.0 has proposed a novel approach to manufacturing. One of the most challenging goals pursued by this concept consists in the adoption of automation technologies to improve humans’ working conditions [1]. In this regard, there is a growing amount of literature that is starting to recognize the importance of workers’ well-being within the workplace, both from a mental (e.g., stress and attention) and physical (e.g., fatigue and muscle exertion) point of view and its consequent impact on efficiency and performance [2, 3]. Recent years have witnessed a crucial shift from a management approach exclusively aimed at optimizing times and methods, towards a more human-centric perspective [4]. As a result, technology and automation should be intended to collaborate with humans rather than replace them. One of the main enabling technologies of this transition is collaborative robotics. In a manufacturing process, collaborative robots (or cobots) are typically used to support humans with repetitive processes. Unlike traditional robots, collaborative robotics is based on the co-presence of humans and robots and on the possibility to work simultaneously in a shared workspace [5, 6]. This new paradigm is referred as Human–Robot Collaboration (HRC). On the one hand, the robot assists humans in repetitive and higher-precision actions; on the other hand, humans intervene where there is a need for greater flexibility. One of the application areas where cobots are most widespread are assembly processes. Collaborative assemblies, in fact, are processes in which operators and robots work simultaneously to assemble a product together.
To obtain the full benefits from HRC, human-related aspects must also be taken into consideration [7]. Accordingly, the evaluation of the effects of HRC on the psychophysical state of humans proves to be crucial in promoting and improving the well-being of operators [8, 9]. In a repetitive assembly task cobots can be used to support the operator, however knowledge about the impact that a cobot can have on the operator's state during entire work shifts is still quite limited.
This paper aims to address this gap by presenting an experimental setting designed to emulate work shifts and investigating the differences between a manual and an HRC repetitive assembly. 4-h shifts of a tile-cutter assembly process were implemented in both manual (i.e., without the help of the cobot) and HRC (i.e., with the cobot support) modalities. Three main aspects were analyzed:
-
(i)
The user experience in terms of perceived workload, affective state, and physical exertion of various body parts.
-
(ii)
The physiological response, in terms of electrodermal activity (EDA) and heart rate variability (HRV) to quantify the operator’s stress.
-
(iii)
The process and product defects generated during the repetitive process.
The main novelty elements of this works are: (i) replicating real-working conditions to capture the effects on stress, user experience, and defectiveness in a repetitive assembly process; (ii) highlighting the differences between repetitive assembly processes carried out manually and with a cobot with respect to the operator perspective.
The paper is organized as follows. Section 2 provides a review of the current literature on human factor in HRC manufacturing processes. Section 3 is concerned with the experimental methodology adopted, while Sect. 4 focuses on the analysis of the results obtained. Finally, Sects. 5 and 6 discuss the main findings of the experimental analysis and future works, respectively.
2 Literature review
2.1 Human–robot collaboration (HRC)
In a collaborative production process, cobots support humans in the most repetitive and strenuous actions, while humans make up for the robot's rigidity with their flexibility and dexterity [5, 6, 10]. One of the main challenges for the implementation of HRC is to provide technologies that make interaction fluent and natural. Wang et al. [11] emphasised the importance of the communicative interface between robots and humans, to achieve a symbiotic HRC. Inkulu et al. [12] highlighted prospects and major challenges related to HRC. Human–robot communication modes, such as gestures and voice, enable fluent and immediate interaction, although they still need deeper investigation. Although the main safety devices are well established and suitable for a collaborative approach for low-load and low speed robots, such safety systems are still rigid and only partially allow symbiotic work with high-load robots working at high speeds. Further exploration of advanced adaptive robotic systems is also needed to improve production efficiency.
Enabling HRC in manufacturing processes implies the removal of barriers used to traditionally divide the work area of humans from that of robots. For this reason, safety remains a topic of primary concern in HRC. The introduction of ISO 10218–1 and ISO 10218–2 defined the main hazards that can be encountered when implementing industrial robots in manufacturing settings. In addition, the subsequent ISO/TS 15,066 allowed for greater robot’s autonomy while working closely with humans. Zanchettin et al. [13] introduced a metric to assess safety in collaborative manufacturing processes. This metric considers distance between man and robots, type of robot and operational speed as crucial variables affecting safety in HRC.
2.2 Human factors in HRC
Working while sharing space and time with a robot can cause stress and fatigue issues in human operators. This is consequently reflected in the quality of the output produced, and thus in the occurrence of product and process defects. Gervasi et al. [7] developed a conceptual framework to evaluate HRC. This work highlighted the importance to adopt a holistic view in the assessment of HRC, including variables such as mental and physical ergonomics, safety, robot adaptiveness, communication and interaction, team organization, ethics, and cybersecurity.
