Abstract
This study investigates the (3 + 1)-dimensional extended Kairat-II model using Lie-Bäcklund symmetry (LBS) and the improved modified extended tanh-function approach (IMETFA). The bifurcation and sensitivity analyses are conducted to understand the stability and chaotic behavior of the model. Through graphical visualizations of phase diagrams, Lyapunov exponents, power spectra, fractal dimension and recurrence plots, the complex dynamics and stability characteristics of the model are elucidated. Additionally, the research focuses on the derivation of dark soliton and various combo soliton solutions of the Kairat-II model. The derived soliton solutions are graphically displayed in 3D and 2D plots. The obtained results are new and have never been reported in the literature for the considered equation.
Similar content being viewed by others
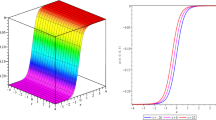
Explore related subjects
Discover the latest articles, news and stories from top researchers in related subjects.Avoid common mistakes on your manuscript.
1 Introduction
Nonlinear integrable partial differential equations (NLIPDEs) are important in many branches of mathematics and research because of their intricate mathematical structures and many practical applications [1, 2]. NLIPDEs are being studied within the framework of soliton theory due to their ability to represent complex nonlinear events, mathematical sophistication, and physical applicability. It has been found that soliton solutions provide a correct explanation for a wide range of physical phenomena, such as plasma waves, water waves, and optical pulses in fibres [3,4,5].
NLIPDEs are often the source of soliton solutions. Analytical solutions are necessary to fully analyse dynamics and apply them to real-world problems [6, 7]. Over time, numerous effective methods have been created to construct analytical solutions for NLIPDEs. Several scholars have used techniques such as the planner dynamical scheme [8], the modified Tanh method [9], the bilinear approach [10], the generalised Kudryashov technique [11], the exponential rational function approach [12, 13], separation of variable method [14], generalized extended function method [15] and the logarithmic transformation approach [16]. The extensive literature on the issue of many types of soliton solutions, such as kink, dark, and periodic solitons [17]. Various authors have studied soliton solutions and chaotic behaviour in a nonlinear Schrödinger model with a random potential [18], regularized long-wave equation [19] and complex Ginzburg-Landau equation [20].
Moreover, research has concentrated on modulation instability, sensitivity analysis, and the behaviour of soliton solutions in a fractional non-linear Schrödinger model with Kerr-Law nonlinearity [21]. Additionally, computational research has been done on modulation instability, stability analysis, and the finding of new travelling wave solutions in a non-dissipative double-dispersive microstrain wave model within microstructured solids [22], in addition to the fractional generalised perturbed KdV equation with a power law kernel [23]. Moreover, a fractional nonlinear tsunami shallow-water mathematical model with both singular and non-singular kernels has been analysed [24]. There has been a lot of recent research on bifurcations and chaotic dynamics for NLIPDEs by different mathematicians and academics, indicating that this is an active field of study [25]. As an illustration, studies have been conducted on the Lie symmetry dynamics, bifurcation, and sensitivity analysis of the Fokas model [26]. Furthermore, the author in [27] studied the Schrödinger model’s dynamical features and complicated analysis. The extended KP model dynamics and solitary waves are described in [28]. Similar to this, [29] studies the Sasa-Satsuma model’s bifurcations and other complex dynamics. Inspired by the previously stated valuable work, we explore the intricate evolutionary dynamics concealed within the Kairat-II model [30]. The model under consideration characterises the curve’s surface shape and finds use in multiple domains, including quantum mechanics, optical fibre, and optical communication [31,32,33].
The following is the standard integrable Kairat-II model [34]
The goal of this work is to investigate certain dynamics that are not covered in the literature, such as LBS, IMETFA, bifurcations, potential chaotic dynamics, and sensitivity to the (3+1)-dimensional extended Kairat-II equation [35]. Consider the (3+1)-dimensional extended Kairat-II equation as:
where \( v_x v_{xt} ~ \& ~v_tv_{xx}\) are nonlinear terms and \( \alpha _1,~\alpha _2~ \& ~\alpha _3\) are positive real numbers.
The following is the structure of this article:
A brief overview of the IMETFA is provided in the Sect. (2). The LBS of the governing model is examined in the Sect. (3). In the Sect. (4), the governing model is transformed into a dynamical system and the phase portrait behaviours are investigated through the application of sensitivity analysis, bifurcation, and chaos. To obtain soliton solutions of Eq. (1.2) via the IMETFA is provided in the Sect. (6). An examination of the physical discussions for produced solutions is provided in the Sect. (7). Finally, Sect. (8) summarizes the findings.
2 General overview of improved modified extended tanh-function approach
The improved modified extended Tanh-function approach (IMETFA) [36] was used to solve a wider variety of NLIPDEs. The approach has often been used to solve NLIPDEs in physics and mathematics.
Assume the generic form of NLIPDEs as
First, apply the wave transformation to get the nonlinear ordinary differential equations (ODEs). After obtaining the ODEs, consider the general solutions of the IMETFA below
where M is the constant of balancing principal and \( \gamma _m~ \& ~g_m\) are real constants that are not zero at the same time and the following auxiliary equation that satisfies the Eq. (2.2) is
where \( u_3,~u _2,~u _1~ \& ~u_0\) are integers. Calculating the constants \(R(\eta )\) and determining the value of m using the balance principle. Following are the cases to Eq. (2.3).
