Abstract
Post-event Interferometric Synthetic Aperture Radar (InSAR) analysis on a stack of 45 C-band SAR images acquired by the ESA Sentinel-1 satellites from 9 October 2014 to 19 June 2017 allowed the identification of a clear precursory deformation signal for the Maoxian landslide (Mao County, Sichuan Province, China). The landslide occurred in the early morning of 24 June 2017 and killed more than 100 people in the village of Xinmo. Sentinel-1 images have been processed through an advanced multi-interferogram analysis capable of maximising the density of measurement points, generating ground deformation maps and displacement time series for an area of 460 km2 straddling the Minjiang River and the Songping Gully. InSAR data clearly show the precursors of the slope failure in the source area of the Maoxian landslide, with a maximum displacement rate detected of 27 mm/year along the line of sight of the satellite. Deformation time series of measurement points identified within the main scarp of the landslide exhibit an acceleration starting from April 2017. A detailed time series analysis leads to the classification of different deformation behaviours. The Fukuzono method for forecasting the time of failure appear to be applicable to the displacement data exhibiting progressive acceleration. Results suggest that satellite radar data, systematically acquired over large areas with short revisiting time, could be used not only as a tool for mapping unstable areas, but also for landslide monitoring, at least for some typologies of sliding phenomena.
Similar content being viewed by others
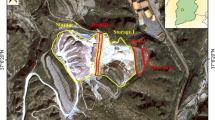
Avoid common mistakes on your manuscript.
Introduction
Large-scale, geohazard-induced displacement mapping became available to researchers and end-users with the development of the interferometric techniques applied to synthetic aperture radar (SAR) data (Fielding et al. 1998; Massonnet et al. 1994; Singhroy et al. 1998, Ferretti et al. 2007). These allowed the generation of digital elevation models, as well as the detection and monitoring of subtle ground deformation phenomena by comparing range information gathered from SAR sensors from two slightly different view angle or at different times.
Over the last two decades, conventional interferometric SAR (InSAR) techniques, based on the analysis of just two acquisitions, have been significantly improved. Today, more and more results are based on multi-interferogram algorithms, which trade the quality of displacement measurements with spatial continuity. In fact, these techniques use long-time series of SAR images to estimate and remove the atmospheric component of the interferometric phase over a sparse grid of coherent points, generally referred to as permanent or persistent scatterers (Ferretti et al. 2001; Kampes 2006; Prati et al. 2010). More recently, more sophisticated techniques have been presented, capable of maximising the spatial density of measurement points by taking advantage of different scattering mechanisms (Ferretti et al. 2011).
InSAR data are now commonly used for a variety of purposes, such as landslide detection and mapping (Herrera et al. 2011; Lu et al. 2012; Cigna et al. 2013; Raspini et al. 2013; Bardi et al., 2016), monitoring and modelling (Berardino et al. 2003; Hilley et al. 2004; Tofani et al. 2013; Komac et al. 2015; Carlà et al. 2016), hazard and risk assessment (Lu et al. 2014) and, through time series analysis, for the identification of changes in deformation rates (Frodella et al. 2016) and seasonal trends (Tomás et al. 2016).
Displacement monitoring is one of the main components of early warning systems (EWSs) applied to landslides (Intrieri et al. 2013). EWSs are usually implemented at local scale, on single slopes (Clark et al. 1996; Froese et al. 2005; Ruch 2009; Michoud et al. 2013; Gigli et al. 2014; Froese and Moreno 2014), where in situ monitoring systems can be installed and operated. On the other hand, EWSs at basin or regional scale are typically developed for rainfall-induced landslides by exploiting records and forecasts of rainfall (Yu et al. 2004; Guzzetti et al. 2007; Capparelli and Tiranti 2010; Thiebes 2012; Lagomarsino et al. 2013). The main limitation of the latter systems is that, contrary to kinematic parameters, rainfall is an indirect indicator of slope instabilities, which increases the risk of missed or false alarms (Lacasse and Nadim 2008; Intrieri et al. 2012).
So far, the long revisiting time and the limited access to satellite radar data suitable for interferometric analyses have been the main limitations to the use of SAR sensors as a tool for monitoring ground deformations over wide areas. However, over the last decade, with the launch of new constellations of satellites, the acquisition frequency increased to the point of making satellite InSAR suitable for displacement monitoring and—under certain circumstances and for some typologies of phenomena—early warning purposes.
In this paper, we present a case study that clearly highlights the potential of the new generation of SAR sensors for wide area monitoring. The Maoxian landslide occurred in the Sichuan province (China) on 24 June 2017. This province is a notoriously landslide-prone and highly seismic region. Rainfall, occurring especially during the monsoon season, contributes to the risk in this region, and cause unstable slopes to be numerous and spread over vast areas. Large-scale displacement monitoring can become a valuable tool for assessing the stability of slopes, particularly when the difficult accessibility of the site or the lack of clear indicators of instability make specific monitoring not feasible or look as not necessary.
