Abstract
Previous numerical simulations have suggested that the area adjacent to Itaipu Lake in Southern Brazil is significantly affecting the local thermal regime through development of a lake breeze. This has led to concerns that soybean growth and development, and consequently yield, has been affected by the creation of the artificial lake in this important agricultural region, but a systematic climatological study of the thermal effects of Itaipu Lake has not been conducted. The objectives of this study were to assess the spatial pattern of minimum and maximum air temperatures in a 10-km-wide area adjacent to Itaipu Lake as affected by distance from the water. Measurements were conducted over 3 years in seven transects along the shore of Itaipu Lake, with five weather stations placed in each transect. Phenological observations in soybean fields surrounding the weather stations were also conducted. Generalized additive models for location, scale, and shape (GAMLSS) analysis indicated no difference in the temperature time series as distance from water increased. Semivariograms showed that the random components in the air temperature were predominant and that there was no spatial structure to the signal. Wind direction measured over the three growing seasons demonstrated that, on average, the development of a lake breeze is limited to a few locations and a few hours of the day, supporting the temporal and spatial analysis. Phenological observations did not show differences in the timing of critical soybean stages. We suggest that the concerns that soybean development is potentially affected by the presence of Itaipu Lake are not supported by the thermal environment observed.
Similar content being viewed by others
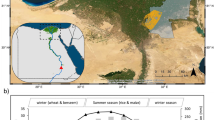
Avoid common mistakes on your manuscript.
Introduction
The moderating effect of large water bodies on air temperature is well-known and is responsible for local climates that allow for productive agriculture in their vicinity, as for example, in the Great Lakes region of North America (Sanderson 2004). Artificially created lakes for hydroelectric power generation have the potential of affecting local climate and generating lake breezes (Klaic and Kvakic 2014) potentially causing a shift in conditions for agriculture in adjacent lands. Lake breezes are driven by the differential heating of water and land and affected by the magnitude of the land’s sensible heat flux, geostrophic wind, water body dimensions, and surface roughness length amongst other geophysical variables (Crosman and Horel 2010).
Itaipu Lake is an artificial lake created on the Paraná river between Brazil and Paraguay when the Itaipu power generation facility became operational in 1982. The lake is 170 km long in the north-south direction, has an average width of 7 km (west-east), depth of 22 m for a total area of 1350 km2, and water volume of 29 × 109 m3. The lake perimeter on the Brazilian side is 1340 km long, and a protective vegetative strip with a mean width of 210 m surrounding the whole extension of the lake was established in 1982. Farmland composed of fertile soils in intensive agricultural production of soybeans, corn, and wheat is present beyond this vegetative strip. Due to the limited width of Itaipu Lake, its impact on the climate of the surrounding farm land should be limited, as onshore penetration of lake breezes is estimated at less than half of the lake width (Segal et al. 1997). However, recent Doppler radar observations of a small reservoir (mean width of ~2 km) concluded that local circulations are also generated by small lakes (Asefi-Najafabady et al. 2012). In addition, numerical simulation and limited measurements of air temperature over Itaipu Lake and at an adjacent land station concluded that the lake is inducing and sustaining a local circulation pattern (Stivari et al. 2003) and reducing the regional thermal amplitude (Stivari et al. 2005). However, numerical simulations with horizontal grid spacing >2 km used in most studies limit the interactions between geophysical variables (e.g., roughness length, sensible heat flux), so that predicted lake breeze onshore penetration might not be realistic (Crosman and Horel 2010). Nevertheless, results from previous studies on the effect of Itaipu Lake (Stivari et al. 2003, 2005) have led to concerns that thermal conditions for an important regional crop such as soybeans could be affected, and a systematic climatological study for the area is needed.
The objectives of this study were as follows: (1) to determine if the spatial pattern of minimum and maximum air temperatures in the area adjacent to Itaipu Lake is affected by distance from the water and (2) assess the effect of Itaipu Lake on available growing degree days and phenological stages of soybeans. Weather stations were installed in seven transects distributed approximately evenly in the north to south direction along the eastern shore of Itaipu Lake. Each transect was composed of five stations, placed at varying distances from the lake center (~300–1200 m to ~10 km), and measurements were conducted over 3 years. Spatial and temporal statistics were applied to the collected data to determine if the distance from the lake explained the variability in observed thermal environment. Our hypotheses were that stations closer to the lake would show different trends in time compared to stations further away from the lake and a spatial pattern in weather variables would be observed.
