Abstract
As society experiences accelerated ageing, understanding the complex biological processes of human ageing, which are affected by a large number of variables and factors, becomes increasingly crucial. Artificial intelligence (AI) presents a promising avenue for ageing research, offering the ability to detect patterns, make accurate predictions, and extract valuable insights from large volumes of complex, heterogeneous data. As ageing research increasingly leverages AI techniques, we present a timely systematic literature review to explore the current state-of-the-art in this field following a rigorous and transparent review methodology. As a result, a total of 77 articles have been identified, summarised, and categorised based on their characteristics. AI techniques, such as machine learning and deep learning, have been extensively used to analyse diverse datasets, comprising imaging, genetic, behavioural, and contextual data. Findings showcase the potential of AI in predicting age-related outcomes, developing ageing biomarkers, and determining factors associated with healthy ageing. However, challenges related to data quality, interpretability of AI models, and privacy and ethical considerations have also been identified. Despite the advancements, novel approaches suggest that there is still room for improvement to provide personalised AI-driven healthcare services and promote active ageing initiatives with the ultimate goal of enhancing the quality of life and well-being of older adults.
Graphical abstract
Overview of the literature review.
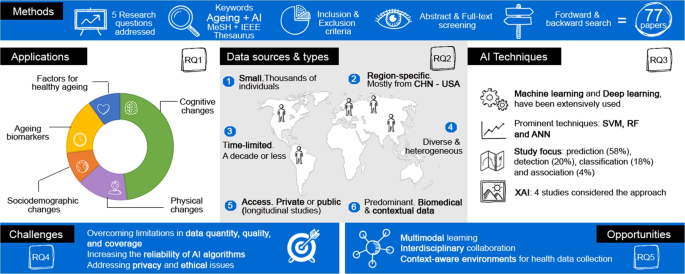
Explore related subjects
Discover the latest articles, news and stories from top researchers in related subjects.Avoid common mistakes on your manuscript.
1 Introduction
Recent health and socio-economic developments are leading to an ageing society, characterised by the growth of the world’s population and the increase in average life expectancy. Consequently, the number of persons aged 65 or over is estimated to double by 2050, reaching 1.6 billion globally [1]. This demographic shift is unsurprisingly forcing global institutions to prioritise initiatives promoting active ageing, such as the Sustainable Development Goals [2] and the Decade of Healthy Ageing (2021-2030) [3]. These initiatives aim to establish frameworks towards healthy lifestyles and overall well-being among the elderly cohort.
Understanding ageing is far from straightforward due to its multidisciplinary nature, encompassing changes in various aspects of human life, including physical, metabolic, psychological, social, emotional, and functional. Recently, the World Health Organisation proposed an innovative model aimed at reshaping clinical practices in elderly healthcare, shifting the focus from a disease-centred approach to one centred around function [4]. Within this framework, the concept of functional ability [3] emerges to refer to people’s abilities (physical, cognitive, social...) to perform their daily living activities according to their basic needs. The functional ability of an individual is determined by three main factors: (i) their intrinsic capacity (i.e., physical and mental capacities, namely locomotor, sensory, vitality, cognition, and psychological), (ii) the environment in which they live (i.e., context), and (iii) how they interact with that environment. This approach offers a new perspective in ageing focusing not on disease but on a holistic understanding that embraces the positive aspects of ageing.
As the ageing of society is an unavoidable reality, healthcare providers must prepare to meet the increasing demand for clinical and public health services, especially in primary care and long-term care [5]. Current healthcare paradigms, such as smart health [6], leveraging technological advances to deliver patient-centred care services tailored to the needs of older adults [7,8,9,10]. Notably, the potential of these paradigms is expected to grow exponentially with the development of sensors at nanoscopic scales and the deployment of ultra-fast data networks with extremely low latency [11, 12]. This advancement enables the collection, transmission, and analysis of vast amounts of heterogeneous data, paving the way for exciting health opportunities, including gaining a comprehensive understanding of the ageing processes, ranging from personalised insights at an intra-body level to broader, cross-national perspectives.
The increasing demand for data-driven solutions in healthcare has propelled the widespread adoption of artificial intelligence (AI) [13], which encompasses a broad range of capabilities from sensory perception to autonomous decision-making. AI systems outperform humans in identifying relevant patterns within large sets of complex, non-linear data through machine learning (ML) and deep learning (DL) [14, 15]. ML, a subset of AI, is the most common method for implementing AI, automating data analysis using algorithms that iteratively recognise patterns and learn from them. In turn, DL is a subset of ML, employing deep neural networks that operate on an unsupervised ML approach, which does not require labelled data. Studies on human ageing, which are evidence-based, require extensive datasets, preferably longitudinal studies that are more likely to capture declines in intrinsic capacity domains [16]. Hence, contemporary research on ageing leverages advanced AI techniques to develop sophisticated models capable of understanding and predicting ageing processes, assessing factors contributing to age-related diseases, developing biomarkers, and discovering potential anti-ageing treatments, among others. Notwithstanding, AI is not a silver bullet solution. AI-generated models may exhibit biases if trained on inaccurate, incomplete, or discriminatory datasets. Since data on elderly population is paramount in research on ageing, these issues could be exacerbated by digital ageism, and potentially resulting in ineffective AI models [17]. All in all, there is no doubt that future developments of AI will unleash a new wave of technological research and innovation in the field of ageing research.
As the fields of AI and human ageing are getting increasingly intertwined, stakeholders and key players interested in cutting-edge applications in this arena require a timely systematic literature review of the existing research. In contrast to existing reviews, which do not follow a systematic methodology or are domain-specific, our study provides a comprehensive understanding of the role of AI in ageing research by offering an overview of the current landscape of AI-enabled applications, as well as the most prominent datasets and algorithms used. Through a content analysis approach, we highlight the growing interest from the academic community and identify three key research streams: (i) classification and summary of AI-based applications, techniques, and datasets across ageing research, (ii) evaluation of the suitability of AI technology to create value in this field while considering its inherent limitations, and (iii) guidance for researchers and stakeholders by outlining promising research directions, challenges, and opportunities that require further research.
The remainder of the article is organised as follows. Section 2 outlines the methodology followed to conduct the systematic review. The descriptive analysis of the retrieved literature is presented in Section 3. Then, Section 4 provides an in-depth discussion on the advancement of AI in ageing research, focusing on key challenges and sketching research opportunities that are expected to be relevant in the years ahead. Finally, Section 5 closes the article with our conclusions.
2 Methods
To provide a rigorous and transparent review, we have adopted the well-known methodology for systematic reviews suggested by Brocke et al. [18] along with adhering to the guidelines of the PRISMA statement [19]. Specifically, our review methodology encompasses the following phases: (i) the definition of the review scope, (ii) the contextualisation of the topic, (iii) the literature search, (iv) the literature analysis and synthesis, and (v) the definition of a research agenda.
2.1 Definition of the review scope
The scope of this review is to provide a timely analysis of the current state-of-the-art about the role of AI in the study of human ageing. To this end, we examine and classify the main goals, techniques, and applications proposed by the scientific community. For the sake of completeness, to align the systematic review to specific objectives, five research questions (RQs) have been defined (see Table 1).
The target audience of this review is multi-disciplinary because it is intended for researchers, practitioners, and stakeholders in the fields of AI, human ageing, longevity, and biotechnology. To smoothly guide the reader through the topic, the review is organised conceptually by grouping similar ideas from different authors, and the articles from the literature are examined from a neutral but critical viewpoint.
2.2 Conceptualisation of the topic
This review focuses on two main topics: AI and ageing. Both terms are conceptually broad, so it is likely that they are often referenced using a variety of synonyms and related words. For the sake of completeness, additional relevant terms have been included in the search string.
Related terms associated with “artificial intelligence” were identified using the IEEE Thesaurus [20], which categorises concepts in engineering and computer science. This tool offers related terminology and vocabulary from high-quality scientific sources, including journal articles and standards. Thus, it is considered a suitable tool for obtaining key terms relevant to this subject of study. Numerous terms were retrieved and organised based on their semantic relationships:
-
As broader terms, the following results were obtained: Computational and artificial intelligence.
-
As related terms, the following results were obtained: Autonomous automobiles; Autonomous vehicles; Computational Intelligence; Data Mining; Feedforward Neural Networks; Generative Adversarial Networks; Independent Component Analysis; Machine Ethics; Minimax techniques; Natural languages; Neural Networks; Neurocontrollers; Pervasive computing; Posthuman; Prediction theory; Radial Basis Function networks; Robot learning; Semantic Web; Software agents; Support Vector Machines; Synapses.
-
As narrower terms, the following results were obtained: Artificial intelligence accelerators; Affective computing; Autonomous robots; Bio-inspired computing; Cognitive systems; Context awareness; Cooperative systems; Decision support systems; Intelligent systems; Knowledge based systems; Knowledge engineering; Learning (artificial intelligence); Learning systems; Machine learning; Prediction methods; Virtual artifact.
Related terms associated with “ageing” were identified using the MeSH Thesaurus [21], a comprehensive and controlled vocabulary related to the life sciences curated by the United States National Library of Medicine. This resources indexes and catalogues biomedical and health-related information. As a result, the following terms were obtained: Aging; Senescence; Biological aging; Aging, biological.
2.3 Literature search
To guarantee the coverage of high-quality scientific literature in this review, we selected the Web of Science database for retrieving publications. The search string was built by combining the two aforementioned terms sets: one containing the list of words related to “artificial intelligence”, and the other one containing the list of words related to “ageing”. Moreover, to obtain the broadest possible coverage on the topic, no timeframe restriction was applied, and the search string was configured to seek across all the article (i.e., title, abstract, keywords, full-text, and metadata). Hence, the resulting search string used to retrieve the literature was as follows:
ALL ((“Artificial Intelligence” OR “Computational and artificial intelligence” OR “Autonomous automobiles” OR “Autonomous vehicles” OR “Computational intelligence” OR “Data Mining” OR “Feedforward neural networks” OR “Generative Adversarial Networks” OR “Independent Component Analysis” OR “Machine Ethics” OR “Minimax techniques” OR “Natural languages” OR “Neural Networks” OR “Neurocontrollers” OR “Pervasive computing” OR “Posthuman” OR “Prediction theory” OR “Radial Basis Function networks” OR “Robot learning” OR “Semantic Web” OR “Software agents” OR “Support Vector Machines” OR “Synapses” OR “Artificial intelligence accelerators” OR “Affective computing” OR “Autonomous robots” OR “Bio-inspired computing” OR “Cognitive systems” OR “Context awareness” OR “Cooperative systems” OR “Decision support systems” OR “Intelligent systems” OR “Knowledge based systems” OR “Knowledge engineering” OR “Learning and Artificial Intelligence” OR “Learning systems” OR “Machine learning” OR “Prediction methods” OR “Virtual artifact”) AND (“ageing” OR “aging” OR “senescence” OR “biological aging” OR “ageing, biological”))
The eligibility of the retrieved literature was evaluated independently by the authors based on a set of predefined inclusion and exclusion criteria, described as follows:
-
The publication was a peer-reviewed research article.
-
The publication was written in English.
-
The full-text of the publication was available.