\(\bullet \) When \(u_0=~u_1=~u_3=0\), we acquired
\(\bullet \) When \(u_0=~u_1=~u_3=0\), we acquired
\(\bullet \) When \(u_0=~u_1=~u_2=0\), we acquired
\(\bullet \) When \(u_0=~u_1=~u_3=0\), we acquired
\(\bullet \) When \(u_1=~u_3=0\), we acquired four solutions to the Eq. (2.3) are displayed in Table 1. When \(u_1=~u_3=0\), these solutions were discovered. Columns 2, 3, and 4 display the values of \( u_0,~u_2~ \& ~u_4\) respectively. \(R(\eta )\) is dependent on the functions of Jacobi elliptics \(cd(\eta ,~\sigma ),~sn(\eta ,~\sigma ),~ns(\eta ,~\sigma ),\)
\( dc(\eta ,~\sigma ),~cn(\eta ,~\sigma ),~ns(\eta ,~\sigma )~ \& ~ds(\eta ,~\sigma ),\) as the fifth column demonstrates. The functions undergo modifications in response to variations in the values of the variables \( \eta ~ \& ~\sigma \).
Use the above cases of Eqs. (2.4-2.14), to acquire the desired soliton solutions which are discussed in Sect. (6).
3 Lie-Bäcklund transformations
This section considers the Lie-Bäcklund transformations provided to create the Lie-Bäcklund symmetry group of the Kairat-II model.
where \(\Xi \) is the Lie Bäcklund infinitesimal and \(\epsilon \) is the group parameter, which depends on \(x,y,z,t,v,v_x,v_v,v_z\) and \(v_t\). It can be demonstrated that under the transformations of Eq. (3.1), stays invariant the Eq. (1.2). The vector field then generates the Lie-Bäcklund symmetry group of Eq. (1.2) in the following form:
where V satisfies the condition \(V^3(\Delta )|_{\Delta =0}=0\), \(\Delta := v_{t}+\frac{1}{6}\delta _1 v_{xxx}+\frac{1}{16}\delta _2\delta _1(v)^2_{xxx}+\frac{15}{32}\delta _2^2v^2v_{x}+\alpha _1v_{x}+ \alpha _2v_{y}+ \alpha _3 v_{z}=0\). Here \(V^3\) satisfies the third prolongation of V defined as
Eq. (1.2) can be extended to get the following invariance condition by using the prolongation \(V^3\):
where
A system of NLIPDEs is obtained by substituting the equations of \(\Xi _x\), \(\Xi _y\), \(\Xi _z\), \(\Xi _t\), \(\Xi _{xx}\) and \(\Xi _{xxx}\) from Eqs. (3.6), (3.7) into Eq. (3.5) and by equating the coefficients of all partial derivatives of the dependent variable v of order greater than one to zero. Upon resolving this NLIPDE system, the Lie-Bäcklund infinitesimal is determined to be
where the real integers are \(b_1\), \(b_2\), \(b_3\) and \(b_4\). Thus, the Lie-Bäcklund symmetries of Eq. (1.2) at first order are produced by
This corresponds to the point symmetries are
4 Creation of system of ODEs from model (1.2)
This part is included for the presentation of the conversion of the suggested model (1.2) into a system of ODEs. First of all, wave transformation converts the proposed NLIPDEs into ODE. Then the resulting ODE is converted into a system of ODEs. Therefore consider the following:
where \(\eta =-t w_0+\tau _1 x+\tau _2 y+\tau _3 z\). The substitution of the Eq. (4.1) into the considered model (1.2), we get the ODE presented below
Integrating the Eq. (4.2) once concerning \(\eta \), we obtain:
Here we consider a real function \(\mathcal {W}(\eta )\), as \(r^{\prime }(\eta )=\mathcal {W}(\eta )\), so the above equation becomes:
Now we convert the suggested Eq. (4.4) into system of ODEs in the following form [37]:
here
5 Dynamical study and some new characteristics of the system (5.1)
This portion of the manuscript presents the dynamical study of the proposed system of ODEs (5.1). The characteristics we are going to explore in this part include the bifurcations, chaos, sensitivity, Poincaré section, return map, Lyapunov exponents and others. All the observations are properly discussed for the convenience.
We take start with bifurcations. Moreover, we graphically visualize the types of fixed or stationary points, which exists in the system. For this, we consider Hamiltonian function as:
To achieve the stationary or fixed points, consider the Eq.(5.1), in the form of:
finding the solution of the above system for \(\mathfrak {O}_{1}\) and \(\mathfrak {O}_{2}\), we get
Furthermore, determinant of Jacobian of system (5.1) is of the form:
The following are the possible cases for different stationary points:
Case I: \(\mathfrak {O}_1>0, ~\mathfrak {O}_2>0\)
Using values as \(\tau _1=20,~\tau _2=10,~\tau _3=0.01,~\alpha _1=1,~\alpha _2=1,~\alpha _3=1,~ w_0=0.1\), there are two real stationary points (SPs), which are: (0, 0) and \((-5,0)\) as depicted in Fig. 1a. It is obvious that, \(\left( 0,0\right) \) is saddle and \(\left( -5,0\right) \) is center point.
