Abstract
Background
Despite the clear clinical diagnostic criteria for necrozoospermia in andrology, the fundamental mechanisms underlying it remain elusive. This study aims to profile the lipid composition in seminal plasma systematically and to ascertain the potential of lipid biomarkers in the accurate diagnosis of necrozoospermia. It also evaluates the efficacy of a lipidomics-based random forest algorithm model in identifying necrozoospermia.
Methods
Seminal plasma samples were collected from patients diagnosed with necrozoospermia (n = 28) and normozoospermia (n = 28). Liquid chromatography–mass spectrometry (LC–MS) was used to perform lipidomic analysis and identify the underlying biomarkers. A lipid functional enrichment analysis was conducted using the LION lipid ontology database. The top 100 differentially significant lipids were subjected to lipid biomarker examination through random forest machine learning model.
Results
Lipidomic analysis identified 46 lipid classes comprising 1267 lipid metabolites in seminal plasma. The top five enriched lipid functions as follows: fatty acid (FA) with ≤ 18 carbons, FA with 16–18 carbons, monounsaturated FA, FA with 18 carbons, and FA with 16 carbons. The top 100 differentially significant lipids were subjected to machine learning analysis and identified 20 feature lipids. The random forest model identified lipids with an area under the curve > 0.8, including LPE(20:4) and TG(4:0_14:1_16:0).
Conclusions
LPE(20:4) and TG(4:0_14:1_16:0), were identified as differential lipids for necrozoospermia. Seminal plasma lipidomic analysis could provide valuable biochemical information for the diagnosis of necrozoospermia, and its combination with conventional sperm analysis may improve the accuracy and reliability of the diagnosis.
Similar content being viewed by others
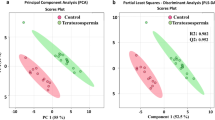
Avoid common mistakes on your manuscript.
1 Background
Necrozoospermia was defined as the presence of less than 58% of alive spermatozoa in semen according to the sixth edition of World Health Organization manual, the test to assess sperm vitality is Eosin-nigrosin staining (WHO, 2021). Necrozoospermia, with an incidence of approximately 0.2–0.5% in male infertility (Boursier et al., 2022). The causes of necrozoospermia are multiple: genital tract infections, testicular hyperthermia, varicocele, hyperthyroidism, spinal cord injury, polycystic kidney disease, anti-sperm antibodies, advanced male age, toxic substances, In 20% of total cases, the cause of necrozoospermia is unknown (Agarwal et al., 2022). There was a relatively limited approach to the management of necrozoospermia, for example: avoiding and treating underlying risks and conditions, frequent ejaculation, drug. However, the treatment effect is not good, and the majority of patients finally obtain pregnancy through assisted reproductive technology, and the sperm was which the use of ICSI with ejaculated or surgically extracted spermatozoa. Magnetic-activated cell sorting was a novel methods for the elimination of dead spermatozoa (Máté et al., 2022). Our previous preliminary research suggests that the occurrence of necrozoospermia is associated with choline and the arachidonic acid metabolic pathway (Deng et al., 2023).
Seminal plasma contains rich substance components, including sugars, lipids, proteins, and trace elements. These components have the potential to influence the occurrence and development of spermatozoa, while also objectively reflecting the functional status of it (Boguenet et al., 2020). Lipids in seminal plasma include cholesterol (free and esterified cholesterol), TGs, phospholipids (SM, PC, and PE), and prostaglandins of the E series. A previous study indicated that alterations in lipid composition in seminal plasma can impact the fertility of spermatozoa, potentially leading to the loss of fertilization function and causing male infertility (Kawano et al., 2011). Therefore, research on the lipid composition of seminal plasma holds significant importance. Lipids play a crucial role in spermatozoa maturation, energy acquisition, and fertilization processes (Kawano et al., 2011). Seminal plasma, as a non-invasive sample, is particularly suitable for discovering the causes of unexplained male infertility and conducting mechanism studies.
Lipidomics, a high-throughput analytical technique based on liquid chromatography–mass spectrometry (LC–MS), systematically analyzes the lipid composition and expression patterns in biological systems (Dalli et al., 2018). Lipidomic analysis efficiently investigates changes in lipid families and compounds during various biological processes, elucidating relevant biological mechanisms and principles.
This study aims to systematically profile the lipid composition in seminal plasma and to ascertain the potential of lipid biomarkers in the accurate diagnosis of necrozoospermia. It also evaluates the efficacy of a lipidomics-based random forest algorithm model in identifying necrozoospermia.
2 Materials and methods
2.1 Semen sample collection and analysis
The study included healthy donors and infertility patients from the Reproductive Medicine Center at Shenzhen Maternity & Child Healthcare Hospital (SZMCH) and approved by the ethics committee (No. SFYLS [2021]038). There are 28 participants in the control group, volunteers had at least two normal semen analysis results, meeting laboratory manual for the examination and processing of human semen (WHO 6th edition) (WHO, 2021). Volunteers in control group were healthy, free from genetic diseases, and sought consultation owing to female-related infertility factors. The case group comprised 28 patients who have been diagnosed as necrozoospermia, with normal hormone levels and no other abnormal physical conditions. Patients were included in the necrozoospermia group if they met the following criteria: spermatozoa forward motility percentage of 0%, spermatozoa viability (eosin–nigrosin staining) < 58%, and sperm concentration equal to or higher than the lower limit of the reference range.
The exclusion criteria were as follows: obesity; metabolic diseases, such as diabetes and thyroid dysfunction; recent acute urinary tract infections, chlamydia, or mycoplasma infections; leukocytospermia; exposure to substances toxic to the reproductive system; abstinence time > 7 days or < 2 days; semen volume < 1 mL.