-
The publication was published in a Q1 ISI-JCR journal, or the number of citation of the publication was above the 75th percentile regarding the citations of other retrieved publications of the same year.
-
The publication provided explicit evidence of the use of AI in the study of human ageing. Thus, publications using AI to study age-related diseases were excluded if they did not specifically address research on human ageing.
-
Thus publication explicitly specify the AI technique used, i.e., stating that it uses AI was not sufficient.
-
The publication was focused on older adults and the elderly to provide a more comprehensive understanding of the ageing experience within these cohorts.
The selection process consisted of two screening phases: abstract screening and full-text screening. Each publication was independently evaluated by two reviewers according to the inclusion criteria. During each phase, each reviewer categorised the publications as either accepted or rejected. Any discrepancies were discussed among the reviewers until a consensus was reached, thus minimising potential reviewer bias. After this initial search, the first selection of publications was accepted for inclusion in this review.
From the initial set of selected publications, a reference-checking phase was conducted to discover additional studies. Concretely, two new searches were conducted: (i) a backward search (i.e., reviewing older literature cited in the selected publications) and (ii) a forward search (i.e., reviewing literature that have cited the selected publications). Each search yielded a new set of publications, which were assessed for eligibility again following the same procedure as in the first selection. This iterative approach ensures a more thorough literature search and adds robustness to this systematic literature review. As a result, a new set of publications was accepted for inclusion in this review as well.
2.4 Literature analysis and synthesis
After selecting the publications to be included in this review, accepted publications were rigorously analysed to extract, characterise, and classify the most relevant information. Then, a conceptual synthesis was adopted to identify common topics and characteristics in the publications. For this reason, the results are discussed from multiple angles, such as the ageing-related application, the kind of data and ageing studies needed, and the AI techniques that have been employed.
2.5 Definition of a research agenda
Beyond providing an exhaustive review of the current state-of-the-art in the field, this review helps set the ground for further research on this topic. Hence, Section 4.3 summarises potential research directions based on our main findings.
3 Results
A total of 11,586 publications were retrieved from the first search, queried on 30th December 2023. Several publications were excluded at first as they did not fulfil some inclusion criteria. Then, 5,823 publications were assessed for eligibility in the abstract screening phase. After that, 5,763 were excluded, mainly because they were not related to human ageing. Hence, the full-text of 369 publications were screened and assessed for eligibility. After full-text screening, 300 were rejected, and 69 publications were accepted to be included in this review. From those 69 accepted publications in the first search, the reference-checking phase, conducted on 29th January 2024, yielded 3,639 articles. After removing duplicated records, already-screened records, and applying objective inclusion criteria, 2,493 publications remained. After abstract screening, 2,376 publications were excluded, and 117 were assessed for eligibility. From those, 8 publications met all the inclusion criteria and were accepted to be included in the review. Therefore, a total of 77 publications are considered in this review. Details of the review methodology are depicted in Fig. 1.
A descriptive analysis of the 77 articles reveals a growing interest of the research community in incorporating AI into ageing studies. While first studies date back to the mid-2000s, when AI was gaining attention, over half of the selected articles (55/77) have been published within the past five years. This trend demonstrates the need for a timely systematic review in this field. Moreover, the majority of these articles have been published in journals within the health and life sciences domains, where Frontiers in Aging Neuroscience, NeuroImage, Human Brain Mapping, and GeroScience stand out.
All 77 articles were thoroughly analysed to extract their main characteristics in terms of scope, used data, and implemented AI techniques (refer to Table 2 for details). The gathered information was used to conceptualise and establish robust classification taxonomies that align with the RQs of this review.
3.1 Taxonomy of AI-based ageing applications
Most authors present AI opportunities within specific application domains, such as frailty [57] and biomarkers [98]. In our study, we provide a generic application-oriented classification similar to others proposed in similar research [36, 97]. Our approach, however, differs in its utilisation of a rigorous review methodology, thus providing a more suitable framework to illustrate current AI developments and future trends in ageing research. Consequently, considering the actual and forthcoming diversity of AI-driven solutions, we present a more comprehensive and in-depth classification of AI-enabled applications for ageing (RQ1).
As outlined in Table 2, articles have been classified into six main application domains: (i) age-related cognitive changes (34 articles), (ii) age-related physical changes (11 articles), (iii) age-related sociodemographic changes (7 articles), (iv) ageing biomarkers (12 articles), (v) factors for healthy ageing (7 articles), and (vi) review articles (6 articles).
Figure 2 illustrates the evolution of the number of articles per application domain. We observe that earlier studies leveraged AI to understand ageing processes according to the individuals’ physical and cognitive changes. Since the mid-2010s, the exploitation of biomarkers has been used to predict the biological age of individuals. This growing interest may be due to the incursion of DL techniques to develop age predictors that offer new possibilities for formerly incompatible data types. Likewise, it is worth noting that, in the last three years, there has been a growing interest in identifying sociodemographic changes and factors for healthy ageing.
3.1.1 Age-related cognitive changes
AI has been extensively employed to explore and analyse the anatomical and functional properties of the brain, with the aim of comprehending the mechanisms underlying age-related cognitive changes. Early studies (2010–2014) laid the foundation for understanding how alterations in brain anatomy affect cognitive function. Typically, anatomical neuroimaging techniques have been applied to identify structural brain regions affected by ageing. During the latter half of the 2010s, research focused on comprehensively analysing the functional connectivity between the brain and age-related changes, as well as detecting and predicting cognitive impairments such as dementia, Alzheimer’s disease, and Parkinson’s disease. As research progressed towards predicting cognitive decline, this structural knowledge was used to identify risk factors influencing deterioration. The ability of AI methods to detect subtle and non-linear patterns in cognitive data facilitates the modelling of complex relationships between such cognitive variables and potentially influencing factors, such as genetics, habits, and environment. Recently, the integration of advanced structural data with predictive modelling techniques using multi-modal and multi-dimensional analyses has led to a more holistic and precise understanding of how brain changes predetermine the risk of cognitive decline.
As ageing affects brain regions differently, understanding structural changes provides valuable insights for the development of diagnostic tools. Initial studies identified group-wise differences in the whole-brain network of macroscopic neural tracts across populations with different age distributions [75]. Also, by means of electroencephalography (EEG), Vysata et al. [87] conducted a retrospective analysis of healthy professional drivers and found a decrease in EEG coherence with age, particularly in the theta and alpha bands associated with decreased cortical connectivity. These studies allowed detailed exploration of the functional connectivity. In [45], authors investigated the effects of common cardiovascular risk factors on grey matter networks within the neocortex. Negative associations of smoking, higher blood pressure, elevated glucose levels, and visceral obesity with structural covariance networks were discovered, suggesting that reducing these factors could potentially delay late-life trajectories of grey matter ageing. The study in Salami et al. [77] revealed a functional connectivity decline within the anterior medial temporal lobe after age 60, and an elevated functional connectivity in the posterior medial temporal lobe that correlates with episodic-memory decline. Likewise, Bidelman et al. [26] focused on speech comprehension in older adults with and without hearing loss, demonstrating altered brain communication patterns in hearing-impaired individuals and highlighting the impact of even mild hearing loss on neural signalling and information processing pathways in the left hemisphere. The work in Schilling et al. [79], microstructural and macrostructural geometrical features of the brain’s connections were quantified by examining brain white matter. Their large-scale study indicated that macrostructural features were more sensitive to heterogeneous white matter decline, potentially making them more useful for studying ageing and enabling comparisons among various diseases and abnormal conditions. Using a different approach, Yamada et al. [94] evaluated changes in brain and cerebrospinal fluid volume with healthy ageing. Results showed that intracranial cerebrospinal fluid volume increased linearly because of the decreased brain volume with ageing, which could help elucidate the pathogenesis of chronic hydrocephalus in adults. Further research on brain ageing patterns, aiming to model the evolution of structural and functional brain changes, has also been conducted by Di et al. [34] and Sone et al. [81].
Regarding brain functioning, several studies shed light on the relationship between brain ageing, cognitive functions, and motor skills. For instance, brain-predicted age difference scores (i.e., the difference between an individual’s brain-predicted age using neuroimaging data and an individual’s chronological age) are used to detect accelerated ageing, often associated with increased mortality risk and poorer physical function. In [27], authors assessed the correlation between these scores and specific cognitive functions, revealing negative correlations between these scores and performance across various cognitive domains, including visual attention, processing speed, and semantic verbal fluency. Thus, the authors demonstrated correlations between increased brain-predicted age differences and reduced cognitive function in domains that are implicated in cognitive ageing. Indeed, substantial differences in linking brain-predicted age differences to cognitive measures may appear depending on the ML algorithm chosen [56]. In this line, Xiong et al. [92] evaluated the performance of different ML algorithms and image modalities for brain age prediction. Authors highlighted that image modality selection is more important than ML algorithm selection in improving the accuracy of brain age prediction. This finding has significant implications for future neuroimaging research endeavours. By means of structural covariance networks, the study in Koini et al. [48] examined the integrity of grey matter networks with age, cognitive performance, and fine motor skills, revealing positive associations between network disintegration and higher-order cognitive functions.
To understand the functional basis of cognitive changes in ageing, researchers have explored default mode (sub-)networks and recognised changes in resting state networks. Lindbergh et al. [59] observed that older adults with high intelligence maintained a more segregated default mode network profile, while Huang et al. [44] suggested that age-related network changes are pivotal in understanding cognitive ageing trajectories and, hence, offer valuable diagnostic tools for neurodegenerative diseases. Similarly, the work in Moezzi et al. [63] aimed to distinguish functional brain networks in young and older adults, revealing reduced connectivity in certain frequency bands associated with attention and consciousness during the resting state in older individuals. The use of resting state data is not new: previous studies already employed it to compare age-related differences in major functional brain networks [86], examine age-related perturbations in various resting state networks [68], and reorganise functional brain networks as predictors of age and cognitive performance [53, 61]. Additionally, the distinctiveness of different neural representations to explain individual differences in cognitive performance among older adults was already evaluated by Park et al. [70], which suggested that neural specificity declines are correlated with cognitive decline.
Beyond ageing, accelerated cognitive deterioration has proven correlated to a number of factors according to recent studies. For instance, Ganguli et al. [40] investigated the role of obesity, diabetes, and other metabolic indices on cognitive decline among older adults. Their findings suggested that obesity and even modest degrees of hyperglycemia could independently predispose individuals to faster cognitive decline. Pereira et al. [71] found associations between cognitive decline and spatial patterns of tau decomposition. In [29], authors highlighted the predominant influence of modifiable factors, such as education and cardiovascular health, over genetic risks, in predicting Alzheimer’s disease. Sun et al. [82] observed correlations between lower pulse pressure, significant stenosis in the posterior circulatory artery, and the risk of cognitive decline. Similarly, the studies in Kimura et al. [47], Noh et al. [66] and Wang et al. [88] identified physical activity, adequate sleep duration, and social engagement as crucial factors for guaranteeing proper cognitive function in older adults. Likewise, Sone et al. [80] has shown that higher life satisfaction is associated with “younger” brains, while diabetes and alcohol consumption negatively impact life satisfaction. However, the effects of life satisfaction on cognitive function may vary considerably among individuals [49], necessitating further research to uncover the mechanisms underlying the heterogeneous effects of life satisfaction on cognitive function.