Case II: \(\mathfrak {O}_1>0, ~\mathfrak {O}_2<0\)
Using values as \(\tau _1=20,~\tau _2=10,~\tau _3=0.01,~\alpha _1=1,~\alpha _2=1,~\alpha _3=1,~ w_0=-0.1\), there is one real SP, which is: (0, 0) as depicted in Fig. 1b and it is a center point.
Case III: \(\mathfrak {O}_1<0, ~\mathfrak {O}_2>0\)
Using values as \(\tau _1=-20,~\tau _2=10,~\tau _3=0.01,~\alpha _1=1,~\alpha _2=1,~\alpha _3=1,~ w_0=0.1\), there are two real SPs, which are: (0, 0) and (1.8, 0) as depicted in Fig. 1c. It is obvious that, \(\left( 0,0\right) \) is center and \(\left( -5,0\right) \) is saddle point.
In Fig. 1a, which shows the visualization of Case I we observe a classical saddle point at the origin, indicated by the hyperbolic contours. These contours are a hallmark of a saddle point where the equilibrium is inherently unstable. The trajectories show a mixed stability scenario: they approach the saddle point in two directions, indicating stability, and diverge in the other two, highlighting instability. This type of saddle point is typical in systems with mixed stability properties, where certain perturbations result in stability, and others lead to instability. In Fig. 1b, which visualize the dynamics of Case II shows the elliptical contours centered around a point suggest a local minimum, depicting a stable equilibrium. The closed-loop trajectories circling this center indicate stable behavior. If perturbed, the system’s trajectories oscillate around the equilibrium without diverging, showcasing local stability. Figure 1a shows the visualization of Case III with another saddle point at the origin. The narrower contours suggest a slightly different dynamic, but the fundamental instability of the saddle point remains. Like typical saddle points, certain directions lead to convergence towards the point, while others cause divergence. Understanding these dynamics is crucial for predicting long-term behavior based on initial conditions. Similarly we can observe the center point as well at \(\mathcal {W}_1=1.8\).
Saddle points are vital in various fields. In optimization, they are critical points where the gradient is zero but are neither local minima nor maxima. They present directions of both ascent and descent, complicating optimization processes. In dynamical systems, saddle points are pivotal for stability analysis, as their presence can lead to divergent trajectories despite initial stability. This knowledge is crucial in machine learning, where they impact neural network training, physics for potential energy surface studies, and economics for equilibrium analysis. These plots provide valuable insights into different equilibrium and stability characteristics, underscoring the importance of understanding a system’s underlying structure. Recognizing and interpreting these points aids in predicting system behavior, optimizing performance, and ensuring stability across various applications.
5.1 Chaos and other characteristics
This portion of the manuscript is devoted to the presentation of the possible chaos, sensitivity and other useful characteristics. To analyze whether chaos exists or not the proposed system can be perturbed. So we consider the proposed system as:
where \(\xi _1\) is amplitude and \(\xi _2\) is frequency of the oscillations in the proposed system. In the figures 2-4, we present the dynamics of the system (5.1) with varying \(\xi _1\) and \(\xi _2\), where other parameters are fixed as \(\tau _1=20,~\tau _2=10,~\tau _3=0.01,~\alpha _1=1,~\alpha _2=1,~\alpha _3=1,~ w_0=0.1\).
In Fig. 2, the phase diagrams and time series plots illustrate the system’s behavior when specific values of amplitude (\(\xi _1\)) and frequency (\(\xi _2\)) are applied. The phase diagram 2a shows a complex, symmetric pattern around the origin, indicating periodic or quasi-periodic dynamics. The curves suggest a stable system with well-defined oscillations influenced by the chosen amplitude and frequency. The time series plots 2b and 2c further elucidate this periodic behavior. The plot of \(\mathcal {W}_1\) versus time 2b displays regular oscillations with consistent peaks and troughs, signifying a stable periodic signal influenced by the frequency \(\xi _2\). Similarly, the time series of \(\mathcal {W}_2\) 2c shows synchronized oscillations with \(\mathcal {W}_1\), underscoring the coupled dynamics of the system’s components under the given parameter set.
Figure 3 presents a different set of dynamics achieved by considering \(\xi _1=3\) and \(\xi _2=1.4\). The phase diagram 3a reveals a more spread-out pattern, indicating a change in the system’s behavior. The larger spread and distinct structure of the curves suggest a higher \(\xi _1\) and low \(\xi _2\), resulting in more complex chaotic dynamics. The intricate pattern points to higher amplitude oscillations and potentially different stability characteristics compared to previous one. The time series plots 3b and 3c support this observation by showing more pronounced oscillations with larger amplitudes. The \(\mathcal {W}_1\) time series 3b reveals higher amplitude oscillations, indicating a stronger influence of the increased amplitude parameter. The \(\mathcal {W}_2\) time series 3c also exhibits similar behavior, with synchronized oscillations that align with those of \(\mathcal {W}_1\). This synchronization highlights the coupled nature of the system’s dynamics under the new parameter set, with both components experiencing larger amplitude oscillations.
