Abstract
There is a need for effective methods to teach critical thinking (CT). One instructional method that seems promising is comparing correct and erroneous worked examples (i.e., contrasting examples). The aim of the present study, therefore, was to investigate the effect of contrasting examples on learning and transfer of CT-skills, focusing on avoiding biased reasoning. Students (N = 170) received instructions on CT and avoiding biases in reasoning tasks, followed by: (1) contrasting examples, (2) correct examples, (3) erroneous examples, or (4) practice problems. Performance was measured on a pretest, immediate posttest, 3-week delayed posttest, and 9-month delayed posttest. Our results revealed that participants’ reasoning task performance improved from pretest to immediate posttest, and even further after a delay (i.e., they learned to avoid biased reasoning). Surprisingly, there were no differences in learning gains or transfer performance between the four conditions. Our findings raise questions about the preconditions of contrasting examples effects. Moreover, how transfer of CT-skills can be fostered remains an important issue for future research.
Similar content being viewed by others
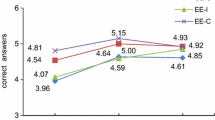
Introduction
Every day, we reason and make many decisions based on previous experiences and existing knowledge. To do so we often rely on a number of heuristics (i.e., mental shortcuts) that ease reasoning processes (Tversky & Kahneman, 1974). Usually, these decisions are inconsequential but sometimes they can lead to biases (i.e., deviating from ideal normative standards derived from logic and probability theory) with severe consequences. To illustrate, a forensic expert who misjudges fingerprint evidence because it verifies his or her preexisting beliefs concerning the likelihood of the guilt of a defendant, displays the so-called confirmation bias, which can result in a misidentification and a wrongful conviction (e.g., the Madrid bomber case; Kassin et al., 2013). Biases occur when people rely on heuristic reasoning (i.e., Type 1 processing) when that is not appropriate, do not recognize the need for analytical or reflective reasoning (i.e., Type 2 processing), are not willing to switch to Type 2 processing or unable to sustain it, or miss the relevant mindware to come up with a better response (e.g., Evans, 2003; Stanovich, 2011). Our primary tool for reasoning and making better decisions, and thus to avoid biases in reasoning and decision making, is critical thinking (CT), which is generally characterized as “purposeful, self-regulatory judgment that results in interpretation, analysis, evaluation, and inference, as well as explanation of the evidential, conceptual, methodological, criteriological, or contextual considerations on which that judgment is based” (Facione, 1990, p. 2).
Because CT is essential for successful functioning in one’s personal, educational, and professional life, fostering students’ CT has become a central aim of higher education (Davies, 2013; Halpern, 2014; Van Gelder, 2005). However, several large-scale longitudinal studies were quite pessimistic that this laudable aim would be realized merely by following a higher education degree program. These studies revealed that CT-skills of many higher education graduates are insufficiently developed (e.g., Arum & Roksa, 2011; Flores et al., 2012; Pascarella et al., 2011; although a more recent meta-analytic study reached the more positive conclusion that students’ do improve their CT-skills over college years: Huber & Kuncel, 2016). Hence, there is a growing body of literature on how to teach CT (e.g., Abrami et al., 2008, 2014; Van Peppen et al., 2018, 2021; Angeli & Valanides, 2009; Niu et al., 2013; Tiruneh et al., 2014, 2016).
However, there are different views on the best way to teach CT; the most well-known debate being whether CT should be taught in a general or content-specific manner (Abrami et al., 2014; Davies, 2013; Ennis, 1989; Moore, 2004). This debate has faded away during the last years, since most researchers nowadays commonly agree that CT can be seen in terms of both general skills (e.g., sound argumentation, evaluating statistical information, and evaluating the credibility of sources) and specific skills or knowledge used in the context of disciplines (e.g., diagnostic reasoning). Indeed, it has been shown that the most effective teaching methods combine generic instruction on CT with the opportunity to integrate the general principles that were taught with domain-specific subject matter. It is well established, for instance, that explicit teaching of CT combined with practice improves learning of CT-skills required for unbiased reasoning (e.g., Abrami et al., 2008; Heijltjes et al., 2014b). However, while some effective teaching methods have been identified, it is as yet unclear under which conditions transfer of CT-skills across tasks or domains can be promoted, that is, the ability to apply acquired knowledge and skills to some new context of related materials (e.g., Barnett & Ceci, 2002).
Transfer has been described as existing on a continuum from near to far, with lower degrees of similarity between the initial and transfer situation along the way (Salomon & Perkins, 1989). Transferring knowledge or skills to a very similar situation, for instance problems in an exam of the same kind as practiced during the lessons, refers to ‘near’ transfer. By contrast, transferring between situations that share similar structural features but, on appearance, seem remote and alien to one another is considered ‘far’ transfer.
Previous research has shown that CT-skills required for unbiased reasoning consistently failed to transfer to novel problem types, i.e., far transfer, even when using instructional methods that proved effective for fostering transfer in various other domains (Van Peppen et al., 2018, 2021; Heijltjes et al., 2014a, 2014b, 2015, and this also applies to CT-skills more generally, see for example Halpern, 2014; Ritchhart & Perkins, 2005; Tiruneh et al., 2014, 2016). This lack of transfer of CT-skills is worrisome because it would be unfeasible to train students on each and every type of reasoning bias they will ever encounter. CT-skills acquired in higher education should transfer to other domains and on-the-job and, therefore, it is crucial to acquire more knowledge on how transfer of these skills can be fostered (and this also applies to CT-skills more generally, see for example, Halpern, 2014; Beaulac & Kenyon, 2014; Lai, 2011; Ritchhart & Perkins, 2005). One instructional method that seems promising is comparing correct and erroneous worked examples (i.e., contrasting examples; e.g., Durkin & Rittle-Johnson, 2012).
Benefits of studying examples
Over the last decades, a large body of research has investigated learning from studying worked examples as opposed to unsupported problem solving. Worked examples consist of a problem statement and an entirely and correctly worked-out solution procedure (in this paper referred to as correct examples; Renkl, 2014; Renkl et al., 2009; Sweller et al., 1998; Van Gog et al., 2019). Typically, studying correct examples is more beneficial for learning than problem-solving practice, especially in initial skill acquisition (for reviews, see Atkinson et al., 2003; Renkl, 2014; Sweller et al., 2011; Van Gog et al., 2019). Although this worked example effect has been mainly studied in domains such as mathematics and physics, it has also been demonstrated in learning argumentation skills (Schworm & Renkl, 2007), learning to reason about legal cases (Nievelstein et al., 2013) and medical cases (Ibiapina et al., 2014), and novices’ learning to avoid biased reasoning (Van Peppen et al., 2021).
The worked example effect can be explained by cognitive load imposed on working memory (Paas et al., 2003a; Sweller, 1988). Cognitive Load Theory (CLT) suggests that—given the limited capacity and duration of our working memory—learning materials should be designed so as to decrease unnecessary cognitive load related to the presentation of the materials (i.e., extraneous cognitive load). Instead, learners’ attention should be devoted towards processes that are directly relevant for learning (i.e., germane cognitive load). When solving practice problems, novices often use general and weak problem-solving strategies that impose high extraneous load. During learning from worked examples, however, the high level of instructional guidance provides learners with the opportunity to focus directly on the problem-solving principles and their application. Accordingly, learners can use the freed up cognitive capacity to engage in generative processing (Wittrock, 2010). Generative processing involves actively constructing meaning from to-be-learned information, by mentally organizing it into coherent knowledge structures and integrating these principles with one’s prior knowledge (i.e., Grabowski, 1996; Osborne & Wittrock, 1983; Wittrock, 1974, 1990, 1992, 2010). These knowledge structures in turn can aid future problem solving (Kalyuga, 2011; Renkl, 2014; Van Gog et al., 2019).
A recent study showed that the worked example effect also applies to novices’ learning to avoid biased reasoning (Van Peppen et al., 2021Footnote 1): participants’ performance on isomorphic tasks on a final test improved after studying correct examples, but not after solving practice problems. However, studying correct examples was not sufficient to establish transfer to novel tasks that shared similar features with the isomorphic tasks, but on which participants had not acquired any knowledge during instruction/practice. The latter finding might be explained by the fact that students sometimes process worked examples superficially and do not spontaneously use the freed up cognitive capacity to engage in generative processing needed for successful transfer (Renkl & Atkinson, 2010). Another possibility is that these examples did not sufficiently encourage learners to make abstractions of the underlying principles and explore possible connections between problems (e.g., Perkins & Salomon, 1992). It seems that to fully take advantage of worked examples in learning unbiased reasoning, students should be encouraged to be actively involved in the learning process and facilitated to focus on the underlying principles (e.g., Van Gog et al., 2004).
The potential of erroneous examples
While most of the worked-example research focuses on correct examples, recent research suggests that students learn at a deeper level and may come to understand the principles behind solution steps better when (also) provided with erroneous examples (e.g., Adams et al., 2014; Barbieri & Booth, 2016; Booth et al., 2013; Durkin & Rittle-Johnson, 2012; McLaren et al., 2015). In studies involving erroneous examples, which are often preceded by correct examples (e.g., Booth et al., 2015), students are usually prompted to locate the incorrect solution step and to explain why this step is incorrect or to correct it. This induces generative processing, such as comparison with internally represented correct examples and (self-)explaining (e.g., Chi et al., 1994; McLaren et al., 2015; Renkl, 1999). Students are encouraged to go beyond noticing surface characteristics and to think deeply about how erroneous steps differ from correct ones and why a solution step is incorrect (Durkin & Rittle-Johnson, 2012). This might help them to correctly update schemas of correct concepts and strategies and, moreover, to create schemas for erroneous strategies (Durkin & Rittle-Johnson, 2012; Große & Renkl, 2007; Siegler, 2002; Van den Broek & Kendeou, 2008; VanLehn, 1999), reducing the probability of recurring erroneous solutions in the future (Siegler, 2002).