Material and methods
Site characterization
The climate in the western region of Paraná state, where Itaipu Lake is located (~25° S and 54° W, Fig. 1a), is classified as humid subtropical (Cfa, according to the Köppen classification; Alvares et al. 2014). Mean monthly air temperature is above 22 °C during the summer months (Dec–Mar) with a tendency of higher precipitation during these months but no defined dry season according to the Köppen classification (Alvares et al. 2014). Mean annual precipitation varies from 1600 to 2000 mm in the studied region (IAPAR 1994).
a Location of seven transects (each with five weather stations indicated by red triangles) along the eastern shore of Itaipu Lake, created due to the hydroelectric dam built on the Paraná river, Brazil. The legend shows the region’s altitude. b View of 200-m forested border that surrounds the whole lake on the eastern side (Brazil). c View of one of the weather stations
Weather stations were installed on the eastern shore of the Paraná river, along the Brazilian margin of Itaipu Lake, in seven transects located in the municipalities of Guaíra (GUA), Mercedes (MER), Pato Bragado (PBG), Santa Helena (two transects; one in the south, SHS, and one in the north, SHN), Itaipulândia (ITA), and Santa Terezinha de Itaipu (STI) (Fig. 1a). At each transect, areas of at least 1 ha were selected from commercial farms which were planted with the same cultivar of soybeans (cultivar V max). Five meteorological stations were installed in these areas along each transect (Table 1), with the closest stations at a distance between 320 and 1220 m and the furthest 9.32 to 11.33 km from the lake shore (Fig. 1b). These distances were chosen based on the suggestion that lake breeze onshore flow is generally less than half of lake width (Segal et al. 1997) (for Itaipu Lake, on average, 3.5 km and a maximum of 6 km). The weather stations were set up in a grassed area within soybean fields and fenced to avoid vandalism (Fig. 1c). The following criteria were observed when selecting the location for weather station installation: elevation <300 m, average slope <8 % toward west, and similar soil type (Latossolo Vermelho; Embrapa 2006) under no tillage, which is commonly used in this region.
Measurements started in October 2010 and continued until March 2013. The growing season for soybeans in this region is from October to February, and the analysis reported here was conducted for the October 2010 to February 2011, October 2011 to February 2012, and October 2012 to February 2013 periods. Dates of occurrence of soybean phenological stages were observed throughout each growing season following procedure by Fehr and Caviness (1977).
Weather measurements
Air temperature and relative humidity sensors (CS215, Campbell Scientific) were mounted inside a radiation shield (41003-5A, Campbell Scientific) on a tower at a height of 2 m. The sensor characteristics are as follows: range −39.2 °C to +60 °C and accuracy for minimum values of ±0.2 °C and for maximum values of ±0.5 °C, with a resolution of 0.1 °C. A data logger (CR1000, Campbell Scientific) was programmed to take readings every 10 s and store values every 1 min and save hourly maximum and minimum values. Daily maximum and minimum values were obtained over 24 h. Additional measurements consisted of wind speed and direction (03002-L34 RM Young) and incoming solar radiation (LI200X-L12, LI-COR).
Temporal statistical analysis
Data collected at each weather station was individually analyzed for temporal trends, and the fitted trends for stations within each transect were compared. Mean minimum and maximum air temperatures over 5 days for 150 days starting on October 1 of each year (2010, 2011, 2012) were obtained for the 35 weather stations. The 5-day mean was used to minimize the autocorrelation between observations according to the Durbin-Watson test, as determined by the function suggested by Zeileis and Hothorn (2002). Models were fitted to each time series (30 data points for each of 35 sites) using the generalized additive models for location, scale and shape (GAMLSS) tool proposed by Rigby and Stasinopoulos (2005). In a GAMLSS model, the observations Y i (for i = 1,…, n; where i = time) are assumed to be independent in time with a distribution function F Y (y i ; θ i ), where θ i = (θ i1,…, θ im ) represents a vector of m distribution parameters accounting for position, scale, and shape. Monotonic link functions g k (·) (k = 1,…, m) relate the parameters of the distribution to the design matrix of the selected covariate (in this case, time t). In this study, we considered six distributions: Gumbel, Weibull, gamma, logistic, lognormal, and normal. Each had two fitted parameters (theta1 and theta2), where theta1 is associated with location and theta2 with scale. A parametric formulation was assumed for the GAMLSS (Rigby and Stasinopoulos 2005) with theta1 described as a function of time (t). The Akaike information criterion (AIC) was used to select the best fit model (Akaike 1974). The quality of the fitting procedure was evaluated by the residual statistics, the Filliben correlation coefficient (Filliben 1975), and visual inspection of worm plots (Buuren and Fredriks 2001). Model parameters were fitted using GAMLSS written in R software (R Core Team 2014). Comparison of model parameters for stations within a transect was performed to determine the existence of significant differences in temporal trends in minimum and maximum air temperatures at varying distances from the lake, for each growing season, using the confidence intervals calculated using the BSagri package (Schaarschmidt 2013).
