Abstract
Background
Gastroparesis is a major complication following complete mesocolic excision (CME) and significantly impacts patient outcomes. This study aimed to create a machine learning model to pinpoint key risk factors before, during, and after surgery, effectively predicting the risk of gastroparesis after CME.
Methods
The study involved 1146 patients with colon cancer, out of which 95 developed gastroparesis. Data were collected on 34 variables, including demographics, chronic conditions, pre-surgery test results, types of surgery, and intraoperative details. Four machine learning techniques were employed: extreme gradient boosting (XGBoost), random forest (RF), support vector machine (SVM), and k-nearest neighbor (KNN). The evaluation involved k-fold cross-validation, receiver operating characteristic (ROC) analysis, calibration curves, decision curve analysis (DCA), and external validation.
Results
XGBoost excelled in its performance for predictive models. ROC analysis showed high accuracy for XGBoost, with area under the curve (AUC) scores of 0.976 for the training set and 0.906 for the validation set. K-fold cross-validation confirmed the model's stability, and calibration curves indicated high predictive accuracy. Additionally, DCA highlighted XGBoost’s superior patient benefits for intervention treatments. An AUC of 0.77 in external validation demonstrated XGBoost's strong generalization ability.
Conclusion
The XGBoost-fueled predictive model for post-surgery colon cancer patients proved highly effective. It underlined gastroparesis as a significant post-operative issue, associated with advanced age, prolonged surgeries, extensive intraoperative blood loss, surgical techniques, low serum protein levels, anemia, diabetes, and hypothyroidism.
Similar content being viewed by others
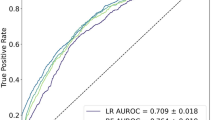
Explore related subjects
Discover the latest articles, news and stories from top researchers in related subjects.Avoid common mistakes on your manuscript.
1 Introduction
Colon cancer is a malignant neoplasm originating from the epithelial cells of the colonic mucosa. According to statistics, the annual incidence of new colon cancer cases worldwide is approximately 8 million, accounting for over one-tenth of all new malignancies. With global population aging and modern dietary habits shifting, the incidence of colon cancer is expected to continue to increase [1]. Currently, various medical institutions employ distinct treatment modalities for colorectal cancer, with radical surgery still being the primary approach for colon cancer management. This method ensures complete mesocolic excision and adheres strictly to the principle of radical tumor treatment [2]. A retrospective study by Pedrazzani confirmed the efficacy of complete mesocolic excision (CME) in reducing the recurrence rate of colon cancer and prolonging patient survival [3]. Nevertheless, postoperative complications following CME still affect patients' prognostic outcomes, such as abdominal infections, intestinal obstruction, anastomotic leakage, and gastroparesis. Among these complications, gastroparesis may be easily overlooked and misdiagnosed. Such patients often present with nonspecific gastrointestinal symptoms such as postprandial nausea and vomiting, further complicating diagnosis [4]. Multiple studies have established that gastroparesis occurrence in postoperative patients increases the risk of tumor recurrence and metastasis [5,6,7]. Furthermore, similar to other complications, gastroparesis results in prolonged hospitalization and has emerged as a progressively daunting public health issue globally [8]. As some gastroparesis patients may experience malnutrition and a decrease in daily activities, it may lead to decreased household income and an increase in the financial burden on the patient's family. Therefore, accurately predicting the occurrence of gastroparesis after total colectomy and identifying patients at high risk is of utmost importance.
Typically, surgeons rely on their clinical experience and examination reports to evaluate the risk of gastroparesis in surgical patients, but this approach has limitations. First, clinical experience is subject to the surgeon's subjective factors and may not accurately predict disease occurrence. Additionally, postoperative examination reports are often delayed, which may impact timely treatment of the patient's condition. Some clinicians use linear models and logistic regression for risk factor studies of postoperative gastroparesis to ensure prediction accuracy [9]. However, postoperative complications result from multifactorial interactions and not a single clinical variable. Furthermore, inadequate sample sizes in the regression model may introduce significant bias to the prediction results. These limitations in regression models have prompted clinical researchers to acknowledge their inadequacy in addressing issues related to clinical disease prediction.