Computer-assisted semen analysis (CASA) system has been used to analyze the volume, concentration, and motility. Eosin–nigrosin staining was employed to assess spermatozoa viability, and Diff–Quik staining was used for spermatozoa morphology analysis. The remaining sperm was centrifuged at 3000×g for 15 min, and seminal plasma samples were stored at − 80 °C for subsequent lipidomic analysis.
2.2 Lipid extraction
Chloroform–methanol solution (2:1, v/v) was added to the sample at − 20 °C. After a series of repeated vortexing and centrifugation for purification, the sample was concentrated using a vacuum concentrator. Finally, the sample was dissolved in isopropanol, filtered through a 0.22 μm membrane, resulting in the test sample for subsequent LC–MS steps.
2.3 LC–MS analysis
The instrument utilized an electrospray ionization source (ESI) operating in both positive and negative ionization modes. The positive ion spray voltage was set at 3.50 kV, and the negative ion spray voltage was set at 2.50 kV. The sheath gas was maintained at 30 arb, and the auxiliary gas at 10 arb. The capillary temperature was set to 325 °C, and a resolution of 35,000 was employed for full scan analysis within the range of 150 to 2000. Higher-energy collisional dissociation (HCD) was used for second-stage fragmentation with a collision voltage of 30 eV. Dynamic exclusion was implemented to eliminate unnecessary MS/MS information (Dalli et al., 2018).
2.4 Data processing
The raw data obtained from MS were individually processed using LipidSearch software (V4.2.28; Thermo Fischer Scientific). This involved lipid annotation, peak alignment, and peak filtering. The key parameters included retention time (R.T.) tolerance = 0.25, m-score threshold = 3. The outcome was a quantitative list containing information, such as lipids, mass-to-charge ratio (m/z), retention time, and peak intensity. To facilitate comparison among data of different magnitudes, sum peak normalization was performed to correct quantitative values.
2.5 Statistical and bioinformatical analysis
Owing to the multidimensional nature of lipidomic data and the high correlation between certain variables, traditional univariate analysis falls short in rapidly, comprehensively, and accurately mining potential information within the data. Therefore, before conducting multivariate statistical analysis on lipidomic data, it is necessary to appropriately transform the data through weighting, i.e., scaling. In this analysis, a self-weighted approach was applied to the data prior to sample classification to obtain more reliable and intuitive results. The multivariate statistical analysis methods employed in this analysis, utilizing the R package Ropls, include (1) principal component analysis (PCA); (2) partial least squares-discriminant analysis (PLS-DA); and (3) orthogonal PLS-DA (OPLS-DA).
The Statistical Package for Social Sciences for Windows (SPSS 26, IBM Corp., Armonk, NY) was used for statistical analyses. A p-value < 0.05 was considered statistically significant. The distribution of data was evaluated by using the Kolmogorov–Smirnov test. Student's t-test or the Mann–Whitney Wilcoxon test was used for comparisons.
For correlation analysis, correlation coefficients tending towards 1 or − 1 indicate a strong linear relationship. A positive correlation tends towards 1, and a negative correlation towards − 1. Statistical testing of lipid association analysis was conducted using the cor.test() function in R, with a p value of less than 0.05 indicating significant correlation (Kawano et al., 2011).
The R package lipidomeR was employed for carbon chain length and carbon saturation analysis of lipids. Correlation network analysis was conducted for differential lipids, and the Leiden community classification algorithm was used for community classification of associated differential lipids. For lipids classified into different communities, the R package for LION software was used for functional enrichment analysis. Lipid functional enrichment analysis utilized the LION lipid ontology database (Molenaar et al., 2019), which links over 50,000 lipid species to four major branches: (1) lipid classification (LIPIDMAPS classification hierarchy); (2) chemical and physical properties (e.g., fatty acid [FA] length and unsaturation, functional group charge, and membrane fluidity); (3) function; and (4) subcellular components (major subcellular localization).
3 Results
3.1 Semen analysis
There were no statistically significant differences in age and abstinence time between the two groups (p > 0.05). However, there were statistically significant differences between the two groups in semen volume, pH value, spermatozoa concentration, forward motility percentage, percentage of normal spermatozoa morphology, survival rate, seminal plasma neutral α-glucosidase, and seminal plasma zinc levels (p < 0.05) (Table 1).
3.2 Quality control of LC–MS
The PCA score plots (Fig. 1A) show minimal variation in QC samples, indicating high method stability and data quality, with good reproducibility evidenced by clustering within the 95% confidence interval. About 79.6% of feature peaks in QC samples had an RSD < 30%, ensuring high-quality data for biomarker detection (Fig. 1B). Lipids were categorized into groups. In the control group, 53 lipid categories with 1591 species were identified, and the top 10 are shown in a pie chart (Fig. 1C). Similarly, the experimental group had 53 categories with 1592 lipid species, with the top 10 displayed in a pie chart (Fig. 1D).
LC–MS sample quality control. A PCA score plot: x-axis shows the first principal component, y-axis the second; red dots are QC samples showing good reproducibility within 95% confidence interval, green dots are non-QC samples. B RSD distribution plot: left axis shows proportion, right axis shows quantity, horizontal axis shows RSD range. C Control group lipid classification pie chart. D Necrozoospermia group lipid classification pie chart. PCA principal component analysis; RSD relative standard deviation; BisMePA bis-methylphosphatidic acid; Cer ceramide; dMePE dimethylphosphatidylethanolamine; Hex1Cer monohexosylceramide; MePC methylphosphatidylcholine; PC phosphatidylcholine; PE phosphatidylethanolamine; PS phosphatidylserine; SM sphingomyelin; TG triglyceride (Color figure online)
The Supplementary Datasheet 1 provides a comprehensive tabulation of various ceramide species, including their identification and quantification details. This dataset enumerates the different types of Cer, AcCa, and BisMePA molecules, detailing their mass-to-charge ratios (m/z), retention times (rt), and the relative abundance of their respective ions across multiple samples. It is crucial for understanding the quantitative analysis of these lipid species within the context of the study.