Human factors have become increasingly relevant in manufacturing process design. Concepts such as stress, fatigue, mental load, and physical ergonomics have long been addressed [14,15,16]. Over the years many tools and methods have been proposed to assess these constructs. Some methods are self-reporting tools that assess subjective perceptions of physical and mental exertion, while others include psychophysiological measures aimed at tracking the psychophysical state of the worker. Examples of widely used self-reporting tools include the NASA-TLX [17] and the Subjective Workload Assessment Technique (SWAT) [18]. Marinescu et al. [19] have pointed out the weakness of these tools, finding them unsuitable and unreliable for continuous process monitoring in manufacturing settings. Consequently, attention has shifted in recent years to understanding the operator's state by including objective physiological measures [20, 21].
Different recent works have shifted the focus to human factors in HRC. Khalid et al. [22] investigated safety of HRC systems when using high-load robots. In defining potential hazards, the authors also included physical and mental strain associated with a collaborative task. Galin and Meshcheryakov [23] analyzed both human- and robot-dependent factors that may impact HRC efficiency. Among human factors, emotional and cognitive aspects were found to be crucial for HRC efficiency. Khamaisi et al. [24] proposed a framework for assessing user experience in manufacturing context, which included questionnaires and non-invasive sensors to collect physiological signals. The aim was to assess the most stressful activities that may negatively impact operators’ performance. Kühnlenz et al. [25] studied the effects on humans of several trajectory patterns of an industrial robot by analyzing heart rate variability (HRV) and electrodermal activity (EDA). Colim et al. [26] established guidelines for designing safe and ergonomic collaborative workstations.
To date a limited number of studies adopted an experimental approach to investigate the effect of HRC on mental and physical workload of operators in repetitive industrial activities. Table 1 summarizes the main articles concerning experimental assessment of human factors in HRC in manufacturing context, including their contribution and unexplored topics. Several works focused on studying the effect of robot trajectories on user experience and stress. Most of the works implemented self-reporting tools, such as the NASA-TLX for assessing workload or the Self-Assessment Manikin (SAM) for collecting users’ affective state. Some pioneering work integrated the use of bionsensors to collect physiological signals to assess human aspects such as stress, cognitive load, and fatigue. However, the study of human factors in repetitive industrial HRC processes is still almost unexplored. The objective of this paper is to address this gap.
3 Methodology description
The experimental campaign was carried out in the "Mind4Lab" collaborative robotics laboratories of “Politecnico di Torino” (Italy). The aim of the experiment was to analyze the differences between manual assembly and collaborative modality in terms of user experience, operator affective state, workload, stress, and physical effort in a repetitive assembly process. This section is concerned with the methodology adopted for this study, describing the experimental setting, participant selection, materials and instruments, and experimental procedure.
3.1 Experimental setting
The experiment consisted of performing a repetitive assembly process aimed at simulating a 4-h work shift [33]. The assembly process was performed both in manual and HRC modality. The process considered in this study concerns the assembly of a tile cutter (Fig. 1). In Table 2 the list of all the components and their identifiers are provided. Figure 2 shows the ten components of the tile cutter and the five bolts with their respective identifiers. At the beginning of the assembly process, the components are arranged on a tray as shown in Fig. 3 and then the tray is placed in the work area (Fig. 4).
The assembly process was broken down into elementary operations (i.e., pick and place, screwing). In manual modality, all operations were performed by the human operator. In collaborative modality, however, the elementary operations were divided between the human operator and the cobot. The cobot was mainly assigned pick and place tasks, while the human operator was assigned tasks requiring more flexibility and dexterity, such as screw positioning and joining process (i.e., screwing and insertions). Table 3 show the details of the elementary operations and the agent assigned to perform them. Elementary operations in Table 3 can be grouped in four main phases (Fig. 5):
-
Phase 1.
The cobot takes the tile cutter's base closer to the operator, positioning it in the assembly area, and then the operator assembles the two side supports. At the end of the assembly operation, the cobot removes the base and supports from the assembly area.
-
Phase 2.
The operator assembles the cutting part with the assistance of the cobot, that keeps the main component in an ergonomic position.
-
Phase 3.
The operator inserts the two rods into the appropriate locations on the cutting component while the cobot returns the base with supports to the assembly area.
-
Phase 4.
The operator puts the two rods against the two side supports, then screws the handle to the cutting mechanism. The cobot takes away the assembled product.
In manual modality, all operations (see Table 3) were performed by humans and both the operations and the assembly sequence are the same as in collaborative modality. In fact, the participants were not required to develop their own assembly strategy, but to stick to the sequence provided in Table 3. This choice was aimed at keeping the experimental setting similar in the two modalities, so as not to introduce additional factors.
3.2 Participants
The experimental campaign involved 36 participants (17 males and 19 females) aged between 20 and 25 with no previous experience with cobots and the tile-cutter assembly, divided into groups of three people. Each group member had a specific role. A participant was in charge of assembling the tile cutter repetitively and continuously. Another participant was responsible for supervising the entire assembly process, reporting any process defect and critical issue. Finally, the third team member was assigned the task of checking the conformity of each assembled product and reporting any product defects.
