Abstract
Because scholarly performance is multidimensional, many different criteria may influence appointment decisions. Previous studies on appointment preferences do not reveal the underlying process on how appointment committee members consider and weigh up different criteria when they evaluate candidates. To identify scholars’ implicit appointment preferences, we used adaptive choice-based conjoint analysis (ACBC), which is able to capture the non-compensatory process of complex decisions like personnel selection. Junior and senior scholars (N = 681) from different countries and types of higher education institutions took part in a hypothetical appointment procedure. A two-step segmentation analysis based on unsupervised and supervised learning revealed three distinct patterns of appointment preferences. More specifically, scholars differ in the appointment criteria they prefer to use, that is, they make different trade-offs when they evaluate candidates who fulfill some but not all of their expectations. The most important variable for predicting scholars’ preferences is the country in which he or she is currently living. Other important predictors of appointment preferences were, for example, scholars’ self-reported research performance and whether they work at a doctorate-granting or not-doctorate-granting higher education institution. A comparison of scholars’ implicit and explicit preferences yielded considerable discrepancies. Through the lens of cognitive bias theory, we contribute to the extension of the literature on professorial appointments by an implicit process perspective and provide insights for scholars and higher education institutions.
Similar content being viewed by others
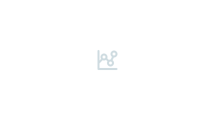
1 Introduction
The question of what influences appointment decisions has been a long-standing and still is a continuing interest of researchers in different scientific fields. Professorial appointments are not only the most important personnel selection decisions of higher education institutions (e.g., Lepori et al. 2015), they also constitute the most important performance evaluation during an academic career. Because scholarly performance is multidimensional (e.g., Aguinis et al. 2014) and professors are expected to perform a large number of different tasks (e.g., Macfarlane 2011), appointment committees use many different criteria to evaluate the performance of candidates. Consequently, the outcome of personnel selection decisions depends on recruiters’ preferences for selection criteria as their preferences determine how they evaluate and select job candidates. In other words, appointment committees need to decide which criteria are most important to them. To better understand the importance of appointment criteria, it is crucial to shed light on the implicit preferences of scholars who serve as members of appointment committees and to identify factors that contribute to differences in their appointment preferences.
Previous research on appointment decisions has employed many different methodological approaches including document analyses (e.g., Subbaye 2018), career trajectories (e.g., van Dijk et al. 2014), surveys and interviews (e.g., Abbott et al. 2010), and experimental designs (e.g., Williams and Ceci 2015), yielding rather mixed and inconclusive results. Many studies inferred the most important selection criteria by analyzing the outcomes of appointment decisions. In particular, they identified the characteristics of successful and unsuccessful candidates for professorships and concluded that publication performance had been the most important criterion for past appointment decisions (e.g., Lutter and Schröder 2016). Contrary, interviews with professors suggest that acquiring grants plays a decisive role in appointment decisions (Macfarlane 2011), and university departments report that they attach most importance to teaching performance and candidate’s fit (Fuerstman and Lavertu 2005; Sheehan et al. 1998). Past research also found that the importance of appointment criteria differs between countries (e.g., Fiedler and Welpe 2008), scientific fields (e.g., Williams and Ceci 2015), and types of higher education institutions (e.g., Landrum and Clump 2004). However, thus far, research does not reveal the underlying process on how appointment committee members consider and weigh up many different criteria of scholarly performance when they evaluate candidates. Many studies focused only on a small subset of appointment criteria, particularly publication performance, and excluded other criteria such as teaching performance and grants. Furthermore, the conclusions from other studies are limited because they measured explicit preferences or used experimental designs that are not suitable for studying complex decisions like appointment decisions.
Accordingly, we aim to (1) identify current appointment preferences in higher education by analyzing scholars’ implicit preferences for performance criteria and (2) provide a more comprehensive overview of the factors that contribute to differences in appointment preferences. For this purpose, we conducted an adaptive choice-based conjoint (ACBC) analysis, allowing us to simulate complex decision-making processes in a realistic and detail-oriented manner, dynamically considering a variety of appointment criteria as well as participants´ individual characteristics. In doing so, we contribute to research on appointment decisions in the following ways: First, we shed light on the underlying process on how appointment committee members weigh up a large number of different criteria when they evaluate the performance of candidates. More specifically, we analyze the trade-offs (i.e., compromises) scholars make when they evaluate candidates who fulfil some but not all of their expectations. Here, we extend previous research on implicit appointment preferences (Fiedler and Welpe 2008) by using adaptive choice-based conjoint analysis. Our contribution lies in particular in the deployment of an exceptional experimental method, which is able to capture a holistic understanding of the non-compensatory process of complex decisions like personnel selection. Second, we determine distinct patterns of appointment preferences and analyze how differences in scholars’ country as well as organizational and individual characteristics are related to differences in appointment preferences. Third, complementing other studies, we consider the results through the lens of cognitive bias theory, in particular heuristics, and thus offer further explanations for the discrepancies between explicit and implicit appointment preferences that may arise from information processing or evolutionary constraints (Haselton et al. 2005). Thereby, we broaden our understanding of current appointment preferences in higher education and how they may influence hiring decisions of university departments and individual scientific careers. Thus, our findings provide insights for scholars and higher education institutions. First, they enhance the transparency of appointment decisions for young scholars who make career decisions. Second, our findings can help higher education institutions to improve the selection process so that appointment decisions are more well-balanced and reflect the opinion of all committee members.
2 Theoretical background
In the following, we first consider cognitive biases as theoretical foundation. Second, we present previous research on professorial appointments decisions. Third, with regard to previous studies, we discuss differences in appointment preferences through the lens of cognitive biases.
2.1 Cognitive bias theory
Both behavioral and psychological research show that cognitive biases in decision-making especially occur in situations of high uncertainty and complexity (Pitz and Sachs 1984). “Cognitive biases are an ever-present ingredient of strategic decision-making” (Das and Teng 1999, p. 757) and, thus, they are also very likely to occur in professorial appointment decisions. In the context of professorial appointment decisions, cognitive biases arise from, among other things, the choice of useful shortcuts that appear promising for successful decision-making (i.e., heuristics). Heuristics, however, result from information-processing or evolutionary mechanisms, meaning that the criteria for strategic decision-making function adequately in principle, but may exhibit systematic errors (Haselton et al. 2005). Regarding professorial appointment decisions, previous research reveals that, despite changing environmental demands (e.g., the need to adopt innovative teaching concepts to counteract declining student numbers), the appointment criteria seem more or less unchanging over a long period of time. Heuristics might emerge due to limited time and information processing abilities and due to the fact that especially employees in positions of power invoke established rules to allocate attentional resources and cognitive effort otherwise (Haselton et al. 2005; Keltner et al. 2003). This explanation can be transferred to professorial appointment decisions, given that professors usually allocate their cognitive resources across a multifaceted range of tasks and, accordingly, may prioritize established appointment criteria, such as publication performance, in their decision-making. In this context, Morewedge and Kahneman (2010) describe dual-system models that explain how automated decisions emerge under errors of judgement. Here, three features are central to the decision-making process: associative coherence, attribute substitution, and processing fluency. These features may also be transferred to the context of professorial appointment decisions, as the fulfillment of specific appointment criteria elicit self-reinforcing responses in associative memory of the appointment committee (i.e., associative coherence); the evaluation of the appointment criteria is accompanied by an unconscious assessment of other dimensions (e.g., a high number of publications suggests high professional aptitude) (i.e., attribute substitution); and the cognitive effort to engage a professor is eased by the adoption of the appointment criteria (i.e., processing speed). Accordingly, cognitive biases, especially heuristics, and concretely dual-system models, serve as an appropriate theoretical basis for explaining the emergence of the underlying process on how appointment committee members weigh up a large number of different criteria when they evaluate the performance of candidates.