3.3 PCA, OPLS-DA
The PCA model, characterized by an R2X(cum) value of 0.529, is substantiated by the tightly clustered distribution of both sample and quality control (QC) data points on the PCA score plot (Fig. 2A). This pattern of distribution suggests that the metabolic profiles among the samples are consistent, which is indicative of high-quality data acquisition where the analytical variance is less than the biological variance.
Presents the results of multivariate statistical analysis for dimension reduction and classification. A PCA score plot demonstrates groupings, with the x and y-axes showing the interpretation degree of the first and second principal components respectively; blue dots represent the necrozoospermia group, while pink dots denote the control group. B PLS-DA Score plot and C OPLS-DA Score plot follow a similar format, further distinguishing between groups. D PCA, E PLS-DA, and F OPLS-DA Loading plots depict the relationship between metabolic molecules and principal components. G OPLS-DA S-plot highlights lipids correlated with key biological components, with significant lipids marked in the upper right and lower left corners. H PLS-DA and I OPLS-DA Permutation test plots assess the models’ reliability, with effective models indicated by lower blue Q2 points than the rightmost blue Q2, and regression lines intersecting the y-axis below 0. PCA principal component analysis; PLS-DA partial least square discriminant analysis; OPLS-DA orthogonal partial least square discriminant analysis (Color figure online)
OPLS-DA, an advanced version of PLS-DA, provided a more refined analysis by reducing model complexity and honing in on relevant information from the X matrix. The OPLS-DA model, with improved validation parameters [R2X(cum) of 0.416, R2Y(cum) of 0.934, and Q2(cum) of 0.686], offered enhanced interpretability and predictability (Figs. 2B, D, F, G). The OPLS-DA S-plot, in particular, was instrumental in identifying lipids strongly correlated with major components in biological processes. The distinct representation of the necrozoospermia and control groups, depicted as blue and pink dots respectively, underscored the effectiveness of these methods in distinguishing metabolic variations between groups. This comprehensive approach to metabolomic analysis highlighted the significance of multivariate statistical methods in revealing intricate details of metabolic differences.
3.4 Differential analysis
There are 373 lipid compounds with statistical differences identified using preset p-value thresholds and variable importance for the projection in the OPLS-DA model (VIP). These included 51 upregulated and 322 downregulated lipid species (see Table 2, Fig. 3).
Analysis of differential lipid compounds. A The correlation heat map shows the correlation between different lipid classes, with red dots indicating positive correlation and blue dots negative correlation. The color intensity reflects the strength of the correlation, and dot size indicates the significance of the correlation (p value). B The clustering heat map displays quantitative lipid values, with color intensity indicating expression levels. It includes a lipid clustering tree and a sample clustering tree. C The lipid class bubble chart plots the log2 fold change of lipids against their classes, using dot color to differentiate lipid categories and dot size to represent the significance of the differences (p value) (Color figure online)
The data was utilized to create a cluster heat map of different lipid classes. Furthermore, the correlation between various lipid classes was analyzed by calculating the Pearson correlation coefficients among them. Lipids changing in the same direction showed positive correlation, while those changing in opposite directions displayed negative correlation.
For each comparison group, differential lipids were visualized using a lipid bubble chart plotted with P values and FC values. Different lipid classes were represented with distinct colors, with each bubble representing a single lipid. This bubble chart intuitively displayed the expression synchrony and differences among various classifications.
3.5 Correlation analysis
The correlations between discriminative lipids and seminal plasma analysis parameters were assessed using Spearman’s rank correlation coefficients. Table 3 displays the correlation coefficients, two-tailed p-values, and the statistical significance of these correlations. The results indicated that age did not show significant correlations with lipid classes or seminal plasma parameters, as all p-values were above 0.05, suggesting a limited impact of age on these parameters.
A moderate positive correlation was observed between the number of abstinence days and seminal plasma volume and pH, with p-values of 0.198 and 0.302, respectively. This suggests that an increase in abstinence days may be associated with a decrease in seminal plasma volume and a lower pH value. However, the correlation between the number of abstinence days and the percentage of progressive motility and sperm viability was not significant, with p-values of 0.197 and 0.172, respectively.
Significant negative correlations were found between seminal plasma volume and certain lipid parameters, particularly with PE(18:1e_22:4) and PE(18:0p_22:4), with correlation coefficients of − 0.581 and − 0.585, respectively, and p-values less than 0.01. This indicates that a decrease in seminal plasma volume is closely associated with reduced levels of these lipid classes. Additionally, seminal plasma volume showed significant correlations with sperm concentration and progressive motility, with p-values of 0.078 and 0.207, respectively, which may imply that a decrease in seminal plasma volume is related to a decline in sperm quality and motility.
The correlations between pH value and lipid parameters were inconsistent, with significant negative correlations for PE(18:1e_22:4) and PE(18:0p_22:4), with correlation coefficients of − 0.372 and − 0.282, respectively, and p-values less than 0.05. This suggests that a decrease in pH value may be related to reduced levels of these lipid classes. However, correlations with other lipid parameters were not significant.
Sperm concentration showed significant correlations with PE(18:1e_22:4) and PE(18:0p_22:4), with correlation coefficients of − 0.585 and − 0.58, respectively, and p-values less than 0.01, indicating that a decrease in sperm concentration may be associated with reduced levels of these lipid classes. In contrast, sperm viability did not show significant correlations with lipid parameters, as all p-values were above 0.05.