Figure 4 presents another set of phase diagrams and time series plots, showcasing the system’s behavior under a different combination of \(\xi _1 = 0.1\) and \(\xi _2 = 2.9\). These visualizations provide further insights into how varying these parameters influences the system’s dynamics. The phase diagram 4a exhibits a highly dense and compact pattern centered around the origin. The curves form a fine, intricate structure, indicating very small amplitude oscillations. The symmetric and dense nature of the pattern suggests a stable, yet highly sensitive, periodic or quasi-periodic behavior influenced by the small amplitude and high frequency. The time series plot for \(\mathcal {W}_1\) in 4b shows oscillations with very small amplitudes. Despite the small scale, the oscillations are regular, indicating stable periodic behavior. The plot reflects the influence of the frequency \(\xi _2\), showing a consistent oscillatory pattern over time. Similarly, the time series plot for \(\mathcal {W}_2\) in 4c reveals oscillations with very small amplitudes. The behavior of \(\mathcal {W}_2\) is synchronized with \(\mathcal {W}_1\), highlighting the coupled dynamics of the system. The regular oscillations suggest that the system remains in a stable periodic state under the given parameter set. By comparing Fig. 2 with Figs. 3 and 4, we observe how varying the amplitude and frequency parameters can lead to significantly different dynamic behaviors. Figure 4 represents a regime of very small amplitude oscillations, leading to a highly sensitive yet stable periodic state. This contrasts with the larger amplitude oscillations seen in Figs. 3 and 4, which resulted in more complex and spread-out dynamic patterns. These visualizations collectively demonstrate the versatility of the system’s dynamics in response to changes in amplitude and frequency.
The effects of the parameter \(\tau _2\) on the dynamics of the proposed model is visualized, in order to observe the coexisting attractors. These attractor projections are evaluated in Fig .5 with the consideration of parameters as \(\tau _1=20,~\tau _2=10,~\tau _3=0.01,~\alpha _1=1,~\alpha _2=1,~\alpha _3=1,~ w_0=0.1,~\xi _1=1,~\xi _2=1\). In subplot 5a with \(\tau _2 = 10\), the phase portrait results in a dense and tightly wound structure around the origin. The system exhibits a highly periodic and regular behavior. The phase diagram shows a series of nested loops, indicating a stable and predictable system where the trajectories converge to a specific pattern over time. When \(\tau _2 = 4\) in subplot 5b, the phase portrait shows two distinct loops intersecting each other. This indicates a change in the system’s dynamics compared to \(\tau _2 = 10\). The system is less stable than in 5a, with more complex trajectories. The intersection suggests the presence of multiple attractors or a more complicated oscillatory behavior, where trajectories can switch between different states. For \(\tau _2 = -4\) in subplot 5c, the phase portrait becomes more intricate and less symmetric. The figure shows a structure that appears to be an elongated and spiraled loop, indicating a more chaotic system. The negative value of \(\tau _2\) introduces instability, causing trajectories to deviate more significantly and explore a larger area of the phase space. This suggests that the system is less predictable and more sensitive to initial conditions.
With \(\tau _2 = 10\) in subplot 5d, the phase portrait is again a complex structure but appears more irregular compared to 5a. The larger value of \(\tau _2\) leads to a denser and more intertwined set of trajectories, indicating a highly dynamic and possibly chaotic system. The trajectories cover a broader range in the phase space, showing that the system exhibits complex behavior with possible multiple frequencies and amplitudes.
High Positive \(\tau _2\) (subplots 5a and 5d) generally leads to denser, more regular structures with periodic behavior. As \(\tau _2\) increases further (as in 5d), the system can exhibit even more complex and possibly chaotic dynamics. Lower Positive \(\tau _2\) (subplot 5b) results in intersecting loops, indicating more complex oscillatory behavior with possible multiple attractors. Negative \(\tau _2\) (subplot 5c) introduces instability and chaotic behavior, causing trajectories to cover a larger area in the phase space and become less predictable. The parameter \(\tau _2\) significantly affects the stability, predictability, and complexity of the system’s dynamics, with higher absolute values leading to more complex and potentially chaotic behavior. The sensitivity of the proposed system towards initial values are presented in the Fig. 6 with the consideration of parameters as \(\tau _1=20,~\tau _2=10,~\tau _3=0.01,~\alpha _1=1,~\alpha _2=1,~\alpha _3=1,~ w_0=0.1,~\xi _1=0\). The Fig. 6, vividly depict the phase diagrams that explore how different initial values influence the system’s dynamics. Focusing on Fig. 6a, which showcases the behavior of \(\mathcal {W}_1\) over time, one can observe periodic oscillations. The trajectories, represented in blue, red, and green, correspond to initial values of (0.1, 0.1), (0.15, 0.15), and (0.2, 0.2) respectively. Interestingly, the blue trajectory, with the smallest initial values, has the large amplitude, whereas the green trajectory, with the largest initial values, shows the least amplitude. The red trajectory lies between these two extremes. Despite these amplitude differences, all trajectories maintain a consistent phase, oscillating around the zero value in synchrony. This phase consistency points to a stable periodic behavior in \(\mathcal {W}_1\), influenced by the initial conditions’ magnitude.