2.2 Influences on professorial appointment decisions
Researchers have used various methods to answer the question of what influences appointment decisions. First, several studies on professorial appointments analyzed documents, in which universities describe the criteria they will use to select candidates, for example, job advertisements (e.g., Finch et al. 2016; Gould et al. 2011; Klawitter 2017; Meizlish and Kaplan 2008; Pikciunas et al. 2016; Winter 1997) and policy documents (e.g., Crothall et al. 1997; Parker 2008; Subbaye 2018). The procedures and resulting contributions are manifold. For instance, Finch et al. (2016) analyzed job advertisements for tenure-track positions and found that business schools in the U.S. attach equal importance to research qualifications (i.e., at least one publication) and teaching qualifications (i.e., teaching experience). However, a major drawback of this approach is that the criteria written down in job advertisements, policy documents and official university documents may not always be the same criteria as those that appointment committees actually use (Finch et al. 2016; Subbaye 2018; van den Brink et al. 2010) and do not give insight into the individual preferences of the committee members who were involved in the decision-making process. Through the lens of cognitive biases, reports of appointment committees are potentially prone to several self-report biases, including introspective bias (e.g., Nisbett and Wilson 1977; Uhlmann et al. 2012) and social desirability (e.g., Arnold and Feldman 1981).
Second, analyzing career trajectories of scholars is one of the most common approaches to determine the criteria underlying the decisions of appointment committees. Based on datasets from scholars (e.g., performance, individual characteristics, appointment decision), researchers identify which variables are the best predictors for being successful in becoming a professor (e.g., Cruz-Castro and Sanz-Menendez 2010; Lutter and Schröder 2016; Pezzoni et al. 2012; Schulze et al. 2008; van Dijk et al. 2014; Youtie et al. 2013). Considering the results, career trajectory studies showed that different aspects of publication performance are related to scholars’ success in appointment procedures. For example, Sanz-Menéndez et al. (2013) found that the more researchers at Spanish universities publish before earning their doctoral degree, the sooner they get promoted to the level of professor. However, in collecting data on scholars’ characteristics, authors focus on information that is publicly available, thus neglecting many potentially relevant appointment criteria. Considering cognitive biases, career trajectory studies suffer from the so-called survivor bias (Jungbauer-Gans and Gross 2013; Lutter and Schröder 2016), which is problematic, as samples do not include the unsuccessful scholars who competed against the successful ones in appointment procedures.
Third, self-reports in the form of surveys and interviews are another common approach in the literature on appointment preferences. Some confirmed that appointment committees consider publication performance an important criterion, but they show that other criteria play a role as well, for example, acquiring grants, teaching performance, and the candidate’s fit to the hiring department (e.g., Macfarlane 2011, pp. 59–60; Sheehan et al. 1998). Studies highlight that appointment preferences are not homogeneous but that there are different appointment preferences depending on various factors. For instance, Landrum and Clump (2004) showed that candidates’ teaching performance is more important to private than to public institutions, whereas public institutions emphasize the number of publications and the acquisition of research grants. However, survey participants are typically asked to consider each appointment criterion independently and to state its importance on a ranking or rating scale. This approach has been criticized because participants tend to rate all items as similarly important (e.g., Orme 2014). Further, scholars’ answers may be biased because they are influenced by the public opinion as to which criteria should be important (i.e., social desirability bias; e.g., Arnold and Feldman 1981).
Up until now, a few studies on appointment preferences employed experimental designs (e.g., Kasten 1984; Steinpreis et al. 1999; Williams and Ceci 2015). During these experiments, narrative summaries or full CVs served as the basis for assessing appointment criteria. The shortcoming of such statistical approaches lies in not adapting to the participants’ responses, thus neglecting the underlying process on how scholars consider and weigh up many different appointment criteria when they evaluate candidates for a professorship. So far, Fiedler and Welpe (2008) are the only ones who applied conjoint analysis to research on professorial appointments. In contrast to narrative summaries, conjoint analysis uses hypothetical candidate profiles that consist of succinct descriptions of candidate characteristics, which reduces the complexity for participants. Thus, conjoint analysis enables the inclusion of more appointment criteria and the systematic variation of all criteria in a within-subject design. More specifically, the authors conducted an adaptive conjoint analysis, which allowed them to include many different appointment criteria, dynamically adapt those criteria to the respondents’ preferences and, in consequence, to study how scholars compare the performance of candidates. Fiedler and Welpe (2008) observe that, on average, prestigious journal publications are most important to management professors, followed by social competency, and person–subject fit. However, this type of conjoint analysis has also several drawbacks, because it does not mimic the decision-making process of appointment procedures. In contrast, adaptive choice-based conjoint (ACBC) analysis allows creating a more realistic and engaging simulation of a complex decision-making process with a variety of appointment criteria. Moreover, the procedure of ACBC analysis (e.g., using non-compensatory heuristics) facilitates focusing on the most important stimuli and providing precise estimations. Overall, the ACBC design allows for detailed insights about the ideal set of appointment criteria. Accordingly, the ACBC analysis validates and synthesizes previous research findings and extends them by providing a holistic, implicit process perspective on appointment preferences. More precisely, the differences between our study and that of Fielder and Welpe (2008) are threefold: first, in our study, we expanded and modified the appointment criteria based on recent literature and new preliminary studies. Second, in the Fiedler and Welpe (2008) study, participants selected their appointment criteria in advance, and only those appointment criteria were considered in the later stages of the survey, probably causing bias. Third, participants in the Fiedler and Welpe (2008) study rated their average candidates on percentages. In relation to actual personnel selection procedures, our design appears more realistic. In the following, we briefly review past findings on factors that contribute to differences in scholars’ appointment preferences, to consider which further aspects should be addressed as part of the ACBC analysis.
2.3 Differences in appointment preferences
Most previous studies that examined differences with regard to appointment criteria focused on differences among countries and among scientific fields. However, they either compared scientific fields in one specific country (e.g., Sanz-Menéndez et al. 2013; Williams and Ceci 2015) or compared countries with regard to one specific scientific field (e.g., Fiedler and Welpe 2008; Pezzoni et al. 2012). Past research also suggests that there are differences in appointment preferences between higher education institutions, which are related to specific organizational characteristics (e.g., Fiedler and Welpe 2008; Finch et al. 2016; Iyer and Clark 1998). This finding indicates that the current needs of departments (e.g., to improve their reputation) may influence how much importance appointment committees attach to specific criteria. Similarly, interviews with university presidents and deans indicate that the strategic objectives of the higher education institution and of the department are most important for defining the profile of an advertised professorship (Kleimann and Klawitter 2016). Although it can be assumed that individual appointment committee members differ in their opinion as to what aspects of scholarly performance are most important, little is known about whether there are systematic differences in appointment preferences depending on individual characteristics. Fiedler and Welpe (2008) provide initial evidence that homophily effects are important in explaining individual differences in appointment preferences between scholars. For example, professors had a stronger preference for candidates with international experience when they had international experience themselves. The homophily effect is accompanied by cognitive biases and may be explained with the dual-system perspective according to Morewedge and Kahneman (2010). In the example given, the presence of international experience may lead to other preferable qualities (e.g., superior language proficiency) being attributed to the candidate at the same time or the coherent and easy evaluation of international experience may result in increased process fluidity. In this context, a more systematic understanding of cognitive biases (e.g., homophily effects, heuristics) on appointment decisions is still lacking. In particular, it is unclear how scholars’ own performance in other areas, such as research, is related to differences in appointment preferences. Furthermore, it is unknown whether there are differences in appointment preferences depending on academic rank. Considering that appointment committees often comprise not only professors, it is crucial to also examine the appointment preferences of scholars who hold positions below the level of professor (e.g., post-doctoral fellows).
Past research reveals a multitude of factors that exert an influence on scholars’ appointment preferences, including country, scientific field, organizational characteristics, and individual characteristics. However, previous studies included only some of these factors while excluding others. Considering that all of the factors described above exert an influence on appointment preferences, it is crucial to identify distinct patterns of implicit appointment preferences (i.e., groups of scholars with similar preferences) and predict scholars´ patterns of implicit appointment preferences based on country, scientific field, as well as individual and organizational characteristics.
3 Method
To assess scholars’ implicit appointment preferences, we apply ACBC analysis (Johnson and Orme 2007). In the following, we describe research design, sample, procedure and conjoint exercise, as well as measures.