However, erroneous examples are typically presented separately from correct examples, requiring learners to use mental resources to recall the gist of the no longer visible correct solutions (e.g., Große & Renkl, 2007; Stark et al., 2011). Splitting attention across time increases the likelihood that mental resources will be expended on activities extraneous to learning, which subsequently may hamper learning (i.e., temporal contiguity effect: e.g., Ginns, 2006). One could, therefore, argue that the use of erroneous examples could be optimized by providing them side by side with correct examples (e.g., Renkl & Eitel, 2019). This would allow learners to focus on activities directly relevant for learning, such as structural alignment and detection of meaningful commonalities and differences between the examples (e.g., Durkin & Rittle-Johnson, 2012; Roelle & Berthold, 2015). Indeed, studies on comparing correct and erroneous examples revealed positive effects in math learning (Durkin & Rittle-Johnson, 2012; Kawasaki, 2010; Loibl & Leuders, 2018, 2019; Siegler, 2002).
The present study
We already indicated that it is still an important open question, which instructional strategy can be used to enhance transfer of CT skills. To reiterate, previous research demonstrated that practice consisting of worked example study was more effective for novices’ learning than practice problem solving, but it was not sufficient to establish transfer. Recent research has demonstrated the potential of erroneous examples, which are often preceded by correct examples. Comparing correct and erroneous examples (from here on referred to as contrasting examples) when presenting them side-by-side, seems to hold a considerable promise with respect to promoting generative processing and transfer. Hence, the purpose of the present study was to investigate whether contrasting examples of fictitious students’ solutions on ‘heuristics and biases tasks’ (a specific sub-category of CT skills: e.g., Tversky & Kahneman, 1974) would be more effective to foster learning and transfer than studying correct examples only, studying erroneous examples only, or solving practice problems. Performance was measured on a pretest, immediate posttest, 3-week delayed posttest, and 9-month delayed posttest (for half of the participants due to practical reasons), to examine effects on learning and transfer.
Based on the literature presented above, we hypothesized that studying correct examples would impose less cognitive load (i.e., lower investment of mental effort during learning) than solving practice problems (i.e., worked example effect: e.g., Van Peppen et al., 2021; Renkl, 2014; Hypothesis 1). Whether there would be differences in invested mental effort between contrasting examples, studying erroneous examples, and solving practice problems, however, is an open question. That is, it is possible that these instructional formats impose a similar level of cognitive load, but originating from different processes: while practice problem solving may impose extraneous load that does not contribute to learning, generative processing of contrasting or erroneous examples may impose germane load that is effective for learning (Sweller et al., 2011). As such, it is important to consider invested mental effort (i.e., experienced cognitive load) in combination with learning outcomes. Secondly, we hypothesized that students in all conditions would benefit from the CT-instructions combined with the practice activities, as evidenced by pretest to immediate posttest gains in performance on instructed and practiced items (i.e., learning: Hypothesis 2). Furthermore, based on cognitive load theory, we hypothesized that studying correct examples would be more beneficial for learning than solving practice problems (i.e., worked example effect: e.g., Van Peppen et al., 2021; Renkl, 2014). Based on the aforementioned literature, we expected that studying erroneous examples would promote generative processing more than studying correct examples. Whether that generative processing would actually enhance learning, however, is an open question. This can only be expected to be the case if learners can actually remember and apply the previously studied information on the correct solution, which arguably involves higher cognitive load (i.e., temporal contiguity effect) than studying correct examples or contrasting examples. As contrasting can help learners to focus on key information and thereby induces generative processes directly relevant for learning (e.g., Durkin & Rittle-Johnson, 2012), we expected that contrasting examples would be most effective. Thus, we predict the following pattern of results regarding performance gains on learning items (Hypothesis 3): contrasting examples > correct examples > practice problems. As mentioned above, it is unclear how the erroneous examples condition would compare to the other conditions.
Furthermore, we expected that generative processing would promote transfer. Despite findings of previous studies in other domains (e.g., Paas, 1992), we found no evidence in a previous study that studying correct examples or solving practice problems would lead to a difference in transfer performance (Van Peppen et al., 2021). Therefore, we predict the following pattern of results regarding performance on non-practiced items of the immediate posttest (i.e., transfer, Hypothesis 4): contrasting examples > correct examples ≥ practice problems. Again, it is unclear how the erroneous examples condition would compare to the other conditions.
We expected these effects (Hypotheses 3 and 4) to persist on the delayed posttests. As effects of generative processing (relative to non-generative learning strategies) sometimes increase as time goes by (Dunlosky et al., 2013), they may be even greater after a delay. For a schematic overview of the hypotheses, see Table 1.
Method
We created an Open Science Framework (OSF) page for this project, where all materials, the dataset, and all script files of the experiment are provided (osf.io/8zve4/).
Participants and design
Participants were 182 first-year ‘Public Administration’ and ‘Safety and Security Management’ students of a Dutch university of applied sciences (i.e., higher professional education), both part of the Academy for Security and Governance. These students were approximately 20 years old (M = 19.53, SD = 1.91) and most of them were male (120 male, 62 female). Before they were involved in these study programs, they completed secondary education (senior general secondary education: n = 122, pre-university: n = 7) or went to college (secondary vocational education: n = 28, higher professional education: n = 24, university education: n = 1).
Of the 182 students (i.e., total number of students in these cohorts), 173 students (95%) completed the first experimental session (see Fig. 1 for an overview) and 158 students (87%) completed both the first and second experimental session. Additionally, 83 of these students (46%) of the Safety and Security Management program completed the 9-month delayed posttest during the first mandatory CT-lesson of their second study year (we had no access to another CT-lesson of the Public Administration program). The number of absentees during a lesson (about 15 in total) is quite common for mandatory lessons in these programs and often due to illness or personal circumstances. Students who were absent during the first experimental session and returned to the second experimental session could not participate in the study because they had missed the intervention phase.
We defined a priori that participants would be excluded in case of excessively fast reading speed. Considering that even fast readers can read no more than 350 words per minute (e.g., Trauzettel-Klosinski & Dietz, 2012), and the text of our instructions additionally required understanding, we assumed that participants who spent < 0.17 s per word (i.e., 60 s/350 words) did not read the instructions seriously. These participants were excluded from the analyses. Due to drop-outs, we decided to split the analyses to include as many participants as possible. We had a final sample of 170 students (Mage = 19.54, SD = 1.93; 57 female) for the pretest to immediate posttest analyses, a subsample of 155 students for the immediate to 3-week delayed posttest analyses (Mage = 19.46, SD = 1.91; 54 female), and a subsample of 82 students (46%) for the 3-week delayed to 9-month delayed posttest (Mage = 19.27, SD = 1.79; 25 female). We calculated a power function of our analyses using the G*Power software (Faul et al., 2009) based on these sample sizes. The power for the crucial Practice Type × Test Moment interaction—under a fixed alpha level of 0.05 and with a correlation between measures of 0.3 (e.g., Van Peppen et al., 2018)—for detecting a small (ηp2 = .01), medium (ηp2 = .06), and large effect (ηp2 = .14) respectively, is estimated at .42, > .99, and 1.00 for the pretest to immediate posttest analyses; .39, > .99, and 1.00 for the immediate to 3-week delayed posttest analyses; and .21, .90, and > .99 for the 3-week to 9-month delayed posttest. Thus, the power of our study should be sufficient to pick up medium-sized interaction effects.
Students participated in a pretest-intervention–posttest design (see Fig. 1). After completing the pretest on learning items (i.e., instructed and practiced during the practice phase), all participants received succinct CT instructions and two correct worked examples. Thereafter, they were randomly assigned to one of four conditions that differed in practice activities during the practice phase: they either (1) compared correct and erroneous examples (‘contrasting examples’, n = 41; n = 35; n = 20); (2) studied correct examples (i.e., step-by-step solutions to unbiased reasoning) and explained why these were right (‘correct examples’, n = 43; n = 40; n = 21); (3) studied erroneous examples (i.e., step-by-step incorrect solutions including biased reasoning) and explained why these were wrong (‘erroneous examples’, n = 43; n = 40; n = 18); or (4) solved practice problems and justified their answers (‘practice problems’, n = 43; n = 40; n = 23). A detailed explanation of the practice activities can be found in the CT-practice subsection below. Immediately after the practice phase and after a 3-week delay, participants completed a posttest on learning items (i.e., instructed and practiced during the practice phase) and transfer items (i.e., not instructed and practiced during the practice phase). Additionally, some students took a posttest after a 9-month delay. Further CT-instructions were given (in three lessons of approx. 90 min) in-between the second session of the experiment and the 9-month follow up. In these lessons, for example, the origins of the concept of CT, inductive and deductive reasoning, and the Toulmin model of argument were discussed. Thus, these data were exploratively analyzed and need to be interpreted with caution.
Materials
In the following paragraphs, the used learning materials, instruments and associated measures, and characteristics of the experimental conditions are described.
CT-skills tests
The CT-skills tests consisted of classic heuristics and biases tasks that reflected important aspects of CT. In all tasks, belief bias played a role, that is, when the conclusion aligns with prior beliefs or real-world knowledge but is invalid or vice versa (Evans et al., 1983; Markovits & Nantel, 1989; Newstead et al., 1992). These tasks require that one recognizes the need for analytical and reflective reasoning (i.e. based on knowledge and rules of logical reasoning and statistical reasoning) and switches to this type of reasoning. This is only possible when heuristic responses are successfully inhibited.