In recent times, the medical community has seen a significant rise in the use of artificial intelligence, which effectively merges computer and data science [10]. Machine learning, a division of artificial intelligence, offers a way to objectify classification criteria, thus enhancing the reliability and validity of disease prediction [11, 12]. State-of-the-art machine learning models can establish the relationship between variables and outcomes using large amounts of data, usually with better stability and accuracy than traditional statistical analysis [13,14,15]. In this study, we analyzed clinical data from patients with colon cancer to develop a machine learning model for predicting the risk factors for developing gastroparesis after CME for colon cancer. The model was capable of identifying individuals at a high risk of developing gastroparesis following colon cancer surgery without relying on conventional imaging (such as abdominal computed tomography scans), thereby reducing healthcare costs.
2 Materials and methods
2.1 Data collection and criteria for post-surgical study
In this research, clinical data were obtained from the Wuxi People's Hospital database, under Nanjing Medical University. The inclusion criteria were as follows: [1] patients who underwent laparoscopic-assisted or traditional open complete mesocolic excision (CME); [2] surgeries conducted by experienced senior physicians skilled in performing CME autonomously; and [3] those with a postoperative pathological diagnosis confirming colon cancer. Exclusion criteria included: (1) patients with other types of malignant tumors; (2) those with confirmed distant metastasis of colon cancer by pathological examination or imaging; (3) patients diagnosed with severe cardiovascular or respiratory disorders; (4) individuals with major organ diseases, such as liver or kidney issues; and (5) cases with incomplete data or patients who were lost to follow-up. Every participant was observed for a minimum of three years post-surgery. This study adhered to the Declaration of Helsinki guidelines and received approval from the Wuxi People’s Hospital Ethics Committee.
2.2 Variables for predicting postoperative gastroparesis
The dataset encompassed 34 variables collected before, during, and after surgery. Preoperative variables, gathered within 24 h prior to surgery, included demographic details (such as gender, age, smoking and alcohol habits, and body mass index), fundamental clinical traits (including the American Society of Anesthesiologists score, nutrition risk screening 2002 score, surgical history, and history of adjuvant therapies like chemotherapy and radiotherapy), basic medical history (anemia, diabetes, hypothyroidism, hypertension, chronic obstructive pulmonary disease, hyperlipidemia, and coronary artery disease), laboratory results (albumin, carcinoembryonic antigen, carbohydrate antigen 19–9), and tumor specifics (such as T-stage, N-stage, nerve invasion, tumor size, and count). Intraoperative variables comprised the surgical type and approach, surgery duration, blood loss, need for transfusion, and arterial oxygen saturation during the operation. Postoperative variables identified laboratory metrics (procalcitonin, C-reactive protein, and serum amyloid A). The primary outcome measured was postoperative gastroparesis.
2.3 Diagnostic criteria for postoperative gastroparesis
The criteria for diagnosing postoperative gastroparesis involve several key points: (1) the manifestation of gastrointestinal symptoms, such as nausea, vomiting, early satiety, bloating, or epigastric pain in the patient; (2) the exclusion of other diseases that may cause the patient's gastrointestinal symptoms, such as mechanical obstruction, drug-related side effects, or metabolic disorders; (3) the exclusion of factors such as the utilization of drugs that affect smooth muscle contraction; and (4) the implementation of transgastric scintigraphy or magnetic resonance imaging to identify delayed gastric emptying [16, 17].