More recently, innovative technological tools have been developed to early detect cognitive decline in large population cohorts. Yamada et al. [93] provided empirical evidence of the capability of automated cognitive assessment through drawing processes by measuring and analysing several drawing-related features such as speed, speed variability, pressure variability, and pen inclination. Moreover, with the advent of context-aware environments, plenty of opportunities arise to leverage the vast amount of data collected from sensors and other IoT devices. For instance, Khodabandehloo et al. [46] proposed continuous remote monitoring of elderly people to support early detection of cognitive decline by using data collected within smart homes. The data-driven nature of this explainable AI solution enables clinicians to make more accurate diagnoses. Furthermore, the works in Na [65] and Tan et al. [83] demonstrated the ability of current AI techniques to predict cognitive impairment using easy-to-collect and non-invasive data available at community healthcare centres and nursing homes.
3.1.2 Age-related physical changes
Age-related physical changes refer to the transformations that occur within an individual’s body as they age. These transformations, generally manifesting as age-related syndromes or conditions, may be accelerated according to the individual’s quality of life and the degree of active ageing. The use of AI can help understand these transformations and pinpoint the most relevant factors to delay them.
Initial studies used ML techniques to evaluate gait decline, one of the most popular physical impairments among older adults. The work in Begg and Kamruzzaman [25] identified different gait patterns between younger individuals and older adults through the analysis of basic temporal/spatial, kinetic, and kinematic gait data. Walking differences in terms of gait velocity were later examined in Yuan et al. [96] using resting state functional MRI data. Indeed, the authors in Hicks et al. [43] observed that one-off measurements of absolute strength and muscle power can help predict mobility and gait decline. Similarly, the relationship between lower extremity strength and mobility appears to be influenced by accelerated brain ageing [85]. Physical changes have also been explored through indirect calorimetry methodologies, as evidenced by Paraschiakos et al. [69], who quantified the physical activity energy expenditure of older people using accelerometer data and physiological indicators.
Frailty, a syndrome characterised by impairments to multiple interrelated physiological systems, has been extensively explored by recent research. In [33], ML models were used to predict individuals at risk of developing frailty in middle-aged and older adults. Interestingly enough, authors provided insights to overcome the encountered imbalance data problem: far fewer individuals are classified as frail than as non-frail, which affects the performance of ML models once predicting the syndrome. By combining undersampling and oversampling techniques, age, chair-rise test, household wealth, balance problems, and self-rated health were the most important frailty predictors. Similarly, Sajeev et al. [76] identified higher body mass index and distress levels, lower dexterity, balance problems, incontinence, and poor sleep quality as key predictors of pre-frailty in adults. Beyond prediction, research interest has extended to strategies for frailty reversal. Wei et al. [89] demonstrated the feasibility of predicting the likelihood of older adults successfully reversing their frailty status after participating in prevention programs.
Sarcopenia, an ageing-associated disorder characterised by a decline in muscle mass, strength, and function, significantly increases the risk of falls, fractures, and physical disability. In this context, Kwon et al. [52] clustered individuals based on nutritional and health-related factors, aiming to identify the main risk factors for sarcopenia. Results indicated that low total energy intake, inadequate levels of physical activity, a high prevalence of chronic diseases, and bad habits (e.g., smoking and high alcohol consumption) emerged as predominant risk factors across all groups. Regarding physical activity, the work by Bae et al. [24] developed a DL model for predicting the decline in physical fitness due to sarcopenia in individuals with potential sarcopenic symptoms. Results showed that the waist circumference, body fat percentage, and performance on the timed up-and-go and sit-and-reach tests were crucial factors for predicting possible sarcopenia. Sarcopenia can be diagnosed through medical images, but outlining irregular contours of abdominal body parts is highly laborious and time-consuming. To solve this, Gu et al. [41] designed a DL-based method to automate the segmentation and quantification of body parts from CT scans, achieving notable accuracy in predicting sarcopenia.
3.1.3 Age-related sociodemographic changes
Populations and societies as a whole play significant roles in shaping ageing processes. Contextual changes, such as social well-being, economic conditions, demographics, and quality of life and care can indirectly affect individuals’ ageing trajectories. The importance of social relations and economic aspects in human longevity was early reported in Ribeiro and Zárate [74]. This corroborated the idea that the environment is a complex structure and that every part of it influences how fast biological ageing happens, even on the prevalence and development of depressive symptoms in old age [91]. From a longitudinal study in the western Pacific region, which combined both ageing and low- and middle-income countries, Molassiotis et al. [64] identified sociodemographic and socioeconomic developments as more important factors to the disease burden among older adults than the health expenditure, whereas non-communicable diseases contributed the most to shorten life expectancy. By clustering similar countries, the authors encouraged regional governments and policymakers to prioritise healthy ageing through coordinated strategic planning. Later, Santamaria-Garcia et al. [78] identified a number of demographic, social, and economic predictors of cognition and functionality in ageing populations. Also, reducing the level of social deprivation among older adults could positively influence their eating habits and dietary quality, thereby enhancing health outcomes during ageing [84]. According to the above, considering sociodemographic data into longitudinal studies is paramount to understanding individual ageing trajectories and predicting health status [37] and quality of life [55].
3.1.4 Ageing biomarkers
Ageing biomarkers are objective measures used to assess individuals’ ageing process and acquire information about their health and functional status. They help understand the biological processes of ageing and provide insights into the underlying causes of age-related diseases. By combining different types of data, such as gene data, biochemical measurements, and images, complex AI models can help practitioners make better predictions about the evolution of individuals’ ageing. Biomarkers are hence useful to develop novel therapies and prevention strategies to delay ageing effects. According to the type of data used, biomarkers are classified into imaging biomarkers (when using images), molecular biomarkers (when using genomic data), and functional biomarkers (when using haematological data or physiological traits, among others).
Regarding imaging biomarkers, research has centred on brain age prediction to understand the relationship between age and brain morphometrics in the human developmental and ageing process. Studies using magnetic resonance imaging (MRI) have shown that the brain undergoes age-related neuroanatomical changes both regionally and at the network level during ageing. Hence, brain age became an early imaging-based biomarker of neural ageing and a potential biomarker for understanding neurodegenerative and age-associated brain diseases [30]. Later, Cole et al. [31] revealed that a brain-predicted age indicative of an older-appearing brain was associated with several aspects, such as weaker grip strength, poorer lung function, slower walking speed, lower fluid intelligence, higher allostatic load, and increased mortality risk. Brain age has been predicted with high accuracy from multiple ways, including topological changes of the brain structural connectivity [58], brain network perspective [51], the measures of grey matter morphology, white matter microstructure, and resting state functional connectivity [54], and structural MRI in combination with blood parameters [73].
Regarding molecular biomarkers, gene expression profiling is widely used to measure or estimate the passage of time in terms of molecular or genetic changes in organisms. These biomarkers are founded on the idea that concrete biological changes at the molecular or genetic level predictably accumulate over time. Specifically, the work by Freitas et al. [39] proposed a data mining approach to discriminate between ageing-related and non-ageing-related DNA repair genes. More recently, Mamoshina et al. [60] developed a tissue-specific biomarker using differential gene expression and pathway analysis to compare signatures of young and old muscle tissues. Supported by the highly accurate prediction results obtained, this kind of biomarkers can be used to identify new molecular targets for anti-ageing therapies. Also, the study in Fielding et al. [38] showed significant associations between cellular senescence biomarkers and physical performance decline in older adults.
System-wide metabolic changes can also be linked to ageing to develop functional biomarkers. In particular, blood tests have shown promise in predicting human chronological age, with markers such as albumin, glucose, alkaline phosphatase, urea, and erythrocytes emerging as particularly relevant indicators [72]. Upon that concept, Gullett et al. [42] later suggested microRNA as a reliable blood-based biomarker of cognitive ageing, and Nusinovici et al. [67] developed a retinal biological age biomarker using retinal photos, demonstrating its efficacy in predicting mortality and morbidity associated with cardiovascular diseases and cancer.
3.1.5 Factors for healthy ageing
Several articles focus on investigating and understanding the factors that contribute the most to active ageing and the prevention of diseases and disabilities. These studies are paramount to develop effective health interventions and policies aimed at promoting not only healthy and active ageing but also improving the quality of life of the elderly.
Sociodemographic, socioeconomic, and psychosocial factors play a crucial role in healthy ageing [28, 62]. For instance, Ahmadi et al. [22] observed that life satisfaction, quality of life, and social relationships were key factors in predicting successful ageing. Similarly, Yu et al. [95] found that factors such as the place of residency, household type, and neighbourhood infrastructure and facilities influenced domain-specific determinants of active ageing. Moreover, the impact of combined daily activities on memory decline was investigated in Krakovska et al. [50]. Authors showed that engaging in various activities had a stronger effect on memory decline, suggesting the potential efficacy of health policies promoting conducting diverse and multiple activities.
Leveraging computational biology, Cruces-Salguero et al. [32] recently conducted a study on centenarian individuals to identify the key characteristics associated with this demographic. Their research emphasised factors such as the number of diseases, medication usage, frequency of medical consultations, and levels of haemoglobin. Notwithstanding, conducting prolonged studies on humans to understand the diverse nature of the ageing processes is impractical. For this reason, Ala-Korpela et al. [23] investigated whether cross-sectional modelling could differentiate between slow and accelerated ageing in a human population. Results indicated a lack of evidence suggesting that cross-sectional modelling of ageing provides insights into the rate at which an individual’s metabolic profiles change over time.
3.1.6 Reviews
As observed, the application of AI in ageing has gathered the attention of the research community over the years. Thus, previous literature reviews have already been elaborated to organise and summarise existing studies in this field. For instance, the works in Fabris et al. [35] and Farrell et al. [36] examined studies that used supervised ML algorithms to gain insights into ageing processes, including the development of data-driven systems-level models. In [97], authors presented a holistic vision of the use of AI in ageing and longevity research, while the particular benefits of ageing biomarkers based on DL techniques were explored in Zhavoronkov and Mamoshina [98]. Interestingly enough, the authors in Woodman and Mangoni [90] presented a thorough overview of the application of AI in geriatric medicine in older populations, where ageing is a key component. Notwithstanding, all these works present methodological limitations. As a systematic study, Leghissa et al. [57] recently explored the use of ML and DL for detecting and predicting frailty in elderly people. For comparative purposes, Table 3 outlines the main characteristics of these reviews and highlights how our literature review differs from them.
3.2 Data in ageing studies: Types and sources
Research on human ageing, especially when adopting AI-driven strategies, relies on data. A wide range of data types has been used, as indicated in Table 2 and graphically depicted in Fig. 3. This section aims to identify the most relevant data types and data sources in ageing studies (RQ2).