The pretest consisted of six classic heuristics and biases items, across two categories (see Online Appendix A for an example of each category): syllogistic reasoning (i.e., logical reasoning) and conjunction (i.e., statistical reasoning) items. Three syllogistic reasoning items measured students’ tendency to be influenced by the believability of a conclusion that is inferred from two premises when evaluating the logical validity of that conclusion (adapted from Evans, 2002). For instance, the conclusion that cigarettes are healthy is logically valid given the premises that all things you can smoke are healthy and that you can smoke cigarettes. Most people, however, indicate that the conclusion is invalid because it does not align with their prior beliefs or real-world knowledge (i.e., belief bias, Evans et al., 1983). Three conjunction items examined to what extent the conjunction rule (P(A&B) ≤ P(B))—which states that the probability of multiple specific events both occurring must be lower than the probability of one of these events occurring alone—is neglected (Tversky & Kahneman, 1983). To illustrate, people have the tendency to judge two things with a causal or correlational link, for example advanced age and occurrence of heart attacks, as more probable than one of these on its own.
The posttests consisted of parallel versions (i.e., structurally equivalent but different surface features) of the six pretest items which were instructed and practiced and, thus, served to assess differences in learning outcomes. Additionally, the posttests contained six items across two non-practiced categories that served to assess differences in transfer performance (see Online Appendix A for an example of each category). Three Wason selection items measured students’ tendency to disprove a hypothesis by verifying rules rather than falsifying them (i.e., confirmation bias, adapted from Stanovich, 2011). Three base-rate items examined students’ tendency to incorrectly judge the likelihood of individual-case evidence (e.g., from personal experience, a single case, or prior beliefs) by not considering all relevant statistical information (i.e., base-rate neglect, adapted from Fong et al., 1986; Stanovich & West, 2000; Stanovich et al., 2016; Tversky & Kahneman, 1974). These transfer items shared similar features with the learning categories, namely, one category requiring knowledge and rules of logic (i.e., Wason selection tasks can be solved by applying syllogism rules) and one category requiring knowledge and rules of statistics (i.e., base-rate tasks can be solved by appropriate probability and data interpretation).
The cover stories of all test items were adapted to the domain of participants’ study program (i.e., Public Administration and Safety and Security Management). A multiple-choice (MC) format with different numbers of alternatives per item was used, with only one correct alternative for each item.
CT-instructions
All participants received a 12 min video-based instruction that started with emphasizing the importance of CT in general, describing the features of CT, and explaining which skills and attitudes are needed to think critically. Thereafter, explicit instructions on how to avoid biases in syllogistic reasoning and conjunction fallacies followed, consisting of two worked examples that showed the correct line of reasoning. The purpose of these explicit instructions was to provide students with knowledge on CT and to allow them to mentally correct initially incorrect responses on the items seen in the pretest.
CT-practice
Participants performed practice activities on the task categories that they were given instructions on (i.e., syllogistic reasoning and conjunction tasks). The CT-practice consisted of four practice tasks, two of each of the task categories. Each practice task was again adapted to the study domain and started with the problem statement (see Online Appendix B for an example of a practice task of each condition). Participants in the correct examples condition were provided with a fictitious student’s correct solution and explanation to the problem, including auxiliary representations, and were prompted to explain why the solution steps were correct. Participants in the erroneous examples condition received a fictitious student’s erroneous solution to the problem, again including auxiliary representations. They were prompted to indicate the erroneous solution step and to provide the correct solution themselves. In the contrasting examples, participants were provided fictitious students’ correct and erroneous solutions to the problem and were prompted to compare the two solutions and to indicate the erroneous solution and the erroneous solution step. Participants in the practice problems condition had to solve the problems themselves, that is, they were instructed to choose the best answer option and were asked to explain how the answer was obtained. Participants in all conditions were asked to read the practice tasks thoroughly. To minimize differences in time investment (i.e., the contrasting examples consisted of considerably more text), we have added self-explanation prompts in the correct examples, erroneous examples, and practice problem conditions.
Mental effort
After each test item and practice-task, participants were asked to report how much effort they invested in completing that task or item on a 9-point subjective rating scale ranging from (1) very, very low effort to (9) very, very high effort (Paas, 1992). This widely used scale in educational research (for overviews, see Paas et al., 2003b; Van Gog & Paas, 2008), is assumed to reflect the cognitive capacity actually allocated to accommodate the demands imposed by the task or item (Paas et al., 2003a).
Procedure
The study was run during the first two lessons of a mandatory first-year CT-course in two, very similar, Security and Governance study programs. Participants were not given CT-instructions in between these lessons. They completed the study in a computer classroom at the participants’ university with an entire class of students, their teacher, and the experiment leader (first author) present. When entering the classroom, participants were instructed to sit down at one of the desks and read an A4-paper containing some general instructions and a link to the computer-based environment (Qualtrics platform). The first experimental session (ca. 90 min) began with obtaining written consent from all participants. Then, participants filled out a demographic questionnaire and completed the pretest. Next, participants entered the practice phase in which they first viewed the video-based CT-instructions and then were assigned to one of the four practice conditions. Immediately after the practice phase, participants completed the immediate posttest. Approximately 3 weeks later, participants took the delayed posttest (ca. 20 min) in their computer classrooms. Additionally, students of the Safety and Security Management program took the 9-month delayed posttest during the first mandatory CT-lesson of their second study year,Footnote 2 which was exactly the same as the 3-week delayed posttest. During all experimental sessions, participants could work at their own pace and were allowed to use scrap paper. Time-on-task was logged during all phase and participants had to indicate after each test item and practice-task how much effort they invested. Participants had to wait (in silence) until the last participants had finished before they were allowed to leave the classroom.
Data analysis
All test items were MC-only questions, except for one learning item and one transfer items with only two alternatives (conjunction item and base-rate item) that were MC-plus-motivation questions to prevent participants from guessing. Items were scored for accuracy, that is, unbiased reasoning; 1 point for each correct alternative on the MC-only questions or a maximum of 1 point (increasing in steps of 0.5) for the correct explanation for the MC-plus-motivation question using a coding scheme that can be found on our OSF-page. Because two transfer items (i.e., one Wason selection item and one base-rate item) appeared to substantially reduce the reliability of the transfer performance measure, presumably as a result of low variance due to floor effects, we decided to omit these items from our analyses. As a result, participants could attain a maximum total score of 6 on the learning items and a maximum score of 4 on the transfer items. For comparability, learning and transfer outcomes were computed as percentage correct scores instead of total scores. Participants’ explanations on the open questions of the tests were coded by one rater and another rater (the first author) coded 25% of the explanations of the immediate posttest. Intra-class correlation coefficients were 0.990 for the learning test items and 0.957 for the transfer test items. After the discrepancies were resolved by discussion, the primary rater’s codes were used in the analyses.
Cronbach’s alpha on invested mental effort ratings during studying correct examples, studying erroneous examples, contrasting examples, and solving practice problems, respectively, was .87, .76, .77, and .65. Cronbach’s alpha on the learning items was .21, .42, .58, and .31 on the pretest, immediate posttest, 3-week delayed posttest, and 9-month delayed posttest, respectively. The low reliability on the pretest might be explained by the fact that a lack of prior knowledge requires guessing of answers. As such, inter-item correlations are low, resulting in a low Cronbach’s alpha. Cronbach’s alpha on the transfer items was .31, .12, and .29 on the immediate, 3-week delayed, and 9-month delayed posttest, respectively. Cronbach’s alpha on the mental effort items belonging to the learning items was .73, .79, .81, and .76 on the pretest, immediate posttest, 3-week delayed posttest, and 9-month delayed posttest, respectively. Cronbach’s alpha on the mental effort items belonging to the transfer items was .71, .75, and .64 on the immediate posttest, 3-week delayed posttest, and 9-month delayed posttest, respectively. However, caution is required in interpreting the above values because sample sizes as in studies like this do not seem to produce sufficiently precise alpha coefficients (e.g. Charter, 2003). Cronbach’s alpha is a statistic and therefore subject to sample fluctuations. Hence, one should be careful with drawing firm conclusions about the precision of Cronbach’s alpha in the population (the parameter) based on small sample sizes (i.e., in reliability literature, samples of 300–400 are considered small, see for instance Charter, 2003; Nunally & Bernstein, 1994; Segall, 1994).
There was no significant difference on pretest performance between participants who stayed in the study and those who dropped out after the first session, t(172) = .38, p = .706, and those who dropped out after the second session, t(172) = − 1.46, p = .146. Furthermore, there was no significant difference in educational background between participants who stayed in the study and those who dropped out after the first session, r(172) = .13, p = .087, and those who dropped out after the second session, r(172) = − .01, p = .860. Finally, there was no significant difference in age between participants who stayed in the study and those who dropped out after the first session, t(172) = − 1.51, p = .134, but there was a difference between participants who stayed in the study and those who dropped out after the second session, t(172) = − 2.02, p = .045. However, age did not correlate significantly with learning performance (minimum p = .553) and was therefore not a confounding variable.
Additionally, participants’ performance during the practice phase was scored for accuracy, that is, unbiased reasoning. In each condition, participants could attain a maximum score of 2 points (increasing in steps of 0.5) for the correct answer on each problem (either MC-only answers or MC-plus-explanation answers), resulting in a maximum total score of 8. The explanations given during practice were coded for explicit relations to the principles that were communicated in the instructions (i.e., principle-based explanations; Renkl, 2014). For instance, participants earned the full 2 points if they explained in a conjunction task that the first statement is part of the second statement and that the first statement therefore can never be more likely than the two statements combined. Participants’ explanations were coded by the first author and another rater independently coded 25% of the explanations. Intra-class correlation coefficients were 0.941, 0.946, and 0.977 for performance in the correct examples, erroneous examples, and practice problems conditions respectively (contrasting examples consisted of MC-only questions). After a discussion between the raters about the discrepancies, the primary rater’s codes were updated and used in the exploratory analyses.