2.4 Development and validation of clinical prediction models
This research employed SPSS and R for statistical analysis to build and assess clinical prediction models. Here are the steps followed: (1) Colon cancer patients from Wuxi People's Hospital from January 2010 to January 2020 were randomly divided into a training set (70%) and a validation set (30%). (2) Data from the training set underwent univariate and multivariate regression analysis. The chi-square test was used for categorical variables, and a t-test for continuous data that followed a normal distribution. For non-normally distributed data, the rank sum test was utilized. A significance level of p < 0.05 was adopted. Logistic regression analysis assessed significant variables from univariate analysis to determine their independent impact on postoperative gastroparesis. The importance of each factor was ranked using models: extreme gradient boosting (XGBoost), random forest (RF), support vector machine (SVM), and k-nearest neighbor algorithm (KNN). Variables consistently ranked in the top ten across all models and significant in the analyses were selected. (3) Prediction models were constructed using these refined variables as inputs for the SVM, RF, XGBoost, and KNN algorithms. Models were appraised based on discrimination, calibration, and clinical utility to find the optimal one. The ROC curve calculated the area under the curve (AUC) to measure predictive capability, and a calibration curve evaluated alignment between predicted and actual data. Decision curve analysis (DCA) gauged patient benefit from relevant treatments. Internal validation utilized k-fold cross-validation. Performance metrics for each algorithm included the AUC, accuracy, sensitivity, specificity, and F1 Score, all with respective 95% confidence intervals. (4) The best model was tested with an external validation set to verify generalizability and predictive efficiency, using ROC curves. (5) Model interpretation was realized through SHAP (Shapley additive explanations), visualizing each feature's impact on predictions with SHAP summary and force plots.
3 Results
3.1 Basic clinical information of the patient
The study included a total of 1146 colon cancer patients, among which 95 patients (8.29%) were diagnosed with gastroparesis (Fig. 1, Table 1). The internal validation set comprised 822 colon cancer patients, of whom 66 patients (8.03%) had gastroparesis. The external validation set consisted of 324 colon cancer patients, with 29 patients (8.95%) having gastroparesis. The original data presented in the study are included in the Table S1.
3.2 Screening for risk factors for postoperative gastroparesis
The results of univariate and multifactorial analyses showed that age, albumin (ALB), history of anemia, history of diabetes mellitus, history of hypothyroidism, history of adjuvant radiotherapy, type of surgery, duration of surgery, intraoperative bleeding, tumor size, and number of tumors were independent influencing factors for gastroparesis (P < 0.05) (Table 2). XGBoost, RF, SVM, and KNN models screened risk factors for postoperative gastroparesis, including advanced age, hypoproteinemia, history of anemia, history of diabetes mellitus, history of hypothyroidism, open surgery, long operative time, and high intraoperative bleeding (Fig. 2A-D). Based on a comprehensive analysis, the variables included in the prediction model were age ≥ 65, hypoproteinemia, history of anemia, history of diabetes mellitus, history of hypothyroidism, open surgery, operative time ≥ 270 min, and intraoperative bleeding ≥ 100 ml.
3.3 Model building and evaluation
The results of the ROC curve analysis revealed that the XGBoost model had a high AUC value of 0.976 in the training set, while the AUC value in the validation set was 0.906, which demonstrated the best performance compared to the other three models (Table 3). Furthermore, the calibration curves of all four models resembled the ideal curves, indicating that there was high agreement between the predicted and actual results. Additionally, the decision curve analysis (DCA) curves showed that all four models had a net clinical benefit when compared to both the full treatment and no treatment plan (Fig. 3 A-D). The study evaluated the generalization ability of the four models using k-fold cross-validation. A sample of 247 cases (30.05%) from the internal validation set was taken as the validation set, and the remaining samples were used as the training set and subjected to tenfold cross-validation. The XGBoost algorithm had an AUC value of 0.8948 ± 0.0551 in the validation set and an AUC value of 0.8340 in the test set, with an accuracy of 0.8745 (Fig. 4 A-C). The RF algorithm showed an AUC value of 0.8931 ± 0.0665 in the validation set and an AUC value of 0.8126 in the test set, with an accuracy of 0.8138. The SVM algorithm exhibited an AUC value of 0.8364 ± 0.1014 in the validation set and an AUC value of 0.7916 in the test set, with an accuracy of 0.8704. Finally, the KNN algorithm had an AUC value of 0.7912 ± 0.0918 in the validation set and an AUC value of 0.6945 in the test set, with an accuracy of 0.9069. After comprehensive comparison, the XGBoost algorithm was selected to construct the model in this study.