To this end, ESA Sentinel-1A and B satellites can be considered a breakthrough. They mount SAR sensors suitable for large-scale mapping (Kalia et al. 2017; Novellino et al. 2017; Vecchiotti et al. 2017), with short revisiting times (6 days, when both sensors are active over the same area) and quite good spatial resolution (Geudtner et al. 2014; Barra et al. 2017; Casagli et al. 2017). The enhanced temporal resolution of Sentinel-1 compared to all previous ESA missions and the regularity of the acquisitions using the same acquisition mode are now paving the way to the use of InSAR data for early detection of movements in places where ground-based monitoring would be unfeasible, like the remote landslide-prone areas in Sichuan.
The aim of this paper is to describe the main kinematic characteristics of the Maoxian landslide in relation to a perspective use of Sentinel-1 multi-temporal observations for early warning purposes. The analysis is of particular interest since our results show that, if InSAR monitoring had been active over this region, an early warning of the imminent failure could have been given in the first days of June 2017.
Description of the Maoxian landslide
The Maoxian landslide occurred at 5:45 a.m. on 24 June 2017. Although the landslide is named after the county where it is located (Maoxian, namely Mao County, Aba Prefecture, Sichuan Province), it actually happened in Xinmo Village (32° 03′ 58′′ N, 103° 39′ 02′′ E, 2300 m a.s.l.) that is about 50 km away from the Maoxian. By the end of June 2017, the Maoxian landslide has buried 62 houses and more than 100 people (Chinese Government 2017a).
It can be classified as a rock avalanche that originated from the crest of a high slope of about 55–60°. The main type of the bedrock in this area is quartzite with cataclastic texture, which tends to fracture and break during high velocity movement, and subsequently to travel with high gravitational potential energy.
At regional scale (Fig. 1), the landslide area is situated at the eastern margin of the Tibetan Plateau, an area with active tectonics that may result from the collision between the Indian and the Eurasian Plates, undergoing abrupt topographic changes from the Sichuan Basin (Jiang et al. 2014). In particular, it is located in the Longmenshan–Minshan Fault zones, where local landforms of high mountains and deep valleys in this area were largely influenced by such active tectonics.
This area is also placed in the middle part of the north trending earthquake zones and is characterised by frequent earthquakes. In history, this area has been struck by several strong earthquakes, including the 1933 Diexi earthquake (M = 7.5), the 1976 Songpan-Pingwu earthquake swarm (M = 7.2) and the 2008 Wenchuan earthquake (M = 8.0) (Fig. 1). The 1933 Diexi earthquake and the 1976 Songpan-Pingwu earthquakes were considered as a consequence of the southern Minjiang Fault and the Huya Fault, respectively (Chen et al. 1994). The 2008 Wenchuan earthquake occurred along the stress-accumulated Longmenshan trust belt with an epicentre located 120 km from Xinmo and ruptured a 9-m slip along the boundary between Longmenshan and the Sichuan Basin (Parsons et al. 2008).
These strong earthquakes may have brought abundance of source materials and caused intensive neotectonic deformations and landslides along the narrow and deep valleys incised by the Minjiang River and its tributaries. For example, the 1933 Diexi earthquake (whose epicentre was located less than 10 km far from Xinmo) has caused about 6800 fatalities directly from the earthquake-triggered landslides and 8000 fatalities from the associated formation of 12 landslide dams (Chang 1934; Dai et al. 2005; Huang 2009). Eight landslide dams remain today and analyses on the preserved soft-sediment deformation structures reveal that their potential causes are earthquakes and the derived landslides (Wang et al. 2011). In addition, the landslides triggered by the 2008 Wenchuan earthquake have brought about 20,000 deaths from 15,000 events and left more than 10,000 potential hazardous sites and 256 landslide dams in this area (Cui et al. 2009; Wang et al. 2013; Xu et al. 2009; Yin et al. 2009). The earthquake affected all the counties within a radius of some hundreds of kilometres and in the sole Maoxian caused 287 new landslides or unstable slopes (Yin et al. 2009).
Although currently no particular evidence shows that the Maoxian landslide was triggered by the repeated earthquakes, considering the history of this region, it is completely possible that strong and frequent seismic shakes might have weakened and partially destabilised the slope from where the 24 June 2017 rock avalanche detached, probably favoured by the intense rainfall recorded in the preceding days. In fact, evidences of ancient instability events (scars near the crest and landforms relatable to old landslide deposits at the foot) are also visible (Fig. 2).
The Maoxian landslide (Fig. 2) initiated at the slope above the Xinmo Village, then slid into the Songping Gully (which was only partially obstructed but not completely dammed) and consequently destroyed the whole village. The estimated volume of the landslide is about 18 million cubic metres (Chinese Government, 2017b) and a total area of 1.5 km2. The height of the source area of the landslide is about 3400 m a.s.l., while the foot of the landslide, where the Xinmo village is located, is with the elevation of about 2300 m a.s.l.; thus, the height difference (H) of this landslide is about 1100 m, with the runout distance (L) of about 2600 m, corresponding to an angle of reach (fahrböschung) of 22°. These morphometric values fit quite well with the empirical graphs for rock avalanches plotting travel angle against volume (Rickenmann 2005) and area against volume (Li 1983), obtained with historical data from literature. Considering the far distance of the source area and the fact that no road and village was built on the high slope, it would have been very difficult to detect early signs of mass movement, let alone issuing a proper early warning at dawn.