3.3 Materials and instrument
The analysis of the effect of HRC on human operators embraces a wide variety of aspects. In this view, an holistic approach should be pursued [7]. In order to explore differences between manual and HRC assembly, data regarding user experience, physiological signals and generated defects were collected in this experimental campaign (Table 4). In the next subsections, more details are provided for each considered aspect.
3.4 Self-reporting tools and questionnaires
Operators’ feedback on manual and collaborative modalities were collected through a set of self-reporting tools. In addition to an initial questionnaire aimed at collecting personal data, self-reporting tools included questionnaires on perceived workload, affective state and physical ergonomics were submitted to participants.
Concerning perceived workload, the NASA-TLX [17] was applied (Fig. 6). This widely used tool takes into account six dimensions composing perceived workload:
-
Mental demand, representing the amount of cognitive and perceptual demand required to complete the task.
-
Physical demand, describing the amount of physical effort a task demands.
-
Temporal demand, related to the perception of time constraints while performing a task.
-
Performance, referring to how well and how satisfied one is with the results obtained.
-
Effort, which describes the amount of mental and physical effort required in accomplishing a certain goal.
-
Frustration, which reflects the degree of discomfort, stress and annoyance experienced while performing the task.
NASA-TLX questionnaire [17]
The final workload score is calculated by averaging the ratings of the previous 6 dimensions, each of which is expressed on a value between 0 and 100 in five-point increments.
The Self-Assessment Manikin (SAM) [21, 34] is a common image-based assessment tool for evaluating an individual's emotional response to a given circumstance or event. SAM was used in this experiment to collect affective state, assessing three dimensions:
-
Valence (or pleasure), which determines whether a feeling is pleasant or negative.
-
Arousal, which describes a person's level of arousal, regardless of whether this arousal is caused by a pleasant or negative feeling.
-
Dominance, which refers to the feeling of being in control of a certain situation.
Finally, to assess the physical fatigue of operators performing the repetitive assembly process, participants were asked to rate perceived exertion in specific areas of the body. Thirteen areas of the body that could be prone to fatigue were identified, as shown by Visser and Straker [35]. The thirteen body areas assessed by participants are: neck, right and left shoulder, left and right upper arm, right and left forearm, right and left hand, upper back, lower back, buttocks, and lower limbs. For each of the 13 areas, perceived discomfort was assessed by participants using the Borg CR10 scale [36,37,38]. The version of Borg CR10 scale adopted in this study is detailed in Table 5. This scale was introduced to assess the level of perceived exertion on a scale ranging from "No exertion at all” to "Maximum exertion". Numerical values associated to categorical judgements are related to a ratio scale [36, 39].
3.5 Physiological signals
Self-reporting tools provide a subjective evaluation of workload perceived. In order to collect objective data related to physiological stress, Empatica E4 wristband was used. Empatica E4 is non-invasive biosensor able to provide EDA data at 4 Hz, heart data through Photopletismogram (PPG) at 64 Hz, and 3-axis accelerometer data at 32 Hz. From PPG and EDA stress indicators can be obtained by measuring HRV (i.e., Heart Rate Variability) and average SCR (i.e., Skin Conductance Response).
The MATLAB package 'Ledalab' was used to process the EDA data. The EDA signal was decomposed using continuous decomposition analysis (CDA) [40] into continuous signals of phasic and tonic activity. The best way to identify tonic activity is through changes in skin conductance level (SCL), which refers to long-term fluctuations in EDA not explicitly caused by external stimuli. Phasic activity, on the other hand, describes brief changes in EDA that are triggered by a typically recognized and externally delivered stimulus. Skin conductance responses (SCRs), i.e., changes in amplitude from the SCL to the peak of the response, can be detected by analyzing the phasic activity signal. In this study the mean SCR was used as a measure of stress and arousal. Furthermore, HRV measures can be obtained from heart data and can also be utilized as a stress and arousal indicator. Due to its widespread application in previous studies, as HRV measure, the Root Mean Square of Successive Differences between Adjacent NN-Intervals (RMSSD) was also utilized in this study as for HRV [16, 41]:
where \(N\) is the number of systolic peaks in the considered time window and \({NN}_{i}\) indicates the time interval between the systolic peak i and i + 1 (Fig. 7).
Example of a PPG signal, where NN-intervals are time intervals between two systolic peaks [42]
3.6 Process and product defects
Another crucial aspect in comparing manual and collaborative assembly is the analysis of occurring defects. Defects can be distinguished in two classes: product and process defects. Product defects consist of anomalies in the product that impair its functionality. The final product then does not conform to specifications. Process defects, on the other hand, refer to all those errors that the human operator or robot can make in an assembly process. Such problems lead to disruptions in the assembly process and result in loss of time and thus efficiency. Table 6 details the main process defects considered in this study.