Spatial statistical analysis
Semivariograms are used in geostatistics to model how a property changes in space. Assuming that there is spatial continuity between adjacent samples, the correlation between spatially adjacent variables is measured (or modeled) with a semivariogram function. For example, a set of n measurements of a property A i taken at locations x i which are placed at certain interval along a transect will give a variance of
where Ā is the mean for A i for all locations. If we apply this to N pairs of values of A i separated by a distance h, we have the semivariogram γ(h):
Semivariogram functions can have different shapes depending mostly on the nature of spatial variability of variable A i . We were interested in determining if air temperature measured along each transect of five weather stations (Table 1) varied independently or according to a determined spatial pattern. If the former was the case, a pure nugget semivariogram would be obtained indicating a lack of spatial pattern along each transect.
Experimental semivariograms along with their models and sample variance for five variables (minimum and maximum air temperatures, relative humidity, solar radiation, and growing degree days (GDD), for base temperature of 10 °C accumulated from emergence to specific phenological stages) were calculated. Each of these variables corresponded to A i in Eqs. 1 and 2. The distance h in Eq. 2 corresponded to the difference in horizontal distance between all possible pairs of stations (1–2, 1–3, 1–4, 1–5, 2–3, 2–4, 2–5, 3–4, 3–5, 4–5). The selected phenological stages of soybean development were as follows: Ve, emergence; R1, beginning bloom; R6, beginning physiological maturity; and R8, full maturity) for the three growing seasons.
All calculations were done using R (R Core Team 2014). As part of an exploratory analysis, tests were performed to confirm that the datasets passed normality tests and that variables did not need to be transformed. A preliminary analysis under a spatiotemporal geostatistical framework (Pebesma 2012; Bivand et al. 2013) did not yield any spatiotemporal relationship, and further work using this approach was not continued.
Semivariograms were calculated using the function “variogram” from the gstat package (Pebesma 2004; Pebesma and Bivand 2005) calculated first using coordinates easting and northing for each transect location and then adding elevation as a coordinate. As results did not differ, only easting and northing were used to define the spatial location of each meteorological variable. Each semivariogram was calculated using meteorological data from 5 days around the date of occurrence of each selected phenological stage (a given date plus 2 days previous and 2 days following) for each site in order to increase the sample number (from one to five). As this results in multiple values of a variable for each location, a small shift was randomly added to the easting and northing coordinates (less than 1 mm) for each date because the algorithm used in gstat does not allow for repeated measurements on the same location.
An omnidirectional linear model with nugget was fitted to the experimental semivariogram using weighted least squares (weight N h × h − 2, with N h the number of point pairs and h the distance) using as starting values the variance of the sample, V (nugget = V × 0.9, partial sill of the linear model = V × 0.01, and range = 100).
Results
Temporal analysis
An overview of the minimum and maximum air temperature data recorded at all stations is given in Fig. 2. Medians are shown, as datasets were not normally distributed. No clear trends were observed between stations in any of the study years. The 5-day minimum and maximum means showed warming between October and February as expected and similar trends for the five stations in each transect (Figs. 3 and 4). Normal or Gumbel was the best fit model selected according to the AIC (data not shown) for all stations and years using the GAMLSS tool, with good quality of residual statistics as given by the Filliben correlation coefficient (>0.97) and worm plot inspection. The time series model adjusted for each station showed clear temporal trends, but model parameters (slope, intercept, sigma) did not show consistent differences between stations within a transect for minimum (Table 2) or maximum temperature (data not shown). For example, slopes were nearly identical and the difference in model intercept between stations varied from 0.5 °C (stations 3 and 5, MER in 2010/2011) to 2.7 °C (stations 3 and 5, SHS in 2010/2011). The weather stations with the highest intercept tended to be station 2 or 3, that is, located 3.4 to 5.5 km from the lakeshore, while the ones with the lowest intercept were located at distances between 7.3 and 11.8 km (stations 4 and 5). Over the three growing seasons, mean differences were 1.0, 0.9, 0.7, 1.5, 1.8, 1.0, and 0.9 °C between the largest and smallest intercepts for sites GUA, MER, PBG, SHN, SHS, ITA, and STI, respectively, but these values were not significantly different.