Westerlies, Asian monsoon and Tibetan Plateau monsoon generally influence the local climate, leaving the river valleys arid and high mountains humid. In Maoxian, the average annual precipitation is about 491 mm, 70–90% of which falls in the raining season between June and September (Jiang et al. 2014). During June 2017, the raining was intermittent, generally without extremely intense events. On 23 June 2017, namely 1 day before the landslide occurrence, the rain recorded at the Songping Gully was relatively heavy (9 mm).
Analysis of Sentinel-1 data at Maoxian landslide
Immediately after the event, a multi-interferogram analysis of the C-band (5.6-cm wavelength) SAR images acquired by Sentinel-1 satellites has been performed. More precisely, the analysis was carried out on Sentinel-1 images acquired with the Interferometric Wide (IW) swath mode, the main acquisition mode over land which acquires data with a 250-km swath at 5 m × 14 m spatial resolution. The available archive of Sentinel-1, including 45 images and spanning the time interval from 9 October 2014 to 19 June 2017, has been acquired and processed. Images have been captured along satellite track n.62 in descending orbit and with an incidence angle of 40.78°.
The extension of the analysed area is about 460 km2, covering a long sector of Minjiang River and of its right tributary, the Songping Gully. More than 130,000 measurement points (MPs) were identified. The main purpose of the InSAR analysis was to retrieve information on ground deformation, with a particular focus on the identification of possible precursory motion of the Maoxian landslide.
Displacement data were obtained using the SqueeSAR technique (Ferretti et al. 2011), a second generation PSInSAR algorithm. As already mentioned, these methods belong to the persistent scatterer interferometry (PSI) family and are based on the processing of long temporal series of co-registered, SAR images acquired over the same target area from the same acquisition geometry. These methods rely on the identification of pixels exhibiting good phase coherence during the entire observation period, via proper statistical analyses. These points are usually referred to as permanent (or persistent) scatterers (PS) (Ferretti et al. 2001). PS usually correspond to anthropic (buildings, metallic structures) or natural objects (rock outcrops, boulders) exhibiting a stable ‘radar signature’ with time. PS represent point-like targets where the response to the radar is dominated by a strong reflecting object within the single-resolution cell. Using this sparse grid of point-like targets, PSI techniques are able to statistically identify, quantify, and remove atmospheric artefacts from SAR phase data and create a history of range variations for each target.
Unlike the PSInSAR approach, the SqueeSAR technique allows for the measurement of surface displacements by exploiting both point-wise coherent scatterers (i.e., the PS) and partially coherent distributed scatterers (DS). DS correspond to low-reflectivity homogeneous areas, whose extension may vary from hundreds to few thousands of square meters, spread over a group of pixels sharing similar radar returns (e.g. scattered outcrops, bare soil, debris-covered zones and non-cultivated lands). This innovative approach provides a significant increase in spatial density of the total MPs, with respect to the traditional PSI techniques, especially in the case of vegetated scenarios (Bellotti et al. 2013; Bardi et al. 2014), enabling a much better understanding of possible ground deformation phenomena threatening a specific area of interest. Considering the wide vegetation cover in the landslide area, most measurement points are represented by DS, with few PS limited to rocky outcrops in the source area and to the Xinmo village.
The SqueeSAR algorithm applied to the Sentinel-1 data-stack provided valuable information on ground movements before the event. PS and DS were detected and classified according to their mean annual line of sight (LOS) velocities (Fig. 3). In our results, positive values correspond to motion toward the satellite, while negative values to motion away from the satellite (in mm/year). Displacement data were calculated with respect to a reference point located on a stable sector north of the Xinmo landslide area (star symbol in Fig. 3). The results indicate the presence of many areas affected by surface displacements along the narrow and deep valleys incised by the Minjiang River and its tributaries. Most of them are likely related to slope instability phenomena. However, the full extent of the moving areas cannot be determined due to the presence of vegetation, which hinders the density of MPs.
The area of the Xinmo village exhibits very low deformation rates, ranging between − 2.0 and 2.0 mm/year, indicating relatively stable ground conditions (green to light blue regions). Nevertheless, SqueeSAR results highlight the presence of active movements in a large sector of the slope above the Xinmo village (Fig. 4). This sector is interpreted as the source area of the landslide. Here, about 700 MPs are identified, with deformation rates exceeding several millimetres per year. The highest deformation rates (red points) were recorded in the upper part of the slope, close to the landslide scarp. Here, velocity values range between − 10 and − 20 mm/year, with peaks of about − 27 mm/year. In this case too, considering the acquisition geometry and the orientation of the slope, the measured deformation rates are consistent with the occurrence of precursory movements over a large sector of the slope affected by the 24 June 2017 landslide. It is worth remarking that this specific sector of the slope was the origin of the sliding event.