Evaluation of the four models for predicting gastroparesis. A ROC curves for the training set of the four models. B ROC curves for the validation set of the four models. C Calibration plots of the four models. The 45° dotted line on each graph represents the perfect match between the observed (y-axis) and predicted (x-axis) complication probabilities. A closer distance between two curves indicates greater accuracy. D DCA curves of the four models. The intersection of the red curve and the All curve is the starting point, and the intersection of the red curve and the None curve is the node within which the corresponding patients can benefit
3.4 Model external validation
The disease prediction model achieved a high degree of accuracy, as evidenced by the external validation set's AUC value of 0.77 (Fig. 4 D).
3.5 Model explanation
The SHAP summary plot demonstrated that various risk factors contribute to gastroparesis after CME, with age greater than or equal to 65, a history of anemia, diabetes mellitus, intraoperative bleeding of 100 ml or more, operative time of 270 min or more, hypoproteinemia, a history of hypothyroidism, and open surgery ranking as the most significant factors (Fig. 5). The SHAP power plot illustrates the predictive analysis of the research model for two patients with colon cancer who had gastroparesis. The model predicted a probability of 0.443 for gastroparesis in the first patient, with the contributing factors being intraoperative bleeding of 100 ml or more, an operative time of 270 min or more, an age of 65 years or older, and a history of diabetes mellitus. Similarly, the model predicted a probability of 0.400 for gastroparesis in the second patient, with the contributing factors being a history of anemia, intraoperative bleeding of 100 ml or more, a history of hypothyroidism, and an age of 65 years or older (Fig. 6 A-B).
SHAP force plot. The contributing variables are arranged in the horizontal line, sorted by the absolute value of their impact. Blue represents features that have a negative effect on disease prediction, with a decrease in SHAP values; red represents features that have a positive effect on disease prediction, with an increase in SHAP values. A Predictive Analysis of Patient I. B Predictive Analysis of Patient II
4 Discussion
The present study aimed to evaluate the performance of four machine learning algorithms in constructing risk prediction models. The XGBoost algorithm was found to exhibit extremely high accuracy and is known for its efficiency, flexibility, and adaptability [18]. In contrast to the RF algorithm, the XGBoost algorithm utilizes the gradient boosting integration strategy, which prioritizes hard-to-classify samples, resulting in better generalization performance and stability of the model [19]. The SVM and KNN algorithms were also observed to have higher accuracy and effectively avoid overfitting issues. However, in addressing the problem of multiple features and large datasets, the XGBoost algorithm utilizes L1 and L2 regularization methods, which can effectively overcome the overfitting problem. Additionally, the XGBoost algorithm can automatically handle missing values and output the contribution of each feature to the prediction result, thereby providing good interpretability. This characteristic makes it more advantageous for multidimensional studies. Therefore, after a comprehensive comparison of the four machine learning algorithms, the XGBoost algorithm was selected to construct a model for predicting the occurrence of gastroparesis after CME.
At the outset of the study, we also considered using other machine learning algorithms for feature selection, such as neural networks, logistic regression, or Bayesian classifiers. However, for the following reasons, we ultimately chose the current combination of algorithms: Firstly, the selected algorithms excel in handling high-dimensional data and complex feature interactions, and their application in medical prediction models has been extensively validated. Neural networks, particularly deep neural networks (DNNs), typically require substantial computational resources. Training can be very time-consuming and demands high-performance hardware, which somewhat limits their clinical applicability. Logistic regression assumes a linear relationship between features and outcomes, which may perform poorly in handling nonlinear relationships and might require feature transformation or interaction terms. Bayesian classifiers assume that all features are conditionally independent given the class, which is rarely true in practice. If there are correlations between features, the performance of Bayesian classifiers may significantly decline. Therefore, considering model interpretability and clinical applicability, we ultimately selected XGBoost, Random Forest (RF), Support Vector Machine (SVM), and k-Nearest Neighbors (KNN) for this study. Of course, this study also addressed the issue of model overfitting. Overfitting is prone to occur when the training data is limited or the model complexity is high. In this study, we used a large amount of data and more training samples, which effectively mitigates overfitting, as the model can learn more generalized features from a larger dataset. Additionally, we employed k-fold cross-validation, which better assesses the model’s generalization ability and aids in selecting more suitable models and parameters during training. Moreover, by validating the model with external data collected from different hospitals and regions, we ensured the model's applicability to a broader patient population, reduced bias in specific datasets, and partially alleviated the effects of overfitting.