In Fig. 6b, illustrating the variable \(\mathcal {W}_2\) over the same time range, similar periodic oscillations are evident. The trajectories, again color-coded based on the same initial conditions as in Fig. 6a, show that \(\mathcal {W}_2\)’s amplitude also decreases with the initial values. The blue trajectory, corresponding to the smallest initial values, has the largest amplitude, while the green trajectory, with the largest initial values, has the least amplitude. The red trajectory is moderate in comparison. As with \(\mathcal {W}_1\), the phase of the oscillations in \(\mathcal {W}_2\) remains consistent, showing that without perturbation the given system has predictable dynamics.
These figures effectively illustrate how slight variations in initial conditions can significantly affect the system’s oscillation amplitude, while the periodic nature and phase consistency remain unaffected. This highlights the system’s sensitivity to initial conditions and underscores the importance of precise initial state settings in predicting the long-term behavior of dynamic systems. The visualizations successfully communicate the stable yet sensitive nature of the system’s response to initial conditions, providing valuable insights into the system’s behavior.
5.2 Characteristics of the proposed system
This part of the manuscript present the dynamical characteristics of the proposed system including Lyapunov exponents (LEs), return map, power spectrum, recurrence plot, fractal dimension and others. The phase diagrams in Fig. 7 offer a comprehensive view of the dynamics in the proposed chaotic system described by equations (5.1). Each subplot provides a unique insight into different aspects of the system’s behavior. Subplot 7a shows the LEs, \(\Gamma _1\) and \(\Gamma _2\) over time (t). These exponents measure how quickly nearby trajectories diverge or converge, which is crucial for determining chaos. Here, \(\Gamma _1\) is positive, indicating that small differences in initial conditions grow exponentially, confirming chaotic behavior. Conversely, \(\Gamma _2\) is negative, pointing to some contracting directions in phase space. The stability of these exponents over time suggests the system remains in a chaotic state, because the dominant LE is positive.
Subplot 7b is return map, presenting the dynamics \(\mathcal {W}_1(n)\) against \(\mathcal {W}_1(n+1)\). It helps to visualize the trajectory in a lower-dimensional space, making it easier to detect periodicities and chaos. The scattered points form a discernible pattern along the diagonal but do not create a simple line or closed curve, indicating the system’s underlying structure and chaotic behavior. Subplot 7c illustrates the power spectrum, highlighting the magnitudes of different frequency components in the system’s response. Peaks at specific frequencies show the dominant oscillation frequencies. Multiple peaks suggest a complex system with many excited frequencies, typical of chaotic systems that exhibit unpredictable and irregular dynamics. Subplot 7d: This is a recurrence plot, a tool for examining when the system’s state returns to a previous state. Both axes represent time (t), and plotted points show the recurrence of states. The complex pattern of points, including diagonal lines and clusters, indicates that while the system revisits similar states, it does so without regular periodicity, further supporting the presence of chaos. Together, these phase diagrams in Fig. 7 characterize the chaotic dynamics of the proposed system (5.1). The LEs confirm chaos through a positive value, the return map shows non-periodic yet structured behavior, the power spectrum reveals multiple frequency components, and the recurrence plot displays complex patterns without regular periodicity. These visualizations collectively demonstrate the system’s LEs, return map, power spectrum vs frequency and the recurrence behavior.
Analyzing a chaotic system can reveal the intricate dance between order and disorder that underpins such phenomena. Figure 8 shows an exploration of fractal dimensions, Poincaré section and strange attractor, which are very crucial in understanding chaos theory.
Subplot 8a showcases the fractal dimension through a box-counting method. The step like behavior observed is known as self similar behavior. In the chaotic systems, such structures are indicative of existence strange attractors having non-integer fractal dimensions. The fractal dimension serves as a measure of the attractor’s complexity; a higher fractal dimension corresponds to a more complex, less predictable system. The simulated figure indicates a fractal structure where the variations in counts at different scales are captured. This is a visual representation of how fractal dimensions describe the chaotic dynamics’ multi-scale nature. Subplot 8b offers a two-dimensional view of the Poincaré section in the \((W_1, W_2)\) plane. This scatter plot, with \(W_1\) and \(W_2\) as state variables, reveals a dense, intricate structure. The butterfly-like shape illustrates that the system’s trajectory doesn’t settle into a periodic orbit but instead explores a fractal structure in phase space. This complexity visually represents chaos, showing the system’s dynamic evolution within a bounded region without repeating itself.
Physical structure of Eq. (6.3), by using different parameter values
Physical structure of Eq. (6.7), by using different parameter values
Physical structure of Eq. (6.9), by using different parameter values
Physical structure of Eq. (6.11), by using different parameter values
Subplot 8c provides a three-dimensional perspective of the strange attractor, plotting the trajectory in the \((W_1(t), W_1(t + \text {delay}), W_1(t + 2 \times \text {delay}))\) space. The delay is considered as 8. This view gives deeper insights into the temporal evolution, displaying the interdependencies and delayed feedback within the chaotic dynamics. The attractor appears twisted and folded, reflecting the system’s long-term unpredictability and sensitivity to initial conditions. This complexity confirms the presence of deterministic chaos, where despite deterministic governing equations, outcomes appear random and highly sensitive to initial conditions.