Besides the simple use of the mean yearly velocity, landslide analysis takes advantage from the information included within the displacement time series (TS) of each MP. TS describe the evolution of deformation over the entire monitoring period (from 9 October 2014 to 19 June 2017) and are of paramount importance for studying the kinematics of a given phenomenon, highlighting potential changes occurred during the monitoring period, such as sudden accelerations prior to a landslide failure.
To enhance information included in the SqueeSAR analysis, following the generation of ground deformation maps, displacement TS of each measurement point have been automatically analysed to identify, in the last 180 days of the TS, any change in the deformation pattern, i.e., points affected by acceleration over the last 6 months (December 2016–June 2017). This trend variation analysis identifies a large cluster of MPs, in the upper part of the Xinmo landslide, affected by a progressive acceleration from April 2017, clearly visible in time series of deformation (Fig. 4).
Then, a manual analysis of the TS within this cluster of points in the source area of the Xinmo landslide has been performed. This enabled to further detail this result by recognising at least three different areas characterised by homogeneous kinematic behaviours which can be grouped in accelerating, linear and stable areas; for each area, a representative point has been selected and plotted (Fig. 5).
Accelerating areas are the most important to detect for early warning purposes, as they are directly related to slope instability. In particular, a slope might incur in a hyperbolic acceleration, called tertiary creep, that typically precedes collapse and from which a time of failure can be forecasted (Saito 1969; Fukuzono 1985; Rose and Hungr 2007; Mufundirwa et al. 2010; Carlà et al. 2017); the most popular method to achieve this prediction has been developed by Fukuzono (1985) and consists in plotting the inverse of the slope velocity against time: the point where the extrapolation of this plot intersects the time axis (corresponding to a theoretical infinite velocity) is an estimation of the time of failure.
For the Maoxian landslide, the accelerating areas are located in the NW sector of the source area. The onset of acceleration can be individuated since the acquisition of 20 April 2017 and led to an average velocity of around 2 mm/day recorded on several MPs between 7 and 19 June 2017 (Fig. 5). This is the area from where it is deducible that the initial failure occurred and then developed in both a retrogressive and progressive mode.
Areas characterised by a linear behaviour (which may correspond with the so-called secondary creep) are index of instability in a state of limit equilibrium. There is no indication about how long this behaviour could last or whether it will evolve into an accelerating or, instead, a stable behaviour (in Fig. 5 MPs evolving from stable to accelerating behaviour have been classified in the latter category). In this case, these areas surround the accelerating ones and are possibly affected by their movement. An area showing a linear behaviour is situated few tens of metres above the landslide, as a further confirmation that, if a trend change does not occur and if the zone is not hit by another collapsing sector, no failure is expected to occur.
Stable MPs are considered for those recording a linear trend of displacement with mean 0; measured movements are explained as noise or neglectable deformations. These resulted to be located outside of the landslide but also, interestingly, within its boundaries, in the SE sector of the source area (Fig. 5). The reasons for this might be varied; for example, an unfavourable satellite LOS with respect to the movement in this part of the slope might have disabled the detection of higher deformations; alternatively, this could have been an actual stable zone later involved by the lateral expansion of the accelerating areas or by the collapse of the above area, at the landslide crest (upon which only hypotheses can be done, due to the absence of any MP). As stated above, an a posteriori forecast of the time of failure can be performed starting from the accelerating TS. In this case, Fukuzono’s (1985) method has been applied to a TS obtained from the one of the accelerating MPs, which has been further smoothed with a 5-sample asymmetric moving average (Fig. 6).
Until Spring 2017, data experience a large variability because when the velocity is almost null, its inverse tends toward infinite. For example, the decreasing trend visible between June and October 2016 is only apparently indicating a tertiary creep, as clearly visible for the displacement plot which does not show a hyperbolic curve (Fig. 6). On the other hand, they start to show a proper trend toward 0, thus making Fukuzono’s method applicable, since 20 April 2017, consistently with the trend change detected automatically. As of 26 May, the extrapolation of the inverse velocity would have indicated a misleading 10 June as the date of failure; a later trend change would have forced to recalculate the time of failure with more updated acquisitions. Using data acquired on 7 June would have forecasted 19 June as time of failure. A forecast performed with data acquired on 19 June (5 days before the landslide occurred) would have predicted the failure on 23 June. The fitting of the trend line in the latter case is good (R 2 = 0.91) and can be considered as a rough indicator of the reliability of the forecast; in any case, the analysis of previous data and the constant acceleration of the landslide would have left little doubts about its imminent failure, to the point that an accurate estimation was already possible with the 26 May measurement.
Discussion: perspective and limitations of InSAR as landslide early warning systems
Although the 24 June 2017 Maoxian event occurred in a landslide-prone area (often caused by earthquakes and connected with the subsequent formation of unstable natural dams), so far, no monitoring network could cover the whole region and provide useful information for early warning procedures.
Data presented in this paper are promising, since they clearly point toward the possibility to use ESA Sentinel-1 data to feed early warning systems, where possibly other layers of information (e.g. precipitation data, optical imagery) are integrated and used to mitigate and manage natural hazards. Compared to previous satellites, Sentinel-1 data couple some favourable characteristics: short revisiting time, regional-scale mapping capability (due to the large—250 km—swath), systematic and regular SAR acquisitions, rapid product delivery and free data access.