For all analyses in this paper, a p-value of .05 was used a threshold for statistical significance. Partial eta-squared (ηp2) is reported as an effect size for all ANOVAs (see Table 3) with ηp2 = .01, ηp2 = .06, and ηp2 = .14 denoting small, medium, and large effects, respectively (Cohen, 1988). Cramer’s V is reported as an effect size for chi-square tests with (having 2 degrees of freedom) V = .07, V = .21, and V = .35 denoting small, medium, and large effects, respectively.
Results
Preliminary analyses
Check on condition equivalence
Before running any of the main analyses, we checked our conditions on equivalence. Preliminary analyses confirmed that there were no a-priori differences between the conditions in educational background, χ2(15) = 15.57, p = .411, V = .18; gender, χ2(3) = 1.21, p = .750, V = .08; performance on the pretest, F(3, 165) = 0.42, p = .739, ηp2 = .01; time spent on the pretest, F(3, 165) = 0.16, p = .926, η2 < .01; and mental effort invested on the pretest, F(3, 165) = 0.80, p = .498, η2 = .01. Further, we estimated two multiple regression models (learning and transfer) with practice type and performance on the pretest as explanatory variables, including the interaction between practice type and performance on the pretest. There was no evidence of an interaction effect (learning: R2 = .07, F(1, 166) = .296, p = .587; transfer: R2 = .07, F(1, 166) = .260, p = .611) and we can, therefore, conclude that the relationship between practice type and performance on the posttest does not depend on performance on the pretest.
Check on time-on-task
The Levene’s test for equality of variances was significant, F(3, 166) = 9.57, p < .001. Therefore, a Brown–Forsythe one-way ANOVA was conducted. This analysis revealed a significant time-on-task (in seconds) difference between the conditions during practice, F(3, 120.28) = 16.19, p < .001, η2 = .22. Pairwise comparisons showed that time-on-task was comparable between erroneous examples (M = 862.79, SD = 422.43) and correct examples (M = 839.58, SD = 298.33) and between contrasting examples (M = 512.29, SD = 130.21) and practice problems (M = 500.41, SD = 130.21). However, time-on-task was significantly higher in the first two conditions compared to the latter two conditions (erroneous examples = correct examples > contrasting examples = practice problems), all p’s < .001. This should be considered when interpreting the results on effort and posttest performance.
Main analyses
Descriptive and test statistics are presented in Table 2, 3, and 4. Correlations between several variables are presented in Table 5. It is important to realize that we measured mental effort as an indicator of overall experienced cognitive load. It is known, though, that the relation with learning depends on the origin of the experienced cognitive load. That is, if it originates mainly from germane processes that contribute to learning, high load would positively correlate with test performance, if it originates from extraneous processes, it would negatively correlate with test performance. Caution is warranted in interpreting these correlations, however, because of the exploratory nature of these correlation analyses, which makes it impossible to control for the probability of type 1 errors. We also exploratively analyzed invested mental effort and time-on-task data on the posttest; however, these analyses did not have much added value for this paper and, therefore, are not reported here but will be provided on our OSF-project page.
Performance during the practice phase
As each condition received different prompts during practice, performance during the practice phase could not be meaningfully compared between conditions and, therefore, we decided to report descriptive statistics only to describe the level of performance during the practice phase per condition (see Table 2). Descriptive statistics showed that participants earned more than half of the maximum total score while studying correct examples or engaging in contrasting examples. Participants who studied erroneous examples or solved practice problems performed worse during practice.
Mental effort during learning
A one-way ANOVA revealed a significant main effect of Practice Type on mental effort invested in the practice tasks. Contrary to hypothesis 1, a Tukey post hoc test revealed that participants who solved practice problems invested significantly less effort (M = 4.28, SD = 1.11) than participants who engaged in contrasting examples (M = 5.08, SD = 1.29, p = .022) or studied erroneous examples (M = 5.17, SD = 1.19, p = .008). There were no other significant differences in effort investment between conditions. Interestingly, invested mental effort during contrasting examples correlated negatively with pretest to posttest performance gains on learning items, indicating that the experienced load originated mainly from extraneous processes (see Table 5).
Test performance
The data on learning items were analyzed with two 2 × 4 mixed ANOVAs with Test Moment (pretest and immediate posttest/immediate posttest and 3-week delayed posttest) as within-subjects factor and Practice Type (correct examples, erroneous examples, contrasting examples, and practice problems) as between-subjects factor. Because transfer items were not included in the pretest, the data on transfer items were analyzed by a 2 × 4 mixed ANOVA with Test Moment (immediate posttest and 3-week delayed posttest) as within-subjects factor and Practice Type (correct examples, erroneous examples, contrasting examples, and practice problems) as between-subjects factor.
Performance on learning items
In line with Hypothesis 2, the pretest-immediate posttest analysis showed a main effect of Test Moment on performance on learning items: participants’ performance improved from pretest (M = 27.26, SE = 1.43) to immediate posttest (M = 49.98, SE = 1.87). In contrast to Hypothesis 3, the results did not reveal a main effect of Practice Type, nor an interaction between Practice Type and Test Moment. The second analysis (N = 154)—to test whether effects are still present after 3 weeks—showed a main effect of Test Moment: participants performed better on the delayed posttest (M = 55.54, SE = 2.16) compared to the immediate posttest (M = 50.95, SE = 2.00). Again, contrary to our hypothesis, there was no main effect of Practice Type, nor an interaction between Practice Type and Test Moment.
Performance on transfer items
The results revealed no main effect of Test Moment. Moreover, in contrast to Hypothesis 4, the results did not reveal a main effect of Practice Type, nor an interaction between Practice Type and Test Moment.Footnote 3
Exploratory analyses
Participants from one of the study programs were tested again after a 9-month delay. Regarding performance on learning items, a 2 × 4 mixed ANOVA with Test Moment (3-week delayed posttest or 9-month delayed posttest) as within-subjects factor and Practice Type (correct examples, erroneous examples, contrasting examples, and practice problems) as between-subjects factor revealed a main effect of Test Moment (see Table 2): participants’ performance improved from 3-week delayed posttest (M = 53.30, SE = 2.69) to 9-month delayed posttest (M = 63.00, SE = 2.24). The results did not reveal a main effect of Practice Type, nor an interaction between Practice Type and Test Moment.
Regarding performance on transfer items, a 2 × 4 mixed ANOVA with Test Moment (3-week delayed posttest and 9-month delayed posttest) as within-subjects factor and Practice Type (correct examples, erroneous examples, contrasting examples, and practice problems) as between-subjects factor revealed a main effect of Test Moment (see Table 2): participants performed lower on the 3-week delayed test (M = 19.25, SE = 1.60) than the 9-month delayed test (M = 24.84, SE = 1.67). The results did not reveal a main effect of Practice Type, nor an interaction between Practice Type and Test Moment.
Discussion
Previous research has demonstrated that providing students with explicit instructions combined with practice on domain-relevant tasks was beneficial for learning to reason in an unbiased manner (Heijltjes et al., 2014a, 2014b, 2015), and that practice consisting of worked example study was more effective for novices’ learning than practice problem solving (Van Peppen et al., 2021). However, this was not sufficient to establish transfer to novel tasks. With the present study, we aimed to find out whether contrasting examples—which has been proven effective for promoting transfer in other learning domains—would promote learning and transfer of reasoning skills.
Findings and implications
Our results corroborate the finding of previous studies (e.g., Heijltjes et al., 2015; Van Peppen et al., 2018, 2021) that providing students with explicit instructions and practice activities is effective for learning to avoid biased reasoning (Hypothesis 1), since we found considerable pretest to immediate posttest gains on practiced items. Moreover, our results revealed that participants’ performance improved even further after a 3-week and a 9-month delay, although the latter finding could also be attributed to the further instructions that were given in courses in-between the 3-week and 9-month follow up. That students improved in the longer term seems to indicate that our instructional intervention triggered active and deep processing and contributed to storage strength. Hence, our findings provide further evidence that a relatively brief instructional intervention including explicit instructions and practice opportunities is effective for learning of CT-skills, which is promising for educational practice.
In contrast to our expectations, however, we did not find any differences among conditions on either learning or transfer (Hypothesis 3). It is surprising that the present study did not reveal a beneficial effect of studying correct examples as opposed to practicing with problems, as this worked example effect has been demonstrated with many different tasks (Renkl, 2014; Van Gog et al., 2019), including heuristics-and-biases tasks (Van Peppen et al., 2021).
Given that most studies on the worked example effect use pure practice conditions or give minimal instructions prior to practice (e.g., Van Gog et al., 2019), whereas the current study was preceded by instructions including two worked examples, one might wonder whether this contributed to the lack of effect. That is, the effects are usually not investigated in a context in which elaborate processing of instructions precedes practice, as in the current (classroom) study, and this may have affected the results. It seems possible that the CT-instructions already had a substantial effect on learning unbiased reasoning, making it difficult to find differential effects of different types of practice activities. This suggestion, however, contradicts the relatively low performance during the practice phase. Moreover, one could argue that if these instructions would lead to higher prior knowledge, it should render the correct worked examples less useful (cf. research on the ‘expertise reversal effect’) and should help those in the other practice conditions perform better on the practice problems, but we did not find that either. Furthermore, these instructions were also provided in a previous study in which a worked example effect was found in two experiments (Van Peppen et al., 2021). A major difference between that prior study and this one, however, is that in the present study, participants were prompted to self-explain while studying examples or solving practice problems. Prompting self-explanations, however, seems to encourage students to engage in deep processing during learning (Chi et al., 1994), especially for students with sufficient prior knowledge (Renkl & Atkinson, 2010). In the present study, this might have interfered with the usual worked-example effect. However, the quality of the self-explanations was higher in the correct example condition than in the problem-solving condition (i.e., performance during the practice phase scores), making the absence of a worked example effect even more remarkable. Given that the worked example effect mainly occurs for novices, one could argue that participants in the current study had more prior knowledge than participants in that prior study; however, it concerned a similar group of students and descriptive statistics showed that students performed comparable on average in both studies.