3.6 Lipid community analysis, correlation and structural feature heatmap
Lipid functional enrichment analysis was conducted using the LION lipid ontology database, which encompasses over 50,000 lipid species. This analysis was focused on four key categories: lipid function, chemical and physical properties, LIPIDMAPS classification, and subcellular localization. The lipids differentiated by the Leiden community classification were the primary focus in our network analysis (Fig. 4A).
Lipidomic analysis: functional enrichment and network features. A LION enrichment bubble plot: Horizontally shows the − log10 FDR q-value, and vertically lists enriched LION database entries. Bubble color and size represent the enrichment p-value significance and the number of lipids enriched, respectively. B Interaction map of lipid molecules: Displays top 50 differential lipids in the necrospermia group with high correlation coefficients and significant p values. C Bee swarm plot of lipid structural differences: Dots represent lipid molecules, color-coded by Leiden community classification, with lines showing significant correlations between lipids. FDR false discovery rate (Color figure online)
For the correlation network analysis, the top 50 lipids, showing significant p value differences, were selected based on criteria of a correlation coefficient greater than 0.8 and a p value less than 0.05. These criteria were also applied to the Leiden community classification, which included all differentially classified lipids (Fig. 4B).
Additionally, a structural feature analysis of the differential lipids was performed using the lipidomeR tool. Regression models were fitted to the lipid quantitation results to systematically interpret lipidomic characteristics. Heat maps were generated to illustrate the relationships between comparison groups and lipids, categorized by lipid class. This analysis, which computed the number of carbon atoms and saturation levels (number of double bonds) in lipids, was limited to those annotated with chain length and saturation information and focused on comparing structural feature differences between two groups (Fig. 4C).
3.7 Machine-learning biomarker discovery
The limitations of traditional statistical methods in biomarker discovery were addressed. These limitations included weak insights into feature interactions and poor predictive classification for new samples. Machine learning approaches were employed for feature selection and extraction. Methods such as PCA were utilized for feature extraction, while filter, wrapper, and embedded methods were used for feature selection. Notably, the wrapper method using random forests (RF) was applied to rank feature importance. Patterns of metabolite expression with functional correlations were revealed, visualized in a two-way hierarchical cluster heatmap (Fig. 5A). This approach identified important feature molecules based on their expression patterns.
Random forest feature ranking using wrapper method. A Importance feature bee plot: displays the top 20 lipid features by importance on the vertical axis, with their absolute average Shapley scores on the horizontal axis, indicating lipid significance. B Box Plot of Important Lipid Features: Compares log2 values of substance measurements in control (a) and experimental (b) groups. Illustrates distribution changes in content. C Cluster Heatmap: Shows relative lipid content levels with color coding (red for higher, blue for lower). The horizontal axis lists samples, the vertical axis lists lipids, and a clustering tree is on the left (Color figure online)
For the analysis of feature importance, SHapley Additive exPlanations (SHAP) were used. This method offers local and global interpretability by assigning Shapley values to each feature, indicating their contribution to model predictions. SHAP was found to outperform traditional methods like RF feature importance, providing better consistency and insight into the positive or negative influence of predictors on the target variable. The absolute average of Shapley values across all samples was calculated to determine the global importance of each feature.
The top 20 lipid features identified as most important included LPE(20:4), SM(d36:2), MGMG(32:1), among others (Fig. 5B, C). These features were crucial in the context of biomarker discovery, showcasing the effectiveness of machine learning in overcoming the limitations of traditional statistical methods.
3.8 Machine-learning ROC prediction analysis
Eight models combining the above-mentioned important features were compared using ROC curves, accuracy, sensitivity, and specificity as evaluation metrics. The top five lipid features by contribution ranking were selected for RF machine learning model analysis, which include LPE(20:4), SM(d36:2), MGMG(32:1), SM(t35:2), and TG(4:0_14:1_16:0). ROC curves were plotted, and lipids with an AUC > 0.8 were identified: LPE(20:4) and TG(4:0_14:1_16:0) (Table 4, Fig. 6, also see Supplementary Datasheet 2). The results of a random forest model analysis focusing on the AUC-related parameters for two lipids, Lysophosphatidylethanolamine (LPE) with the chain length 20:4 and Triglyceride (TG) with the chain lengths 4:0, 14:1, and 16:0. This analysis includes the area under the curve (AUC), sensitivity, specificity, and their 95% Confidence Intervals (CIs). The AUC for LPE(20:4) is reported as 0.81, with a sensitivity of 0.75 and specificity of 0.56, and the respective 95% CIs for sensitivity and specificity are 0.45–1 and 0.23–0.88. In the case of TG(4:0_14:1_16:0), the AUC is slightly higher at 0.82, with a sensitivity of 0.63 and a specificity of 1.0. The 95% CIs for sensitivity range from 0.29 to 0.969, while the specificity is consistently at 1.0 across its CI. They have high AUC values and a balance between sensitivity and specificity in differentiating conditions, indicating their potential to be significant biomarkers.
ROC prediction analysis using machine learning for top 5 biomarkers. A Overall machine learning ROC curve, illustrating diagnostic accuracy with lower false positive rates indicated by closer proximity to the upper-left corner. B–F Individual ROC curves for LPE(20:4), SM(d36:2), MGMG(32:1), SM(t35:2), and TG(4:0_14:1_16:0), respectively. All curves demonstrate diagnostic performance, with lower false positive rates on the horizontal axis and higher true positive rates on the vertical axis, assessing diagnostic accuracy. KNN K-nearest neighbor, RF random forest, SVM support vector machine, GNB Gaussian Navie bayes, LR logistic regression, DT decision tree, AdaBoost adaptive boosting; LPE lysophosphatidylethanolamine; ROC receiver operating characteristics; TG triglyceride
4 Discussion
Lipids are biologically organic molecules that are insoluble in water but soluble in nonpolar solvents. They can be categorized from a chemical structure and biosynthesis perspective into fatty acids (FAs), glycerolipids, glycerophospholipids, sphingolipids (SPs), sterolipids, prenolipids, saccharolipids, polyketides, and others. Lipids play a crucial role in regulating various life processes, including energy conversion, substance transport, information recognition and transmission, cell development and differentiation, and apoptosis. Moreover, abnormal lipid metabolism is closely associated with the onset and progression of various diseases such as atherosclerosis, diabetes, obesity, Alzheimer's disease, and cancer (Yoon et al., 2022).