Summary of the types of data used in ageing research. Legend: {BB: Blood biochemistry, CA: Cognitive assessment test, CD: Clinical data, CT: Computed tomography scan, D: Demographic, DW: Drawing, DXA: Dual-energy X-ray absorptiometry measurements, EEG: Electroencephalogram, FA: Functional assessment, GE: Gene expression profile, GT: Gait test, IoT: Internet of Things sensors, MRA: Magnetic resonance angiography scan, MRI: Magnetic resonance imaging scan, NP: Neuropsychological test, PET: Positron emission tomography scan, PM: Physical measures, RE: Retinal photographs, S: Survey}
Biomedical data are the most prominent group of data types by providing health parameters and physiological markers that help study ageing phenomena at a microscopic scale. In this group, we highlight the different types of techniques to produce in-body images, such as MRI scans [27, 30, 31, 44, 45, 48, 51, 53, 54, 56, 58, 59, 61, 68, 70, 73, 75, 77, 79,80,81, 83, 85, 92, 94, 96], positron emission tomography (PET) scans [34, 71], and computed tomography (CT) scans [41]. In this context, most MRI images are brain scans to identify cognitive changes by determining the functional activity of brain regions along ageing as well as discover novel biomarkers. Besides images, biochemical blood measurements [23, 38, 40, 45, 72, 73, 83] and genetic data [39, 42, 60] are usually used in addition to more generic clinical and physical information (e.g., muscle strength, balance, endurance...).
Cognitive data help interpret the complexity of mental and neuropsychological dimensions of individuals by means of cognitive assessment tests (e.g., Mini-Mental State Examination) [47, 93] and neuropsychological tests [40, 48, 83]. Another commonly used kind of data is behavioural data, which provide observable patterns of individuals. These data are generally used to detect and predict pattern changes over time due to ageing. In this context, gait analysis uses spatiotemporal, kinetic, and kinematic measurements collected from inertial sensors and motion cameras [25, 43, 55, 66, 85, 96]. Lastly, contextual data refer to background information providing perspective and a broader understanding of individuals, such as social, economic and demographic indicators [32, 43, 47], wearable- and smart home IoT-collected data [46, 69], and other data collected through surveys [22, 23, 28, 29, 33, 37, 49, 50, 52, 55, 62, 64, 65, 74, 76, 78, 83, 84, 88, 89, 91, 95].
Some studies do not only use a single type of data but combine and integrate multiple types of data [23, 32, 38, 40, 43, 45, 47, 48, 52, 54, 55, 69, 73, 76, 83, 85, 89, 93, 96]. Particularly, imaging data are usually combined with other data types, such as blood biochemistry, physical measurements, neuropsychological tests, and surveys. This multidimensional approach enriches the understanding of factors influencing ageing, their impact on health, and the development of targeted interventions to promote healthy ageing and the well-being of older populations.
To foster research on ageing, different populations have been studied through longitudinal ageing datasets. These datasets have been analysed using AI techniques by some of these works, such as the China Health and Retirement Longitudinal Study (CHARLS) [95], the Chinese Longitudinal Healthy Longevity Survey (CLHLS) [88], the Colombian Survey on Health, Well-Being, and Aging (SABE) [78], the English Longitudinal Study of Aging (ELSA) [28, 33, 37, 49, 74], the Korean Longitudinal Study of Aging (KLoSA) [65], the Health and Retirement Study (HRS) [29, 49, 50], and the Genotype-Tissue Expression (GTEx) [60], among others. A description of the most well-known public datasets intended for research on ageing is provided in Table 4. As observed, these datasets consider small populations (thousands of individuals), which hinders the ability to extrapolate results to larger scales, e.g., at a national level. The high cost to create these datasets (technical infrastructure, human resources...) is certainly a limiting factor.
3.3 AI techniques: approaches and classification
Research on ageing leverages AI techniques, particularly ML techniques, to extract meaningful knowledge from vast amounts of data (see Table 2 and Fig. 4). This section aims to identify and classify the most commonly used AI techniques (RQ3).
ML is a discipline within the field of AI which, by means of algorithms, provides computers with the ability to identify complex patterns from large amounts of data. Contrary to rule-based systems or expert systems, which require a set of predefined rules and domain knowledge introduced by experts, ML algorithms imitate the way that humans learn without being explicitly programmed. ML models are hence useful to explore associations and make more accurate classifications and predictions than their statistical counterparts and confer greater flexibility when modelling unstructured and nonlinear data. However, ML models require large data samples, which are not always possible, to achieve high accuracy and have been criticised in the latest years for their lack of interpretability and explainability [99].
The studies of this systematic review leverage ML techniques for different purposes, namely prediction (i.e., estimating or forecasting future values or outcomes using historical data or observed characteristics), detection (i.e., identifying the presence or absence of specific events or patterns in datasets), classification (i.e., assigning data to groups or predefined classes based on their characteristics), and association (i.e., identifying relationships and connections among elements in datasets). As reported in Table 2, more than half of the studies reviewed concentrate on prediction tasks (58%), followed by detection and classification tasks (20% and 18%, respectively), whereas association tasks have little relevance (4%).
Supervised and unsupervised learning are examples of two different types of ML approaches. On the one hand, supervised learning algorithms are trained on a set of labelled data, i.e., the input data is paired with the desired output, so they learn to predict the output from new input data. Supervised learning is often used for classification, regression, and object detection tasks. On the other hand, unsupervised learning algorithms are trained on a set of unlabelled data, i.e., the input data is not paired with the desired output, so they learn to find patterns and relationships in the data. Unsupervised learning is often used for tasks such as clustering, dimensionality reduction, feature selection, and anomaly detection.
Summary of the AI techniques used in ageing research. Legend: {AB: AdaBoost, ANN: Artificial neural network, CaRT: Classification and regression tree, CB: CatBoost, CNN: Convolutional neural network, DBS: DBSCAN, DR: Dual regression, DT: Decision tree, ENET: Elastic net, GB: GradientBoost, GPR: Gaussian process regression, H: Holistic atlases of functional networks and interactions, ICA: Independent component analysis, KM: k-means, KNN: k-nearest neighbours, Lasso: Least absolute shrinkage and selection operator, LDA: Linear discriminant analysis, LgR: Logistic regression, LiR: Linear regression, MCP: Minimax concave penalty, NB: Naive Bayes, OLS: Ordinary least squares regression, PCA: Principal component analysis, RF: Random forest, Ridge: Ridge regression, RNN: Recurrent neural network, RVR: Relevance vector regression, SCA: Smoothly clipped absolute deviation, SOM: Self-organised maps, SVM: Support vector machines, SVR: Support vector regression, TSC: Two-step clustering, XGB: Extreme gradient boosting}
One of the most extensively used supervised ML algorithms is support vector machines (SVM), useful to solve both complex classification and regression problems by performing optimal data transformations that determine boundaries between data points based on predefined classes in a multidimensional space. In ageing studies, SVMs have been helpful in automatically recognising ageing-related changes in gait patterns between young and elderly walking [25], distinguishing age-related differences in biomedical data like images [61, 63], as well as predicting cognitive impairment [66, 70], frailty [33], and quality of life indicators [55]. Similarly, classification and regression problems are also commonly addressed using random forest (RF) and decision tree (DT) algorithms, which can handle both categorical and continuous data. DTs are a non-parametric method that predicts the value of a target variable by learning simple decision rules inferred from the data features. Ageing studies have used DTs to develop ageing predictor models from highly-dimensional datasets [22, 42], predict cognitive decline in adults using physical and imaging data [66, 82], and classify longevity profiles from longitudinal data [74]. However, DTs are prone to overfitting the training data, especially when trees become very deep or complex, resulting in poor performance on new, unseen data. To solve this problem, RFs are a combination of DTs whose results are accumulated into a final output. Hence, most studies prefer using RF rather than DT algorithms. For instance, RF methods have been used to predict cognitive function and changes [29, 47, 48, 50, 88, 93], classify and predict factors related to successful ageing and quality of life [22, 28, 76, 95], and detect undesirable age-related patterns [84].
In the realm of pure ML classification algorithms, the logistic regression (LgR) algorithm, naive Bayes (NB) algorithm, and linear discriminant analysis (LDA) stand out. These algorithms help predict discrete outputs, distinguish between different categories, or identify which of several classes an observation belongs to. LgR is a fast, scalable algorithm for binary classifications, especially when there are no complex relationships among data features, to e.g., analyse sarcopenia associations [52], and identify the main characteristics linked to centenarians [32]. NB is a probabilistic classifier based on the Bayes theorem with the “naive” assumption of independence among features/predictors, i.e., the presence of one particular feature does not affect the other, which is not always true in the real world. For instance, NB classifiers were used to discriminate between ageing-related and non-ageing related DNA repair genes [39]. Multi-class classification problems are solved using LDA, aimed at finding a linear combination of features that maximises the separation between two or more classes. LDA is a fundamental tool in pattern recognition in order to recognise recurring patterns or structures in data [75].
Whereas ML classification algorithms are intended to predict or classify discrete values, ML regression algorithms provide prediction tools to investigate the relationship between a dependent, continuous variable and independent variables (predictors). The most basic regression model is linear regression (LiR), which has been successfully used to predict the physical and mental components associated with health-related quality of life [55]. However, LiR fails to identify the most relevant predictor variables, especially when data is highly dimensional. To overcome this, LiR models have been adapted to improve variable selection and model regularisation. Among others, the most popular ones are Lasso regression, Ridge regression, and Elastic Net (ENET) regression which combines the Lasso and Ridge regression models. For instance, these three algorithms performed similarly in predicting brain age [51].
Unsupervised learning algorithms are suited for analysing complex, highly correlated, unlabelled data. One of the most popular algorithms is independent component analysis (ICA), which aims to separate and identify the underlying independent sources in large, multivariate datasets. Hence, ICA helps extract the most relevant features from data and understand hidden structures. This technique is suitable for understanding age-related impairments and ageing factors by analysing brain signals and brain images [44, 45, 68, 71, 77, 96]. Also, unsupervised learning enables discovering natural groups within data without any prior knowledge. For instance, groups of similar individuals based on health-related factors [52] or longevity profiles [74] were identified using clustering algorithms, such as k-means (KM) and DBSCAN (DBS) algorithms, respectively.
In recent years, DL, a branch within the ML field, has gained increasing attention. DL relies on artificial neural networks (ANN), capable of learning complex and intricate patterns and relationships within data and, then, using these patterns to make predictions or decisions about new data. ANNs are designed to imitate how humans think and learn by means of layers of interconnected nodes (called neurons) that work together to apply nonlinear data transformations. By building computational models with multiple processing layers, networks can create multiple levels of abstraction to represent the data. Ageing studies have taken advantage of ANNs to predict sarcopenia [24] and ageing-related cognitive variables [53], as well as to develop ageing biomarkers [58, 60, 72]. There are different types of ANNs for solving specific tasks. On the one hand, convolutional neural networks (CNN) are prevalent in image processing, such as MRI and CT scans, for predicting brain age [30, 73], biological age [67] and sarcopenia [41], and detecting brain changes [79, 94]. On the other hand, recurrent neural networks (RNN) work with sequential or temporal data to estimate, for instance, mortality using the history of previous health states [37], or physical activity energy expenditure using sensor data [69].