3.1 Research design
Conjoint analysis was initially applied to the experimental evaluation of consumer preferences (Green and Rao 1971). ACBC analysis provides a modified form of conjoint analysis, aiming at capturing implicit preferences of decision makers in complex decisions. Complex decisions, such as personnel selection, are defined by a multitude of choice alternatives and different selection criteria (Dijksterhuis et al. 2006). Such decisions involve a two-step process. First, screening of the alternatives takes place. Second, a final decision of choice is reached. In this process, so-called non-compensatory decision making additionally occurs, including simplifying heuristics such as must-have criteria (“cut-offs”). Our study design imitates this type of decision making by creating customized candidate profiles for each participant. Using participants' previous responses as a basis, the profiles are created in order to generate increasingly difficult trade-off decisions. More precisely, after screening all potential candidates and deciding who should be taken into further consideration (‘screening section’), appointment committees compare the remaining candidates to one another until they reach a final decision (‘choice task section’). Contrary to adaptive conjoint analysis (Fiedler and Welpe 2008), in which participants evaluate candidate profiles on a rating scale (e.g., how likely they would appoint the candidate from 0 to 100%), adaptive choice-based conjoint analysis requires participants to choose between candidates. Because choice tasks are more similar to the nature of personnel selection, they are better able to identify implicit preferences that underlie real-world decision behavior (Cunningham et al. 2010). The method takes into account that, “when people make real decisions, they often narrow down the choices to an acceptable consideration set (with respect to must-have and must-avoid features) and then make a final choice within the consideration set”.Footnote 1 Compared to previous methodological approaches, including the adaptive conjoint analysis of Fiedler and Welpe (2008), adaptive choice-based conjoint analysis allows to measure appointment preferences in a more realistic way and with greater validity.
3.2 Sample
We recruited junior and senior scholars from different countries by asking scientific associations to forward a survey invitation to their members. Here, we followed a systematic approach by using different distribution channels to generate the most representative sample possible. In particular, we contacted scientific associations from different fields that shared our request via e-mail and newsletters with their members. Furthermore, we posted our survey invitation to various list-servs of the Academy of Management. All participants who completed the survey had the opportunity to take part in a prize draw and win either a smart watch or a pair of noise-cancelling headphones. Of the 1637 participants who started the survey, we excluded 814 participants who dropped out of the survey early. From the remaining participants, we excluded 32 participants who were not members of the target sample, i.e., who were not scholars and most presumably not familiar with appointment criteria (students: n = 10; no researcher or not employed at a higher education institution: n = 22). Thirteen participants indicated that they had not answered the questions thoroughly in a follow-up question to the conjoint exercise or in a comment box. In addition, we excluded 15 participants with very low response times, specifically, participants who spent less than five seconds on three or more of the survey pages of the conjoint exercise. Further 82 participants had to be excluded due to missing values on variables that we used in the analyses. In total, we excluded 956 participants. The final sample comprised 681 participants. Figure 1 provides an overview of the data that we excluded to arrive at our final sample.
Fifty-three percent (n = 358) of the participants were male and the average age was 41.61 years (SD = 11.66). Sixty-three percent (n = 429) were professors (with 24% being full professors, n = 161), 15% were doctoral candidates (n = 104), and 22% held other positions between the level of doctoral candidate and professor (e.g., postdoctoral fellow or lecturer; n = 148). The majority of participants worked at doctorate-granting universities (85%, n = 576). Most participants were from Germany (37%, n = 252) or the U.S. (31%, n = 210) and worked in the social sciences (91%, n = 617; natural sciences: n = 22, humanities: n = 16, medical sciences: n = 14, engineering and technologies: n = 10, agricultural sciences: n = 2). Table 1 gives an overview of the quantity of scientific fields and the corresponding associations that we contacted.
Furthermore, Table 2 provides an overview of the cross-distribution of participants in terms of country and academic degree.
3.3 Procedure and conjoint exercise
In advance, for selecting and formulating attributes (i.e., appointment criteria) and levels (i.e., intensity or expression of the appointment criteria) for the conjoint analysis, we followed general recommendations in the literature (e.g., Aiman-Smith et al. 2002; Karren and Barringer 2002; Orme 2014). Based on a review of past research and on an analysis of job advertisements for professorships, we composed an initial list of eleven appointment criteria. To validate our choice, we conducted two pre-studies with experts on appointment procedures (scholars who had been appointment committee members, higher education researchers, and university representatives for professorial appointments).Footnote 2 The final list comprised 13 appointment criteria, which we used as attributes for the conjoint analysis:
-
1.
Number of publications
-
2.
Publication outlets
-
3.
Authorship of publications
-
4.
Research area
-
5.
Orientation toward mainstream
-
6.
Grants acquired
-
7.
Number of seminars and lectures
-
8.
Teaching activities
-
9.
Teaching evaluations
-
10.
Teaching concept
-
11.
Management and leadership experience
-
12.
International orientation
-
13.
Integration into scientific community
For all of these appointment criteria, at least one expert indicated that the criterion played a role in all or almost all appointment procedures. The list of appointment criteria includes both quantitative criteria (e.g., number of publications) and qualitative criteria (e.g., research area).
As recommended in the literature (Orme 2014), we chose three to five levels for each attribute―from very low to very high performance―to represent the full range of performance of candidates for professorships. The pre-studies and an additional pre-test of the questionnaire confirmed the appropriateness of the levels. Appendix 1 gives an overview of the attributes and levels used in the conjoint analysis, including the definitions shown to participants before and during the conjoint tasks.
To implement and host the survey, we used Lighthouse Studio (Version 9) by Sawtooth Software, Inc.. The first part of the survey comprised questions that were important for later stages of the survey (e.g., country, current position). In part two, participants took part in the conjoint exercise, which will be described in more detail in the following. In addition, part two included several follow-up questions (Aiman-Smith et al. 2002; Hair et al. 2014) and a measure of participants’ explicit appointment preferences. Part three consisted of measures of individual and organizational characteristics (e.g., participants’ rating of their own scholarly performance or their perception of politics at their higher education institution), which we used as predictors in a segmentation analysis.
In the introduction of the conjoint exercise, participants were asked to imagine that, at the department where they are currently employed, the position of a full professor is vacant, and that they serve as a member on the appointment committee. Subsequently, they saw a list of the appointment criteria (attributes) that the committee would use to evaluate the candidates, including a definition of each criterion.
In the next section (usually referred to as build-your-own section of adaptive choice-based conjoint studies), participants described the performance of an average candidate for a full professorship in their own scientific subfield. Because the pre-studies suggested that differences among scientific fields are most likely for the criteria number of publications, authorship of publications, number of seminars and lectures, and grants acquired, participants described the average candidate only with regard to these four attributes. We first created the levels of attributes (e.g., for publications: 10, 50, 100, 200, 300 publications) based on a ranking of a reputable German-language business and financial periodical and then validated these levels using expert interviews. Based on the levels that a participant selected to be average, the design algorithm of the software created a set of candidate profiles that the participant would evaluate in the subsequent screening section. The set of candidate profiles for each participant comprised the full range of levels for each attribute, but levels below or above the average appeared less often in the candidate profiles. Consequently, the candidate profiles were more realistic and plausible for participants. Levels of attributes that were not included in the build-your-own section were equally likely to appear in the candidate profiles of the screening section. We increased the number of attributes per candidate profile that deviate from the average level to the maximum of three, which significantly reduced the survey length (Goodwin 2013; Orme 2009).
In the screening section, participants were told that 24 candidates had applied for the professorship and that they are expected to screen the potential candidates. The screening section comprised six tasks, that is, the 24 candidate profiles were shown on six survey pages with four profiles each. On every page, participants indicated whether they would further consider a candidate or not, separately for each candidate. In between the screening tasks, we asked participants to select characteristics that they consider to be unacceptable or a must-have. Based on the participants’ answers to the screening tasks, the design algorithm of the software compiled a list of levels that the participants might consider to be unacceptable (e.g., “Only co-authorship”) or a must-have (e.g., “At least: Acceptable teaching concept”). Participants were asked to choose from this list the one characteristic that was most unacceptable or most important to them, respectively. They could also indicate that none of the levels was totally unacceptable or an absolute requirement. Every time a participant selected an unacceptable or must-have characteristic, the set of candidate profiles for the subsequent screening tasks was adapted so that from then on, all candidates fulfilled this requirement.