Studies [20,21,22] have demonstrated the effectiveness of machine learning algorithms in clinical diagnosis and prognosis, as they can predict adverse outcomes in disease progression more accurately than traditional diagnostic methods. In this study, machine learning algorithms were employed to construct a predictive model that identified treatment measures that did more harm than good for colon cancer patients, prompting clinicians to adjust the treatment plan accordingly. The model also reduced the financial burden on patients' families, avoided nonessential tests, and minimized the side effects of diagnostic instruments on patients. Most importantly, the model accurately identified high-risk patients for timely interventional treatment, improving patient prognosis. SHAP analysis was utilized to visualize and interpret the model, revealing that advanced age, longer operative time, higher intraoperative bleeding, surgical approach, hypoproteinemia, anemia, history of diabetes mellitus, and history of hypothyroidism were risk factors for postoperative gastroparesis in CME [23, 24].
As patients grow older, various changes occur in the digestive system, such as decreased secretion of digestive enzymes and reduced peristaltic capacity of the smooth muscles of the gastrointestinal tract. These changes increase the risk of postoperative gastroparesis in elderly patients to some extent. Additionally, the tissues of the gastrointestinal tract are more brittle and have a higher likelihood of peripheral atherosclerosis in elderly patients, making them more susceptible to bleeding during surgery [25]. Furthermore, elderly patients often have chronic cardiovascular and respiratory diseases that can affect the nerves and muscles controlling the digestive system, leading to delayed gastric emptying. Due to decreased compensatory function, elderly patients have a reduced capacity to respond to the stress of surgery, which further exacerbates the disturbance of gastrointestinal motility. More importantly, elderly patients require a longer time to resume normal activities after surgery, which may slow down the movement of food in the stomach and intestines. In conclusion, postoperative gastroparesis in elderly patients is the result of a combination of factors. The study by Meng et al., which included 563 oncology patients, demonstrated a strong association between advanced age and postoperative gastroparesis after abdominal surgery, further supporting the findings of this study [26].
The present study aligns with prior research, identifying surgery as a significant determinant of postoperative complications [27, 28]. The surgical procedure itself can cause damage to the stomach's nerves and muscles, leading to impaired contraction and emptying function. Traditional open radical colon cancer surgery, requiring an incision in the patient’s lower left abdomen, results in prolonged operation time, extensive resection, substantial postoperative pain, and slow patient recovery. In contrast, laparoscopic surgery offers greater delicacy and convenience, allowing the operator to intuitively identify the lesion size and surrounding tissues and perform precise operations such as tissue separation and intestinal wall anastomosis, thereby avoiding damage to normal gastrointestinal tract tissues. Furthermore, laparoscopic surgery offers a natural advantage of minimal invasiveness. By using this technique to clamp the intestinal wall, the patient's mesentery and lymph nodes sustain less harm, and the gastrointestinal tract's blood supply is barely affected, thus reducing the chance of ischemia‒reperfusion injury. This benefit also hastens the recovery of postoperative gastrointestinal motility. Several studies have demonstrated that laparoscopic surgery patients exhibit faster gastrointestinal recovery and fewer postoperative complications, including those with gastric and intestinal cancer [29, 30]. Consequently, we contend that surgical type is a crucial factor in the development of postoperative gastroparesis. In this study, prolonged operative time and increased intraoperative bleeding were found to be two other important factors contributing to postoperative gastroparesis. These factors are often associated with the complexity of the surgical procedure and the risk of inadvertent injury to gastric omental vessels and lymph nodes, resulting in an exacerbation of the inflammatory response and disruption of normal gastrointestinal tract movements [31]. Furthermore, increased intraoperative bleeding can lead to impaired hemodynamics in the gastric mucosa and other intestinal segments, which delays the recovery of gastrointestinal tissues. Longer surgical times also require increased use of anesthetic drugs that inhibit sympathetic constrictor nerve fibers, causing vascular smooth muscle diastole and a decrease in blood pressure. This decrease can result in serious complications such as gastroparesis. Anesthetic drugs may also affect the function of the vagus nerve, resulting in persistent spasm of the pyloric sphincter and impacting gastric emptying efficacy [32]. Therefore, it is crucial for the surgical team to adequately plan the surgical procedure preoperatively and work closely together intraoperatively to improve surgical efficiency, shorten the operative time, and minimize the occurrence of postoperative gastroparesis.