Sentinel-1 data were processed using the SqueeSAR technique to obtain the highest spatial density of measurement points over the area of interest. These have been analysed after the event and showed that around 100 MPs clustered in a 50,000 m2 surface within the source area of the landslide exhibited a strong acceleration in the time period between the end of April and the beginning of May 2017. The application of the inverse velocity method, after basic filtering procedures, revealed that the date of failure could have been estimated with reasonable accuracy immediately after the acquisition of 26 May. The presence of a clear hyperbolic displacement curve and the corresponding linear trend toward zero in the inverse velocity plot are strong hints of an incoming failure. If an early warning system was in place at the time, the evacuation of Xinmo village would have been highly advisable, even though Fukuzono’s method is, naturally, subject to missed or false alarms. For a detailed dissertation upon the confidence of landslide forecasting methods refer to Intrieri and Gigli (2016).
It should be stressed that, in this area, the full potential of the ESA Sentinel constellation has not been exploited, since most of the acquisitions are characterised by a return period of 24 days until February 2017 and 12 days afterwards. Assuming a 6-day revisiting time (which is achieved when both Sentinel-1A and Sentinel-1B acquire on the same area), a clear trend on the inverse velocity plot could have been highlighted even at an earlier time.
This means that the transition from historical analysis of ground deformation to a sort of continuous monitoring at regional scale using satellite radar data is now possible, thanks to the increased temporal frequency of the acquisitions and computational power now available, allowing the rapid generation of ground deformation maps and displacement time series immediately after every new SAR acquisition.
In general, the presence of a single outlier in the time series of an InSAR measurement point is not enough to provide a reliable and robust warning signal. Without prior information, even relying on the best InSAR algorithms available today, in order to keep low the false alarm rate, at least 4–5 samples “out of statistics” are necessary before any warning could be activated, thus reducing the lead time between the issuing of a warning and the response of the population. This means that only those landslides experiencing progressive acceleration over more than a month have a chance to be predicted today. Furthermore, satellites have an unfavourable LOS to detect movements directed toward N or S and this can further reduce their ability to provide useful information of landslide prediction.
However, the application of automatic algorithms for the detection of trend changes in displacement time series can provide a unique contribution to the early identification of any anomalous trend and/or acceleration. A supervised analysis on the MPs for the Maoxian landslide produced similar results for both identifying the onset of acceleration and for localising MPs affected by anomalous trends. For sure, the use of automatic anomalous trend detection at regional scale can provide a tool for a preliminary warning (possibly by applying the inverse velocity method) and can significant improve warning procedures and save precious time.
This paper represents the feasibility stage of a whole process that, after appropriate testing, validation and refinement, might lead to the implementation of proper satellite landslide early warning systems based on continuous processing of Sentinel-1 data. Then, the biggest issues will not be of technical nature, but mostly social and related to an efficient conveyance of the warnings and response of the population.
Conclusions
The Maoxian landslide occurred on 24 June 2017 in the Sichuan Province (China). It can be classified as a 13 million m3 (Fan et al. 2017) rock avalanche that resulted in a runout distance of about 2600 m. It has been analysed through a multi-interferometric approach applied to 45 C-band SAR images acquired by the Sentinel-1 satellites from 9 October 2014 to 19 June 2017. InSAR results highlighted the presence of active movements in the source area of the Maoxian landslide with a maximum displacement rate detected of 27 mm/year along the line of sight of the satellite. Displacement time series in the area of the main scarp of the Maoxian landslide revealed pre-failure signs, with a clear acceleration from late April 2017. Time series in this part of the source area have been classified according to their deformation behaviours, which showed how the failure initiated from a 50,000 m2 area located near the crest of the mountain and then developed progressively and retrogressively.
Fukuzono’s method for the forecasting of the time of failure has been successfully applied, pointing out that an accurate estimation of the failure time was already possible since the beginning of June. The obtained results were all obtained without any in situ survey and show that a prompt data analysis for the early identification of any anomalous trend and/or acceleration of ground deformation using satellite radar data is now possible. Moreover, continuous monitoring of ground deformation at regional scale using Sentinel-1 is now feasible thanks to the short revisiting time, the regional-scale mapping capability, the regularity of acquisitions, the free data access, as well as the availability of new InSAR algorithms capable of maximising the spatial density of measurement points.