The introductory text of the choice task section informed the participants that there are still candidates left after screening the applicant pool and that the appointment committee has to make a final decision. Every page of the choice task section showed three candidate profiles and participants were asked to select the candidate who would be the best choice. Levels that were the same for all three candidates in one choice task were grayed out so that participants could focus only on the differences. Up to 13 of the 24 candidate profiles were brought forward from the screening section to the choice task section, depending on the number of candidate profiles a participant marked as “would further consider this candidate” in the screening section. Consequently, the choice task section comprised between one and six choice tasks. One participant skipped the choice task section because she selected only two candidates in the screening section.
After the conjoint exercise, we assessed participants’ explicit appointment preferences by asking them to select—from the list of all 13 attributes—the three criteria that had been most important to them for choosing among the candidates. The following figure illustrates our research design (Fig. 2).
Research Design (according to Brand and Baier 2020)
3.4 Measures
Participants rated their own scholarly performance with regard to research, teaching, and the acquisition of research grants (Ringelhan et al. 2013). They were asked to indicate, on a 5-point scale from 1 (less successful) to 5 (more successful), how successful their career development has been so far compared with other scientists in their scientific subfield (1) at their institution, (2) in the country they currently work in, and (3) worldwide. The scientific subfield that the participants had selected at the beginning of the survey was inserted automatically into the question (e.g., “compared with other scientists in psychology”). The items with regard to research performance, teaching performance, and grants performance were averaged, respectively. All three scales were reliable (research performance: α = 0.82, teaching performance: α = 0.87, grants performance: α = 0.88).
We used the scale by Murphy (1992) to assess participants’ leadership self-efficacy, that is, the “confidence in the knowledge, skills, and abilities associated with leading others” (Hannah et al. 2008, p. 669). The scale comprises eight items, of which two were slightly adapted by replacing the terms “students” and “peers” with “scientists”, for example, “I know a lot more than most scientists about what it takes to be a good leader”. Participants gave their answers on a 5-point scale (1 strongly disagree, 2 disagree, 3 neither agree or disagree, 4 agree, 5 strongly agree). Cronbach’s alpha was 0.84.
Additionally, the following item measured the participants’ leadership experience: “When it comes to leadership, I have…” (1 No experience, 4 Some experience, 7 Extensive experience). Three items measured the importance of grants, that is, the extent to which acquiring research grants is important to the participant’s department (e.g., “The department of the institution I currently work for stresses the importance of acquiring research grants”). Participants answered these questions on a 7-point scale from 1 (strongly disagree) to 7 (strongly agree). Cronbach’s alpha was 0.84. Furthermore, we used three of six items of the perceptions of politics scale (Hochwarter et al. 2003; Treadway et al. 2005) to measure participants’ “individual observation of others’ self-interested behaviors, such as the selective manipulation of organizational policies” (Treadway et al. 2005, p. 872). We slightly adapted the wording so that the items referred to the higher education institution at which the participants currently work, for example, “In this institution, people are working ‘behind the scenes’ to ensure they get their piece of the pie”. The items we used had the highest factor loadings in the study by Hochwarter et al. (2003). Participants gave their answers on a 7-point scale (1 strongly disagree, 7 strongly agree). Cronbach’s alpha was 0.88. The following item measured the participants’ appointment experience: “When it comes to appointment procedures, I have…” (1 No experience, 4 Some experience, 7 Extensive experience).
Further, we operationalized the discrepancy between implicit and explicit appointment preferences as follows. We assigned a value of 1 to a criterion if there was a discrepancy between a participant’s implicit and explicit preferences. There was a discrepancy if (1) the criterion was among the three criteria with the highest relative attribute importances (i.e., implicit preference based on conjoint analysis), but the participant had not selected it as one of the three most important criteria (explicit preference), or (2) the participant had selected it as one of three most important criteria (explicit preference), but it was not among the three criteria with the highest relative attribute importances (implicit preference). In turn, we assigned a value of 0 to a criterion if there was no discrepancy between implicit and explicit preference. For the three criteria a participant had selected to be most important (explicit preferences), we also measured the degree of discrepancy. If there was no discrepancy, we again assigned a value of 0. If the criterion was not among the three criteria with the highest relative attribute importances (implicit preference), we assigned values from 1 (rank 4 of relative attribute importances) to 10 (rank 13 of relative attribute importances).
4 Results
The results are structured as follows. First, we show scholars’ average, implicit appointment preferences, using counting analysis and the Hierarchical Bayes estimation. Second, we report the discrepancies between scholars’ implicit and explicit preferences. By identifying current appointment preferences in higher education and analyzing scholars’ implicit preferences for performance criteria, we address the first aim of our study. Third, we present clusters of scholars who have different patterns of appointment preferences, using segmentation analysis. By providing a more comprehensive overview of the factors that contribute to differences in appointment decisions, we address our second aim.
4.1 Counting analysis
In the build-your-own section, most participants described an average candidate as a scholar who has written 50 publications, has published mainly together with other scholars, has acquired $ 100,000 through research grants, and has given 25 seminars or lectures. In the screening section, participants selected an average of 13.85 candidates (SD = 3.66, range: 2–24) as “would further consider this candidate”. In accordance with the build-your-own section, about one fourth of participants indicated (1) that candidates should have at least 50 publications and at least few publications in top-tier journals, (2) that they should have acquired at least $ 100,000 through research grants, and (3) that they should have held at least 25 seminars and lectures. Approximately one of six participants reported that they require candidates to have teaching evaluations with an average result of at least 3 out of 5, and one of eight participants requires candidates to have a teaching concept of at least acceptable quality. On average, participants marked 1.53 attribute levels as must have (SD = 1.03, range: 0–5) and 1.85 attribute levels as unacceptable (SD = 1.26, range: 0–8).
4.2 Hierarchical Bayes estimation
We used hierarchical Bayes (HB; see, e.g., Kruschke et al. 2012; Lenk et al. 1996) to estimate the part-worth utilities of the attribute levels in Lighthouse Studio. Table 3 shows the part-worth utilities of the attribute levels and the relative attribute importances from the HB analysis. On average, the appointment criterion publication outlets had the largest influence on participants’ choices during the conjoint exercise, as reflected by the highest relative attribute importance (14.13%). The second most important attributes were number of publications (12.07%) and grants acquired (11.82%). The confidence intervals of these two latter attribute importances overlap, indicating that there is no significant difference. The least important attributes were orientation toward mainstream (4.00%), management and leadership experience (3.71%), and candidates’ fit to the department, that is, research area (4.43%) and teaching activities (3.58%). The part-worth utilities indicate participants’ preferences for the different attribute levels, and non-overlapping confidence intervals indicate that scholars’ preference for one level is significantly higher than their preference for another level. For example, candidates who have mainly published alone (mainly single authorship) are preferred the most (13.13) and candidates who have only published together with other scholars (only co-authorship) are preferred the least (− 16.47). The preference for the other two levels, that is, only single authorship (0.00) and mainly co-authorship (3.33), lies in between the highest and the lowest part-worth utilities. They do not differ significantly from each other, as indicated by overlapping confidence intervals.
4.3 Discrepancies between implicit and explicit appointment preferences
The appointment criteria differed with regard to both overall discrepancy and type of discrepancy (see Fig. 3). The criteria number of publications, grants acquired, and number of seminars and lectures showed the highest overall discrepancy between implicit and explicit preferences. For each of these criteria, about one third of the participants had discrepant preferences. In comparison, the preferences for the criteria orientation toward mainstream and teaching activities were discrepant for only 6% of the participants. The criteria number of publications, number of seminars and lectures, and grants acquired (i.e., all of the quantitative criteria except of teaching evaluations) were discrepant mostly because scholars implicitly prefer these criteria but do not report them to be important. Only few participants had discrepancies regarding quantitative criteria because they had explicit but not implicit preferences for the criteria. In turn, this type of discrepancy was typical for qualitative appointment criteria, particularly for authorship of publications, research area, management and leadership experience, and integration into scientific community. For example, 170 participants had a discrepancy between their implicit and explicit preference for authorship of publications. Most of these participants (72%, n = 122) reported that it was one of three most important criteria to them for choosing among the candidates while it was, in fact, not one of the three most important criteria according to the relative attribute importance.
