Abstract
A long-term multichannel electroencephalogram recording plays a crucial role in recognizing the epileptic seizure activities from the brain lobes. This research study investigates the automated detection of epileptic seizures from multichannel electroencephalogram recordings using Teager energy feature. A supervised back-propagation neural network model was implemented to classify the inter-ictal seizures. The study was conducted on multichannel electroencephalogram data that was obtained from Institute of Neuroscience, Ramaiah Memorial Hospital, Bengaluru, India, after ethical clearance from the from the Institutional Ethics Board. Initially, notch filter was applied to remove the 50 Hz power line noise from raw electroencephalogram followed by independent component analysis to remove eye blinks and muscular activities. A time domain feature called Teager energy was estimated which detects the rapid changes in the given electroencephalogram time series. A 1 s windowing was introduced to ensure stationarity for estimation of Teager energy. The descriptive and box plot analysis ensures the suitability of the Teager energy for the seizure detection. The performance of the multilayer perceptron neural network classifier was evaluated using sensitivity, specificity, and false detection rate. Simulation results showed the highest sensitivity, specificity and false detection rate of 96.66%, 99.15%, and 0.30 per hour respectively. It can be concluded that procedure can be applied for real-time seizure detection.





Similar content being viewed by others
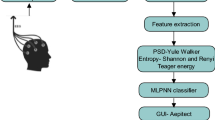
References
New to seizures and Epilepsy, Epilepsy Foundation (2017) https://www.epilepsy.com/learn/about-epilepsy-basics/what-epilepsy. Accessed 5 Jan 2018
EEG (2017) https://www.epilepsy.com/learn/diagnosis/eeg. Accessed 5 Jan 2018
Dabye AS, Issaka MA, Gueye L (2015) Localization of epileptic seizure with an approach based on the PSD with an autoregressive model. arXiv preprint arXiv:1506.00947
Kamath C (2013) A new approach to detect epileptic seizures in electroencephalograms using Teager energy. ISRN Biomed Eng. https://doi.org/10.1155/2013/358108
Herta J et al (2015) Prospective assessment and validation of rhythmic and periodic pattern detection in NeuroTrend: a new approach for screening continuous EEG in the intensive care unit. Epilepsy Behav 49:273–279
Abbassi R, Esmaielpour E (2017) Selecting statistical characteristics of brain signals to detect epileptic seizures using discrete wavelet transform and perceptron neural network. Int J Interact Multimed Artif Intell 4:33–38
Zhou W, Liu Y, Yuan Q, Li X (2013) Epileptic seizure detection using lacunarity and Bayesian linear discriminant analysis in intracranial EEG. IEEE Trans Biomed Eng 60:3375–3381
Xia YS, Leung H (2006) Nonlinear spatial-temporal prediction based on optimal fusion. IEEE Trans Neural Netw 17:975–988
Guruva Reddy A, Narava S (2013) Artifact removal from EEG signals. Int J Comput Appl 77(13):1–3
Kamath C (2013) Teager energy based filter-bank Cepstra in EEG classification for seizure detection using radial basis function neural network. ISRN Biomedical Engineering, Hindawi. https://doi.org/10.1155/2013/498754
Samiee K, Kovacs P, Gabbouj M (2015) Epileptic seizure classification of EEG time-series using rational discrete short-time Fourier transform. IEEE Trans Biomed Eng 62:541–552
Zeng K, Jiaqing Y, Yinghua W (2016) Automatic detection of absence seizures with compressive sensing EEG. Neurocomputing 171:497–502. https://doi.org/10.1016/j.neucom.2015.06.076
Venkataraman V (2014) Brain dynamics based automated epileptic seizure detection. Annual International Conference of the IEEE Engineering in Medicine and Biology Society, Chicago, pp 946–949. https://doi.org/10.1109/EMBC.2014.6943748
Ratham H et al (2016) Classification of epileptic EEG signals based on simple random sampling and sequential feature selection. Brain Inform 3:85–91
Koren J et al (2005) Prediction of rhythmic and periodic EEG patterns and seizures on continuous EEG with early epileptiform discharges. Epilepsy Behav 49:286–289. https://doi.org/10.1016/j.yebeh.2015.04.044
Agustina GC, Lorena O, Pablo D, Eric L (2015) Automatic detection of epileptic seizures in long-term EEG records, Universidad Nacional de San Juan (UNSJ), San Juan, Argentina. Comput Biol Med 57:66–73
Helal AEM, Seddi AF, Eldosoky M, Hussein AAF (2014) An efficient method for epileptic seizure detection in long-term EEG recordings. J Biomed Sci Eng 7:963–972
Li M, Cui Y, Yang J (2013) Automatic removal of ocular artifact from EEG with DWT and ICA method. Appl Math Inform Sci 7:809–816
Besio WG et al (2014) High-frequency oscillations recorded on the scalp of patients with epilepsy using tripolar concentric ring electrodes. IEEE J Trans Eng Health Med 2:1–11
Zandi AS, Javidan M, Dumont GA, Tafreshi R (2010) Automated real-time epileptic seizure detection in scalp EEG recordings using an algorithm based on wavelet packet transform. IEEE Trans Biomed Eng 57:1639–1651
Shoeb A et al (2004) Patient-specific seizure onset detection. Epilepsy Behav 5:483–498
Bhattacharyya S et al (2011) Feature selection for automatic burst detection in the neonatal electroencephalogram. IEEE J Emerg Select Top Circuits Syst 1:469–479
Shen CP (2013) A physiology-based seizure detection system for multichannel EEG. PLoS ONE 8:1–9
Ji Z et al (2015) An automatic spike detection system based on elimination of false positives using the large-area context in the scalp EEG. IEEE Trans Biomed Eng 58:2478–2488
Raghu S, Sriraam N, Pradeep Kumar G, Hegde AS (2018) A novel approach for real time recognition of epileptic seizures using minimum variance modified fuzzy entropy. IEEE Trans Biomed Eng. https://doi.org/10.1109/TBME.2018.2810942
Raghu S, Sriraam N (2017) Optimal configuration of multilayer perceptron neural network classifier for recognition of intracranial epileptic seizures. Expert Syst Appl 89:205–222
Raghu S, Sriraam N, Pradeep Kumar G (2016) Classification of epileptic seizures using wavelet packet log energy and norm entropies with recurrent Elman neural network classifier. Cognit Neurodyn 11:51–66
Probability and Statistics (2014) http://www.statisticshowto.com/probability-and-statistics/z-score. Accessed 5 Jan 2018
Acknowledgements
The authors would like to thank the doctors, Institute of Neuroscience, Ramaiah Memorial Hospital, Bengaluru, India, for granting permission to use the EEG data. We would also like to acknowledge them for their constant support in data annotation and effective discussions on epilepsy.
Author information
Authors and Affiliations
Corresponding author
Ethics declarations
Conflict of interest
The authors declare that they have no conflict of interest.
Ethical approval
The proposed study makes use EEG from Ramaiah Memorial College and Hospitals, Bengaluru, India, after appropriate ethical clearance was taken.
Rights and permissions
About this article
Cite this article
Sriraam, N., Tamanna, K., Narayan, L. et al. Multichannel EEG based inter-ictal seizures detection using Teager energy with backpropagation neural network classifier. Australas Phys Eng Sci Med 41, 1047–1055 (2018). https://doi.org/10.1007/s13246-018-0694-z
Received:
Accepted:
Published:
Issue Date:
DOI: https://doi.org/10.1007/s13246-018-0694-z