Together, these figures illuminate the multi-faceted nature of chaos within the system. The fractal dimension is in simulated subplot 8a, while subplot 8b and subplot 8c graphically demonstrate the Poincaré section and strange attractor, respectively. These visualizations are essential for grasping the intricate dynamics and predicting long-term behavior, albeit with limitations due to sensitivity to initial conditions.
6 Application of IMETFA to governing model
To acquire multiple soliton solutions to governing model (1.2), using the IMETFA that is described in section (2). Insert Eq. (4.1), into Eq. (1.2), to acquire NLODEs given in Eq. (4.2). After integration acquire the required NLODEs given in Eq. (4.3). Now, according to Eq. (4.4), apply the homogeneous balance principle, we get \(M=2\) as below
where \(\mathcal {W}(\eta )=r'(\eta ),\) inserting the Eq. (6.1) and required derivative of Eq. (6.1) into Eq. (4.3), and collecting all the coefficients of the same of power of \(R(\eta )\) to get the system of equations. After solving the equations we obtain some solution sets. Here, we take one set as:
set-1:
When applying the above set-1 into Eqs. (2.4-2.14), we get the multiple soliton solutions,
Physical structure of Eq. (6.13), by using different parameter values
7 Simulations and physical discussions
The analytical results provide physical representations of the extracted solutions, which are displayed in Figs. 9, 10, 11, 12 and 13. 3D plots along with the projection of contour plots and 2D plots are used to illustrate the results that have been derived. These solutions and previous findings from different methodologies have been reviewed and contrasted in the literature. Equation (1.2) presented a distinct structure; it looks for more intriguing, widely applicable, and original soliton waveforms. Various types of solution are produced by Eq. (1.2). The proposed technique’s efficiency and specialization in solving NLIPDEs are made clear by comparing it with existing approaches. Fig. 9, illustrates the solution presented in Eq. (6.3) by using the values of a parameter \(\tau _1=0.2,~\tau _2=0.7,~\tau _3=0.9,~\alpha _2=0.3,~y=0,~z=0,~w_0=0.5,~\gamma _0=1.3.\) The solution of \(v_{1,1}\) of Eq. (6.3) represents the combo of hyperbolic, inverse trigonometric and solutions of dark soliton. Figure 10, illustrates the solution presented in Eq. (6.7) by using the values of a parameter \(\tau _1=0.2,~\tau _2=0.7,~\tau _3=0.9,~\alpha _2=0.3,~y=0,~z=0,~w_0=0.5,~\gamma _0=1.3.\) The solution of \(v_{1,5}\) of Eq. (6.7) represents the combo of hyperbolic, and logarithmic solitons. Figure 11, illustrates the solution presented in Eq. (6.9) by using the values of a parameter are \(\tau _1=0.2,~\tau _2=0.7,~\tau _3=0.9,~\alpha _2=0.3,~y=0,~z=0,~w_0=0.5,~\gamma _0=1.3.\) The solution of \(v_{1,7}\) of Eq. (6.9) highlights the combo of inverse dark kink type soliton. Figure 12, illustrates the solution presented in Eq. (6.11) by using the values of a parameter are \(\tau _1=0.2,~\tau _2=0.7,~\tau _3=0.9,~\alpha _2=0.3,~y=0,~z=0,~w_0=0.5,~\gamma _0=1.3.\) The solution of \(v_{1,9}\) of Eq. (6.11) gives the combo of singular and logarithmic dark soliton. Figure 13, illustrates the solution presented in Eq. (6.13) by using the values of a parameter \(\tau _1=0.2,~\tau _2=0.7,~\tau _3=0.9,~\alpha _2=-0.3,~y=0,~z=0,~w_0=0.5,~\gamma _0=1.3.\) The solution of \(v_{1,11}\) of Eq. (6.13) elucidates the combo of periodic and logarithmic periodic soliton.
8 Conclusion
This comprehensive study on the (3+1)-dimensional extended Kairat-II model has demonstrated the efficacy of Lie-Bäcklund symmetry and the extended modified Tanh-function approach in deriving various soliton solutions. Various dynamical features have been analyzed for considered model. The bifurcation and sensitivity analyses have revealed critical insights into the stability and chaotic nature of the model, highlighting its sensitivity to initial conditions and parameter variations. The detailed graphical visualizations, including phase diagrams, Poincaré sections, fractal dimension, power spectrum, recurrence plot, return map and strange attractors, have provided a clear understanding of the model’s dynamic properties. These results have underscored the model’s potential for addressing complex real-world problems and pave the way for future research in nonlinear dynamics and soliton theory. Further, the findings include multiple types of combo solitons, each exhibiting distinct physical behaviors which have not been reported previously in the literature. This study not only advances the mathematical techniques for solving NLPDEs but also enhances their applicability to integrable systems in various scientific and engineering domains.
Data availability
No data were used in this paper.