The sum of the degree of discrepancy over all three explicitly preferred appointment criteria (i.e., criteria that a participant selected as one of the three most important criteria) showed that there are considerable individual differences among scholars. On average, the participants had an overall degree of discrepancy of 5.96 (SD = 4.85, range = 0–24). Only 13% of the participants had no discrepancy between their implicit and explicit appointment preferences, that is, the three criteria that they reported to be the most important to them for choosing among the candidates were the criteria with the highest relative attribute importance.
4.4 Segmentation analysis
Segmentation analysis is a technique employed in the behavioral sciences and psychology to divide a larger target group into distinct and homogeneous subgroups, i.e., segments. These segments are comprised of individuals who share similar characteristics, needs, attitudes, or behaviors. In order to identify distinct patterns of appointment preferences and explore how differences among scholars are related to differences in their appointment preferences, we conducted a segmentation analysis. We followed the approach by Deal (2014), who proposes a two-level segmentation process combining unsupervised and supervised machine learning methods (see Fig. 4). In Level 1, we segmented the sample of participants into subsamples based on their appointment preferences. In Level 2, we used covariates to predict the clusters build in Level 1Footnote 3 (Fig. 6 depicts the 14 covariates we used as predictors of cluster membership). In doing so, we were able to uncover hidden patterns within the data, as this method unveiled previously undiscovered segments or clusters of scholars with similar appointment preferences.
Two-level segmentation process (adapted from Deal 2014)
Figure 5 shows the relative attribute importances for each of the three clusters, which allows inferences about the trade-offs. The clusters vary widely regarding the question of which appointment criteria are most important for evaluating candidates. Participants in the “research” cluster have a strong preference for candidates who have published in top-tier journals and written a large number of publications. Many participants require candidates to have at least 50 publications and to have at least a few publications in top-tier journals; one out of ten participants even requires candidates to have published mainly in top-tier journals. The third most important attribute for participants in the research cluster is grants acquired but it is only half as important as the most important attribute publication outlets, implying that participants in this cluster would be likely to accept candidates with a sufficient number of publications to perform less well in other criteria, such as grants (which illustrates the trade-off that commission members make). Furthermore, participants in the research cluster had less discrepancies between their implicit and explicit appointment preferences than participants in the other two clusters. Participants in the “grants” cluster attach most importance to grants acquired. For 30% of participants, it is an absolute requirement that candidates have acquired at least $ 100,000. The second most important attributes are number of seminars and lectures, teaching concept, and number of publications. Overall, the appointment preferences of participants in the grants cluster are more balanced than those of participants in the other two clusters, as suggested by a smaller range between the least important attribute (teaching activities: 3.89%) and the most important attribute (grants acquired: 14.06%). Participants in the “teaching” cluster have a strong preference for candidates with positive teaching evaluations. This attribute is more than twice as important as the second most important attributes publication outlets, number of publications, teaching concept, grants acquired, and number of seminars and lectures. For the majority of participants in the teaching cluster (64.01%) it is a must-have that candidates have at least teaching evaluations with an average of 3 out of 5; one out of ten participants requires candidates to have teaching evaluations of at least 4 out of 5.
Figure 6 depicts the variable importances (i.e., mean decrease in accuracy) of the final 3-clusters-solution (CCEA 2; see appendices 2–4) in Level 2 of the segmentation analysis. Variable importance indicates which predictor variables are most important for classifying respondents into the clusters, that is, which covariates distinguish the clusters the most. Among the covariates, country yielded the highest variable importance, followed by research performance, type of institution, importance of grants, and teaching performance.
In order to distinguish the clusters, we describe differences among the clusters regarding the covariates (i.e., predictors of cluster membership) by means of descriptive statistics, Kruskal–Wallis tests (for continuous variables), and Chi-square tests (for categorical variables). We found significant differences with regard to all covariates except of age (H(2) = 1.44, p = 0.487), gender (Χ2 = 5.78, p = 0.054), and leadership experience (H(2) = 3.77, p = 0.152). For example, participants in the research cluster reported a higher research performance than participants in the grants cluster and the teaching cluster. Participants in the teaching cluster reported a lower importance of acquiring grants, a higher teaching performance, and a higher leadership self-efficacy. Participants in the grants cluster have less appointment experience than participants in the teaching cluster and slightly less appointment experience than participants in the research cluster. Chi-square tests revealed a significant association between cluster membership and scientific field (Χ2 = 21.77, p < 0.001), type of institution (Χ2 = 22.65, p < 0.001), current position (Χ2 = 34.55, p < 0.001), and country (Χ2 = 95.29, p < 0.001), respectively. Scholars from not doctorate-granting institutions were less present in the research cluster (z = − 3.23) and more present in the teaching cluster (z = 2.53). Professors were less present in the grants cluster (z = − 2.39) and scholars holding a position other than professor or doctoral candidate were less present in the research cluster (z = − 2.10) and the teaching cluster (z = − 2.50) and were more present in the grants cluster (z = 3.62). Scholars from the U.S. were less present in the grants cluster (z = − 5.14) and more present in the teaching cluster (z = 5.57), whereas scholars from Germany were more present in the grants cluster (z = 3.98) and less present in the teaching cluster (z = − 3.58).
5 Discussion
Conducting an ACBC analysis, we found that appointment preferences are not homogeneous across all scholars. Instead, there are different patterns of appointment preferences, which are related to factors such as country, organizational characteristics of higher education institutions and departments, and individual differences between scholars. Furthermore, our study revealed that scholars have three different patterns of appointment preferences. With regard to the results of prior work that in part contradict our findings, our study offers the following explanations: While a few studies identify publication performance as the most important criterion for professorial appointments (e.g., Lutter and Schröder 2016), interviews and reports conclude that the acquisition of grants (Macfarlane 2011) as well as teaching performance and candidate fit (Fuerstman and Lavertu 2005; Sheehan et al. 1998) play a central role in appointment decisions. Interestingly, these three criteria (i.e., number of publications, number of seminars and lecturers, and grants acquired) reveal the greatest discrepancy between explicit and implicit preferences. Applying the ACBC analysis, we have demonstrated that the discrepancy arises primarily because scholars prefer these criteria implicitly, but do not mention them explicitly. Thus, the mismatches in research may arise, first, from the methods used. For example, interviews deliberately create a trusting environment so that participants more likely mention their actual preferences (such as fit of the candidate). Second, the mismatches may be explained by other factors that contribute to differences in the decision. For instance, scholars from Germany attach greater importance to the acquisition of research grants, whereas scholars from the U.S. and from not doctorate-granting institutions attach greater importance to teaching evaluations. Accordingly, it matters considerably where the data has been collected. In the following, we will discuss the theoretical and practical implications in more detail.
5.1 Contributions
A major contribution of our study is the adoption of a comprehensive method. In contrast to methodological approaches used in previous work, ACBC analysis takes into account that scholars make trade-off decisions between appointment criteria because candidates are likely to fulfil some but not all expectations. By mimicking the decision process of appointment decisions, the method provides new insights into implicit preferences of appointment committee members, which affect how they evaluate job candidates and the outcome of appointment decisions. More generally, we suggest that ACBC analysis opens up new possibilities to study complex decisions like personnel selection decisions in a realistic and detailed manner. Our study demonstrates the great potential of ACBC analysis as a superior method to further enhance our understanding of recruiters’ implicit preferences for selection criteria and the use of non-compensatory decision rules.