Most research studies employing AI techniques, including those reviewed in this systematic review, only focus on the performance of their models, often neglecting the interpretability and explainability of such AI models. The black-box nature of ML models, which are unable to provide clear explanations for their decisions, results in a lack of transparency. This has prompted the emergence of explainable AI (XAI) [100], the next frontier in AI research. XAI encompasses a set of processes and methods designed to enable humans to comprehend and trust the outcomes generated by ML algorithms. In this review, only four studies have considered this approach. Concretely, Bae et al. [24] employed the SHapley Additive exPlanations (SHAP) and the Local Interpretable Model-Agnostic Explanations (LIME) methods to assess the functioning of the AI model’s predictions, which helped the authors understand which characteristics contributed most significantly to predicting sarcopenia. Similarly, [89, 93] evaluated their resulting AI models using the SHAP method. Finally, Khodabandehloo et al. [46] presented a novel architecture with a built-in AI explanatory module designed to detect symptoms of cognitive decline, thereby enhancing support for clinicians in diagnostic decision-making.
4 Discussion
Despite the progressive research advances in ageing studies, there is still a long road ahead to foster effective active ageing initiatives and promote healthy lifestyles among older adults. Building upon the main findings from the literature, we next discuss the main challenges in the field (RQ4) and outline future opportunities for ageing research leveraging the full potential of AI (RQ5).
4.1 Main findings
As the world’s population ages, the pursuit of a longer and healthier life has become a priority for scientists and researchers worldwide. Ageing involves complex biological processes influenced by various factors, including genetics, diseases, disorders, social habits, lifestyle, and environmental exposures [101]. Due to the complexity inherent in these processes, traditional computing methods are insufficient in identifying underlying ageing phenomena. In this context, AI has emerged as a key tool capable of discovering hidden patterns within large, complex, heterogeneous, and nonlinear datasets to make accurate age-related predictions. The integration of AI into the study of human ageing not only yields a deeper understanding of these processes but also enables the development of long-term strategies for building preventative and sustainable healthcare systems. The main findings of our review are enumerated below.
AI enhances cognitive ageing assessments
AI has been predominantly used to study cognitive changes associated with ageing, particularly in identifying subtle patterns and changes in the structure and function of the human brain. ML techniques demonstrate proficiency in developing complex models from imaging data, especially MRI and PET scans, to detect or predict abnormal cognitive ageing trajectories. Specifically, affected brain regions can be assessed with high precision, and brain biomarkers can be later developed for the early detection of potential cognitive impairments, such as dementia. Thus, AI improves cognitive ageing assessment and enables the implementation of personalised and advanced intervention strategies to promote proper cognitive health.
AI facilitates early detection of abnormal physical conditions
AI has proven useful in evaluating age-related physical changes. ML algorithms are employed to identify patterns in physical health status and detect early indicators of abnormal conditions such as mobility impairments, sarcopenia, or frailty, which may increase the risk of falls or functional decline. Given the predictive nature of such studies, these insights are crucial for clinicians to intervene at early stages and implement strategies to mitigate or delay their effects.
Contextual factors influence ageing
Beyond physical and cognitive conditions, contextual factors such as social, economic, demographic, and lifestyle variables significantly influence ageing patterns. Although these aspects were not traditionally considered in early studies, they are increasingly being taken into account in more recent research. Adopting positivist perspectives towards old age enables the development of digital health interventions aimed at assisting individuals in modifying their daily habits to promote healthy ageing. By leveraging data from wearable devices and other IoT sources, AI provides personalised recommendations for exercise, diet, and other lifestyle factors that could affect the ageing processes.
DL techniques facilitate biomarkers development
As computational capabilities have increased, AI models have evolved towards more complex structures, mainly using DL approaches with neural network architectures. These models can analyse genetic, haematological, molecular, and imaging data, among other sources, to identify biomarkers aimed at predicting aspects of functional ageing and even facilitating specific longevity interventions. Although biomarkers are objective indicators of the biological ageing process, their standardisation, validation, and reproducibility are hindered by biological diversity [102]. Overcoming these obstacles requires not only sophisticated DL-based models but also a concerted effort to collect and provide inclusive, diverse, and longitudinal data to advance research in this arena.
Need for longitudinal datasets
Research on ageing requires longitudinal datasets that track individuals’ health trajectories, lifestyle changes, and socioeconomic circumstances across multiple waves. These datasets are indispensable for understanding ageing dynamics upon which AI models are built to understand ageing processes. While datasets like the HRS, ELSA, and CHARLS encompass data from thousands of individuals over decades, most datasets are constrained by shorter duration and smaller sample sizes due to the elevated costs involved in their generation.
4.2 Challenges
While AI holds promising potential, several challenges must be addressed to grasp the full potential of these AI-based systems for research on ageing. These challenges have been classified into (i) data challenges, (ii) algorithmic and modelling challenges, and (iii) operational challenges. Regarding data challenges, the following aspects have been identified:
Data quality and completeness
ML algorithms rely heavily on large amounts of high-quality, complete, consistent, and noise-free data. Data quality issues and inadequate sample sizes may not accurately reflect reality, leading to model inefficiencies when applied to new data samples. More extensive longitudinal datasets are needed to mitigate these limitations because current publicly available data repositories often comprise limited population samples.
Data labelling
Supervised ML algorithms require labelled data, which involves standardised assessments and objective annotation. For example, labelling brain images to distinguish between those of healthy adults and those of adults with cognitive impairments. This annotation process is often manual and time-consuming, often requiring the expertise of multiple specialists. Incorporating human knowledge into AI models (human-in-the-loop [103]) helps identify and correct data problems, algorithmic biases, and potential erroneous results.
Accessibility to electronic health records (EHR)
EHRs are a suitable tool to provide standardised, reliable, and interoperable health records that facilitate the analysis of health and physiological parameters, as well as the tracking of diagnoses, procedures, and interventions across large populations. The adoption of EHRs in ageing research would significantly alleviate data integration and management issues. However, accessibility to EHRs may be limited due to bureaucracy and regulatory obstacles along with high implementation and maintenance costs, posing serious challenges in obtaining complete health histories.
Reliability of self-reported data
Self-reported surveyed data, including sociodemographic information, habits, diet, and test evaluations, allow for frequent measurements and precise projections of ageing’s impact. However, self-reported data can be non-representative and biased, relying on individuals’ memory and self-perception.
Next, the following challenges related to AI algorithmic and modelling aspects have been identified:
Lack of generalisation in AI ageing-related models
Most AI-generated models for ageing are ad-hoc solutions trained on concrete data specific to particular regions and cohorts. Recent research by Chekroud et al. [104] revealed that ML models often perform well on one dataset but poorly on other datasets, indicating a lack of generalisation. Validating ML models across different contexts is crucial to ensure their reliability.
Multimodal learning
Combining data from various sources and types, such as biomedical, imaging, demographic, and contextual data, can achieve a comprehensive understanding of ageing-related processes. Unfortunately, this is uncommon. Data fusion enables multimodal learning [105] to improve model performance and reduce dependency on large datasets. However, this presents further challenges including data quality, granularity, interoperability, and temporal misalignment issues.
Continuous re-evaluation of models
As new data waves are introduced into longitudinal datasets, previously unobserved patterns or shifts in population characteristics may emerge, revealing behaviours not detected in the original datasets. This involves periodic retraining and revalidation of ML models to ensure their accuracy and relevance. Consequently, models must adapt to these potential changes to maintain their performance across evolving datasets. The maintenance of these models requires substantial computational resources.
Trustworthiness and interpretability
Enhancing the trustworthiness of AI algorithms is essential. In line with the XAI paradigm, efforts should concentrate on developing novel methods for interpreting AI models to reduce their black-box nature. Making AI decisions comprehensible and explainable will significantly increase trust in this technology.
Last, the following operational challenges have been identified within this context:
Computational cost
DL-based approaches are computationally intensive, requiring high-performance computing infrastructures with elevated computational, memory, storage, and energy consumption costs. While these approaches are suitable for automatically extracting features and estimating millions of parameters within models, they also require extensive amounts of labelled data to achieve high performance, which limits their scalability in real-life ageing applications.
Data protection and ethical considerations
Both data protection and ethical considerations are fundamental in human ageing studies. Practices such as anonymising personally identifiable information, obtaining informed consent, and implementing strict access controls, as outlined in the EU General Data Protection Regulation, are necessary. However, as data collection becomes more opportunistically and ubiquitous through wearables, sensors, and IoT devices, these aspects must be further emphasised. From the ethics perspective, discriminatory models must be avoided and minority profiles cannot be disregarded during AI training [106]. In this context, the recently adopted AI Act within the EU represents an initial but very important step toward regulating the use of AI [107, 108].
4.3 Opportunities and promising applications
The intersection of AI and ageing research represents a promising frontier capable of revolutionising our understanding of ageing processes and developing innovative solutions to promote healthy ageing. Encouraging interdisciplinary collaboration among computer scientists, AI specialists, physicians, ageing researchers, and other professionals is crucial for overcoming the aforementioned challenges. Efforts must be devoted to developing proactive initiatives and preparing healthcare systems to minimise the impact of severe age-related diseases and unhealthy ageing in the years to come. To this end, the main opportunities and promising application in the field are described below.
Understanding ageing traits
The complexity of biological processes along with the lack of appropriate analytical tools hamper the understanding of the interplay of ageing traits and their role in age-related dysfunction. Although age-related traits have been identified [109], understanding their interrelationship across biological, environmental, and genetic domains remains open. Specifically, DL techniques are promising in uncovering relationships with cellular function, tissue-specific physiology, and biomarker development through the analysis of omics data.
Contextualisation of ageing processes
While age-related factors have traditionally been considered intrinsic, such as genetics and epigenetic changes, contextual factors like diet, exercise, lifestyle, and smoking and drinking habits are now recognised for their influence in accelerating the ageing process [110]. Analysing these habits, behaviours, and sociodemographic aspects can reveal different patterns and dynamics related to human ageing. Continuous collection and monitoring of these variables are essential for studying changes at an individual level over time. Promoting studies that incorporate the analysis of extrinsic and contextual variables to complement findings from current ageing studies is desirable.
Augment data collection with Wearable and mobile technology
User-centred biomedical data collected from wearable and mobile devices could augment traditional health records. For instance, these technology can support the automatic monitoring and assessment of intrinsic capacity in older adults following the practices outlined in the ICOPE guidelines [111]. This automation enables closer tracking of patients evolution, beyond traditional physician visits. This mass sensorisation paves the way to study digital biomarkers, opening new avenues for research in ageing and healthcare [112].
Towards context-aware health paradigms
Context-aware paradigms, such as smart health, offer numerous ageing-oriented solutions. Leveraging data gathered from the user’s environment (e.g., smart homes, nursing homes...) through sensors and IoT devices, diverse routines, habits, and patterns can be detected. These insights enable the provision of personalised services to older adults, encompassing health status monitoring, prediction of health trajectories, early detection of cognitive or physical decline, healthy habits recommendation, and assessment of quality of life and well-being.
Crowdsourcing-based solutions
Crowdsourcing-based data solutions present exciting opportunities for studying human ageing. This approach involves collecting, labelling, and classifying large datasets with the help of mass collaboration, aiding in the training, validation, and improvement of ML algorithms. Active participation from the global community would provide a diverse and extensive dataset. Addressing data quality issues could help identify risk factors across different cohorts and develop large-scale biomarkers.