References
Bardi F, Frodella W, Ciampalini A, Bianchini S, Del Ventisette C, Gigli G, Fanti R, Moretti S, Basile G, Casagli N (2014) Integration between ground based and satellite SAR data in landslide mapping: the San Fratello case study. Geomorphology 223:45–60
Bardi F, Raspini F, Ciampalini A, Kristensen L, Rouyet L, Lauknes TR, Frauenfelder R, Casagli N (2016) Space-borne and ground-based InSAR data integration: the Åknes test site. Remote Sens 8(3):237
Barra A, Monserrat O, Crosetto M, Cuevas-Gonzalez M, Devanthéry N, Luzi G, Crippa B (2017) Sentinel-1 data analysis for landslide detection and mapping: first experiences in Italy and Spain. In Workshop on World Landslide Forum Springer, Cham, pp. 201–208
Bellotti F, Bianchi M, Colombo D, Ferretti A, Tamburini A (2013) Advanced InSAR techniques to support landslide monitoring. In Mathematics of planet earth, Proceedings of 15th Annual Conference for the International Association for Mathematical Geosciences, Madrid, Spain, 2–6 September, 287–290
Berardino P, Costantini M, Franceschetti G, Iodice A, Pietranera L, Rizzo V (2003) Use of differential SAR interferometry in monitoring and modelling large slope instability at Maratea (Basilicata, Italy). Eng Geol 68(1–2):31–51
Capparelli G, Tiranti D (2010) Application of the MoniFLaIR early warning system for rainfall-induced landslides in piedmont region (Italy). Landslides 4:401–410. https://doi.org/10.1007/s10346-009- 0189-9
Carlà T, Raspini F, Intrieri E, Casagli N (2016) A simple method to help determine landslide susceptibility from spaceborne InSAR data: the Montescaglioso case study. Environ Earth Sci 75(24):1–12. https://doi.org/10.1007/s12665-016- 6308-8
Carlà T, Intrieri E, Di Traglia F, Nolesini T, Gigli G, Casagli N (2017) Guidelines on the use of inverse velocity method as a tool for setting alarm thresholds and forecasting landslides and structure collapses. Landslides, 14(2): 517–534. doi: https://doi.org/10.1007/s10346-016- 0731-5
Casagli N, Tofani V, Morelli S, Frodella W, Ciampalini A, Raspini F, Intrieri E (2017) Remote sensing techniques in landslide mapping and monitoring, keynote lecture. In workshop on world landslide forum. Springer, Cham, pp.19
Chang LQ (1934) Investigation report on Diexi earthquake of Sichuan. Geol Rev 3(3):251–291 (in Chinese)
Chen SF, Wilson CJL, Deng QD, Zhao XL, Zhi LL (1994) Active faulting and block movement associated with large earthquakes in the min Shan and Longmen mountains, northeastern Tibetan plateau. J Geophys Res Solid Earth 99:24025–24038. https://doi.org/10.1029/94JB02132
Chinese Government (2017a) http://english.gov.cn/news/top_news/2017/06/24/content_281475696976294.htm. Accessed on 20 July 2017
Chinese Government (2017b) http://www.gov.cn/xinwen/2017-06/24/content_5205161.htm. Accessed on 20 July 2017. In Chinese
Cigna F, Bianchini S, Casagli N (2013) How to assess landslide activity and intensity with persistent scatterer interferometry (PSI): the PSI-based matrix approach. Landslides 10(3):267–283
Clark AR, Moore R, Palmer JS (1996) Slope monitoring and early warning systems: application to coastal landslide on the south and east coast of England, UK. In: Senneset K (ed) Landslides, 7th international symposium on landslides. Balkema, Rotterdam, pp 1531–1538
CNN (2017) http://edition.cnn.com/2017/06/26/asia/china-landslide-rescue-called-off/index.html. Accessed on 14 July 2017
Cui P, Zhu YY, Han YS, Chen XQ, Zhuang JQ (2009) The 12 may Wenchuan earthquake-induced landslide lakes: distribution and preliminary risk evaluation. Landslides 6:209–223. https://doi.org/10.1007/s10346-009- 0160-9
Dai FC, Lee CF, Deng JH, Tham LG (2005) The 1786 earthquake-triggered landslide dam and subsequent dam-break flood on the Dadu River, southwestern China. Geomorphology 65(3):205–221. https://doi.org/10.1016/j.geomorph.2004.08.011
Fan JR, Zhang XY, Su FH, Ge YG, Tarolli P, Yang ZY, Zeng C, Zeng Z (2017) Geometrical feature analysis and disaster assessment of the Xinmo landslide based on remote sensing data. J Mt Sci 14(9):1677–1688
Ferretti A, Prati C, Rocca F (2001) Permanent scatterers in SAR interferometry. IEEE Trans Geosci Remote Sens 39(1):8–20. https://doi.org/10.1109/36.898661
Ferretti A, Monti-Guarnieri A, Prati C, Rocca F, Massonnet D (2007) InSAR principles: guidelines for SAR interferometry processing and interpretation. ESA Publications, TM-19. ISBN 92-9092-233-8. Available at: http://www.esa.int/About_Us/ESA_Publications
Ferretti A, Fumagalli A, Novali F, Prati C, Rocca F, Rucci A (2011) A new algorithm for processing interferometric data-stacks: SqueeSAR. IEEE Trans Geosci Remote Sens 49(9):3460–3470. https://doi.org/10.1109/TGRS.2011.2124465