Median values for maximum air temperature (empty symbols) and minimum air temperature (closed symbols) recorded at each transect at varying distances from Itaipu Lake over the three growing seasons (2010/2011 (circles), 2011/2012 (diamonds), 2012/2013 (squares)). X values are slightly offset for each year for the sake of clarity. Error bars indicate 95 % confidence interval
Minimum air temperature averaged over 5 days during the soybean growing season (Oct 1 to Feb 28) for Guaíra (GUA) (a–c), Santa Helena do Norte (STN) (d–f), and Santa Terezinha de Itaipu (STI) (g–i), respectively, the most northerly, middle, and southerly location studied. Different symbols indicate the five transects at each location. The left panel shows year 2010/2011, middle panel 2011/2012, and right panel 2012/2013 data
Maximum air temperature averaged over 5 days during the soybean growing season (Oct 1 to Feb 28) for Guaíra (GUA) (a–c), Santa Helena do Norte (STN) (d–f), and Santa Terezinha de Itaipu (STI) (g–i), respectively, the most northerly, middle, and southerly location studied. Different symbols indicate the five transects at each location. The left panel shows year 2010/2011, middle panel 2011/2012, and right panel 2012/2013 data
Spatial analysis
Spatial analysis of all variables (minimum and maximum temperatures, relative humidity, solar radiation, and GDD) for all transects and all growing seasons studied showed a flat experimental semivariogram described by two models, nugget or nugget + linear equation. An example of a semivariogram obtained for these variables (except solar radiation) and one location and time is shown in Fig. 5. The variance explained by the model (partial sill) and variance not explained by the model (nugget, or randomness of measured variable) obtained for GDD at each site and different phenological stages during the study years are shown in Table 3. The nugget corresponded from 87 to 92 % of the total variance for all semivariograms analyzed, and the partial sill was negligible. Semivariograms obtained for the other meteorological variables produced very similar results and are not shown.
Semivariogram for a maximum and b minimum air temperatures, c relative humidity, and d growing degree days obtained using data for 5 days around the seed filling stage (R6) for the transect in Santa Terezinha de Itaipu (STI). u 2 refers to the units of each measurement variables squared (°C2 for temperature, %2 for relative humidity, and (°C day−1)2)
Discussion
The derived intercept and slope of the times series models for all seasons studied showed the same trends for each station, that is, no significant difference in the fitted parameters, indicating similarity in the temperature time series between stations within the 10-km transect. This was contrary to the expected trend of decreasing minimum (increasing maximum) air temperature with distance from lakeshore typically observed for larger water bodies (Im et al. 2009). Likely large-scale synoptic factors had an overriding effect producing similar air temperature conditions at all stations. In a study of the representativeness of European climate stations, Orlowsky and Seneviratne (2014) found high-rank-based correlations between stations in regions where large-scale circulation controls daily variability in air temperature.
Semivariograms for meteorological variables yielded models with large nuggets, almost equal to the variance, which is indicative of a lack of spatial structure; this was the case for all sites, years, and phenological stages analyzed (Table 3). This means that there was a lack of spatial pattern in the measured transects. Nugget values up to approximately 30 % of the total variance are expected for nonrandom spatial patterns (Wackernagel 2003). In our case, if the distance from the lake was the main predictive variable, much smaller nuggets would have been obtained for each transect. Janis and Robeson (2004) used a point-centered semivariogram, applied to time-averaged diurnal temperature range, to determine how well 301 weather stations within the central USA represented climate over large spatial scales. They found significant spatial patterns with small nuggets for the majority of the stations, indicating that stations were spatially representative of the large-scale climate. The scale of our study differs significantly from this analysis, given that the spatial separation of our stations within each transect was much smaller. However, Burcsu et al. (2001) demonstrated the use of semivariograms applied to remote sensing data to a somewhat similar question in forests, that is, identification of the distance within a transect where edge effects of a clearing propagated into a surrounding forest. We did not find any published applications of geostatistics to weather station transects, probably because this is an unusual configuration, not found in climate networks.