5 Conclusion
Population ageing is a global phenomenon that profoundly impacts economic, social, and health conditions worldwide. AI is shifting many sectors with groundbreaking technological advances, and research on ageing is no exception. The adoption of AI tools is gradually contributing to improving our understanding of the ageing processes. However, the rapid evolution of AI techniques along with the steady advances in ageing research foster the need for a timely review of the state-of-the-art in this field. By following a rigorous review methodology, this article has presented a systematic literature review of the current knowledge on AI for ageing research. The research questions outlined in Table 1 summarise the main goals of our research, which include summarising the main applications, resources, and techniques employed in these studies, as well as highlighting the challenges and promising opportunities in this domain. These research questions are discussed in order next:
RQ1: How has AI currently been used for the study of human ageing?
The potential of AI in studying human ageing is vast, but its application must be justified. In Section 3.1, we have identified the main goals and scope in which AI has been adopted to research on ageing. Our analysis categorises studies into five main areas: (i) age-related cognitive changes, (ii) age-related physical changes, (iii) age-related sociodemographic changes, (iv) ageing biomarkers, and (v) factors for healthy ageing. This taxonomy helps identify the main research areas where AI can make significant contributions. While the majority of studies fall under the first category, focusing on using AI to understand brain function and predict cognitive decline, the rise of DL techniques holds promise for developing ageing biomarkers. Each article has been thoroughly described by carefully emphasising its objectives and contributions.
RQ2: What data sources and data types have been used?
Research on ageing is evidence-based, requiring enormous datasets to derive meaningful knowledge. In Section 3.2, we have observed a wide diversity and heterogeneity in the datasets used for ageing research. Biomedical-related data is predominant, as expected, but it is worth noting the relevance of imaging data, especially from brain regions taken from MRI, PET or CT scans. The analysis of physical measurements, genetic data, and blood measurements is relevant as well. Notwithstanding, there is a growing recognition of the importance of contextual data, which helps understanding ageing considering, not only biomedical data, but also social, economic, and demographic factors as well as lifestyle and daily habits. Understanding ageing within its proper context is essential for developing targeted ageing initiatives tailored to specific populations. Moreover, to foster research on ageing, there exist some public datasets from longitudinal studies. Despite that, these datasets are relatively small (considering only thousands of individuals), region-specific (mostly from China and the United States), and time-constrained (data collected within a decade or less). Addressing these limitations and promoting more comprehensive longitudinal studies, despite their associated costs, is critical to accelerating the pace of ageing research.
RQ3: How AI techniques have been used in current research on ageing?
The number of AI techniques employed for ageing research is vast, as observed in Section 3.3. While ML models are well-suited for classification, prediction, and clustering tasks, DL models are gaining popularity due to their increased efficiency, but at the price of higher computational costs. In a nutshell, SVM, RF, and ANN emerge as the most prominent techniques in the field. However, these methods generally lack interpretability and explainability. To improve the transparency of AI-made decisions, studies are starting to integrate XAI approaches, although their adoption will be pivotal in the years ahead.
RQ4: Which are the main challenges in the field?
As summarised in Section 4.2, continuous efforts are directed towards refining AI models to better comprehend ageing processes. However, challenges such as insufficient data training, data quality issues, and the lack of worldwide longitudinal datasets demonstrate that there is still a long road ahead to overcome all data challenges. Relying on data is first and foremost when adopting AI strategies. Leveraging the context-aware IoT network could enable seamless collection of continuous health data from large populations, though this must be accompanied by efforts to address privacy and ethical issues. Moreover, there is a pressing need to develop interpretable and explainable AI methods to reduce their current black-box nature. Building trust in these systems will be crucial for their successful deployment in real-life scenarios.
RQ5: Which opportunities remain open for future research directions?
Despite the steady progress in the field, research on ageing can still evolve rapidly with the latest AI enhancements. As discussed in Section 4.3, we foresee numerous promising opportunities within the smart healthcare paradigm by leveraging the sensing and communication network of context-aware environments at the time of collecting health information. This information can help develop personalised AI-driven healthcare services and promote active ageing initiatives to improve the quality of life and well-being of older people.
We believe that the information analysed and the research questions answered in this review reflect the current state-of-the-art with high fidelity. As such, this article provides a fruitful and interdisciplinary ground of research and a clear overview of the challenges and opportunities that may need attention in the future. As a final note, we sustain that research on ageing should not focus on adding years to life, but on adding life to years.
Data Availibility
For the sake of transparency, the screening files used in this systematic literature review as well as the PRISMA checklists are available at the following link: https://smarttechresearch.com/opendata/AIageing.
Code Availability
Not applicable.
References
United Nations (2022) World Population Prospects 2022: Summary of Results. Technical Report UN DESA/POP/2022/TR/NO. 3, United Nations Department of Economic and Social Affairs, Population Division, New York, USA
United Nations (2023) The Sustainable Development Goals Report 2023, Special. Technical report, United Nations Department of Economic and Social Affairs, New York, USA
World Health Organization (2020) Decade of healthy ageing: baseline report. Technical report, World Health Organization, Geneva, Switzerland
Beard JR, Officer A, De Carvalho IA, Sadana R, Pot AM, Michel J-P, Lloyd-Sherlock P, Epping-Jordan JE, Peeters GG, Mahanani WR et al (2016) The World report on ageing and health: a policy framework for healthy ageing. The Lancet. 387(10033):2145–2154
Jaul E, Barron J (2017) Age-related diseases and clinical and public health implications for the 85 years old and over population. Front Public Health 5:335
Solanas A, Patsakis C, Conti M, Vlachos IS, Ramos V, Falcone F, Postolache O, Pérez-Martínez PA, Di Pietro R, Perrea DN et al (2014) Smart health: a context-aware health paradigm within smart cities. IEEE Commun Mag 52(8):74–81
Batista E, Borràs F, Martínez-Ballesté A (2015) Monitoring People with MCI: Deployment in a Real Scenario for Low-Budget Smartphones. In: Proc. 6th International Conference on Information, Intelligence, Systems and Applications (IISA), Corfú, Greece, pp. 1–6. IEEE
Casino F, Patsakis C, Batista E, Borras F, Martinez-Balleste A (2017) Healthy routes in the smart city: a context-aware mobile recommender. IEEE Softw 34(6):42–47
Maresova P, Krejcar O, Barakovic S, Husic JB, Lameski P, Zdravevski E, Chorbev I, Trajkovik V (2020) Health-related ICT solutions of smart environments for elderly - systematic review. IEEE Access. 8:54574–54600
Ferre M, Batista E, Solanas A, Martínez-Ballesté A (2021) Smart health-enhanced early mobilisation in intensive care units. Sensors. 21(16):5408
Batista E, Moncusi MA, López-Aguilar P, Martínez-Ballesté A, Solanas A (2021) Sensors for context-aware smart healthcare: a security perspective. Sensors. 21(20):6886
Batista E, Lopez-Aguilar P, Solanas A (2023) Smart health in the 6G era: bringing security to future smart health services. IEEE Communications Magazine 1–7
Secinaro S, Calandra D, Secinaro A, Muthurangu V, Biancone P (2021) The role of artificial intelligence in healthcare: a structured literature review. BMC Med Inform Decis Mak 21(125):1–23
Meskó B, Drobni Z, Bényei É, Gergely B, Győrffy Z (2017) Digital health is a cultural transformation of traditional healthcare. Mhealth 3
Solanas A, Casino F, Batista E, Rallo R (2017) Trends and challenges in smart healthcare research: a journey from data to wisdom. In: Proc. IEEE 3rd international forum on Research and Technologies for Society and Industry (RTSI), pp 1–6. IEEE
Bernal MC, Martínez-Ballesté A, Solanas A (2021) A review of data sources for the study of ageing. In: Proc. IEEE 45th annual Computers, Software, and Applications Conference (COMPSAC), pp 1843–1848. IEEE
Chu CH, Nyrup R, Leslie K, Shi J, Bianchi A, Lyn A, McNicholl M, Khan S, Rahimi S, Grenier A (2022) Digital ageism: challenges and opportunities in artificial intelligence for older adults. Gerontologist 62(7):947–955
Brocke J, Simons A, Niehaves B, Riemer K, Plattfaut R, Cleven, A (2009) Reconstructing the giant: on the importance of rigour in documenting the literature search process. In: Proc. 17th European Conference on Information Systems (ECIS), Verona, Italy, pp. 2206–2217
Moher, D., Liberati, A., Tetzlaff, J., Altman, D.G., Group, P. (2009) Preferred reporting items for systematic reviews and meta-analyses: the PRISMA statement. Ann Intern Med 151(4):264–269
Institute of Electrical and Electronics Engineers: IEEE Thesaurus (2024). https://www.ieee.org/publications/services/thesaurus.html
National Library of Medicine: Medical Subject Headings (MeSH) (2024). https://www.nlm.nih.gov/mesh
Ahmadi M, Nopour R, Nasiri S (2023) Developing a prediction model for successful aging among the elderly using machine learning algorithms. Digital Health. 9:20552076231178424
Ala-Korpela M, Lehtimäki T, Kähönen M, Viikari J, Perola M, Salomaa V, Kettunen J, Raitakari OT, Mäkinen V-P (2023) Cross-sectionally calculated metabolic aging does not relate to longitudinal metabolic changes—support for stratified aging models. The Journal of Clinical Endocrinology & Metabolism. 108(8):2099–2104
Bae J-H, Seo J-W, Kim DY (2023) Deep-learning model for predicting physical fitness in possible sarcopenia: analysis of the Korean physical fitness award from 2010 to 2023. Front Public Health 11:1241388
Begg R, Kamruzzaman J (2005) A machine learning approach for automated recognition of movement patterns using basic, kinetic and kinematic gait data. J Biomech 38(3):401–408