Compared to normal sperm motility values, patients with asthenozoospermia show a significant increase in the ratio of cholesterol sulfate to lipids in seminal plasma (Lopalco et al., 2019). Alcohol abuse impacts the lipid composition of seminal plasma, primarily manifested by a significant decrease in levels of sphingomyelin (SM) and phosphatidylethanolamine (PE), along with an increased cholesterol-to-phospholipid ratio (Gomathi et al., 1993). High-performance liquid chromatography–mass spectrometry analysis of human seminal plasma identified the three most abundant lipid classes as cholesterol, SM, and phosphatidylcholine (PC), while the three most varied classes were PC, PE, and triglyceride (TG) (Qi et al., 2018). In our analysis of lipids in seminal plasma from two groups, the top three categories in terms of abundance were PE, PC, and ceramide (Cer). Our lipid species detection was more comprehensive than that of Qi et al., but the top two categories remained the same. A study on oligozoospermia patients’ seminal plasma identified 37 metabolites with decreased concentrations, including 17 PCs and four SMs, with acylcarnitine and free l-carnitine being the most distinctive metabolites (Boguenet et al., 2020).
Our study revealed a decrease in TG content in the seminal plasma of necrozoospermia patients. As a primary energy source for many tissues, the identified differential lipids TG(4:0_14:1_16:0) and TG(4:0_14:1_18:0) suggest poor sperm vitality in necrozoospermia patients, correlated with low TG content in seminal plasma. This finding is consistent with the results of Li et al. indicating a correlation between decreased sperm vitality and low TG concentration in seminal plasma (Torres-Puig et al., 2022). Kim et al. demonstrated that local metabolism of TG in the male reproductive system can affect sperm production and vitality (Kim et al., 2017). A medium-chain TG-replacement diet can improve sperm quality in patients with asthenozoospermia, with low levels of serum non-esterified fatty acids being an effective non-pharmacological treatment method (Masaki et al., 2017). In azoospermia patients, the concentration of TG(14:1_16:0_18:3) in seminal plasma is one of the indicators predicting the success rate of sperm retrieval in testicular microdissection, with a sensitivity of 61%, specificity of 83%, and an AUC of 0.75 (Gamidov et al., 2022). Our study, using random forest model analysis, identified TG(4:0_14:1_16:0) as a potential biomarker for diagnosing necrozoospermia, with a sensitivity of 63%, specificity of 100%, and an AUC of 0.82.
It is demonstrated that PE(18:1e_22:4) and PE(18:0p_22:4) are downregulated among differential lipids. Yolk freezing protection solutions containing PE can reduce damage to spermatozoa membranes and vitality caused by freezing (Pillet et al., 2012). Lipidomic studies of seminal plasma in patients with pituitary stalk interruption syndrome observed a downregulation of PE, consistent with our research findings (Guo et al., 2022). Li et al. demonstrated that PE and TG serve as biomarkers for diagnosing Kallmann syndrome (Li et al., 2021). Phospholipids on the mitochondrial membrane primarily consist of phosphatidylcholine (PC), phosphatidylethanolamine (PE), and cardiolipin (CL), accounting for approximately 40%, 30%, and 15% of the total lipid composition, respectively (Samuel & Shulman, 2018). PE exhibits excellent antioxidant properties and plays a crucial role in semen liquefaction, inflammatory reactions, and semen coagulation (Deepinder et al., 2007). Mitochondria provide energy for most cells, and when the PE content in mitochondria decreases, cellular oxygen consumption, cellular adenosine triphosphate (ATP) levels, and the ATP generation rates significantly decrease. This indicates a correlation between the occurrence of necrozoospermia and a decline in spermatozoa mitochondrial function. A study by Somashekar et al. confirmed the crucial role of PE binding protein-4 (PEBP4) in spermatozoa production, maturation, and vitality (Somashekar et al., 2017). Its presence is positively correlated with regulating spermatozoa maturation and fertilization capability, suggesting PEBP4 as a potential reproductive indicator for spermatozoa quality and fertilization capability.
From the differential analysis, we selected lipids with a p-value ranking in the top 100 and identified lipid biomarkers through machine learning analysis using random forests. The top 5 important lipid features were LPE(20:4), SM(d36:2), MGMG(32:1), SM(t35:2), and TG(4:0_14:1_16:0). Lysophosphatidylethanolamine (LPE) is a product of phosphatidylethanolamine hydrolysis by phospholipase A1/A2 and is a component of cell membranes; it is identified as a potential biomarker for necrozoospermia, with a sensitivity of 75%, specificity of 56%, and an AUC value of 0.81 in this study. While this indicates a significant association with sperm vitality, we recognize its insufficient specificity and the wide confidence interval. An UPLC-MS-based seminal plasma lipidomics study identified lysophosphatidylethanolamine (LPE) with a specific acyl chain length of 20:4, revealing an increased pattern in seminal plasma across various physiological states of sperm, including activation and the acrosome reaction (Cheng et al., 2023). The upregulation of LPE 20:4 is particularly noteworthy as it may reflect dynamic changes in sperm membrane composition and function, which are crucial for sperm motility and fertilization capacity. This underscores the role of LPE 20:4 as a potential biomarker in seminal plasma, highlighting the significance of LPE 20:4 in the complex chain of events leading to successful fertilization. Further research is needed to elucidate the biological mechanisms of LPE(20:4) in sperm function and to confirm its utility as a diagnostic marker for necrozoospermia. Another lysophosphatidylethanolamine (LPE) with a specific acyl chain length of O-16:1 also shows a significant association with male infertility (Correnti et al., 2023). Levels of LPE (O-16:1) were found to be increased in the seminal plasma of infertile men. The increase in LPE may reflect alterations in the composition and function of the sperm membrane, which are crucial for the sperm viability and fertilization potential. The prospect of utilizing LPE as a biomarker is an area that future studies will aim to investigate, encompassing laboratory validation and a detailed testing of its correlation with the physiological state of sperm.