3.7 Experimental procedure
Each group was involved in two 4-h assembly sessions: one in manual modality (Manual) and the other in HRC modality (HRC) with random order. Within each session, a 10-min break was included after 2 h of work, thus dividing the session in two parts (Part 1 and Part 2). In Fig. 8 the flowchart for an experimental session is presented. After a brief introduction concerning experimental objectives, the participants took place in the work area and the details of the assembly task were presented. Afterwards, the participant who had to perform the assembly was equipped with the biosensors. In order to collect accurate EDA data using the Empatica E4 biosensor, 15 min were required for the electrodes to firmly adhere to the participant's left wrist. Meanwhile, a couple of practice trials were performed in the selected assembly modality so that the participants would become familiar with their roles. Next, the participant was invited to relax for 2 min to record the baseline of the physiological signals and then the 4-h assembly session began. The second and third participants supervised each session by taking note of occurring process defects and checked the conformity of the final product to specifications, respectively. The participant in charge of assembly was administered the NASA-TLX, SAM, BDM questionnaire at mid-session (before the 10-min break) (Part 1) and at the end of the session (Part 2). At the conclusion of the session, general feedbacks on the experiment were collected.
4 Results and analysis
In this section, the obtained results of the experiment are presented and analyzed.
4.1 Perceived workload (NASA-TLX)
Figure 9 shows an overall graphical comparison between the HRC and manual assembly modality for each NASA-TLX dimension. Additionally, since the normality assumption was not rejected with the Shapiro-Wilks test for each dimension [43], paired sample t-test were implemented to highlight significant differences between modalities. This test is suitable for analyzing paired data, as it considers the within-subject effect.
The assembly process was generally perceived more physical demanding (Physical Demand) in Manual. This may be due to the fact that in the HRC setting the robot is supportive in handling the larger components. In the mid-session this difference was found to be significant (Part 1: p = 0.038), however it was not at the end of the session (Part 2: p = 0.092).
Concerning Temporal Demand, no significant difference emerged between the two assembly modalities. This was confirmed by the paired sample t-test both mid-session (Part 1: p = 0.61) and at the end of the session (Part 2: p = 0.83).
Regarding Performance, no difference emerged at mid-session, and it was confirmed by the paired sample t-test (Part 1: p = 0.80). Interestingly, a deterioration can be seen for the HRC setting at the end of the session. This may be due to a task learning factor, which led to a feeling of lower efficiency compared to manual assembly, where process performance depends solely on the operator. However, the difference between the two modalities was found to be not significant (Part 2: p = 0.11).
A significant increase in Mental Demand can be observed in Manual, meaning that in this setting the assembly required more mental effort. This effect resulted significant according to the paired sample t-test both mid-session (Part 1: p = 0.025) and at the end of the session (Part 2: p = 0.039).
The perceived Effort was higher for the Manual setting in the mid-session and the paired sample t-test highlighted a significant difference (Part 1: p = 0.035). However, no significant difference emerged at end of session (Part 2: p = 0.51).
No difference in Frustration emerged between the two modalities at mid-session and the paired sample t-test confirmed this result (Part 1: p = 0.69). At the end of the session, however, an increase could be observed in the HRC setting, although the difference between the two modes was not found to be significant (Part 2: p = 0.12). This increase may be related to a learning factor that led to a feeling of greater constraint of the cobot in the process.
The overall perceived Workload is initially slightly higher in the manual setting, as can be seen in Fig. 10. However, according to the paired sample t-test, this difference was not found to be significant (Part 1: p = 0.12). At the end of the session, the difference in Workload between the two modalities is significantly reduced, which was also confirmed by the paired sample t-test (Part 2: p = 0.83).
4.2 Affective state
Figure 11 shows an overall graphical comparison between the HRC and manual assembly modality for SAM dimension. Significant differences were analyzed using the Wilcoxon signed-rank test that is suitable for analyzing paired ordinal data [44].
Regarding Valence, slightly higher ratings were reported in the HRC setting in the middle of the session, probably due to the novelty effect introduced by the cobot. However, the difference between the two modalities was found to be not significant according to the Wilcoxon signed-rank test (Part 1: p = 0.41). At end session, a general decrease in Valence can be observed as well as a not significant difference between the HRC and manual settings (Part 2: p = 0.39).
For Arousal, participants were not particularly agitated, and no significant difference emerged between the two assembly modalities. This was confirmed by the Wilcoxon signed-rank test both mid-session (Part 1: p = 0.82) and at the end of the session (Part 2: p = 0.89).
At mid-session, higher Dominance resulted in manual setting and the difference with the HRC session was significant according to the Wilcoxon signed-rank test (Part 1: p = 0.037). This means that participants felt more in control of the situation in a manual setting. However, at end of the session, the difference between the two modalities was not significant (Part 2: p = 0.37).
4.3 Perceived physical exertion
Figure 12 presents an overall graphical comparison between the HRC and manual assembly modality for each BDM dimension. The significance of the differences between the two modalities was checked using the Wilcoxon signed-rank test. At mid-session, slightly more fatigue can be seen in the manual session for the left upper arm (Part 1: p = 0.048), left forearm (Part 1: p = 0.054), and upper back (Part 1: p = 0.076). However, no significant differences between the HRC and manual modalities emerged across the different dimensions at both mid-session and end of session. This means that the perceived physical fatigue in different parts of the body was comparable between the two modalities.