Previous research reveals contradictory results. The reasons for these contradictory results are twofold: First, different methods produce mixed, incomplete results. Second, previous studies focus on specific countries or scientific fields, leading to limited results. We have demonstrated that ACBC is capable of providing holistic and valid results, accounting for differences in terms of countries, scientific fields, etc., while gathering data across multiple decisions in one run, reducing participant load, and increasing the accuracy of the results. Employing ACBC analysis to study scholars’ appointment preferences thus extends prior research in several ways. First, as we included more appointment criteria and provided participants with a greater amount of selection options than most previous studies (e.g., Kasten 1984; Steinpreis et al. 1999; Williams and Ceci 2015), we offer a more extensive overview of the criteria that play a role in professorial appointments. Second, as we subsequently identify patterns of implicit appointment preferences and determine variables that can predict scholars´ patterns of implicit preferences, we enhance the understanding of factors that contribute to differences in the importance of appointment criteria found in previous research. Compared to previous studies, our findings are based on an international and more diverse sample, including junior and senior scholars from different types of higher education institutions. We reveal differences in appointment preferences among countries (in total 48 countries, particularly between Germany and the U.S.). Furthermore, we show how organizational and individual characteristics are related to scholars’ appointment preferences. In doing so, we validate previous results and provide explanations for mismatches in the literature, which may be related, for example, to the chosen method. In this context, we analyze the influence of additional characteristics, such as scholars’ current position and the importance of acquiring research grants for the department.
Contrary to other studies in this area, we explain discrepancies between explicit and implicit appointment criteria, considering cognitive biases. Especially for quantitative appointment criteria, implicit preferences may arise from the features of associative memory outlined by Morewedge and Kahneman (2010). In the context of a professorial appointment, the number of publications or the amount of grants raised might be related to typical (possibly self-achieved) performance criteria (i.e., associative coherence) and other positive characteristics, such as high level of dedication and research performance (i.e., attribute substitution). In addition, quantitative criteria, i.e., measurable quantities, simplify and accelerate decision making (i.e., process fluidity), which is particularly important for professors with various cognitive responsibilities. Accordingly, we provide evidence of the underlying process on how scholars jointly consider and weigh up a large number of different appointment criteria when they evaluate the scholarly performance of candidates for professorships.
Moreover, our findings have practical implications for higher education institutions and scholars because we provide a more comprehensive understanding of appointment preferences in higher education. We enhance the transparency of appointment decisions, which is important for young scholars who make career decisions. Furthermore, we identify distinct patterns of appointment preferences, thus, helping scholars to understand, which performance criteria are important for successfully applying for full professorships (Sutherland 2017). In particular, scholars can benefit from knowing that their specific profile (e.g., a focus on teaching rather than research performance) is most advantageous when they apply for professorships at certain types of higher education institutions or in a specific country. Our findings are also helpful for higher education institutions. The knowledge that appointment committee members differ in their appointment preferences could be used to improve the selection process. For example, a selection process that ensures that all committee members have the chance to express their opinion on the candidates may result in a more well-balanced decision. The results also point to recent changes in the performance measurement practices in academia because the criteria that are considered important for getting appointed to a tenured professorship reflect those aspects of scholarly performance that are highly valued by higher education institutions and by the scientific community (Bianco et al. 2016; Jackson et al. 2017; Parker 2008).
5.2 Limitations and future research
The following limitations need to be taken into account in the interpretation of our findings and should be considered in future studies on appointment preferences. First, the procedure of appointing full professors differs between higher education systems in different countries (e.g., Laudel 2017). Being aware of these differences, we developed the scenario of our conjoint exercise to be as general as possible so that it would be realistic to scholars from different countries. Nevertheless, country-specific aspects related to the procedure of appointing full professors may have been neglected, limiting the generalizability of the results. Thus, specificities of appointment systems may lead to systematic differences in appointment preferences and should be examined in future research.
Second, most participants were from Germany or the U.S. and, despite the different distribution channels of the survey, mainly social scientists participated in our study. We assume that social scientists are more familiar with completing questionnaires than scholars from other scientific fields, which explains the high percentage of social scientists in our sample. To analyze the representativeness of our sample for social scientists in Germany and the U.S., we compared various recent statistical data from central sources (e.g., the German Federal Statistical Office). Based on data concerning the share of females in our sample and concerning the average age at appointment to professor ranks, we feel confident that our sample is representative for social scientists in Germany and the U.S. However, a larger number of participants from other countries and scientific fields is necessary to generalize our findings, of course.
Third, to take into account the multidimensionality of scholarly performance, we included many different appointment criteria. The large number of attributes are likely to have triggered participants to use simplifying heuristics (i.e., non-compensatory decision rules that focus on a small subset of all available criteria) and we contend that this situation accurately reflects the complexity of appointment decisions. Adaptive choice-based conjoint analysis can capture these non-compensatory decision rules through unacceptable and must-have criteria so that the large number of attributes and the use of non-compensatory decision rules does not impair but enhance the validity of our results. In fact, appointment criteria other than those we selected may play a role in appointment decisions as well (e.g., academic awards; Lutter and Schröder 2016; Sheehan and Haselhorst 1999). Accordingly, these other criteria were omitted from our study. Future studies could include additional criteria by using partial-profile designs, in which each choice task includes a random subset of all conjoint attributes (Orme 2014).
Fourth, according to the number-of-levels-effect, attributes with more levels tend to receive higher importance scores compared to attributes with fewer levels (e.g., Currim et al. 1981; De Wilde et al. 2008; Wittink et al. 1990). Therefore, the criteria number of publications, grants acquired, and number of seminars and lectures may be slightly less important than the results suggest, whereas candidates’ management and leadership experience may be slightly more important. In this context, one might also argue that the levels of the attributes especially for “number of publications” are quite high. However, we determined the levels based on a publicly available ranking and additionally validated them in expert interviews; thus, we are confident that these levels are at least suitable for the field of social sciences (and, in particular, for the management field). Another argument in support of an appropriate choice of levels is that the higher levels were (implicitly) preferred by the participants themselves. Future studies that place greater emphasis on other scientific fields should, however, re-examine the levels and, if necessary, lower the number of publications in particular.
Fifth, the ACBC analysis provided a more realistic and engaging simulation of a complex decision-making process with a variety of attributes at the individual level. However, the method neglects real-life interactions with other committee members and their influence on decision making. Thus, future research may investigate causes of discrepancy between implicit and explicit preferences, for instance, considering social desirability or a potential lack of self-insight. Finally, future studies should take into account that appointment decisions are not made by individual scholars but committees, that is, groups of scholars. Narayan et al. (2011) showed that individuals revise their preferences when they learn about the preferences of peers, particularly when they are unsure and peers are sure about their preferences. Considering that committee members usually differ in their expertise and their status (e.g., professors and doctoral candidates), it would be interesting to examine how they influence each other’s appointment preferences.
6 Conclusion
This study identified current appointment preferences in higher education. An ACBC analysis revealed scholars’ implicit preferences in the evaluation of candidates for a full professorship. On average, the three most important appointment criteria are the extent to which a candidate has published in top-tier journals, the total number of publications, and the sum of money acquired through research grants. Overall, the results suggest that scholars base their appointment decisions more on candidates’ research performance than on their teaching performance and that they rely more on quantitative criteria than on qualitative criteria to evaluate scholarly performance. In line with previous studies (e.g., Fiedler and Welpe 2008; Iyer and Clark 1998; Landrum and Clump 2004; Pezzoni et al. 2012), we found that appointment preferences are not homogeneous across all scholars. Heterogeneity might be explained through cognitive biases. Depending on individual experiences (e.g., self-achieved successes), country-specific professorial appointment selection procedures, and (regular, informal) interactions with commission members, heuristics emerge that simplify strategic decision making. Our results reveal that quantitative criteria in particular, being quick and easy to evaluate, are implicitly preferred. A segmentation analysis revealed that scholars have three distinct patterns of appointment preferences, which are related to factors such as country, organizational characteristics of higher education institutions and departments (e.g., type of higher education institution), and individual differences between scholars (e.g., scholars’ own research performance). Country is the most important predictor for scholars’ appointment preferences, serving to forecast the corresponding cluster. For example, scholars from the U.S. are more likely to attach most importance to teaching evaluations, whereas scholars from Germany are more likely to value the acquisition of grants above all other criteria. Considering cognitive bias theory, the study contributes to literature on professorial appointments and provides important insights for personnel selection research. Furthermore, our findings offer valuable practical implications for higher education institutions and scholars regarding appointment procedures and career decisions.
Data availability
The data that support the findings of this study are available from the corresponding author upon request.
Notes
The results of the pre-studies are available from the authors on request.
A detailed and technical description of the segmentation analysis is available from the authors on request.