As a major lipid component of cell membranes, SM constitutes 12.5% of the total membrane lipids in human spermatozoa (Flesch & Gadella, 2000). The hydrolysis of SM to generate ceramide (Cer) increases spermatozoa acquisition capability. It also facilitates a faster and more intense acrosome reaction, promoting spermatozoa–egg fusion and enhancing the reproductive capacity of male animals (Ahumada-Gutierrez et al., 2019). SM synthase 2, located in the testes and spermatozoa of male animals, exhibits reduced expression in spermatozoa from patients with asthenozoospermia, indicating a correlation between decreased SM production and reduced spermatozoa motility (Li et al., 2020). SM(d36:2) and SM(d18:1_18:1) are differentially expressed lipids, with their downregulation indicating a correlation between the occurrence of necrozoospermia and decreased levels of SM in seminal plasma. In patients with pituitary stalk interruption syndrome, seminal plasma lipids such as SM(d38:2), SM(d40:2), SM(d36:1), SM(d34:1), SM(d42:1), and SM(d42:3) were also downregulated, aligning with our findings and suggesting abnormal nutritional conditions in the spermatozoa survival microenvironment (Guo et al., 2022).
5 Strengths and limitations
This study employed an untargeted lipidomics approach to investigate the changes in seminal plasma lipids in necrospermia patients, providing a comprehensive understanding of the lipidomic profile in these individuals. Additionally, through the application of random forest machine learning, potential biomarkers were identified.
The current diagnostic methods for necrozoospermia predominantly involve traditional semen analysis, which assesses sperm count, motility, and morphology, alongside staining techniques such as Eosin-Nigrosin, which distinguishes live from dead sperm cells based on their ability to exclude or take up certain dyes (WHO, 2021). Despite their widespread use in clinical settings, we have noted that these methods may fail to provide adequate information in certain circumstances, particularly in cases of idiopathic necrozoospermia. Our study investigates lipidomics analysis as a promising adjunctive diagnostic tool, with the goal of improving the accuracy and reliability of diagnosis.
However, the study has its limitations as these putative biomarkers were not validated in vitro. The future research plans include conducting in vitro experiments to verify the specificity and sensitivity of these biomarkers, as well as to assess their accuracy in disease diagnosis. Another key aspect of ongoing research will involve developing a refined random forest algorithm model based on the lipidomic data of necrospermia patients. The model will undergo further external multi-centre validation to evolve into a more potent omics and Artificial Intelligence-based diagnostic tool. Such advancements would aim to predict fertility outcomes and assist clinicians in making more precise assisted reproductive strategies.
6 Conclusions
LPE(20:4) and TG(4:0_14:1_16:0), were identified as differential lipids for necrozoospermia. Seminal plasma lipidomic analysis could provide valuable biochemical information for the diagnosis of necrozoospermia, and its combination with conventional sperm analysis may improve the accuracy and reliability of the diagnosis.
Data availability
The raw data presented in this study are openly available in the OMIX database (https://ngdc.cncb.ac.cn/omix/view/OMIX006226): accession No. OMIX006226.
Abbreviations
- AD:
-
Alzheimer’s disease
- ATP:
-
Adenosine triphosphate
- BisMePA:
-
Bis-methylphosphatidic acid
- CASA:
-
Computer-assisted semen analysis
- Cer:
-
Ceramide
- CL:
-
Cardiolipin
- Co:
-
Coenzyme
- dMePE:
-
Dimethylphosphatidylethanolamine
- FDR:
-
False discovery rate
- GM:
-
Ganglioside
- Hex1Cer:
-
Monohexosylceramide
- Hex2Cer:
-
Dihexosylceramide
- Hex3Cer:
-
Trihexosylceramide
- LC–MS:
-
Liquid chromatography–mass spectrometry
- LPE:
-
Lysophosphatidylethanolamine
- MePC:
-
Methylphosphatidylcholine
- MS:
-
Mass spectrometry
- OPLS-DA:
-
Orthogonal partial least square discriminant analysis
- PC:
-
Phosphatidylcholine
- PCA:
-
Principal component analysis
- PE:
-
Phosphatidylethanolamine
- PEBP4:
-
Phosphatidylethanolamine binding protein-4
- PG:
-
Phosphatidylglycerol
- PI:
-
Phosphatidylinositol
- PLS:
-
Partial least square
- PLS-DA:
-
Partial least square discriminant analysis
- PS:
-
Phosphatidylserine
- QA:
-
Quality assurance
- QC:
-
Quality control
- ROC:
-
Receiver operating characteristics
- ROC:
-
Receiver operating characteristics
- RSD:
-
Relative standard deviation
- RSD:
-
Relative standard deviation
- SHOP:
-
SHapley Additive exPlanations
- SM:
-
Sphingomyelin
- SPH:
-
Sphingosine
- StE:
-
Stigmasterol ester
- SZMCH:
-
Shenzhen Maternity & Child Healthcare Hospital
- TG:
-
Triglyceride
- TG:
-
Triglyceride
- VIP:
-
Variable importance in projection
References
Agarwal, A., Sharma, R. K., Gupta, S., Boitrelle, F., Finelli, R., Parekh, N., Durairajanayagam, D., Saleh, R., Arafa, M., Cho, C. L., et al. (2022). Sperm vitality and necrozoospermia: Diagnosis, management, and results of a Global Survey of Clinical Practice. World Journal of Men’s Health, 40, 228–242.