4.4 Physiological response
Figure 13 shows the distributions of the physiological response between the HRC and manual modalities. Since for both Mean_SCR and the RMSSD the normality assumption was rejected by the Shapiro–Wilk test, the Wilcoxon signed-rank test was used to check significant differences.
Boxplot comparison of physiological response (Mean_SCR for EDA and RMSSD for HRV) between HRC and manual assembly modality at half-session (Part 1) (a) and end of session (Part 2) (b). Significance of the Wilcoxon signed-rank test is reported as follows: (.) p≈ 0.05, (*) 0.05 > p ≥ 0.01, (**) 0.01 > p ≥ 0.001, (***) p < 0.001
Regarding EDA, a greater average SCR (Mean_SCR) was observed in the manual setting at mid-session and the difference resulted almost significant (Part 1: p = 0.052). More stress in the manual setting may result from more cognitive effort required to remember some operations, such as assembling the cutting component of the tile cutter. At the end of session, the difference between the two modalities was not significant (Part 2: p = 0.57).
With respect to HRV, in the manual setting a higher RMSSD can be noted at both mid-session and end of session, leading to potentially slightly less stressful situations. Both the differences resulted significant according to the Wilcoxon signed-rank test (Part 1: p = 0.009; Part 2: p = 0.009). It is interesting also to observe a general increase of the RMSSD between the first and second part of the session.
4.5 Process and product defects
In Fig. 14, a graphical comparison of process defects between HRC and manual modalities is provided. The normality assumption was not rejected by the Shapiro–Wilk test. In manual setting, more process defects can be observed at both mid-session and the end of session. Moreover, the difference between the two modalities emerged to be significant according to the paired sample t-test (Part 1: p = 0.02; Part 2: p = 0.007). This result highlights that the robot intervention also supported the participants from a cognitive point of view. Specifically, through the operations performed by the robot that paced the process, the operator more easily remembered the next operations to be performed.
Figure 15 provides a graphical comparison of product defects between HRC and manual modalities. The normality assumption was rejected by the Shapiro–Wilk test. In manual setting, slightly more product defects can be observed at both mid-session and the end of session. However, according to the Wilcoxon signed-rank test, the differences between the two modalities were not significant (Part 1: p = 0.37; Part 2: p = 0.075).
Figures 16, 17 and 18 show the Pareto charts of the process defects divided by modality and by the agent that caused them. Considering all 12 groups, a total of 928 trials were carried out in manual modality and 705 in HRC modality. As can be seen, with regard to the defects caused by humans (Figs. 16 and 17), 75% of these are represented in both cases by: “Dropping of nuts and washers”, “Wrong part positioning”, “Incorrect assembly” and “Dropping of parts”. These defects were often caused by both mental and physical fatigue of the operators. Even from these preliminary data, however, it can be seen that the cobot reduces the frequency of occurrence. Note, for example, the significant reduction in the number of times the defect 'Wrong part positioning' was found. Therefore, although fewer trials were carried out in HRC modality, “wrong part positioning” accounted for 18% of total human process defects in collaborative modality and 27% in manual modality. Cobots, in fact, support the operator in following the correct assembly sequences, bringing the correct parts to use and in the correct position to complete the assembly. Concerning the process failures caused by the cobot, it can be noticed that most of them consist of emergent blockages mainly due to collision with objects. The presence of force sensors in the cobots determines, for safety reasons, the emergency stop of the cobot in the presence of impacts. This, on the one hand, is crucial to ensure safety of the human operators within the work area, while, on the other hand, it may lead to lower productivity of the collaborative process compared to a manual one.
5 Discussion
Comparison of the two settings, manual and HRC, for a repetitive assembly process revealed interesting differences in user experience, physiological feedback, and performance (Table 7).
From the workload point of view, the perceived Mental Demand was higher in the manual setting. This was mainly due to the fact that the robot, by moving and positioning the various components,indirectly helped the operator to remember the sequence of operations. Initially, in the first part of the session, the gap between the manual setting and the collaborative setting was greater in terms of perceived Physical Demand and Effort. However, due to the learning process of the participants, this difference was quite attenuated at the end of the shift. Regarding physical exertion, a slightly higher perception of fatigue of the entire left upper limb in the manual setting could be noted, however, this difference was attenuated at the end of the session.
The presence of the cobot generated in the participants a lower sense of situational dominance (Dominance) in the first part of the session. However, in the second part this effect practically disappeared. It is also interesting to note that there were fewer process defects in the HRC setting. Most of the process defects involved incorrect selection, positioning, and assembly of components that occurred during the assembly of the cutting mechanism (Phase 2, see Table 3). In the HRC setting, the robot always held component C2 of the cutting mechanism in the same way, thus making it easier for participants to remember how to position and assemble the other components. Such support therefore allowed fewer defects to be made.