Another potential explanation might lie in the number of practice tasks, which differed between the prior study (nine tasks: Van Peppen et al., 2021) and present study (four tasks), and which might moderate the effect of worked examples. The mean scores on the pretests as well as the performance progress in the practice problem condition was comparable with the previous study, but the progress of the worked example condition was considerably smaller. As it is crucial for a worked example effect that the worked-out solution procedures are understood, it might be that the effect did not emerge in the present study because participants did not get sufficient worked examples during practice.
This might perhaps also explain why contrasting examples did not benefit learning or transfer in the present study. Possibly, students first need to gain a better understanding of the subject matter with heuristics-and-biases tasks before they are able to benefit from aligning the examples (Rittle-Johnson et al., 2009). In particular the lack of transfer effects might be related to the duration or extensiveness of the practice activities; even though students learned to solve reasoning tasks, their subject knowledge may have been insufficient to solve novel tasks. As such, it can be argued that establishing transfer needs longer or more extensive practice. Contrasting examples seem to help students extend and refine their knowledge and skills through engaging in comparing activities and analyzing errors, that is, they seem to help them to correctly update schemas of correct concepts and strategies and to create schemas for erroneous strategies reducing the probability of recurring erroneous solutions in the future. However, more attention may need to be paid to the acquisition of the new knowledge and integration with wat students already know (see the Dimensions of Learning framework; Marzano et al., 1993). Potentially, having contrasting examples preceded by a more extensive instruction phase to guarantee a better understanding of logical and statistical reasoning would enhance learning and establish transfer. Another possibility would be to provide more guidance in the contrasting examples, as has been done in previous studies by explicitly marking the erroneous examples as incorrect and prompting students to reflect or elaborate on the examples (e.g., Durkin & Rittle-Johnson, 2012; Loibl & Leuders, 2018, 2019). It should be noted though, that the lower time on task in the contrasting condition might also be indicative of a motivational problem; whereas the side-by-side presentation was intended to encourage deep processing, it might have had the opposite effect that students might have engaged in superficial processing, just scanning to see where differences in the examples lay, without thinking much about the underlying principles. This idea is confirmed by the finding that invested mental effort during comparing correct and erroneous examples correlated negatively with performance gains on learning items, indicating that the experienced load originated mainly from extraneous processes. It would be interesting in future research to manipulate knowledge gained during instruction to investigate whether prior knowledge indeed moderates the effect of contrasting examples and to examine the interplay between contrasting examples, reflection/elaboration prompts, and final test performance.
Another possible explanation for the lack of a beneficial effect of contrasting examples might be related to the self-explanations prompts that were provided in the correct examples, erroneous examples, and practice problems conditions. Although the prompts differ, it is important to note that the explicit instruction to compare the solution process likely evokes self-explaining processes as well. The reason we added self-explanation prompts to the other conditions was to rule out an effect of prompting as such, as well as a potential effect of time on task (i.e., the text length in the contrasting examples condition was considerably longer than in the other conditions). The positive effect of contrasting examples might have been negated by a positive effect of the self-explanation prompts given in the other conditions. However, had we found a positive effect of comparing, as we expected, our design would have increased the likelihood that this was due to the comparison process and not just to more in-depth processing or higher processing time through self-explaining. Unexpectedly, we did find time-on-task differences between conditions during practice, but this does not seem to affect our findings. Time-on-task during practice was not correlated with learning and transfer posttest performance. This also becomes apparent from the condition means, i.e., the conditions with the lowest time-on-task means did not differ on learning and transfer compared to the conditions with the highest time-on-task means.
The classroom setting might also explain why there were no differential effects of contrasting examples. This study was conducted as part of an existing course and the learning materials were relevant for the course/exam and. Because of that, students’ willingness to invest effort in their performance may have been higher than is generally the case in psychological laboratory studies: their performance on such tasks actually mattered (intrinsically or extrinsically) to them. As such, students in the control conditions may have engaged in generative processing themselves, for instance by trying to compare the given correct (or erroneous) examples with internally represented erroneous (or correct) solutions. Therefore, it is possible that effects of generative processing strategies such as comparing correct and erroneous examples found in the psychological laboratory—where students participate to earn required research credits and the learning materials are not part of their study program—might not readily transfer to field experiments conducted in real classrooms.
The absence of differential effects of the practice activities on learning and transfer may also be related to the affective and attitudinal dimension of CT. Attitudes and perceptions about learning affect learning (Marzano et al., 1993), probably even more so in the CT-domain than in other learning domains. Being able to think critically relies heavily on the extent to which one possesses the requisite skills and is able to use these skills, but also on whether one is inclined to use these skills (i.e., thinking dispositions; Perkins et al., 1993).
The present study raises further questions about how transfer of CT-skills can be promoted. Although several studies have shown that to enhance transfer of knowledge or skills, instructional strategies should contribute to storage strength by effortful learning conditions that trigger active and deep processing (desirable difficulties; e.g., Bjork & Bjork, 2011), the present study—once again (Van Peppen et al., 2018, 2021; Heijltjes et al., 2014a, 2014b, 2015)—showed that this may not apply to transfer of CT-skills. This lack of transfer could lie in inadequate recall of the acquired knowledge, recognition that the acquired knowledge is relevant to the new task, and/or the ability to actually map that knowledge onto the new task (Barnett & Ceci, 2002). Following this, a further study should elucidate what the underlying mechanism(s) is/are to shed more light on how to promote transfer of CT-skills.
Limitations and strengths
One limitation of this study is that our measures showed low levels of reliability. Under these circumstances, the probability of detecting a significant effect—given one exists—are low (e.g., Cleary et al., 1970; Rogers & Hopkins, 1988), and subsequently, the chance that Type 2 errors have occurred in the current study is relatively high. In our study, the low levels of reliability can probably be explained by the multidimensional nature of the CT-test, that is, it represents multiple constructs that do not correlate with each other. Performance on these tasks depends not only on the extent to which that task elicits a bias (resulting from heuristic reasoning), but also on the extent to which a person possesses the requisite mindware (e.g., rules or logic or probability). Thus, systematic variance in performance on such tasks can either be explained by a person’s use of heuristics or his/her available mindware. If it differs per item to what extent a correct answer depends on these two aspects, and if these aspects are not correlated, there may not be a common factor explaining all interrelationships between the measured items. Moreover, the reliability issue may have increased even more since multiple task types were included in the CT-skills tests, requiring different, and perhaps uncorrelated, types of mindware (e.g., rules of logic or probability). Future research, therefore, would need to find ways to improve CT measures (i.e., decrease measurement error), for instance by narrowing down the test into a single measurable construct, or should utilize measures known to have acceptable levels of reliability (LeBel & Paunonen, 2011). The latter option seems challenging, however, as multiple studies report rather low levels of reliability of tests consisting of heuristics and biases tasks (Aczel et al., 2015; West et al., 2008) and revealed concerns with the reliability of widely used standardized CT tests, particularly with regard to subscales (Bernard et al., 2008; Bondy et al., 2001; Ku, 2009; Leppa, 1997; Liu et al., 2014; Loo & Thorpe, 1999). This raises the question whether these issues are related to the general construct CT. To achieve further progress in research on instructional methods for teaching CT, more knowledge on the construct validity of CT in general and unbiased reasoning in particular is needed. When the aim is to evaluate CT as a whole, one should perhaps move towards a more holistic measurement method, for instance by performing pairwise comparisons (i.e., comparative judgment; Bramley & Vitello, 2018; Lesterhuis et al., 2017). If, however, the intention is to measure specific aspects of CT, one should indicate specifically which aspect of CT to measure and select a suitable test for that aspect. Mainly considering that individual aspects of CT may not be as strongly correlated as thought and then could not be included in one scale.
Another point worth mentioning, is that we opted for assessing invested mental effort, which reflects the amount of cognitive load students experienced. This is informative when combined with their performance (for a more elaborate discussion, see Van Gog & Paas, 2008). Moreover, research has shown that it is important to measure cognitive load immediately after each task (e.g., Schmeck et al., 2015; Van Gog et al., 2012) and the mental effort rating scale (Paas, 1992) is easy to apply after each task. However, it unfortunately does not allow us to distinguish between different types of load. It should be noted, though, that it seems very challenging to do this with other measurement instruments (e.g., Skulmowski & Rey, 2017). Also, instruments that might be suited for this purpose, for example the rating scale developed by Leppink et al. (2013), would have been too long to apply after each task in the present study.
A strength of the current study is that it was conducted in a real educational setting as part of an existing CT course, which increases ecological validity. Despite the wealth of worked examples research, classroom studies are relatively rare. Interestingly, (multi-session) classroom studies on math and chemistry have also failed to find the worked example effect, although—in contrast to the present study—worked examples often did show clear efficiency benefits compared to practice problems (McLaren et al., 2016; Van Loon-Hillen et al., 2012). In line with our finding, a classroom study by Isotani et al. (2011) indicated that (high prior knowledge) students did not benefit more from studying erroneous examples than from correct examples or practice problems. As discussed earlier in the discussion, the classroom setting might explain the absence of generative processing strategies on learning and transfer. This suggests a theoretical implication, namely that beneficial effects of such strategies might become smaller when the willingness to invest increases and vice versa.