Ahumada-Gutierrez, H., Peñalva, D. A., Enriz, R. D., Antollini, S. S., & Cascales, J. J. L. (2019). Mechanical properties of bilayers containing sperm sphingomyelins and ceramides with very long-chain polyunsaturated fatty acids. Chemistry and Physics of Lipids, 218, 178–186.
Boguenet, M., Bocca, C., Bouet, P. E., Serri, O., Chupin, S., Tessier, L., Blanchet, O., & El Hachem, H. (2020). Metabolomic signature of the seminal plasma in men with severe oligoasthenospermia. Andrology, 8, 1859–1866.
Boursier, A., Dumont, A., Boitrelle, F., Prasivoravong, J., Lefebvre-Khalil, V., Robin, G., & Barbotin, A. L. (2022). Necrozoospermia: The tree that hides the forest. Andrology, 10, 642–659.
Cheng, X., Xie, H., Xiong, Y., Sun, P., Xue, Y., & Li, K. (2023). Lipidomics profiles of human spermatozoa: Insights into capacitation and acrosome reaction using UPLC-MS-based approach. Frontiers in Endocrinology (lausanne), 14, 1273878.
Correnti, S., Preianò, M., Fregola, A., Gamboni, F., Stephenson, D., Savino, R., D’Alessandro, A., & Terracciano, R. (2023). Seminal plasma untargeted metabolomic and lipidomic profiling for the identification of a novel panel of biomarkers and therapeutic targets related to male infertility. Frontiers in Pharmacology, 14, 1275832.
Dalli, J., Colas, R. A., Walker, M. E., & Serhan, C. N. (2018). Lipid mediator metabolomics via LC-MS/MS profiling and analysis. Methods in Molecular Biology, 1730, 59–72.
Deepinder, F., Chowdary, H. T., & Agarwal, A. (2007). Role of metabolomic analysis of biomarkers in the management of male infertility. Expert Review of Molecular Diagnostics, 7, 351–358.
Deng, T., Li, X., & Yao, B. (2023). Metabonomic analysis of seminal plasma in necrozoospermia patients based on liquid chromatography–mass spectrometry. Translational Andrology and Urology, 12, 1101–1114.
Flesch, F. M., & Gadella, B. M. (2000). Dynamics of the mammalian sperm plasma membrane in the process of fertilization. Biochimica Et Biophysica Acta (BBA) - Reviews on Biomembranes, 1469, 197–235.
Gamidov, S. I., Shatylko, T. V., Tambiev, A. K., Tokareva, A. O., Chagovets, V. V., Bitsoev, T. B., Starodubtseva, N. L., Popova, A. Y., & Frankevich, V. E. (2022). Prognostic ability of seminal plasma lipidomic analysis in predicting the success of microTESE in men with azoospermia. Urologiia, 77, 32–37.
Gomathi, C., Balasubramanian, K., Bhanu, N. V., Srikanth, V., & Govindarajulu, P. (1993). Effect of chronic alcoholism on semen–studies on lipid profiles. International Journal of Andrology, 16, 175–181.
Guo, Y., Li, X., Wang, X., Li, H., Luo, G., Si, Y., Wu, X., & Li, Y. (2022). Seminal plasma metabolomics and lipidomics profiling to identify signatures of pituitary stalk interruption syndrome. Orphanet Journal of Rare Diseases, 17, 267.
Kawano, N., Yoshida, K., Miyado, K., & Yoshida, M. (2011). Lipid rafts: Keys to sperm maturation, fertilization, and early embryogenesis. Journal of Lipid Research, 2011, 264706.
Kim, N., Nakamura, H., Masaki, H., Kumasawa, K., Hirano, K. I., & Kimura, T. (2017). Effect of lipid metabolism on male fertility. Biochemical and Biophysical Research Communications, 485, 686–692.
Li, X., Luo, T., Li, H., & Yan, N. (2020). Sphingomyelin synthase 2 participate in the regulation of sperm motility and apoptosis. Molecules, 25, 4231.
Li, X., Wang, X., Li, H., Li, Y., & Guo, Y. (2021). Seminal plasma lipidomics profiling to identify signatures of Kallmann syndrome. Frontiers in Endocrinology (lausanne), 12, 692690.
Lopalco, P., Vitale, R., Cho, Y. S., Totaro, P., Corcelli, A., & Lobasso, S. (2019). Alteration of cholesterol sulfate/seminolipid ratio in semen lipid profile of men with oligoasthenozoospermia. Frontiers in Physiology, 10, 1344.
Masaki, H., Kim, N., Nakamura, H., Kumasawa, K., Kamata, E., Hirano, K. I., & Kimura, T. (2017). Long-chain fatty acid triglyceride (TG) metabolism disorder impairs male fertility: A study using adipose triglyceride lipase deficient mice. Molecular Human Reproduction, 23, 452–460.
Máté, G., Balló, A., Márk, L., Czétány, P., Szántó, Á., & Török, A. (2022). Magnetic-activated cell sorting as a method to improve necrozoospermia-related asthenozoospermic samples. Journal of Clinical Medicine, 11(10), 2914.