From a physiological point of view, it was noticed that in the first part of the session the Mean_SCR was higher in the manual session. This may be due to higher initial stress from trying to remember how to assemble the cutting mechanism correctly, a phase in which more process defects were also generated. From the HRV, more relaxation was observed in the manual setting, which may have also led to more distractions. Thus, the presence of the cobot may have contributed to more sustained attention during the session, which also led to the generation of fewer process defects.
Participant feedback indicated that in general the support of the cobot in assembling the cutting mechanism was appreciated, making the operation more immediate. Of the HRC setting, it was also appreciated that the cobot indirectly helped with its operations to remember the operator's next operations. However, of the manual setting, greater freedom in component handling (especially in the assembly phase of the cutting mechanism) and greater autonomy in task timing were appreciated, which increased perceived efficiency.
6 Conclusions
The aim of this paper was to propose a novel experimental setting to reproduce a set of 4-h work shifts of a repetitive collaborative assembly process. By implementing this setting, it is possible to conduct studies on the effects of a prolonged interaction with a cobot on user experience, human state and generated defects in a manufacturing context. Additionally, the use of non-invasive biosensors makes it feasible to gather objective data about the operator's psychophysical state without interfering with the process. To the best of the authors’ knowledge, no previous study has experimentally investigated the differences between manual and collaborative repetitive assembly processes. Consequently, this work represents a first approach to bridging this gap.
Experimental results revealed some differences between the manual and HRC settings. In the manual setting, slightly more physical effort was observed as well as higher cognitive effort, mainly due to remembering how to assemble some components. Although the cobot introduced a few more constraints in the process, it allowed the operator to perform fewer process defects (e.g., incorrect selection, placement, and assembly of components). This result highlights how HRC can be a valuable support for the operator not only from a physical but also a cognitive point of view. From a physiological point of view, through EDA a slightly higher stress was noted initially in the manual setting due to trying to remember how to assemble certain components. Observing the HRV, however, it was noticed generally more relaxation in the manual setting, thus leading to more distraction during the process.
Some limitations of the study are present. The number of participants involved is quite limited. Also, the participants involved were not real operators and consequently had no experience in assembly processes in manufacturing. However, this allowed for no bias to be introduced with respect to one modality over another.
Future work will focus on expanding findings by increasing the sample of participants and further investigating the relationship between cognitive workload, stress, and process defects. In addition, operators working in manufacturing will be involved to explore any differences and preferences compared to people with no previous experience.
References
Maddikunta PKR, Pham Q-V, B P, Deepa N, Dev K, Gadekallu TR, Ruby R, Liyanage M (2022) Industry 5.0: A survey on enabling technologies and potential applications. J Ind Inf Integr 26:100257. https://doi.org/10.1016/j.jii.2021.100257
Gualtieri L, Rauch E, Vidoni R (2021) Emerging research fields in safety and ergonomics in industrial collaborative robotics: A systematic literature review. Robot Comput Integr Manuf. 67, 101998. https://doi.org/10.1016/j.rcim.2020.101998
Zare M, Croq M, Hossein-Arabi F, Brunet R, Roquelaure Y (2016) Does Ergonomics Improve Product Quality and Reduce Costs? A Review Article. Hum Factors Ergon Manuf Serv Ind 26:205–223. https://doi.org/10.1002/hfm.20623
Gervasi R, Mastrogiacomo L, Maisano DA, Antonelli D, Franceschini F (2022) A structured methodology to support human–robot collaboration configuration choice. Prod Eng 16:435–451. https://doi.org/10.1007/s11740-021-01088-6
Bauer A, Wollherr D, Buss M (2008) Human–robot collaboration: a survey. Int J Humanoid Robot 05:47–66. https://doi.org/10.1142/S0219843608001303
Goodrich MA, Schultz AC (2007) Human-robot interaction: a survey. Now, Boston, Mass
Gervasi R, Mastrogiacomo L, Franceschini F (2020) A conceptual framework to evaluate human-robot collaboration. Int J Adv Manuf Technol 108:841–865. https://doi.org/10.1007/s00170-020-05363-1
Gervasi R, Aliev K, Mastrogiacomo L, Franceschini F (2022) User Experience and Physiological Response in Human-Robot Collaboration: A Preliminary Investigation. J Intell Robot Syst 106:36. https://doi.org/10.1007/s10846-022-01744-8