References
Gupta, R.K., Sharma, M.: An extension to direct method of Clarkson and Kruskal and Painlevé analysis for the system of variable coefficient nonlinear partial differential equations. Qual. Theory Dyn. Syst. 23(3), 115 (2024)
Wazwaz, Abdul-Majid.: A Hamiltonian equation produces a variety of Painlevé integrable equations: solutions of distinct physical structures. Int. J. Num. Methods Heat Fluid Flow 34(4), 1730–1751 (2024)
Ullah, N., Asjad, M.I., Hussanan, A., Akgül, A., Alharbi, Wedad R., Algarni, H., Yahia, I.S.: Novel wave structures for two nonlinear partial differential equations arising in the nonlinear optics via Sardar-subequation method. Alex. Eng. J. 71, 105–113 (2023)
Saifullah, S., Ahmad, S., Khan, M.A., ur Rahman, M.: Multiple solitons with fission and multi waves interaction solutions of a (3+ 1)-dimensional combined pKP-BKP integrable equation. Phys. Scripta 99(6), 065242 (2024)
Wazwaz, A.M., Alhejaili, W., El-Tantawy, S.A.: Analytical study on two new (3+ 1)-dimensional Painlevé integrable equations: kink, lump, and multiple soliton solutions in fluid mediums. Phys. Fluids 35(9), 093119 (2023)
Ali, Asghar, Ahmad, Jamshad, Javed, Sara: Exploring the dynamic nature of soliton solutions to the fractional coupled nonlinear Schrödinger model with their sensitivity analysis. Opt. Quant. Electron. 55(9), 810 (2023)
Javed, Sara, Ali, Asghar, Muhammad, Taseer: Dynamical perspective of bifurcation analysis and soliton solutions to (1+ 1)-dimensional nonlinear perturbed Schrödinger model. Opt. Quant. Electron. 56(6), 1013 (2024)
Javed, Sara, Ali, Asghar, Ahmad, Jamshad, Hussain, Rashida: Study the dynamic behavior of bifurcation, chaos, time series analysis and soliton solutions to a Hirota model. Opt. Quant. Electron. 55(12), 1114 (2023)
Wang, Yue-Yue., Zhang, Yu-Peng., Dai, Chao-Qing.: Re-study on localized structures based on variable separation solutions from the modified tanh-function method. Nonlinear Dyn. 83(3), 1331–1339 (2016)
Gu, Y., Zia, S.M., Isam, M., Manafian, J., Hajar, A., Abotaleb, M.: Bilinear method and semi-inverse variational principle approach to the generalized (2+ 1)-dimensional shallow water wave equation. Res. Phys. 45, 106213 (2023)
Kumar, Sachin, Niwas, Monika: Optical soliton solutions and dynamical behaviours of Kudryashov’s equation employing efficient integrating approach. Pramana 97(3), 98 (2023)
Wazwaz, Abdul-Majid., Gui-Qiong, Xu.: Variety of optical solitons for perturbed Fokas-Lenells equation through modified exponential rational function method and other distinct schemes. Optik 287, 171011 (2023)
Zhu, C., Al-Dossari, M., Rezapour, S., Gunay, B.: On the exact soliton solutions and different wave structures to the (2+ 1) dimensional Chaffee-Infante equation. Res. Phys. 57, 107431 (2024)
Zhu, C., Al-Dossari, M., Rezapour, S., Shateyi, S.: On the exact soliton solutions and different wave structures to the modified Schrödinger’s equation. Res. Phys. 54, 107037 (2023)
Kai, Yue, Yin, Zhixiang: Linear structure and soliton molecules of Sharma-Tasso-Olver-Burgers equation. Phys. Lett. A 452, 128430 (2022)
Zhu, C., Al-Dossari, M., Rezapour, S., Shateyi, S., Gunay, B.: Analytical optical solutions to the nonlinear Zakharov system via logarithmic transformation. Res. Phys. 56, 107298 (2024)
Parasuraman, E.: Stability of kink, anti kink and dark soliton solution of nonlocal Kundu Eckhaus equation. Optik 290, 171279 (2023)
Ali, A., Ahmad, J., Javed, S., Hussain, R., Alaoui, M.K.: Numerical simulation and investigation of soliton solutions and chaotic behavior to a stochastic nonlinear Schrödinger model with a random potential. Plos one 19(1), e0296678 (2024)
Kai, Yue, Ji, Jialiang, Yin, Zhixiang: Study of the generalization of regularized long-wave equation. Nonlinear Dyn. 107(3), 2745–2752 (2022)
Zhu, C., Al-Dossari, M., Rezapour, S., Alsallami, S.A.M., Gunay, B.: Bifurcations, chaotic behavior, and optical solutions for the complex Ginzburg-Landau equation. Res. Phys. 59, 107601 (2024)
Chahlaoui, Younes, Ali, Asghar, Javed, Sara: Study the behavior of soliton solution, modulation instability and sensitive analysis to fractional nonlinear Schrödinger model with Kerr Law nonlinearity. Ain Shams Eng. J. 15(3), 102567 (2024)
Hameedullah, Rafiullah, Saifullah, S., Ahmad, S., Rahman, M.U.: Stability, modulation instability analysis and new travelling wave solutions of non-dissipative double-dispersive microstrain wave model within micro-structured solids. Opt. Quant. Electron. 56(2), 223 (2024)
Bagheri, Majid, Khani, Ali: Analytical method for solving the factional order generalized KdV equation by a beta-fractional derivative. Adv. Math. Phys. 2020(1), 8819183 (2020)
Khater, M.M.: Characterizing shallow water waves in channels with variable width and depth; computational and numerical simulations. Chaos Solitons Fract. 173, 113652 (2023)