Molenaar, M. R., Jeucken, A., Wassenaar, T. A., van de Lest, C. H. A., Brouwers, J. F., & Helms, J. B. (2019). LION/web: A web-based ontology enrichment tool for lipidomic data analysis. Gigascience. https://doi.org/10.1093/gigascience/giz061
Pillet, E., Labbe, C., Batellier, F., Duchamp, G., Beaumal, V., Anton, M., Desherces, S., Schmitt, E., & Magistrini, M. (2012). Liposomes as an alternative to egg yolk in stallion freezing extender. Theriogenology, 77, 268–279.
Qi, C., Baojun, J., Jie, C., Jingjing, W., Shuqiang, C., & Xiaohong, W. (2018). Identification of human seminal plasma lipid components by high performance liquid chromatography–tandem mass spectrometry multiple reaction monitoring. Journal of Lanzhou University: Medical Edition, 44, 8.
Samuel, V. T., & Shulman, G. I. (2018). Nonalcoholic fatty liver disease as a nexus of metabolic and hepatic diseases. Cell Metabolism, 27, 22–41.
Somashekar, L., Selvaraju, S., Parthipan, S., Patil, S. K., Binsila, B. K., Venkataswamy, M. M., Karthik Bhat, S., & Ravindra, J. P. (2017). Comparative sperm protein profiling in bulls differing in fertility and identification of phosphatidylethanolamine-binding protein 4, a potential fertility marker. Andrology, 5, 1032–1051.
Torres-Puig, S., García, V., Stærk, K., Andersen, T. E., Møller-Jensen, J., Olsen, J. E., & Herrero-Fresno, A. (2022). “Omics” technologies—What have they told us about uropathogenic Escherichia coli fitness and virulence during urinary tract infection? Frontiers in Cellular and Infection Microbiology, 12, 824039.
World Health Organization. (2021). WHO laboratory manual for the examination and processing of human semen (6th ed.). Geneva: World Health Organization.
Yoon, J. H., Seo, Y., Jo, Y. S., Lee, S., Cho, E., Cazenave-Gassiot, A., Shin, Y. S., Moon, M. H., An, H. J., Wenk, M. R., & Suh, P. G. (2022). Brain lipidomics: From functional landscape to clinical significance. Science Advances, 8, 9317.
Acknowledgements
The authors express their gratitude to all participants for their cooperation in contributing to the study. Additionally, heartfelt appreciation is extended to the Andrology Lab in Shenzhen Maternity and Child Healthcare Hospital for their invaluable technical support throughout the course of this research.
Funding
This research was funded by the Shenzhen Science and Technology Innovation Committee, Grant Number KJYY20180703173402020 to Prof. Jilong Yao and Shenzhen Key Medical Discipline Construction Fund (Grant No. SZXK031) to Reproductive medicine centre of SZMCH. Further financial backing came from the Shenzhen-Hong Kong-Macau Science and Technology Program (Type C), under Grant Number SGDX2020110309500101. The funders had no role in study design, data collection and analysis, decision to publish, or preparation of the manuscript.
Author information
Authors and Affiliations
Contributions
Conceptualization and methodology: TQ.D., WX.W., B.Y, JL.Y. and ZH.F.; software, validation and formal analysis: TQ.D., WX.W., YH.Z., JB.P., and KX.C.; investigation: TQ.D., WX.W., YH.Z., YL.X. and XM.L.; resources: JL.Y., TQ.D., XM.L. and WX.W.; data curation: JB.P., ZH.F. and KX.C; writing—original draft preparation: TQ.D., WX.W., ZH.F. and YL.X.; writing—review and editing: TQ.D, WX.W., JL.Y, XM.L., B.Y, YH.Z., JB.P., and KX.C.; visualization: TQ.D. and WX.W.; supervision: JL.Y. and XM.L.; funding acquisition: JL.Y. and XM.L. All authors have read and agreed to the published version of the manuscript.
Corresponding authors
Ethics declarations
Conflict of interest
The authors declare no conflict of interest.
Ethics approval and consent to participate
The study was conducted in accordance with the Declaration of Helsinki and approved by the Ethics Committee of SZMCH (No. SFYLS [2021]038). Informed consent was obtained from all patients involved in the study.
Consent for publication
Since it is the scientific research of clinical samples without individual patient data or images, patient consent for publication is not applicable.
Additional information
Publisher's Note
Springer Nature remains neutral with regard to jurisdictional claims in published maps and institutional affiliations.
Supplementary Information
Below is the link to the electronic supplementary material.
Supplementary file1 (XLSX 2511 KB)
Supplementary Datasheet 1: List of Sample Identification and Quantification
Supplementary file2 (XLSX 14 KB)
Supplementary Datasheet 2: Quantitative identification results of the top five lipids ranked by Random Forest in all samples
Supplementary file3 (DOCX 21 KB)
Supplementary Materials and Methods
Rights and permissions
Open Access This article is licensed under a Creative Commons Attribution 4.0 International License, which permits use, sharing, adaptation, distribution and reproduction in any medium or format, as long as you give appropriate credit to the original author(s) and the source, provide a link to the Creative Commons licence, and indicate if changes were made. The images or other third party material in this article are included in the article's Creative Commons licence, unless indicated otherwise in a credit line to the material. If material is not included in the article's Creative Commons licence and your intended use is not permitted by statutory regulation or exceeds the permitted use, you will need to obtain permission directly from the copyright holder. To view a copy of this licence, visit http://creativecommons.org/licenses/by/4.0/.
About this article
Cite this article
Deng, T., Wang, W., Fu, Z. et al. Lipidomics random forest algorithm of seminal plasma is a promising method for enhancing the diagnosis of necrozoospermia. Metabolomics 20, 57 (2024). https://doi.org/10.1007/s11306-024-02118-x
Received:
Accepted:
Published:
DOI: https://doi.org/10.1007/s11306-024-02118-x