Gervasi R, Barravecchia F, Mastrogiacomo L, Franceschini F (2022) Applications of affective computing in human-robot interaction: State-of-art and challenges for manufacturing. Proc Inst Mech Eng Part B J Eng Manuf. 09544054221121888. https://doi.org/10.1177/09544054221121888
Bruno G, Antonelli D (2018) Dynamic task classification and assignment for the management of human-robot collaborative teams in workcells. Int J Adv Manuf Technol 98:2415–2427. https://doi.org/10.1007/s00170-018-2400-4
Wang L, Gao R, Váncza J, Krüger J, Wang XV, Makris S, Chryssolouris G (2019) Symbiotic human-robot collaborative assembly. CIRP Ann 68:701–726. https://doi.org/10.1016/j.cirp.2019.05.002
Inkulu AK, Bahubalendruni MVAR, Dara A K S (2021) Challenges and opportunities in human robot collaboration context of Industry 4.0 - a state of the art review. Ind Robot Int J Robot Res Appl. ahead-of-print. https://doi.org/10.1108/IR-04-2021-0077
Zanchettin AM, Ceriani NM, Rocco P, Ding H, Matthias B (2016) Safety in human-robot collaborative manufacturing environments: Metrics and control. IEEE Trans Autom Sci Eng 13:882–893. https://doi.org/10.1109/TASE.2015.2412256
Gawron VJ (2008) Human Performance, Workload, and Situational Awareness Measures Handbook. CRC Press, Boca Raton
Wickens CD (2008) Multiple Resources and Mental Workload. Hum Factors 50:449–455. https://doi.org/10.1518/001872008X288394
Young MS, Brookhuis KA, Wickens CD, Hancock PA (2015) State of science: mental workload in ergonomics. Ergonomics 58:1–17. https://doi.org/10.1080/00140139.2014.956151
Hart SG, Staveland LE (1988) Development of NASA-TLX (Task Load Index): Results of Empirical and Theoretical Research. In: Hancock, P.A. and Meshkati, N. (eds.) Advances in Psychology. pp. 139–183. North-Holland
Reid GB, Nygren TE (1988) The Subjective Workload Assessment Technique: A Scaling Procedure for Measuring Mental Workload. In: Hancock, P.A. and Meshkati, N. (eds.) Advances in Psychology. pp. 185–218. North-Holland
Marinescu AC, Sharples S, Ritchie AC, Sánchez López T, McDowell M, Morvan HP (2018) Physiological Parameter Response to Variation of Mental Workload. Hum Factors 60:31–56. https://doi.org/10.1177/0018720817733101
Argyle EM, Marinescu A, Wilson ML, Lawson G, Sharples S (2021) Physiological indicators of task demand, fatigue, and cognition in future digital manufacturing environments. Int J Hum Comput Stud. 145:102522. https://doi.org/10.1016/j.ijhcs.2020.102522
Bradley MM, Lang PJ (1994) Measuring emotion: The self-assessment manikin and the semantic differential. J Behav Ther Exp Psychiatry 25:49–59. https://doi.org/10.1016/0005-7916(94)90063-9
Khalid A, Kirisci P, Ghrairi Z, Pannek J, Thoben K-D (2017) Towards Implementing Safety and Security Concepts for Human-Robot Collaboration in the context of Industry 4.0. In: 39th International MATADOR Conference on Advanced Manufacturing
Galin RR, Meshcheryakov RV (2020) Human-Robot Interaction Efficiency and Human-Robot Collaboration. In: Kravets, A.G. (ed.) Robotics: Industry 4.0 Issues & New Intelligent Control Paradigms. pp. 55–63. Springer International Publishing, Cham
Khamaisi, R.K., Brunzini, A., Grandi, F., Peruzzini, M., Pellicciari, M.: UX assessment strategy to identify potential stressful conditions for workers. Robot. Comput.-Integr. Manuf. 78, 102403 (2022). https://doi.org/10.1016/j.rcim.2022.102403
Kühnlenz B, Erhart M, Kainert M, Wang Z-Q, Wilm J, Kühnlenz K (2018) Impact of trajectory profiles on user stress in close human-robot interaction. Autom. 66:483–491. https://doi.org/10.1515/auto-2018-0004
Colim A, Faria C, Cunha J, Oliveira J, Sousa N, Rocha LA (2021) Physical Ergonomic Improvement and Safe Design of an Assembly Workstation through Collaborative Robotics. Safety 7:14. https://doi.org/10.3390/safety7010014
Kulic D, Croft E (2005) Anxiety detection during human-robot interaction. In: 2005 IEEE/RSJ International Conference on Intelligent Robots and Systems. pp. 616–621
Arai T, Kato R, Fujita M (2010) Assessment of operator stress induced by robot collaboration in assembly. CIRP Ann 59:5–8. https://doi.org/10.1016/j.cirp.2010.03.043
Dehais F, Sisbot EA, Alami R, Causse M (2011) Physiological and subjective evaluation of a human–robot object hand-over task. Appl Ergon 42:785–791. https://doi.org/10.1016/j.apergo.2010.12.005
Lasota PA, Shah JA (2015) Analyzing the Effects of Human-Aware Motion Planning on Close-Proximity Human-Robot Collaboration. Hum Factors 57:21–33. https://doi.org/10.1177/0018720814565188
Ustunel Z, Gunduz T (2017) Human-robot collaboration on an assembly work with extended cognition approach. J. Adv. Mech. Des. Syst. Manuf. 11, JAMDSM0057–JAMDSM0057. https://doi.org/10.1299/jamdsm.2017jamdsm0057