Ahmad, Shabir, Saifullah, Sayed: Analysis of the seventh-order Caputo fractional KdV equation: applications to the Sawada-Kotera-Ito and Lax equations. Commun. Theor. Phys. 75(8), 085002 (2023)
Refaie Ali, A., Alam, M.N., Parven, M.W.: Unveiling optical soliton solutions and bifurcation analysis in the space-time fractional Fokas-Lenells equation via SSE approach. Sci. Rep. 14(1), 2000 (2024)
Zhou, C., He, X.T., Chen, S.: Basic dynamic properties of the high-order nonlinear Schrödinger equation. Phys. Rev. A 46(5), 2277 (1992)
Alazman, I., Alkahtani, B.S., ur Rahman, M., Mishra, M.N.: Nonlinear complex dynamical analysis and solitary waves for the (3+ 1)-D nonlinear extended quantum Zakharov-Kuznetsov equation. Res. Phys. 58, 107432 (2024)
Ismael, H.F.: Bifurcation and chaotic behaviors to the Sasa-Satsuma and higher-order Sasa-Satsuma equations in fluid dynamics and nonlinear optics. Opt. Quant. Electron. 55(14), 1271 (2023)
Awadalla, Muath, Zafar, Asim, Taishiyeva, Aigul, Raheel, Muhammad, Myrzakulov, Ratbay, Bekir, Ahmet: The analytical solutions to the M-fractional Kairat-II and Kairat-X equations. Front. Phys. 11, 1335423 (2023)
Awadalla, Muath, Zafar, Asim, Taishiyeva, Aigul, Raheel, Muhammad, Myrzakulov, Ratbay, Bekir, Ahmet: The analytical solutions to the M-fractional Kairat-II and Kairat-X equations. Front. Phys. 11, 1335423 (2023)
Iqbal, Mujahid, Dianchen, Lu., Alammari, Maha, Seadawy, Aly R., Alsubaie, Nahaa E., Umurzakhova, Zhanar, Myrzakulov, Ratbay: A construction of novel soliton solutions to the nonlinear fractional Kairat-II equation through computational simulation. Opt. Quant. Electron. 56(5), 845 (2024)
Iqbal, M., Lu, D., Seadawy, A.R., Alomari, F.A., Umurzakhova, Z., Myrzakulov, R.: Constructing the soliton wave structure to the nonlinear fractional Kairat-X dynamical equation under computational approach. Mod. Phys. Lett. B 18, 2450396 (2024)
Myrzakulova, Zh., Manukure, S., Myrzakulov, R., Nugmanova, G.: Integrability, geometry and wave solutions of some Kairat equations. arXiv preprint arXiv:2307.00027 (2023)
Wazwaz, Abdul-Majid.: Extended (3+ 1)-dimensional Kairat-II and Kairat-X equations: Painlevé integrability, multiple soliton solutions, lump solutions, and breather wave solutions. Int. J. Num. Methods Heat Fluid Flow 34(5), 2177–2194 (2024)
Hussein, Hisham H., Ahmed, Hamdy M., Alexan, Wassim: Analytical soliton solutions for cubic-quartic perturbations of the Lakshmanan-Porsezian-Daniel equation using the modified extended tanh function method. Ain Shams Eng. J. 15(3), 102513 (2024)
Khan, A., Saifullah, S., Ahmad, S., Khan, M.A., Rahman, M.U.: Dynamical properties and new optical soliton solutions of a generalized nonlinear Schrödinger equation. Eur. Phys. J. Plus 138(11), 1059 (2023)
Acknowledgements
The authors extend their appreciation to the Deanship of Research and Graduate Studies at King Khalid University for funding this work through Large Research Project under grant number RGP2/308/45.
Funding
Not applicable.
Author information
Authors and Affiliations
Corresponding author
Additional information
Publisher's Note
Springer Nature remains neutral with regard to jurisdictional claims in published maps and institutional affiliations.
Rights and permissions
Springer Nature or its licensor (e.g. a society or other partner) holds exclusive rights to this article under a publishing agreement with the author(s) or other rightsholder(s); author self-archiving of the accepted manuscript version of this article is solely governed by the terms of such publishing agreement and applicable law.
Open Access This article is licensed under a Creative Commons Attribution 4.0 International License, which permits use, sharing, adaptation, distribution and reproduction in any medium or format, as long as you give appropriate credit to the original author(s) and the source, provide a link to the Creative Commons licence, and indicate if changes were made. The images or other third party material in this article are included in the article’s Creative Commons licence, unless indicated otherwise in a credit line to the material. If material is not included in the article’s Creative Commons licence and your intended use is not permitted by statutory regulation or exceeds the permitted use, you will need to obtain permission directly from the copyright holder. To view a copy of this licence, visit http://creativecommons.org/licenses/by/4.0/.
About this article
Cite this article
Almheidat, M., Alqudah, M., Alderremy, A.A. et al. Lie-bäcklund symmetry, soliton solutions, chaotic structure and its characteristics of the extended (3 + 1) dimensional Kairat-II model. Nonlinear Dyn (2024). https://doi.org/10.1007/s11071-024-10325-3
Received:
Accepted:
Published:
DOI: https://doi.org/10.1007/s11071-024-10325-3