Conclusion
To conclude, based on the findings of the present study, comparing correct and erroneous examples (i.e., contrasting examples) does not seem to be a promising instructional method to further enhance learning and transfer of specific—and specifically tested—CT skills. Consequently, our findings raise questions about the preconditions of contrasting examples effects and effects of generative processing strategies in general, such as the setting in which they are presented to students. Further research on the exact boundary conditions, through solid laboratory and classroom studies, is therefore recommended. Moreover, this study provides valuable insights for educational practice. That is, providing students with explicit CT-instruction and the opportunity to practice with domain-relevant problems in a relatively short instructional intervention has the potential to improve learning. The format of the practice tasks does not seem to matter much, although a prior study did find a benefit of studying correct examples, which might therefore be the safest bet. Finally, this study again underlines the great difficulty of designing instructions to enhance CT-skills in such a way that these would also transfer across tasks/domains.
Data availability
All data, script files, and materials are provided on the Open Science Framework (OSF) project page that we created for this study (anonymized view-only link: https://osf.io/8zve4/?view_only=ca500b3aeab5406290310de34323457b).
Code availability
Not applicable.
Notes
This study investigated effects of interleaved practice (as opposed to blocked practice) on students’ learning and transfer of unbiased reasoning. Given that interleaved practice seems to impose high cognitive load, which may hinder learning, it was additionally tested whether this effect interacts with the format of the practice tasks (i.e., correct examples or practice problems).
We had no access to another CT-lesson of the Public Administration program, so due to practical reasons, students of this program were not administered to the 9-month delayed posttest.
We also exploratively analyzed the learning and transfer data for each individual measurement point and we analyzed performance on single learning and transfer items. The outcomes did not deviate markedly from the findings on sum scores (i.e., no effects of Practice Type were found). Test statistics can be found on our OSF-project page and the descriptive statistics of performance per single item can be found in Table 4.
References
Abrami, P. C., Bernard, R. M., Borokhovski, E., Wade, A., Surkes, M. A., Tamim, R., & Zhang, D. (2008). Instructional interventions affecting critical thinking skills and dispositions: A stage 1 meta-analysis. Review of Educational Research, 78, 1102–1134. https://doi.org/10.3102/0034654308326084
Abrami, P. C., Bernard, R. M., Borokhovski, E., Waddington, D. I., Wade, C. A., & Persson, T. (2014). Strategies for teaching students to think critically: A meta-analysis. Review of Educational Research, 85, 275–314. https://doi.org/10.3102/0034654314551063
Aczel, B., Bago, B., Szollosi, A., Foldes, A., & Lukacs, B. (2015). Measuring individual differences in decision biases: Methodological considerations. Frontiers in Psychology. https://doi.org/10.3389/fpsyg.2015.01770
Adams, D. M., McLaren, B. M., Durkin, K., Mayer, R. E., Rittle-Johnson, B., Isotani, S., & Van Velsen, M. (2014). Using erroneous examples to improve mathematics learning with a web-based tutoring system. Computers in Human Behavior, 36, 401–411. https://doi.org/10.1016/j.chb.2014.03.053
Angeli, C., & Valanides, N. (2009). Instructional effects on critical thinking: Performance on ill-defined issues. Learning and Instruction, 19, 322–334. https://doi.org/10.1016/j.learninstruc.2008.06.010
Arum, R., & Roksa, J. (2011). Limited learning on college campuses. Society, 48, 203–207. https://doi.org/10.1007/s12115-011-9417-8
Atkinson, R. K., Renkl, A., & Merrill, M. M. (2003). Transitioning from studying examples to solving problems: Effects of self-explanation prompts and fading worked-out steps. Journal of Educational Psychology, 95, 774–783. https://doi.org/10.1037/0022-0663.95.4.774
Barbieri, C., & Booth, J. L. (2016). Support for struggling students in algebra: Contributions of incorrect worked examples. Learning and Individual Differences, 48, 36–44. https://doi.org/10.1016/j.lindif.2016.04.001
Barnett, S. M., & Ceci, S. J. (2002). When and where do we apply what we learn?: A taxonomy for far transfer. Psychological Bulletin, 128, 612–636. https://doi.org/10.1037/0033-2909.128.4.612
Beaulac, G. & Kenyon, T. (2014). Critical thinking education and debiasing. Informal Logic, 34, 341–363. https://doi.org/10.22329/il.v34i4.4203
Bernard, R. M., Zhang, D., Abrami, P. C., Sicoly, F., Borokhovski, E., & Surkes, M. A. (2008). Exploring the structure of the Watson-Glaser Critical Thinking Appraisal: One scale or many subscales? Thinking Skills and Creativity, 3, 15–22. https://doi.org/10.1016/j.tsc.2007.11.001
Bjork, E. L., & Bjork, R. A. (2011). Making things hard on yourself, but in a good way: Creating desirable difficulties to enhance learning. In M. A. Gernsbacher, R. W. Pew, & J. R. Pomerantz (Eds.), Psychology and the real world: Essays illustrating fundamental contributions to society (pp. 59–68). Worth Publishers.
Bondy, K. N., Koenigseder, L. A., Ishee, J. H., & Williams, B. G. (2001). Psychometric properties of the California Critical Thinking Tests. Journal of Nursing Measurement, 9, 309–328. https://doi.org/10.1891/1061-3749.9.3.309
Booth, J. L., Lange, K. E., Koedinger, K. R., & Newton, K. J. (2013). Using example problems to improve student learning in algebra: Differentiating between correct and incorrect examples. Learning and Instruction, 25, 24–34. https://doi.org/10.1016/j.learninstruc.2009.10.001
Booth, J. L., Oyer, M. H., Paré-Blagoev, E. J., Elliot, A. J., Barbieri, C., Augustine, A., & Koedinger, K. R. (2015). Learning algebra by example in real-world classrooms. Journal of Research on Educational Effectiveness, 8, 530–551. https://doi.org/10.1080/19345747.2015.1055636
Bramley, T., & Vitello, S. (2018). The effect of adaptivity on the reliability coefficient in adaptive comparative judgement. Assessment in Education: Principles, Policy & Practice, 2018, 1–16. https://doi.org/10.1080/0969594X.2017.1418734
Charter, R. A. (2003). Study samples are too small to produce sufficiently precise reliability coefficients. The Journal of General Psychology, 130, 117–129.
Chi, M. T. H., de Leeuw, N., Chiu, M., & LaVancher, C. (1994). Eliciting self-explanation improves understanding. Cognitive Science, 18, 439–477. https://doi.org/10.1207/s15516709cog1803_3
Cleary, T. A., Linn, R. L., & Walster, G. W. (1970). Effect of reliability and validity on power of statistical tests. Sociological Methodology, 2, 130–138. https://doi.org/10.1037/a0031026
Cohen, J (1988). Statistical power analysis for the behavioral sciences (2nd. ed., reprint). Psychology Press.
Davies, M. (2013). Critical thinking and the disciplines reconsidered. Higher Education Research & Development, 32, 529–544. https://doi.org/10.1080/07294360.2012.697878
Dunlosky, J., Rawson, K. A., Marsh, E. J., Nathan, M. J., & Willingham, D. T. (2013). Improving students’ learning with effective learning techniques: Promising directions from cognitive and educational psychology. Psychological Science in the Public Interest, 14, 4–58. https://doi.org/10.1177/1529100612453266
Durkin, K., & Rittle-Johnson, B. (2012). The effectiveness of using incorrect examples to support learning about decimal magnitude. Learning and Instruction, 22, 206–214. https://doi.org/10.1016/j.learninstruc.2011.11.001
Ennis, R. H. (1989). Critical thinking and subject specificity: Clarification and needed research. Educational Researcher, 18, 4–10. https://doi.org/10.3102/0013189X018003004
Evans, J. S. B. (2002). Logic and human reasoning: An assessment of the deduction paradigm. Psychological Bulletin, 128, 978–996. https://doi.org/10.1037/0033-2909.128.6.978
Evans, J. S. B. (2003). In two minds: Dual-process accounts of reasoning. Trends in Cognitive Sciences, 7, 454–459. https://doi.org/10.1016/j.tics.2003.08.012
Evans, J. S. B., Barston, J. L., & Pollard, P. (1983). On the conflict between logic and belief in syllogistic reasoning. Memory & Cognition, 11, 295–306. https://doi.org/10.3758/BF03196976
Facione, P. A. (1990). Critical thinking: A statement of expert consensus for purposes of educational assessment and instruction. The California Academic Press.
Faul, F., Erdfelder, E., Buchner, A., & Lang, A. G. (2009). Statistical power analyses using G* Power 3.1: Tests for correlation and regression analyses. Behavior Research Methods, 41, 1149–1160. https://doi.org/10.3758/BRM.41.4.1149.
Flores, K. L., Matkin, G. S., Burbach, M. E., Quinn, C. E., & Harding, H. (2012). Deficient critical thinking skills among college graduates: Implications for leadership. Educational Philosophy and Theory, 44, 212–230. https://doi.org/10.1111/j.1469-5812.2010.00672.x
Fong, G. T., Krantz, D. H., & Nisbett, R. E. (1986). The effects of statistical training on thinking about everyday problems. Cognitive Psychology, 18, 253–292. https://doi.org/10.1016/0010-0285(86)90001-0
Ginns, P. (2006). Integrating information: A meta-analysis of the spatial contiguity and temporal contiguity effects. Learning and Instruction, 16, 511–525. https://doi.org/10.1016/j.learninstruc.2006.10.001
Grabowski, B. (1996). Generative learning. Past, present, and future. In D. H. Jonassen (Ed.), Handbook of research for educational communications and technology (pp. 897–918). Macimillian Library Reference.
Große, C. S., & Renkl, A. (2007). Finding and fixing errors in worked examples: Can this foster learning outcomes? Learning and Instruction, 17, 612–634. https://doi.org/10.1016/j.learninstruc.2007.09.008
Halpern, D. F. (2014). Critical thinking across the curriculum: A brief edition of thought & knowledge. Routledge.