Fielding EJ, Blom RG, Goldstein RM (1998) Rapid subsidence over oil fields measured by SAR interferometry. Geophys Res Lett 25:3215–3218
Frodella W, Ciampalini A, Gigli G, Lombardi L, Raspini F, Nocentini M, Scardigli C, Casagli N (2016) Synergic use of satellite and ground based remote sensing methods for monitoring the san Leo rock cliff (northern Italy). Geomorphology 264:80–94
Froese CR, Moreno F (2014) Structure and components for the emergency response and warning system on Turtle Mountain, Alberta, Canada. Nat Hazards 70(3):1689–1712. https://doi.org/10.1007/s11069-011- 9714-y
Froese CR, Murray C, Cavers DS, Anderson WS, Bidwell AK, Read RS, Cruden DM, Langenberg W (2005) Development and implementation of a warning system for the south peak of Turtle Mountain. Hungr O, In
Fukuzono T (1985) A new method for predicting the failure time of slopes. Proceedings of the 4th International Conference & Field Workshop on Landslides, Tokyo, pp 145–150
Geudtner D, Torres R, Snoeij P, Davidson M, Rommen B (2014) Sentinel-1 system capabilities and applications. In Geoscience and remote sensing symposium (IGARSS), IEEE International, Quebec, pp. 1457–1460
Gigli G, Intrieri E, Lombardi L, Nocentini M, Frodella W, Balducci M, Venanti L, Casagli N (2014) Event scenario analysis for the design of rockslide countermeasures. J Mt Sci 6(11):1521–1530. https://doi.org/10.1007/s11629-014- 3164-4
Guzzetti F, Peruccacci S, Rossi M, Stark CP (2007) Rainfall thresholds for the initiation of landslides in central and southern Europe. Meteorog Atmos Phys 98(3):239–267. https://doi.org/10.1007/s00703-007-0262-7
Herrera G, Notti D, Garcıa-Davalillo JC, Mora O, Cooksley G, Sanchez M, Arnaud A, Crosetto M (2011) Landslides analysis with C- and X-band satellite SAR data: the Portalet landslide area. Landslides 8:195–206
Hilley G, Bürgmann R, Ferretti A, Novali F, Rocca F (2004) Dynamics of slow-moving landslides from permanent Scatterer analysis. Science 304:1952–1955
Huang RQ (2009) Some catastrophic landslides since the twentieth century in the southwest of China. Landslides 6:69–81. https://doi.org/10.1007/s10346-009- 0142-y
Intrieri E, Gigli G (2016) Landslide forecasting and factors influencing predictability. Nat Hazards Earth Sci Syst 16(12):2501–2510. https://doi.org/10.5194/nhess-2016-221 Available at: http://www.nat-hazards-earth-syst-sci.net/16/2501/2016/nhess-16-2501-2016.pdf
Intrieri E, Gigli G, Mugnai F, Fanti R, Casagli N (2012) Design and implementation of a landslide early warning system. Eng Geol 147-148:124–136. https://doi.org/10.1016/j.enggeo.2012.07.017
Intrieri E, Gigli G, Casagli N, Nadim F (2013) Brief communication: landslide early warning system: toolbox and general concepts. Nat Hazards Earth Syst Sci 13:85–90. https://doi.org/10.5194/nhess-13- 85-2013
Jiang HC, Mao X, Xu HY, Yang HL, Ma XL, Zhong N, Li YH (2014) Provenance and earthquake signature of the last deglacial Xinmocun lacustrine sediments at Diexi, East Tibet. Geomorphology 204:518–531. https://doi.org/10.1016/j.geomorph.2013.08.032
Kalia AC, Frei M, Lege T (2017) A Copernicus downstream-service for the nationwide monitoring of surface displacements in Germany. Remote Sens Environ. https://doi.org/10.1016/j.rse.2017.05.015
Kampes, B.M. (2006) Radar interferometry—persistent scatterer technique. Springer. ISBN-10 1-4020-4576-X (HB)
Komac M, Holley R, Mahapatra P, van der Marel H, Bavec M (2015) Coupling of GPS/GNSS and radar interferometric data for a 3D surface displacement monitoring of landslides. Landslides 12(2):241–257
Lacasse S Nadim F (2008) Landslide risk assessment and mitigation strategy. In the First World LandslideForum, United Nations University, Tokyo, Japan, pp 31–61
Lagomarsino D, Segoni S, Fanti R, Catani F (2013) Updating and tuning a regional-scale landslide early warning system. Landslides 10(1):91–97. https://doi.org/10.1007/s10346-012- 0376-y
Li T (1983) A mathematical model for predicting the extent of a major rockfall. Z Geomorphol 27(4):473–482
Livemint (2017) http://www.livemint.com/Politics/SdBgBlRxzmQQL3L9bZiBhM/Second-landslide-hits-stricken-site-in-southwest-China.html. Accessed on 14 July 2017
Lu P, Casagli N, Catani F, Tofani V (2012) Persistent scatterers interferometry hotspot and cluster analysis (PSI-HCA) for detection of extremely slow-moving landslides. Int J Remote Sens 33(2):466–489
Lu P, Casagli N, Tofani V, Catani F (2014) Quantitative hazard and risk assessment for slow-moving landslides from persistent scatterer interferometry. Landslides 11(4):685–696