References
Abbott A, Cyranoski D, Jones N, Maher B, Schiermeier Q, Van Noorden R (2010) Metrics: do metrics matter? Nature 465:860–862. https://doi.org/10.1038/465860a
Aguinis H, Shapiro DL, Antonacopoulou EP, Cummings TG (2014) Scholarly impact: a pluralist conceptualization. Acad Manag Learn Educ 13:623–639. https://doi.org/10.5465/amle.2014.0121
Aiman-Smith L, Scullen SE, Barr SH (2002) Conducting studies of decision making in organizational contexts: a tutorial for policy-capturing and other regression-based techniques. Organ Res Methods 5:388–414. https://doi.org/10.1177/109442802237117
Arnold HJ, Feldman DC (1981) Social desirability response bias in self-report choice situations. Acad Manag J 24:377–385. https://doi.org/10.5465/255848
Bianco M, Gras N, Sutz J (2016) Academic evaluation: universal instrument? Tool for development? Minerva 54:399–421. https://doi.org/10.1007/s11024-016-9306-9
Brand BM, Baier D (2020) Adaptive CBC: are the benefits justifying its additional efforts compared to CBC? Arch Data Sci 6:1–22. https://doi.org/10.5445/KSP/1000098011/06
Crothall J, Callan V, Härtel CEJ (1997) Recruitment and selection of academic staff: perceptions of department heads and job applicants. J High Educ Policy Manag 19:99–110. https://doi.org/10.1080/1360080970190202
Cruz-Castro L, Sanz-Menendez L (2010) Mobility versus job stability: assessing tenure and productivity outcomes. Res Policy 39:27–38. https://doi.org/10.1016/j.respol.2009.11.008
Cunningham CE, Deal K, Chen Y (2010) Adaptive choice-based conjoint analysis: a new patient-centered approach to the assessment of health service preferences. Patient 3:257–273. https://doi.org/10.2165/11537870-000000000-00000
Currim IS, Weinberg CB, Wittink DR (1981) Design of subscription programs for a performing arts series. J Consum Res 8:67–75. https://doi.org/10.1086/208842
Das TK, Teng BS (1999) Cognitive biases and strategic decision processes: an integrative perspective. J Manag Stud 36:757–778. https://doi.org/10.1111/1467-6486.00157
De Wilde E, Cooke ADJ, Janiszewski C (2008) Attentional contrast during sequential judgments: a source of the number-of-levels effect. J Mark Res 45:437–449. https://doi.org/10.1509/jmkr.45.4.437
Deal K (2014) Segmenting patients and physicians using preferences from discrete choice experiments. Patient 7:5–21. https://doi.org/10.1007/s40271-013-0037-9
Dijksterhuis A, Bos MW, Nordgren LF, Van Baaren RB (2006) On making the right choice: the deliberation-without-attention effect. Science 311:1005–1007. https://doi.org/10.1126/science.1121629
Fiedler M, Welpe IM (2008) “If you don’t know what port you are sailing to, no wind is favorable”: appointment preferences of management professors. Schmalenbach Bus Rev 60:4–31. https://doi.org/10.1007/BF03396757
Finch D, Deephouse DL, O’Reilly N, Massie T, Hillenbrand C (2016) Follow the leaders? An analysis of convergence and innovation of faculty recruiting practices in US business schools. High Educ 71:699–717. https://doi.org/10.1007/s10734-015-9931-5
Fuerstman D, Lavertu S (2005) The academic hiring process: a survey of department chairs. PS: Political Sci Politics 38:731–736. https://doi.org/10.1017/S1049096505050225
Goodwin RJ (2013) Quantitative marketing research solutions in a traditional manufacturing firm: update and case study. Proceedings of the sawtooth software conference October 2013, 13–37. https://www.sawtoothsoftware.com/training/conferences/100-support/proceedings/1426-proceedings2013
Gould LA, Fowler SK, del Carmen A (2011) Faculty employment trends in criminology and criminal justice. J Crim Justice Educ 22:247–266. https://doi.org/10.1080/10511253.2010.517771
Green PE, Rao VR (1971) Conjoint measurement for quantifying judgmental data. J Mark Res 8:355–363. https://doi.org/10.1177/002224377100800312
Hair JF, Black WC, Babin BJ, Anderson RE (2014) Multivariate data analysis (7th, new international ed.). Pearson.
Hannah ST, Avolio BJ, Luthans F, Harms PD (2008) Leadership efficacy: review and future directions. Leadersh Q 19:669–692. https://doi.org/10.1016/j.leaqua.2008.09.007
Haselton MG, Nettle D, Andrews PW (2005) The evolution of cognitive bias. In: Buss DM (ed) The handbook of evolutionary psychology. John Wiley & Sons, pp 724–746
Hochwarter WA, Kacmar C, Perrewe PL, Johnson D (2003) Perceived organizational support as a mediator of the relationship between politics perceptions and work outcomes. J Vocat Behav 63:438–456. https://doi.org/10.1016/S0001-8791(02)00048-9
Iyer VM, Clark D (1998) Criteria for recruitment as assistant professor of accounting in colleges and universities. J Educ Bus 74:6–10. https://doi.org/10.1080/08832329809601652
Jackson JK, Latimer M, Stoiko R (2017) The dynamic between knowledge production and faculty evaluation: perceptions of the promotion and tenure process across disciplines. Innov High Educ 42:193–205. https://doi.org/10.1007/s10755-016-9378-3
Johnson RM, Orme B (2007) A new approach to adaptive CBC. Sawtooth software research paper series. http://www.sawtoothsoftware.com/download/techpap/acbc10.pdf
Jungbauer-Gans M, Gross C (2013) Determinants of success in university careers: Findings from the German academic labor market [Erfolgsfaktoren in der Wissenschaft—Ergebnisse aus einer Habilitiertenbefragung an deutschen Universitäten]. Z Soziol 42:74–92. https://doi.org/10.1515/zfsoz-2013-0106
Karren RJ, Barringer MW (2002) A review and analysis of the policy-capturing methodology in organizational research: guidelines for research and practice. Organ Res Methods 5:337–361. https://doi.org/10.1177/109442802237115
Kasten KL (1984) Tenure and merit pay as rewards for research, teaching, and service at a research university. J High Educ 55:500–514. https://doi.org/10.1080/00221546.1984.11780662
Keltner D, Gruenfeld DH, Anderson C (2003) Power, approach, and inhibition. Psychol Rev 110:265–284. https://doi.org/10.1037/0033-295X.110.2.265
Klawitter M (2017) Die Besetzung von Professuren an deutschen Universitäten. Empirische Analysen zum Wandel von Stellenprofilen und zur Bewerber(innen)auswahl. Doctoral dissertation, Universität Kassel. https://kobra.uni-kassel.de/bitstream/handle/123456789/2017091253474/DissertationMarenKlawitter.pdf?sequence=7
Kleimann B, Klawitter M (2016) Governanceeffekte auf Berufungsverfahren Jahrestagung der Gesellschaft für Hochschulforschung, Munich (Germany).