The lack of spatial structure and high correspondence of time series models fitted to observations indicate that distance from Itaipu Lake does not have a significant differentiating effect on the thermal environment of adjacent land. Indeed, phenological observations confirmed that there was no difference in the thermal regime between transects as stages occurred approximately at the same time with very little variation between stations at a given site (Table 4).
The lack of temperature gradient induced by the lake presence can be explained by a weak to nonexistent lake breeze for most of the measurement period. Wind direction and speed according to time of day averaged over the three growing seasons (Fig. 6) show predominant wind directions from the east or northeast, which is consistent with large-scale average synoptic patterns (Wagner et al. 1989). A switch to south/southwest direction in the afternoon, consistent with the development of a lake breeze, can be observed at some of the locations (e.g., PBG). However, this lake breeze was of limited duration and clearly did not affect the overall thermal regime of the area surrounding the lake. The land surface sensible heat flux, ambient geostrophic wind, water body dimensions, and surface aerodynamic resistance are some of the geophysical variables that affect the development of sea and lake breezes (Crosman and Horel 2010). Strong land surface heating during daytime, and the associated sensible heat flux, will result in the horizontal temperature gradient required for lake breeze development. We suggest that intensive latent heat flux associated with soybean evapotranspiration would induce lower sensible heat fluxes than required for strong lake breeze development. In addition, the presence of the 200-m vegetative strip with a tall canopy of large aerodynamic roughness length would also be detrimental for lake breeze development as the frictional drag works to diminish the development of horizontal pressure gradients associated with lake breezes (Crosman and Horel 2010). Finally, the small width (~7 km) of the water body is another factor, as dimensions of the water body have a strong effect on lake breeze development.
Our results somewhat contradict previous analysis which suggested that the Itaipu Lake reduced the amplitude of the diurnal air temperature regionally (Stivari et al. 2005). However, this conclusion was based on comparison of temperature measured over the lake with land stations and air temperature temporal trends (before and after lake formation) that could have been confounded with other climatic trends. In a previous study, Stivari et al. (2005) found evidence that Itaipu Lake induced daytime lake breezes; however, authors cautioned that it is difficult to reproduce average circulation patterns with the numerical model used. Ideally, future studies could investigate the mechanisms controlling lake breeze development in the Itaipu Lake region through detailed measurements of surface-atmosphere energy exchange over contrasting land use, using ground-based flux towers and airborne flux measurements combined with numerical models (e.g., Samuelsson and Tjernström 2001). Contrasting land use on the Paraguayan and Brazilian side of Itaipu Lake provides further opportunities for testing the effect of controlling variables such as surface roughness and land sensible heat flux.
Conclusions
Detailed measurements of air temperature over three soybean growing seasons in transects adjacent to Itaipu Lake did not show a significant effect of distance from shore as shown by temporal and spatial analysis. The GAMLSS analysis allowed for adjustment of models to the time series obtained at each weather station, but fitted parameters indicated no difference in the time series as distance from lake increased. The semivariograms showed that the random components in the air temperature signal were predominant and that there was no spatial structure to the signal. Average wind direction measured over the three growing seasons demonstrated that the development of a lake breeze is limited to a few locations and a few hours of the day, supporting the spatial and temporal analyses. Soybean phenological observations are in agreement with these analyses, as no differences in the occurrence of significant stages were observed.