Heijltjes, A., Van Gog, T., Leppink, J., & Paas, F. (2014a). Improving critical thinking: Effects of dispositions and instructions on economics students’ reasoning skills. Learning and Instruction, 29, 31–42. https://doi.org/10.1016/j.learninstruc.2013.07.003
Heijltjes, A., Van Gog, T., & Paas, F. (2014b). Improving students’ critical thinking: Empirical support for explicit instructions combined with practice. Applied Cognitive Psychology, 28, 518–530. https://doi.org/10.1002/acp.3025
Heijltjes, A., Van Gog, T., Leppink, J., & Paas, F. (2015). Unraveling the effects of critical thinking instructions, practice, and self-explanation on students’ reasoning performance. Instructional Science, 43, 487–506. https://doi.org/10.1002/acp.3025
Huber, C. R., & Kuncel, N. R. (2016). Does college teach critical thinking? A meta-analysis. Review of Educational Research, 86, 431–468. https://doi.org/10.3102/0034654315605917
Ibiapina, C., Mamede, S., Moura, A., Elói-Santos, S., & van Gog, T. (2014). Effects of free, cued and modelled reflection on medical students’ diagnostic competence. Medical Education, 48, 796–805. https://doi.org/10.1111/medu.12435
Isotani, S., Adams, D., Mayer, R. E., Durkin, K., Rittle-Johnson, B., & McLaren, B. M. (2011). Can erroneous examples help middle-school students learn decimals? In Proceedings of the sixth European conference on technology enhanced learning: Towards ubiquitous learning (EC-TEL-2011).
Kalyuga, S. (2011). Cognitive load theory: How many types of load does it really need? Educational Psychology Review, 23, 1–19. https://doi.org/10.1007/s10648-010-9150-7
Kassin, S. M., Dror, I. E., & Kukucka, J. (2013). The forensic confirmation bias: Problems, perspectives, and proposed solutions. Journal of Applied Research in Memory and Cognition, 2, 42–52. https://doi.org/10.1016/j.jarmac.2013.01.001.
Kawasaki, M. (2010). Learning to solve mathematics problems: The impact of incorrect solutions in fifth grade peers’ presentations. Japanese Journal of Developmental Psychology, 21, 12–22.
Ku, K. Y. L. (2009). Assessing students’ critical thinking performance: Urging for measurements using multi-response format. Thinking Skills and Creativity, 4, 70–76. https://doi.org/10.1016/j.tsc.2009.02.001
Lai, E. R. (2011). Critical thinking: A literature review. Pearson’s Research Reports, 6, 40–41.
LeBel, E. P., & Paunonen, S. V. (2011). Sexy but often unreliable: The impact of unreliability on the replicability of experimental findings with implicit measures. Personality and Social Psychology Bulletin, 37, 570–583. https://doi.org/10.1177/0146167211400619
Leppa, C. J. (1997). Standardized measures of critical thinking: Experience with the California Critical Thinking Tests. Nurse Education, 22, 29–33.
Leppink, J., Paas, F., Van der Vleuten, C. P., Van Gog, T., & Van Merriënboer, J. J. (2013). Development of an instrument for measuring different types of cognitive load. Behavior Research Methods, 45, 1058–1072. https://doi.org/10.3758/s13428-013-0334-1
Lesterhuis, M., Verhavert, S., Coertjens, L., Donche, V., & De Mayer, S. (2017). Comparative judgement as a promising alternative to score competences. In E. Cano & G. Ion (Eds.), Innovative practices for higher education assessment and measurement (pp. 119–138). IGI Global. https://doi.org/10.4018/978-1-5225-0531-0.ch007
Liu, O. L., Frankel, L., & Roohr, K. C. (2014). Assessing critical thinking in higher education: Current state and directions for next-generation assessment. ETS Research Report Series, 2014, 1–23. https://doi.org/10.1002/ets2.12009
Loibl, K., & Leuders, T. (2018). Errors during exploration and consolidation—The effectiveness of productive failure as sequentially guided discovery learning. Journal für Mathematik-Didaktik, 39, 69–96. https://doi.org/10.1007/s13138-018-0130-7
Loibl, K., & Leuders, T. (2019). How to make failure productive: Fostering learning from errors through elaboration prompts. Learning and Instruction, 62, 1–10. https://doi.org/10.1016/j.learninstruc.2019.03.002
Loo, R., & Thorpe, K. (1999). A psychometric investigation of scores on the Watson-Glaser critical thinking appraisal new forms. Educational and Psychological Measurement, 59, 995–1003. https://doi.org/10.1177/00131649921970305
Markovits, H., & Nantel, G. (1989). The belief-bias effect in the production and evaluation of logical conclusions. Memory & Cognition, 17, 11–17. https://doi.org/10.3758/BF03199552
Marzano, R. J., Pickering, D., & McTighe, J. (1993). Assessing student outcomes: Performance assessment using the Dimensions of Learning Model. Association for Supervision and Curriculum Development.
McLaren, B. M., Adams, D. M., & Mayer, R. E. (2015). Delayed learning effects with erroneous examples: A study of learning decimals with a web-based tutor. International Journal of Artificial Intelligence in Education, 25, 520–542. https://doi.org/10.1007/s40593-015-0064-x
McLaren, B. M., Van Gog, T., Ganoe, C., Karabinos, M., & Yaron, D. (2016). The efficiency of worked examples compared to erroneous examples, tutored problem solving, and problem solving in computer-based learning environments. Computers in Human Behavior, 55, 87–99. https://doi.org/10.1016/j.chb.2015.08.038
Moore, T. (2004). The critical thinking debate: How general are general thinking skills? Higher Education Research & Development, 23, 3–18. https://doi.org/10.1080/0729436032000168469
Newstead, S. E., Pollard, P., Evans, J. St. B. T., & Allen, J. L. (1992). The source of belief bias effects in syllogistic reasoning. Cognition, 45, 257–284. https://doi.org/10.1016/0010-0277(92)90019-E
Nievelstein, F., Van Gog, T., Van Dijck, G., & Boshuizen, H. P. (2013). The worked example and expertise reversal effect in less structured tasks: Learning to reason about legal cases. Contemporary Educational Psychology, 38, 118–125. https://doi.org/10.1007/s11251-008-9076-3
Niu, L., Behar-Horenstein, L. S., & Garvan, C. W. (2013). Do instructional interventions influence college students’ critical thinking skills? A meta-analysis. Educational Research Review, 9, 114–128. https://doi.org/10.1016/j.edurev.2012.12.002
Nunally, J. C., & Bernstein, I. H. (1994). Psychometric theory (3rd ed.). McGraw-Hill.
Osborne, R. J., & Wittrock, M. C. (1983). Learning science: A generative process. Science Education, 67, 489–508. https://doi.org/10.1002/sce.3730670406
Paas, F. (1992). Training strategies for attaining transfer or problem solving skills in statistics: A cognitive-load approach. Journal of Educational Psychology, 84, 429–434. https://doi.org/10.1037/0022-0663.84.4.429
Paas, F., Renkl, A., & Sweller, J. (2003a). Cognitive load theory and instructional design: Recent developments. Educational Psychologist, 38, 1–4. https://doi.org/10.1207/S15326985EP3801_1
Paas, F., Tuovinen, J. E., Tabbers, H., & Van Gerven, P. W. (2003b). Cognitive load measurement as a means to advance cognitive load theory. Educational Psychologist, 38, 63–71. https://doi.org/10.1207/S15326985EP3801_8
Pascarella, E. T., Blaich, C., Martin, G. L., & Hanson, J. M. (2011). How robust are the findings of Academically Adrift? Change: The Magazine of Higher Learning, 43, 20–24. https://doi.org/10.1080/00091383.2011.568898
Perkins, D. N., & Salomon, G. (1992). Transfer of learning. In T. Husen & T. N. Postelwhite (Eds.), The international encyclopedia of educational (2nd ed., Vol. 11, pp. 6452–6457). Pergamon Press.
Perkins, D. N., Jay, E., & Tishman, S. (1993). Beyond abilities: A dispositional theory of thinking. MerrillPalmer Quarterly, 39, 1–21.
Renkl, A. (1999). Learning mathematics from worked-out examples: Analyzing and fostering self-explanations. European Journal of Psychology of Education, 14, 477–488. https://doi.org/10.1007/BF03172974
Renkl, A. (2014). Toward an instructionally oriented theory of example-based learning. Cognitive Science, 38, 1–37. https://doi.org/10.1111/cogs.12086
Renkl, A., & Atkinson, R. K. (2010). Learning from worked-out examples and problem solving. In J. Plass, R. Moreno, & R. Brünken (Eds.), Cognitive load theory and research in educational psychology (pp. 89–108). Cambridge University Press.
Renkl, A., & Eitel, A. (2019). Self-explaining: Learning about principles and their application. In J. Dunlosky & K. Rawson (Eds.), The Cambridge handbook of cognition and education (pp. 528–549). Cambridge University Press.
Renkl, A., Hilbert, T., & Schworm, S. (2009). Example-based learning in heuristic domains: A cognitive load theory account. Educational Psychology Review, 21, 67–78. https://doi.org/10.1007/s10648-008-9093-4
Ritchhart, R., & Perkins, D. N. (2005). Learning to think: The challenges of teaching thinking. In K. J. Holyoak & R. G. Morrison (Eds.), The Cambridge handbook of thinking and reasoning (pp. 775–802). Cambridge University Press.
Rittle-Johnson, B., Star, J. R., & Durkin, K. (2009). The importance of prior knowledge when comparing examples: Influences on conceptual and procedural knowledge of equation solving. Journal of Educational Psychology, 101, 836–852. https://doi.org/10.1037/a0016026.