Bidelman GM, Mahmud MS, Yeasin M, Shen D, Arnott SR, Alain C (2019) Age-related hearing loss increases full-brain connectivity while reversing directed signaling within the dorsal-ventral pathway for speech. Brain Struct Funct 224(8):2661–2676
Boyle R, Jollans L, Rueda-Delgado LM, Rizzo R, Yener GG, McMorrow JP, Knight SP, Carey D, Robertson IH, Emek-Savaş DD et al (2021) Brain-predicted age difference score is related to specific cognitive functions: a multi-site replication analysis. Brain Imaging Behav 15(1):327–345
Caballero FF, Soulis G, Engchuan W, Sánchez-Niubó A, Arndt H, Ayuso-Mateos JL, Haro JM, Chatterji S, Panagiotakos DB (2017) Advanced analytical methodologies for measuring healthy ageing and its determinants, using factor analysis and machine learning techniques: the ATHLOS project. Sci Rep 7(1):43955
Casanova R, Saldana S, Lutz MW, Plassman BL, Kuchibhatla M, Hayden KM (2020) Investigating predictors of cognitive decline using machine learning. The Journals of Gerontology: Series B - Psychological Sciences and Social Sciences. 75(4):733–742
Cole JH, Poudel RPK, Tsagkrasoulis D, Caan MWA, Steves C, Spector TD, Montana G (2017) Predicting brain age with deep learning from raw imaging data results in a reliable and heritable biomarker. Neuroimage 163:115–124
Cole JH, Ritchie SJ, Bastin ME, Valdés Hernández MC, Maniega SM, Royle N, Corley J, Pattie A, Harris SE, Zhang Q et al (2018) Brain age predicts mortality. Mol Psychiatry 23(5):1385–1392
Cruces-Salguero S, Larrañaga I, Mar J, Matheu A (2023) Descriptive and predictive analysis identify centenarians’ characteristics from the Basque population. Front Public Health 10:1096837
Cunha Leme DE, De Oliveira C (2023) Machine learning models to predict future frailty in community-dwelling middle-aged and older adults: the ELSA cohort study. Journals of Gerontology: Series A - Biological Sciences and Medical Sciences. 78(11):2176–2184
Di X, Wölfer M, Amend M, Wehrl H, Ionescu TM, Pichler BJ, Biswal BB (2019) The Alzheimer’s disease neuroimaging initiative: interregional causal influences of brain metabolic activity reveal the spread of aging effects during normal aging. Hum Brain Mapp 40(16):4657–4668
Fabris F, Magalhães JPD, Freitas AA (2017) A review of supervised machine learning applied to ageing research. Biogerontology 18(2):171–188
Farrell S, Stubbings G, Rockwood K, Mitnitski A, Rutenberg A (2021) The potential for complex computational models of aging. Mech Ageing Dev 193:111403
Farrell S, Mitnitski A, Rockwood K, Rutenberg AD (2022) Interpretable machine learning for high-dimensional trajectories of aging health. PLoS Comput Biol 18(1):1009746
Fielding RA, Atkinson EJ, Aversa Z, White TA, Heeren AA, Achenbach SJ, Mielke MM, Cummings SR, Pahor M, Leeuwenburgh C, LeBrasseur NK (2022) Associations between biomarkers of cellular senescence and physical function in humans: observations from the lifestyle interventions for elders (LIFE) study. GeroScience. 44(6):2757–2770
Freitas AA, Vasieva O, Magalhães JP (2011) A data mining approach for classifying DNA repair genes into ageing-related or non-ageing-related. BMC Genomics 12(1):1–11
Ganguli M, Beer JC, Zmuda JM, Ryan CM, Sullivan KJ, Chang C-CH, Rao RH (2020) Aging, diabetes, obesity, and cognitive decline: a population-based study. J Am Geriatr Soc 68(5):991–998
Gu S, Wang L, Han R, Liu X, Wang Y, Chen T, Zheng Z (2023) Detection of sarcopenia using deep learning-based artificial intelligence body part measure system (AIBMS). Front Physiol 14:46
Gullett JM, Chen Z, O’Shea A, Akbar M, Bian J, Rani A, Porges EC, Foster TC, Woods AJ, Modave F et al (2020) MicroRNA predicts cognitive performance in healthy older adults. Neurobiol Aging 95:186–194
Hicks GE, Shardell M, Alley DE, Miller RR, Bandinelli S, Guralnik J, Lauretani F, Simonsick EM, Ferrucci L (2012) Absolute strength and loss of strength as predictors of mobility decline in older adults: the InCHIANTI study. Journals of Gerontology: Series A - Biological Sciences and Medical Sciences. 67(1):66–73
Huang C-C, Hsieh W-J, Lee P-L, Peng L-N, Liu L-K, Lee W-J, Huang J-K, Chen L-K, Lin C-P (2015) Age-related changes in resting-state networks of a large sample size of healthy elderly. CNS Neuroscience & Therapeutics. 21(10):817–825
Kharabian Masouleh S, Beyer F, Lampe L, Loeffler M, Luck T, Riedel-Heller SG, Schroeter ML, Stumvoll M, Villringer A, Witte AV (2018) Gray matter structural networks are associated with cardiovascular risk factors in healthy older adults. Journal of Cerebral Blood Flow & Metabolism. 38(2):360–372
Khodabandehloo E, Riboni D, Alimohammadi A (2021) HealthXAI: collaborative and explainable AI for supporting early diagnosis of cognitive decline. Futur Gener Comput Syst 116:168–189
Kimura N, Aso Y, Yabuuchi K, Ishibashi M, Hori D, Sasaki Y, Nakamichi A, Uesugi S, Fujioka H, Iwao S et al (2019) Modifiable lifestyle factors and cognitive function in older people: a cross-sectional observational study. Front Neurol 10:401
Koini M, Duering M, Gesierich BG, Rombouts SARB, Ropele S, Wagner F, Enzinger C, Schmidt R (2018) Grey-matter network disintegration as predictor of cognitive and motor function with aging. Brain Struct Funct 223:2475–2487
Komura T, Cowden RG, Chen R, Andrews RM, Shiba K (2023) Estimating the heterogeneous effect of life satisfaction on cognitive functioning among older adults: evidence of US and UK national surveys. SSM - Mental Health. 4:100260
Krakovska O, Christie GJ, Farzan F, Sixsmith A, Ester M, Moreno S (2021) Healthy memory aging - the benefits of regular daily activities increase with age. Aging (Albany NY). 13(24):25643
Kuo C-Y, Lee P-L, Hung S-C, Liu L-K, Lee W-J, Chung C-P, Yang AC, Tsai S-J, Wang P-N, Chen L-K et al (2020) Large-scale structural covariance networks predict age in middle-to-late adulthood: a novel brain aging biomarker. Cereb Cortex 30(11):5844–5862
Kwon Y-J, Kim HS, Jung D-H, Kim J-K (2020) Cluster analysis of nutritional factors associated with low muscle mass index in middle-aged and older adults. Clin Nutr 39(11):3369–3376
La Corte V, Sperduti M, Malherbe C, Vialatte F, Lion S, Gallarda T, Oppenheim C, Piolino P (2016) Cognitive decline and reorganization of functional connectivity in healthy aging: the pivotal role of the salience network in the prediction of age and cognitive performances. Frontiers in Aging Neuroscience. 8:204
Lange A-MG, Anatürk M, Suri S, Kaufmann T, Cole JH, Griffanti L, Zsoldos E, Jensen DEA, Filippini N, Singh-Manoux A et al (2020) Multimodal brain-age prediction and cardiovascular risk: the Whitehall II MRI sub-study. Neuroimage 222:117292
Lee M, Noh Y, Youm C, Kim S, Park H, Noh B, Kim B, Choi H, Yoon H (2021) Estimating health-related quality of life based on demographic characteristics, questionnaires, gait ability, and physical fitness in Korean elderly adults. Int J Environ Res Public Health 18(22):11816
Lee WH (2023) The choice of machine learning algorithms impacts the association between brain-predicted age difference and cognitive function. Mathematics. 11(5):1229
Leghissa M, Carrera Á, Iglesias CA (2023) Machine learning approaches for frailty detection, prediction and classification in elderly people: a systematic review. Int J Med Informatics 178:105172
Lin L, Jin C, Fu Z, Zhang B, Bin G, Wu S (2016) Predicting healthy older adult’s brain age based on structural connectivity networks using artificial neural networks. Comput Methods Programs Biomed 125:8–17
Lindbergh CA, Zhao Y, Lv J, Mewborn CM, Puente AN, Terry DP, Renzi-Hammond LM, Hammond BR, Liu T, Miller LS (2019) Intelligence moderates the relationship between age and inter-connectivity of resting state networks in older adults. Neurobiol Aging 78:121–129
Mamoshina P, Volosnikova M, Ozerov IV, Putin E, Skibina E, Cortese F, Zhavoronkov A (2018) Machine learning on human muscle transcriptomic data for biomarker discovery and tissue-specific drug target identification. Front Genet 9:242
Meier TB, Desphande AS, Vergun S, Nair VA, Song J, Biswal BB, Meyerand ME, Birn RM, Prabhakaran V (2012) Support vector machine classification and characterization of age-related reorganization of functional brain networks. Neuroimage 60(1):601–613
Mirzaeian R, Nopour R, Asghari Varzaneh Z, Shafiee M, Shanbehzadeh M, Kazemi-Arpanahi H (2023) Which are best for successful aging prediction? Bagging, boosting, or simple machine learning algorithms? Biomed Eng Online 22(1):85
Moezzi B, Pratti LM, Hordacre B, Graetz L, Berryman C, Lavrencic LM, Ridding MC, Keage HAD, McDonnell MD, Goldsworthy MR (2019) Characterization of young and old adult brains: an EEG functional connectivity analysis. Neuroscience 422:230–239
Molassiotis A, Kwok SWH, Leung AYM, Tyrovolas S (2022) Associations between sociodemographic factors, health spending, disease burden, and life expectancy of older adults (70+ years old) in 22 countries in the Western Pacific Region, 1995–2019: estimates from the Global Burden of Disease (GBD) Study 2019. GeroScience. 44(2):925–951
Na K-S (2019) Prediction of future cognitive impairment among the community elderly: a machine-learning based approach. Sci Rep 9(1):3335
Noh B, Yoon H, Youm C, Kim S, Lee M, Park H, Kim B, Choi H, Noh Y (2021) Prediction of decline in global cognitive function using machine learning with feature ranking of gait and physical fitness outcomes in older adults. Int J Environ Res Public Health 18(21):11347
Nusinovici S, Rim TH, Yu M, Lee G, Tham Y-C, Cheung N, Chong CCY, Da Soh Z, Thakur S, Lee CJ et al (2022) Retinal photograph-based deep learning predicts biological age, and stratifies morbidity and mortality risk. Age Ageing 51(4):065
Onoda K, Ishihara M, Yamaguchi S (2012) Decreased functional connectivity by aging is associated with cognitive decline. J Cogn Neurosci 24(11):2186–2198
Paraschiakos S, Sá CR, Okai J, Slagboom PE, Beekman M, Knobbe A (2022) A recurrent neural network architecture to model physical activity energy expenditure in older people. Data Min Knowl Disc 36(1):477–512
Park J, Carp J, Hebrank A, Park DC, Polk TA (2010) Neural specificity predicts fluid processing ability in older adults. J Neurosci 30(27):9253–9259
Pereira JB, Harrison TM, La Joie R, Baker SL, Jagust WJ (2020) Spatial patterns of tau deposition are associated with amyloid, ApoE, sex, and cognitive decline in older adults. Eur J Nucl Med Mol Imaging 47(9):2155–2164
Putin E, Mamoshina P, Aliper A, Korzinkin M, Moskalev A, Kolosov A, Ostrovskiy A, Cantor C, Vijg J, Zhavoronkov A (2016) Deep biomarkers of human aging: application of deep neural networks to biomarker development. Aging (Albany NY). 8(5):1021
Ren B, Wu Y, Huang L, Zhang Z, Huang B, Zhang H, Ma J, Li B, Liu X, Wu G et al (2022) Deep transfer learning of structural magnetic resonance imaging fused with blood parameters improves brain age prediction. Hum Brain Mapp 43(5):1640–1656
Ribeiro CE, Zárate LE (2019) Classifying longevity profiles through longitudinal data mining. Expert Syst Appl 117:75–89