References
Akaike H (1974) A new look at the statistical model identification. IEEE Trans Autom Control 19(6):716–723
Alvares CA, Stape JL, Sentelhas PC, Goncalves JLM, Sparovek G (2014) Meteorol. Zeit 22(6):711–728
Asefi-Najafabady S, Knupp K, Mecikalski JR, Welch RM (2012) Radar observations of mesoscale circulations induced by a small lake under varying synoptic-scale flows. J Geophys Res 117. doi:10.1029/2011JD016194
Bivand RS, Pebesma E, Gomez-Rubio G (2013) Applied spatial data analysis with R, 2nd edn. Springer, NY
Burcsu TK, Robeson SM, Meretsky VJ (2001) Identifying the distance of vegetative edge effects using Landsat TM data and geostatistical methods. Geocarto Int 16:61–70
Crosman ET, Horel JD (2010) Sea and lake breezes: a review of numerical studies. Boundary-Layer Meteorol 137:1–29
Embrapa (2006) Sistema brasileiro de classificação de solos. Centro Nacional de Pesquisa de Solos. Rio de Janeiro, Brasil, 412p
Fehr WR, Caviness CE (1977) Stages of soybean development. Ames: Iowa State University of Science and Technology, 11 p. (Special Report, 80)
Filliben JJ (1975) The probability plot correlation coefficient test for normality. Technometrics 17(1):111–117
Janis M, Robeson S (2004) Determining the spatial representativeness of air-temperature records using variogram-nugget time series. Phys Geogr 25(6):513–530
Klaic ZB, Kvakic M (2014) Modeling the impacts of a man-made lake on the meteorological conditions of the surrounding areas. J Appl Meteorol Climatol 53:1121–1142
Iapar (1994) Cartas climáticas do Estado do Paraná. 49 p. (IAPAR, Documento 18)
Im H-K, Rathouz PJ, Frederick JE (2009) Space–time modeling of 20 years of daily air temperature in the Chicago metropolitan region. Environmetrics 20:494–511
Orlowsky B, Seneviratne SI (2014) On the spatial representativeness of temporal dynamics at European weather stations. Int J Climatol 34:3154–3160
Pebesma EJ (2004) Multivariable geostatistics in S: the gstat package. Comput Geosci 30:683–691
Pebesma EJ (2012) Spacetime: spatio-temporal data in R. J Statist Soft 51(7):1–30
Pebesma EJ, Bivand RS (2005) Classes and methods for spatial data in R. R News 5(2) http://cran.r-project.org/doc/Rnews/
R Core Team (2014) R: a language and environment for statistical computing. R Foundation for Statistical Computing, Vienna, Austria. http://www.R-project.org/
Rigby RA, Stasinopoulos DM (2005) Generalized additive models for location, scale and shape (with discussion). Appl Statist 54:507–554
Samuelsson P, Tjernström M (2001) Mesoscale flow modification induced by land-lake surface temperature and roughness differences. J Geophys Res 106(D12):12,419–12,435
Sanderson M (2004) Weather and climate in Southern Ontario. University of Waterloo. Dept. of Geography Publication Series no. 58, 126 p.
Schaarschmidt F (2013) BSagri: statistical methods for safety assessment in agricultural field trials. R package version 0.1-8. http://CRAN.R-project.org/package=BSagri
Segal M, Leuthold M, Arritt RW, Anderson C, Shen J (1997) Small lake daytime breezes: some observational and conceptual evaluations. Bull Am Meteorol Soc 78(6):1135–1147
Stivari SMS, de Oliveira AO, Karam HA, Soares J (2003) Patterns of local circulation in the Itaipu lake area: numerical simulations of lake breeze. J Appl Meteorol 42:37–50
Stivari SMS, De Oliveira AP, Soares J (2005) On the climate impact of the local circulation in the Itaipu Lake area. Clim Chang 72:103–121
Wackernagel H (2003) Multivariate geostatistics: an introduction with applications. Springer, Berlin, Germany
Wagner CS, Bernardes, LRM, Correa AR, Borrozzino E (1989) Velocity and predominant direction of winds in Parana State (in Portuguese). Londrina, IAPAR, 56 p, IAPAR, Technical Bulletin 26
Zeileis A, Hothorn T (2002) Diagnostic checking in regression relationships. R News 2(3):7–10, http://CRAN.R-project.org/doc/Rnews/
Acknowledgments
This project was funded by the FAPEAGRO and Instituto Agronomico do Parana, Londrina, Paraná, Brazil.
Author information
Authors and Affiliations
Corresponding author
Rights and permissions
Open Access This article is licensed under a Creative Commons Attribution 4.0 International License, which permits use, sharing, adaptation, distribution and reproduction in any medium or format, as long as you give appropriate credit to the original author(s) and the source, provide a link to the Creative Commons licence, and indicate if changes were made.
The images or other third party material in this article are included in the article’s Creative Commons licence, unless indicated otherwise in a credit line to the material. If material is not included in the article’s Creative Commons licence and your intended use is not permitted by statutory regulation or exceeds the permitted use, you will need to obtain permission directly from the copyright holder.
To view a copy of this licence, visit https://creativecommons.org/licenses/by/4.0/.
About this article
Cite this article
Wagner-Riddle, C., Werner, S.S., Caramori, P. et al. Determining the influence of Itaipu Lake on thermal conditions for soybean development in adjacent lands. Int J Biometeorol 59, 1499–1509 (2015). https://doi.org/10.1007/s00484-015-0960-7
Received:
Revised:
Accepted:
Published:
Issue Date:
DOI: https://doi.org/10.1007/s00484-015-0960-7