Roelle, J., & Berthold, K. (2015). Effects of comparing contrasting cases on learning from subsequent explanations. Cognition and Instruction, 33, 199–225. https://doi.org/10.1080/07370008.2015.1063636
Rogers, W. T., & Hopkins, K. D. (1988). Power estimates in the presence of a covariate and measurement error. Educational and Psychological Measurement, 48, 647–656. https://doi.org/10.1177/0013164488483008
Salomon, G., & Perkins, D. N. (1989). Rocky roads to transfer: Rethinking mechanism of a neglected phenomenon. Educational Psychologist, 24, 113–142. https://doi.org/10.1207/s15326985ep2402_1.
Schmeck, A., Opfermann, M., Van Gog, T., Paas, F., & Leutner, D. (2015). Measuring cognitive load with subjective rating scales during problem solving: Differences between immediate and delayed ratings. Instructional Science, 43, 93–114. https://doi.org/10.1007/s11251-014-9328-3
Schworm, S., & Renkl, A. (2007). Learning argumentation skills through the use of prompts for self-explaining examples. Journal of Educational Psychology, 99, 285–296. https://doi.org/10.1037/0022-0663.99.2.285
Segall, D. O. (1994). The reliability of linearly equated tests. Psychometrika, 59, 361–375.
Siegler, R. S. (2002). Microgenetic studies of self-explanations. In N. Grannot & J. Parziale (Eds.), Microdevelopment: Transition processs in development and learning (pp. 31–58). Cambridge University Press.
Skulmowski, A., & Rey, G. D. (2017). Measuring cognitive load in embodied learning settings. Frontiers in Psychology, 8, 1191. https://doi.org/10.3389/fpsyg.2017.01191
Stanovich, K. E. (2011). Rationality and the reflective mind. Oxford University Press.
Stanovich, K. E., & West, R. F. (2000). Individual differences in reasoning: Implications for the rationality debate? Behavioral and Brain Sciences, 23, 645–665. https://doi.org/10.1017/S0140525X00003435
Stanovich, K. E., West, R. K., & Toplak, M. E. (2016). The rationality quotient: Toward a test of rational thinking. MIT Press.
Stark, R., Kopp, V., & Fischer, M. R. (2011). Case-based learning with worked examples in complex domains: Two experimental studies in undergraduate medical education. Learning and Instruction, 21, 22–33. https://doi.org/10.1016/j.learninstruc.2009.10.001
Sweller, J. (1988). Cognitive load during problem solving: Effects on learning. Cognitive Science, 12, 257–285. https://doi.org/10.1207/s15516709cog1202_4
Sweller, J., Van Merriënboer, J. J., & Paas, F. G. (1998). Cognitive architecture and instructional design. Educational Psychology Review, 10, 251–296.
Sweller, J., Ayres, P., & Kalyuga, S. (Eds.). (2011). Measuring cognitive load. In Cognitive load theory (pp. 71–85). Springer.
Tiruneh, D. T., Verburgh, A., & Elen, J. (2014). Effectiveness of critical thinking instruction in higher education: A systematic review of intervention studies. Higher Education Studies, 4, 1–17. https://doi.org/10.5539/hes.v4n1p1
Tiruneh, D. T., Weldeslassie, A. G., Kassa, A., Tefera, Z., De Cock, M., & Elen, J. (2016). Systematic design of a learning environment for domain-specific and domain-general critical thinking skills. Educational Technology Research and Development, 64, 481–505. https://doi.org/10.1007/s11423-015-9417-2
Trauzettel-Klosinski, S., & Dietz, K. (2012). Standardized assessment of reading performance: The new International Reading Speed Texts IReST. Investigative Ophthalmology & Visual Science, 53, 5452–5461. https://doi.org/10.1167/iovs.11-8284.
Tversky, A., & Kahneman, D. (1974). Judgment under uncertainty: Heuristics and biases. Science, 185, 1124–1131. https://doi.org/10.1126/science.185.4157.1124
Tversky, A., & Kahneman, D. (1983). Extensional versus intuitive reasoning: The conjunction fallacy in probability judgment. Psychological Review, 90, 293–315. https://psycnet.apa.org. https://doi.org/10.1037/0033-295X.90.4.293
Van den Broek, P., & Kendeou, P. (2008). Cognitive processes in comprehension of science texts: The role of co-activation in confronting misconceptions. Applied Cognitive Psychology, 22, 335–351. https://doi.org/10.1002/acp.1418
Van Gelder, T. V. (2005). Teaching critical thinking: Some lessons from cognitive science. College Teaching, 53, 41–48. https://doi.org/10.3200/CTCH.53.1.41-48
Van Gog, T., & Paas, F. (2008). Instructional efficiency: Revisiting the original construct in educational research. Educational Psychologist, 43, 16–26. https://doi.org/10.1080/00461520701756248
Van Gog, T., Paas, F., & Van Merriënboer, J. J. (2004). Process-oriented worked examples: Improving transfer performance through enhanced understanding. Instructional Science, 32, 83–98. https://doi.org/10.1023/B:TRUC.0000021810.70784.b0
Van Gog, T., Kirschner, F., Kester, L., & Paas, F. (2012). Timing and frequency of mental effort measurement: Evidence in favour of repeated measures. Applied Cognitive Psychology, 26, 833–839. https://doi.org/10.1002/acp.2883
Van Gog, T., Rummel, N., & Renkl, A. (2019). Learning how to solve problems by studying examples. In J. Dunlosky & K. Rawson (Eds.), The Cambridge handbook of cognition and education (pp. 183–208). Cambridge University Press.
VanLehn, K. (1999). Rule-learning events in the acquisition of a complex skill: An evaluation of cascade. The Journal of the Learning Sciences, 8, 71–125. https://doi.org/10.1207/s15327809jls0801_3
Van Loon-Hillen, N. H., Van Gog, T., & Brand-Gruwel, S. (2012). Effects of worked examples in a primary school mathematics curriculum. Interactive Learning Environments, 20, 89–99. https://doi.org/10.1080/10494821003755510
Van Peppen, L. M., Verkoeijen P. P. J. L., Heijltjes, A. E. G., Janssen, E. M., Koopmans, D., & Van Gog, T. (2018). Effects of self-explaining on learning and transfer of critical thinking skills. Frontiers in Education, 3, 100. https://doi.org/10.3389/feduc.2018.00100.
Van Peppen, L. M., Verkoeijen, P. P., Kolenbrander, S. V., Heijltjes, A. E., Janssen, E. M., & van Gog, T. (2021). Learning to avoid biased reasoning: Effects of interleaved practice and worked examples. Journal of Cognitive Psychology, 33, 304–326. https://doi.org/10.1080/20445911.2021.1890092.
West, R. F., Toplak, M. E., & Stanovich, K. E. (2008). Heuristics and biases as measures of critical thinking: Associations with cognitive ability and thinking dispositions. Journal of Educational Psychology, 100, 930–941. https://doi.org/10.1037/a0012842
Wittrock, M. C. (1974). Learning as a generative process. Educational Psychologist, 11, 87–95. https://doi.org/10.1080/00461527409529129
Wittrock, M. C. (1990). Generative processes of comprehension. Educational Psychologist, 24, 345–376. https://doi.org/10.1207/s15326985ep2404_2
Wittrock, M. C. (1992). Generative learning processes of the brain. Educational Psychologist, 27, 531–541. https://doi.org/10.1207/s15326985ep2704_8
Wittrock, M. C. (2010). Learning as a generative process. Educational Psychologist, 45, 40–45. https://doi.org/10.1080/00461520903433554
Acknowledgements
This research was funded by The Netherlands Organisation for Scientific Research (Project Number 409-15-203). The authors would like to thank Stefan V. Kolenbrander for his help with running this study and Esther Stoop and Marjolein Looijen for their assistance with coding the data.
Funding
This research was funded by The Netherlands Organisation for Scientific Research (Project Number 409-15-203).
Author information
Authors and Affiliations
Contributions
LP, PV, AH, and TG contributed to the conception and design of the study. LP and EM prepared the materials. LP collected the data, organized the database, and performed the statistical analyses. LP, PV, and TG interpreted the data. LP wrote the original draft of the manuscript and PV, AH, EM, and TG provided critical revision of the manuscript. All authors read and approved the submitted version of the manuscript.
Corresponding author
Ethics declarations
Conflict of interest
The authors declare that there is no conflict of interest.
Ethical approval
In accordance with the guidelines of the ethical committee at the Department of Psychology, Education and Child studies, Erasmus University Rotterdam, the study was exempt from ethical approval procedures because the materials and procedures were not invasive. All subjects gave written informed consent prior to participating in this study.
Additional information
Publisher's Note
Springer Nature remains neutral with regard to jurisdictional claims in published maps and institutional affiliations.
Supplementary Information
Below is the link to the electronic supplementary material.
Rights and permissions
Open Access This article is licensed under a Creative Commons Attribution 4.0 International License, which permits use, sharing, adaptation, distribution and reproduction in any medium or format, as long as you give appropriate credit to the original author(s) and the source, provide a link to the Creative Commons licence, and indicate if changes were made. The images or other third party material in this article are included in the article's Creative Commons licence, unless indicated otherwise in a credit line to the material. If material is not included in the article's Creative Commons licence and your intended use is not permitted by statutory regulation or exceeds the permitted use, you will need to obtain permission directly from the copyright holder. To view a copy of this licence, visit http://creativecommons.org/licenses/by/4.0/.
About this article
Cite this article
van Peppen, L.M., Verkoeijen, P.P.J.L., Heijltjes, A.E.G. et al. Enhancing students’ critical thinking skills: is comparing correct and erroneous examples beneficial?. Instr Sci 49, 747–777 (2021). https://doi.org/10.1007/s11251-021-09559-0
Received:
Accepted:
Published:
Issue Date:
DOI: https://doi.org/10.1007/s11251-021-09559-0