Gualtieri L, Fraboni F, De Marchi M, Rauch E (2022) Development and evaluation of design guidelines for cognitive ergonomics in human-robot collaborative assembly systems. Appl Ergon. 104:103807. https://doi.org/10.1016/j.apergo.2022.103807
Gervasi R, Mastrogiacomo L, Franceschini F (2022) Human-robot collaboration in a repetitive assembly process: a preliminary investigation on operator’s experience and product quality outputs. In: Proceedings book of the 5th International Conference on Quality Engineering and Management. pp. 72–92. International Conference on Quality Engineering and Management, Braga, Portugal
Lang PJ (1980) Behavioral treatment and bio-behavioral assessment: Computer applications. In: Sidowski, J.B., Johnson, J.H., and Williams, T.A. (eds.) Technology in mental health care delivery systems. pp. 119–137. Norwood, NJ: Ablex
Visser JL, Straker LM (1994) An investigation of discomfort experienced by dental therapists and assistants at work. Aust Dent J 39:39–44. https://doi.org/10.1111/j.1834-7819.1994.tb05545.x
Borg GAV (1982) Psychophysical bases of perceived exertion. Med Sci Sports Exerc. 14, 377–381
Foster C, Hector LL, Welsh R, Schrager M, Green MA, Snyder AC (1995) Effects of specific versus cross-training on running performance. Eur J Appl Physiol 70:367–372. https://doi.org/10.1007/BF00865035
Impellizzeri FM, Rampinini E, Coutts AJ, Sassi A, Marcora SM (2004) Use of RPE-Based Training Load in Soccer. Med Sci Sports Exerc 36:1042–1047. https://doi.org/10.1249/01.MSS.0000128199.23901.2F
Franceschini F, Galetto M, Maisano D (2019) Designing Performance Measurement Systems: Theory and Practice of Key Performance Indicators. Springer International Publishing
Benedek M, Kaernbach C (2010) Decomposition of skin conductance data by means of nonnegative deconvolution. Psychophysiology 47:647–658. https://doi.org/10.1111/j.1469-8986.2009.00972.x
Kim H-G, Cheon E-J, Bai D-S, Lee YH, Koo B-H (2018) Stress and Heart Rate Variability: A Meta-Analysis and Review of the Literature. Psychiatry Investig 15, 235–245. https://doi.org/10.30773/pi.2017.08.17
Vaqueiro YR, Parsinejad P, Sipahi R, Lorenzo JAM (2018) Development of a combined time-frequency technique for accurate extraction of pNN50 metric from noisy heart rate measurements. Int J Intell Robot Appl 2:193–208. https://doi.org/10.1007/s41315-018-0052-z
Shapiro SS, Wilk MB (1965) An analysis of variance test for normality (complete samples)†. Biometrika 52:591–611. https://doi.org/10.1093/biomet/52.3-4.591
Wilcoxon F (1945) Individual Comparisons by Ranking Methods. Biom Bull 1:80–83. https://doi.org/10.2307/3001968
Funding
Open access funding provided by Politecnico di Torino within the CRUI-CARE Agreement. This work has been partially supported by “Ministero dell’Istruzione, dell’Università e della Ricerca” Award “TESUN-83486178370409 finanziamento dipartimenti di eccellenza CAP. 1694 TIT. 232 ART. 6”.
The authors respect the Ethical Guidelines of the Journal. Informed consent was obtained from all individual participants included in the study.
Author information
Authors and Affiliations
Contributions
All authors contributed to the study conception and design. Material preparation, data collection and analysis were performed by R. Gervasi and M. Capponi. The first draft of the manuscript was written by R. Gervasi and M. Capponi under the supervision of L. Mastrogiacomo and F. Franceschini. All authors read and approved the final manuscript.
Corresponding author
Ethics declarations
Competing interests
The authors declare that they have no conflict of interest.
Additional information
Publisher's Note
Springer Nature remains neutral with regard to jurisdictional claims in published maps and institutional affiliations.
Rights and permissions
Open Access This article is licensed under a Creative Commons Attribution 4.0 International License, which permits use, sharing, adaptation, distribution and reproduction in any medium or format, as long as you give appropriate credit to the original author(s) and the source, provide a link to the Creative Commons licence, and indicate if changes were made. The images or other third party material in this article are included in the article's Creative Commons licence, unless indicated otherwise in a credit line to the material. If material is not included in the article's Creative Commons licence and your intended use is not permitted by statutory regulation or exceeds the permitted use, you will need to obtain permission directly from the copyright holder. To view a copy of this licence, visit http://creativecommons.org/licenses/by/4.0/.
About this article
Cite this article
Gervasi, R., Capponi, M., Mastrogiacomo, L. et al. Manual assembly and Human–Robot Collaboration in repetitive assembly processes: a structured comparison based on human-centered performances. Int J Adv Manuf Technol 126, 1213–1231 (2023). https://doi.org/10.1007/s00170-023-11197-4
Received:
Accepted:
Published:
Issue Date:
DOI: https://doi.org/10.1007/s00170-023-11197-4