Kruschke JK, Aguinis H, Joo H (2012) The time has come: Bayesian methods for data analysis in the organizational sciences. Organ Res Methods 15:722–752. https://doi.org/10.1177/1094428112457829
Landrum RE, Clump MA (2004) Departmental search committees and the evaluation of faculty applicants. Teach Psychol 31:12–17. https://doi.org/10.1207/s15328023top3101_4
Laudel G (2017) How do national career systems promote or hinder the emergence of new research lines? Minerva 55:341–369. https://doi.org/10.1007/s11024-017-9314-4
Lenk PJ, Desarbo WS, Green PE, Young MR (1996) Hierarchical Bayes conjoint analysis: recovery of partworth heterogeneity from reduced experimental design. Mark Sci 15:173–191. https://doi.org/10.1287/mksc.15.2.173
Lepori B, Seeber M, Bonaccorsi A (2015) Competition for talent. Country and organizational-level effects in the internationalization of European higher education institutions. Res Policy 44:789–802. https://doi.org/10.1016/j.respol.2014.11.004
Lutter M, Schröder M (2016) Who becomes a tenured professor, and why? Panel data evidence from German sociology, 1980–2013. Res Policy 45:999–1013. https://doi.org/10.1016/j.respol.2016.01.019
Macfarlane B (2011) Professors as intellectual leaders: formation, identity and role. Stud High Educ 36:57–73. https://doi.org/10.1080/03075070903443734
Meizlish D, Kaplan M (2008) Valuing and evaluating teaching in academic hiring: a multidisciplinary, cross-institutional study. J Higher Educ 79:489–512. https://doi.org/10.1080/00221546.2008.11772114
Morewedge CK, Kahneman D (2010) Associative processes in intuitive judgment. Trends Cogn Sci 14:435–440. https://doi.org/10.1016/j.tics.2010.07.004
Murphy SE (1992) The contribution of leadership experience and self-efficacy to group performance under evaluation apprehension. Doctoral dissertation. https://digital.lib.washington.edu/researchworks/bitstream/handle/1773/9167/9230410.pdf
Narayan V, Rao VR, Saunders C (2011) How peer influence affects attribute preferences: a Bayesian updating mechanism. Mark Sci 30:368–384. https://doi.org/10.1287/mksc.1100.0618
Nisbett RE, Wilson TD (1977) Telling more than we can know: verbal reports on mental processes. Psychol Rev 84:231–259. https://doi.org/10.1037/0033-295X.84.3.231
Orme B (2009) Fine-tuning CBC and adaptive CBC questionnaires. Sawtooth Software Research paper series. https://sawtoothsoftware.com/resources/technical-papers/fine-tuning-cbc-and-adaptive-cbc-questionnaires
Orme B (2014) Getting started with conjoint analysis: strategies for product design and pricing research. Research Publishers LLC. https://books.google.de/books?id=wHUsnwEACAAJ
Parker J (2008) Comparing research and teaching in university promotion criteria. High Educ Q 62:237–251. https://doi.org/10.1111/j.1468-2273.2008.00393.x
Pezzoni M, Sterzi V, Lissoni F (2012) Career progress in centralized academic systems: social capital and institutions in France and Italy. Res Policy 41:704–719. https://doi.org/10.1016/j.respol.2011.12.009
Pikciunas KT, Cooper JA, Hanrahan KJ, Gavin SM (2016) The future of the academy: who’s looking for whom? J Crim Justice Educ 27:362–380. https://doi.org/10.1080/10511253.2016.1142590
Pitz GF, Sachs NJ (1984) Judgment and decision: theory and application. Annu Rev Psychol 35:139–163
Ringelhan S, Wollersheim J, Welpe IM, Fiedler M, Spörrle M (2013) Work motivation and job satisfaction as antecedents of research performance: Investigation of different mediation models. In: Dilger A (ed) Performance management im Hochschulbereich. Springer Gabler, pp 7–38. https://doi.org/10.1007/978-3-658-03348-4_2
Sanz-Menéndez L, Cruz-Castro L, Alva K (2013) Time to tenure in Spanish universities: an event history analysis. PLoS ONE 8:e77028. https://doi.org/10.1371/journal.pone.0077028
Schulze GG, Warning S, Wiermann C (2008) What and how long does it take to get tenure? The case of economics and business administration in Austria, Germany and Switzerland. Ger Econ Rev 9:473–505. https://doi.org/10.1111/j.1468-0475.2008.00450.x
Sheehan EP, Haselhorst H (1999) A profile of applicants for an academic position in social psychology. J Soc Behav Personal 14:23–30
Sheehan EP, McDevitt TM, Ross HC (1998) Looking for a job as a psychology professor? Factors affecting applicant success. Teach Psychol 25:8–11. https://doi.org/10.1207/s15328023top2501_3
Steinpreis RE, Anders KA, Ritzke D (1999) The impact of gender on the review of the curricula vitae of job applicants and tenure candidates: a national empirical study. Sex Roles 41:509–528. https://doi.org/10.1023/A:1018839203698
Subbaye R (2018) Teaching in academic promotions at South African universities: a policy perspective. High Educ Pol 31:245–265. https://doi.org/10.1057/s41307-017-0052-x
Sutherland KA (2017) Constructions of success in academia: an early career perspective. Stud High Educ 42:743–759. https://doi.org/10.1080/03075079.2015.1072150
Treadway DC, Ferris GR, Hochwarter W, Perrewé P, Witt LA, Goodman JM (2005) The role of age in the perceptions of politics–job performance relationship: a three-study constructive replication. J Appl Psychol 90:872–881. https://doi.org/10.1037/0021-9010.90.5.872
Uhlmann EL, Leavitt K, Menges JI, Koopman J, Howe M, Johnson RE (2012) Getting explicit about the implicit: a taxonomy of implicit measures and guide for their use in organizational research. Organ Res Methods 15:553–601. https://doi.org/10.1177/1094428112442750
van den Brink M, Benschop Y, Jansen W (2010) Transparency in academic recruitment: a problematic tool for gender equality? Organ Stud 31:1459–1483. https://doi.org/10.1177/0170840610380812
van Dijk D, Manor O, Carey LB (2014) Publication metrics and success on the academic job market. Curr Biol 24:R516–R517. https://doi.org/10.1016/j.cub.2014.04.039
Williams WM, Ceci SJ (2015) National hiring experiments reveal 2:1 faculty preference for women on STEM tenure track. Proc Natl Acad Sci 112:5360–5365. https://doi.org/10.1073/pnas.1418878112
Winter PA (1997) Faculty position advertisements in educational administration: Analysis and a theoretical framework for improving administrative practice Annual meeting of the national council of professors of educational administration, Vail, CO.
Wittink DR, Krishnamurthi L, Reibstein DJ (1990) The effect of differences in the number of attribute levels on conjoint results. Market Lett 1:113–123
Youtie J, Rogers J, Heinze T, Shapira P, Tang L (2013) Career-based influences on scientific recognition in the United States and Europe: longitudinal evidence from curriculum vitae data. Res Policy 42:1341–1355. https://doi.org/10.1016/j.respol.2013.05.002
Funding
Open Access funding enabled and organized by Projekt DEAL. This work was supported by the German Federal Ministry of Education and Research [grant number 01PY13012]. The software Lighthouse Studio and the software Convergent Cluster & Ensemble Analysis (CCEA) was provided by Sawtooth Software Inc. as part of a student grant.
Author information
Authors and Affiliations
Contributions
LG, JS-W and IW contributed to the study conception and design. Conceptualization were performed by LG, JS-W and IW. Data curation, formal analysis, methodology, validation, visualization, and the writing of the first draft of the manuscript was executed by LG. MR, LG and JS-W revised previous versions of the manuscript. All authors read and approved the final manuscript.
Corresponding author
Ethics declarations
Conflict of Interest
The authors have no relevant financial or non-financial interests to disclose.
Ethical approval
Not applicable.
Consent to participate
All authors agreed with the content, gave explicit consent to submit, and obtained consent from the responsible authorities at the institute/organization where the work has been carried out.
Additional information
Publisher's Note
Springer Nature remains neutral with regard to jurisdictional claims in published maps and institutional affiliations.
Appendix
Appendix
See Appendix Tables 4, 5, 6, 7.
Rights and permissions
Open Access This article is licensed under a Creative Commons Attribution 4.0 International License, which permits use, sharing, adaptation, distribution and reproduction in any medium or format, as long as you give appropriate credit to the original author(s) and the source, provide a link to the Creative Commons licence, and indicate if changes were made. The images or other third party material in this article are included in the article's Creative Commons licence, unless indicated otherwise in a credit line to the material. If material is not included in the article's Creative Commons licence and your intended use is not permitted by statutory regulation or exceeds the permitted use, you will need to obtain permission directly from the copyright holder. To view a copy of this licence, visit http://creativecommons.org/licenses/by/4.0/.
About this article
Cite this article
Graf, L., Rimbeck, M., Stumpf-Wollersheim, J. et al. Academic success is in the eye of the beholder: understanding scholars’ implicit appointment preferences through adaptive choice-based conjoint analysis. J Bus Econ (2023). https://doi.org/10.1007/s11573-023-01184-2
Accepted:
Published:
DOI: https://doi.org/10.1007/s11573-023-01184-2
Keywords
- Appointment preferences
- Higher education
- Adaptive choice-based conjoint analysis
- Implicit preferences
- Decision-making
- Personnel selection