Robinson EC, Hammers A, Ericsson A, Edwards AD, Rueckert D (2010) Identifying population differences in whole-brain structural networks: a machine learning approach. Neuroimage 50(3):910–919
Sajeev S, Champion S, Maeder A, Gordon S (2022) Machine learning models for identifying pre-frailty in community dwelling older adults. BMC Geriatr 22(1):794
Salami A, Wåhlin A, Kaboodvand N, Lundquist A, Nyberg L (2016) Longitudinal evidence for dissociation of anterior and posterior MTL resting-state connectivity in aging: links to perfusion and memory. Cereb Cortex 26(10):3953–3963
Santamaria-Garcia H, Moguilner S, Rodriguez-Villagra OA, Botero-Rodriguez F, Pina-Escudero SD, O’Donovan G, Albala C, Matallana D, Schulte M, Slachevsky A et al (2023) The impacts of social determinants of health and cardiometabolic factors on cognitive and functional aging in Colombian underserved populations. GeroScience 1–19
Schilling KG, Archer D, Yeh F-C, Rheault F, Cai LY, Hansen C, Yang Q, Ramdass K, Shafer AT, Resnick SM et al (2022) Aging and white matter microstructure and macrostructure: a longitudinal multi-site diffusion MRI study of 1218 participants. Brain Struct Funct 227(6):2111–2125
Sone D, Beheshti I, Shinagawa S, Niimura H, Kobayashi N, Kida H, Shikimoto R, Noda Y, Nakajima S, Bun S et al (2022) Neuroimaging-derived brain age is associated with life satisfaction in cognitively unimpaired elderly: a community-based study. Transl Psychiatry 12(25):1–6
Statsenko Y, Habuza T, Smetanina D, Simiyu GL, Uzianbaeva L, Neidl-Van Gorkom K, Zaki N, Charykova I, Al Koteesh J, Almansoori TM et al (2022) Brain morphometry and cognitive performance in normal brain aging: age-and sex-related structural and functional changes. Frontiers in Aging Neuroscience. 13:713680
Sun S, Liu D, Zhou Y, Yang G, Cui L-B, Xu X, Guo Y, Sun T, Jiang J, Li N et al (2023) Longitudinal real world correlation study of blood pressure and novel features of cerebral magnetic resonance angiography by artificial intelligence analysis on elderly cognitive impairment. Frontiers in Aging Neuroscience. 15:1121152
Tan WY, Hargreaves C, Chen C, Hilal S (2023) A machine learning approach for early diagnosis of cognitive impairment using population-based data. J Alzheimers Dis 91(1):449–461
Tatoli R, Lampignano L, Donghia R, Castellana F, Zupo R, Bortone I, De Nucci S, Campanile G, Lofù D, Vimercati L et al (2022) Dietary customs and social deprivation in an aging population from Southern Italy: a machine learning approach. Front Nutr 9:811076
Vaughan BA, Simon JE, Grooms DR, Clark LA, Wages NP, Clark BC (2022) Brain-predicted age difference moderates the association between muscle strength and mobility. Frontiers in Aging Neuroscience. 14:808022
Vergun S, Deshpande AS, Meier TB, Song J, Tudorascu DL, Nair VA, Singh V, Biswal BB, Meyerand ME, Birn RM et al (2013) Characterizing functional connectivity differences in aging adults using machine learning on resting state fMRI data. Front Comput Neurosci 7:38
Vysata O, Kukal J, Prochazka A, Pazdera L, Simko J, Valis M (2014) Age-related changes in EEG coherence. Neurol Neurochir Pol 48(1):35–38
Wang S, Wang W, Li X, Liu Y, Wei J, Zheng J, Wang Y, Ye B, Zhao R, Huang Y et al (2022) Using machine learning algorithms for predicting cognitive impairment and identifying modifiable factors among Chinese elderly people. Frontiers in Aging Neuroscience. 14:977034
Wei M, He S, Meng D, Yang G, Wang Z (2023) Hybrid exercise program enhances physical fitness and reverses frailty in older adults: insights and predictions from machine learning. J Nutr Health Aging 27(10):894–902
Woodman RJ, Mangoni AA (2023) A comprehensive review of machine learning algorithms and their application in geriatric medicine: present and future. Aging Clin Exp Res 35(11):2363–2397
Wu Y, Su B, Chen C, Zhao Y, Zhong P, Zheng X (2023) Urban-rural disparities in the prevalence and trends of depressive symptoms among Chinese elderly and their associated factors. J Affect Disord 340:258–268
Xiong M, Lin L, Jin Y, Kang W, Wu S, Sun S (2023) Comparison of machine learning models for brain age prediction using six imaging modalities on middle-aged and older adults. Sensors. 23(7):3622
Yamada Y, Shinkawa K, Kobayashi M, Badal VD, Glorioso D, Lee EE, Daly R, Nebeker C, Twamley EW, Depp C et al (2022) Automated analysis of drawing process to estimate global cognition in older adults: preliminary international validation on the US and Japan data sets. JMIR Formative Research. 6(5):37014
Yamada S, Otani T, Ii S, Kawano H, Nozaki K, Wada S, Oshima M, Watanabe Y (2023) Aging-related volume changes in the brain and cerebrospinal fluid using artificial intelligence-automated segmentation. Eur Radiol 33(10):7099–7112
Yu J, Huang W, Kahana E (2022) Investigating factors of active aging among Chinese older adults: a machine learning approach. Gerontologist 62(3):332–341
Yuan J, Blumen HM, Verghese J, Holtzer R (2015) Functional connectivity associated with gait velocity during walking and walking-while-talking in aging: a resting-state fMRI study. Hum Brain Mapp 36(4):1484–1493
Zhavoronkov A, Mamoshina P, Vanhaelen Q, Scheibye-Knudsen M, Moskalev A, Aliper A (2019) Artificial intelligence for aging and longevity research: recent advances and perspectives. Ageing Res Rev 49:49–66
Zhavoronkov A, Mamoshina P (2019) Deep aging clocks: the emergence of AI-based biomarkers of aging and longevity. Trends Pharmacol Sci 40(8):546–549
Black JE, Kueper JK, Williamson TS (2023) An introduction to machine learning for classification and prediction. Fam Pract 40(1):200–204
Minh D, Wang HX, Li YF, Nguyen TN (2022) Explainable artificial intelligence: a comprehensive review. Artif Intell Rev 55(1):3503–3568
MacNee W, Rabinovich RA, Choudhury G (2014) Ageing and the border between health and disease. Eur Respir J 44(5):1332–1352
Moqri M, Herzog C, Poganik JR, Justice J, Belsky DW, Higgins-Chen A, Moskalev A, Fuellen G, Cohen AA, Bautmans I et al (2023) Biomarkers of aging for the identification and evaluation of longevity interventions. Cell 186(18):3758–3775
Wu X, Xiao L, Sun Y, Zhang J, Ma T, He L (2022) A survey of human-in-the-loop for machine learning. Futur Gener Comput Syst 135:364–381
Chekroud AM, Hawrilenko M, Loho H, Bondar J, Gueorguieva R, Hasan A, Kambeitz J, Corlett PR, Koutsouleris N, Krumholz HM et al (2024) Illusory generalizability of clinical prediction models. Science 383(6679):164–167
Baltrušaitis T, Ahuja C, Morency L-P (2018) Multimodal machine learning: a survey and taxonomy. IEEE Trans Pattern Anal Mach Intell 41(2):423–443
World Health Organization (2021) Ethics and governance of artificial intelligence for health: WHO guidance. Technical report, World Health Organization, Geneva, Switzerland
European Union (2021) Proposal for a regulation of the European Parliament and the Council laying down harmonised rules on Artificial Intelligence (Artificial Intelligence Act) and amending certain Union legislative acts. EUR-Lex 52021PC0206:1–108
European Union (2023) Artificial Intelligence Act: deal on comprehensive rules for trustworthy AI. Document 20231206IPR15699, Press Release
López-Otín C, Blasco MA, Partridge L, Serrano M, Kroemer G (2023) Hallmarks of aging: an expanding universe. Cell 186(2):243–278
Navarro C, Salazar J, Díaz MP, Chacin M, Santeliz R, Vera I, Parra H, Bernal MC, Castro A, Escalona D et al (2023) Intrinsic and environmental basis of aging: a narrative review. Heliyon. 9(8):18239
World Health Organization (2019) Integrated care for older people (ICOPE): guidance for person-centred assessment and pathways in primary care. Technical report, World Health Organization, Geneva, Switzerland
Piau A, Steinmeyer Z, Cesari M, Kornfeld J, Beattie Z, Kaye J, Vellas B, Nourhashemi F (2021) Intrinsic capacitiy monitoring by digital biomarkers in Integrated Care For Older People (ICOPE). The Journal of Frailty & Aging. 10:132–138
Funding
Open Access funding provided thanks to the CRUE-CSIC agreement with Springer Nature. This work is supported by Ministerio de Ciencia, Innovación y Universidades, Gobierno de España (Agencia Estatal de Investigación, Fondo Europeo de Desarrollo Regional -FEDER-, European Union) under the research grant PID2021-127409OB-C33 CONDOR. Also, this work is partially founded by AGAUR research group 2021SGR-00111 “ASCLEPIUS: Smart Technology for Smart Healthcare”. This work is also supported by International Experts Program promoted by the Ministerio de Ciencia, Tecnología e Innovación through Instituto Colombiano de Crédito Educativo y Estudios Técnicos en el Exterior - ICETEX, and internal funds for research strengthening from Universidad Simón Bolívar, Vicerrectoría de Investigación, Extensión e Innovación, Colombia.
Author information
Authors and Affiliations
Contributions
Mary Carlota Bernal: Conceptualisation, Methodology, Validation, Formal analysis, Visualisation, Data curation, Writing - Original Draft. Edgar Batista: Methodology, Formal analysis, Visualisation, Writing - Original Draft, Writing - Review & Editing. Antoni Martínez-Ballesté: Methodology, Formal analysis, Writing - Review & Editing, Supervision, Funding acquisition. Agusti Solanas: Conceptualisation, Methodology, Formal analysis, Writing - Review & Editing, Supervision, Funding acquisition.
Corresponding author
Ethics declarations
Conflict of Interest
The authors declare that they have no known competing financial interests or personal relationships that could have appeared to influence the work reported in this paper.
Ethics Approval and Consent to Participate
Not applicable.
Consent for Publication
Not applicable.
Materials Availability
Not applicable.
Additional information
Publisher's Note
Springer Nature remains neutral with regard to jurisdictional claims in published maps and institutional affiliations.
Rights and permissions
Open Access This article is licensed under a Creative Commons Attribution 4.0 International License, which permits use, sharing, adaptation, distribution and reproduction in any medium or format, as long as you give appropriate credit to the original author(s) and the source, provide a link to the Creative Commons licence, and indicate if changes were made. The images or other third party material in this article are included in the article’s Creative Commons licence, unless indicated otherwise in a credit line to the material. If material is not included in the article’s Creative Commons licence and your intended use is not permitted by statutory regulation or exceeds the permitted use, you will need to obtain permission directly from the copyright holder. To view a copy of this licence, visit http://creativecommons.org/licenses/by/4.0/.
About this article
Cite this article
Bernal, M., Batista, E., Martínez-Ballesté, A. et al. Artificial intelligence for the study of human ageing: a systematic literature review. Appl Intell (2024). https://doi.org/10.1007/s10489-024-05817-z
Accepted:
Published:
DOI: https://doi.org/10.1007/s10489-024-05817-z