Massonnet D, Feigl KL, Rossi M, Adragna F (1994) Radar interferometric mapping of deformation in the year after the Landers earthquake. Nature 369:227–230
Michoud C, Bazin S, Blikra LH, Derron MH, Jaboyedoff M (2013) Experiences from site-specific landslide early warning systems. Nat Hazards Earth Syst Sci 13(10):2659–2673. https://doi.org/10.5194/nhess-13-2659-2013
Mufundirwa A, Fujii Y, Kodama J (2010) A new practical method for prediction of geomechanical failure. Int J Rock Mech Min Sci 47:1079–1090
Novellino A, Cigna F, Brahmi M, Sowter A, Bateson L, Marsh S (2017) Assessing the feasibility of a national InSAR ground deformation map of great Britain with Sentinel-1. Geosciences 7(2):19
Parsons T, Ji C, Kirby E (2008) Stress changes from the 2008 Wenchuan earthquake and increased hazard in the Sichuan basin. Nature 454:509–510
Prati C, Ferretti A, Perissin D (2010) Recent advances on surface ground deformation measurement by means of repeated space-borne SAR observations. J Geodyn 49(3):161–170
Raspini F, Moretti S, Casagli N (2013) Landslide mapping using SqueeSAR data: Giampilieri (Italy) case study. In: Landslide science and practice. Springer, Berlin Heidelberg, pp 147–154
Rickenmann D (2005) Runout prediction methods. In: Jakob M, Hungr O (eds) Debris-flow hazards and related phenomena. Praxis, Chichester, pp 305–324
Rose ND, Hungr O (2007) Forecasting potential rock slope failure in open pit mines using the inverse-velocity method. Int J Rock Mech Min Sci 44:308–320
Ruch C (2009) Georisiken. Aktive Massenbewegungen Albtrauf LGRB-Nachrichten 8:1–2 In German
Saito M (1969) Forecasting time of slope failure by tertiary creep. Proceedings, 7th international conference on soil mechanics and foundation engineering, Mexico City, 677–683
Singhroy V, Mattar KE, Gray AL (1998) Landslide characterisation in Canada using interferometric SAR and combined SAR and TM images. Adv Space Res 21:465–476
Thiebes B (2012) Landslide analysis and early warning systems: local and regional case study in the Swabian Alb. Springer Science & Business Media, Berlin
Tofani V, Raspini F, Catani F, Casagli N (2013) Persistent scatterer interferometry (PSI) technique for landslide characterization and monitoring. Remote Sens 5(3):1045–1065
Tomás R, Li Z, Lopez-Sanchez JM, Liu P, Singleton A (2016) Using wavelet tools to analyse seasonal variations from InSAR time-series data: a case study of the Huangtupo landslide. Landslides 13(3):437–450
Vecchiotti F, Peduto D, Strozzi T (2017) Multi-sensor a priori PSI visibility map for nationwide landslide detection in Austria. In: Workshop on world landslide forum. Springer, Cham, pp 45–52
Wang P, Zhang B, Qiu WL, Wang JC (2011) Soft-sediment deformation structures from the Diexi paleo-dammed lakes in the upper reaches of the Minjiang river, East Tibet. J Asian Earth Sci 40:865–872
Wang JJ, Zhao D, Liang Y, Wen HB (2013) Angle of repose of landslide debris deposits induced by 2008 Sichuan earthquake. Eng Geol 156:103–110
Xu Q, Fan XM, Huang RQ, Van Westen C (2009) Landslide dams triggered by the Wenchuan earthquake, Sichuan Province, south west China. Bull Eng Geol Environ 68(3):373–386
Yin Y, Wang F, Sun P (2009) Landslide hazards triggered by the 2008 Wenchuan earthquake, Sichuan, China. Landslides 6(2):139–152
Yu YF, Lam JS, Siu CK, Pun WK (2004). Recent advance in landslip warning system. Proceedings of the 1 day seminar on recent advances in geotechnical engineering, organized by the Hong Kong Institution of Engineers Geotechnical Division: 139–147
Acknowledgements
The authors gratefully acknowledge Planet for making available the post-event optical image used in this analysis and the Copernicus programme for the free access to Sentinel-1 and Sentinel-2 images processed in this analysis. The InSAR analysis has been self-financed by TRE ALTAMIRA.
Author information
Authors and Affiliations
Corresponding author
Rights and permissions
Open Access This article is distributed under the terms of the Creative Commons Attribution 4.0 International License (http://creativecommons.org/licenses/by/4.0/), which permits unrestricted use, distribution, and reproduction in any medium, provided you give appropriate credit to the original author(s) and the source, provide a link to the Creative Commons license, and indicate if changes were made.
About this article
Cite this article
Intrieri, E., Raspini, F., Fumagalli, A. et al. The Maoxian landslide as seen from space: detecting precursors of failure with Sentinel-1 data. Landslides 15, 123–133 (2018). https://doi.org/10.1007/s10346-017-0915-7
Received:
Accepted:
Published:
Issue Date:
DOI: https://doi.org/10.1007/s10346-017-0915-7