Furthermore, this study aimed to explicate the rationale behind the postoperative gastroparesis prediction model using two samples. In the disease prediction analysis of the second sample, the patient's nutritional status was considered one of the crucial predictors. Plasma albumin, despite its small molecular weight, plays a pivotal role in maintaining plasma and tissue fluid osmolality. Hypoalbuminemia leads to decreased plasma colloid osmotic pressure, which increases the risk of intestinal wall edema and consequently adversely affects gastrointestinal motility. Additionally, patients with hypoalbuminemia exhibit lower numbers and lower activity of antibody synthase, which makes them more susceptible to complications such as postoperative infection and anastomotic leakage, thereby hampering normal gastrointestinal recovery. These findings indicate that clinicians must adequately evaluate the preoperative albumin level and manage postoperative symptoms in a timely manner in patients, as well as review all nutritional indicators of patients. Parenteral nutrition should be enhanced in patients with hypoproteinemia, while appropriate supplementation with a high-protein diet, when possible, can help reduce the risk of hypoalbuminemia and the development of postoperative gastroparesis [33]. Similarly, patients with anemia are more susceptible to unfavorable outcomes such as postoperative gastroparesis. Patients who have undergone right hemicolectomy often experience tumor infiltration that destroys the mucosa and submucosal vessels in the lining of the colon, resulting in malnutrition-related diseases such as iron deficiency anemia. Hemoglobin, which is responsible for carrying oxygen in the blood, is crucial for the adequate oxygen supply to body tissues. Patients with anemia experience tissue hypoxia due to the lack of hemoglobin, which severely impacts the normal movement of the gastrointestinal smooth muscles. Additionally, the small perigastric vessels play a significant nutritional role in the perigastric vagus nerve. Ahmad et al. demonstrated that preoperative hemoglobin levels were an independent risk factor for the development of delayed gastric emptying [34]. The incidence of gastroparesis is substantially higher in anemic patients than in nonanemic patients, highlighting the need for careful attention to gastrointestinal dysfunction in anemic patients and the timely implementation of preventive measures against gastrointestinal complications.
The present investigation has additionally uncovered that the underlying ailment exerts a more pronounced impact on the development of postoperative gastroparesis. Diabetic patients frequently exhibit neuropathic changes that affect both their autonomic and visceral nerves, thereby constraining the movement of the smooth muscles of the gastrointestinal tract [35]. Farrugia et al. demonstrated that a reduction in Cajal mesenchymal cells, an essential cell type responsible for controlling GI motility, is a crucial mechanism for delayed gastric emptying in an animal model, thus further substantiating the current study's findings [36]. Moreover, it is our belief that fluctuations in blood glucose due to perioperative fasting serve as an important trigger for the development of gastroparesis in patients. Such patients frequently develop insulin resistance after surgery, which impedes the secretion and release of gastrin and impacts the function of the autonomic nerves that oversee gastric emptying [37]. The rate of gastric emptying was found to be strongly associated with diabetes in a multicenter study spanning 30 years, which was carried out by clinical researchers globally [37, 38]. Similarly, individuals with hypothyroidism experience similar effects. Thyroxine plays a vital role in maintaining the body's physiological functions, particularly in motor function. However, clinicians tend to concentrate on limb movements in hypothyroid patients and tend to ignore glandular organ activity. Pathologists who performed pathological biopsies of gastric tissues from hypothyroid patients found edema and thickening of gastric mucosal cells in hypothyroid patients with surrounding inflammatory cell infiltration [39]. Based on this, Ghoshal conducted a clinical study involving 60 hypothyroid patients and discovered that decreased thyroxine was closely associated with decreased gastrointestinal motility [40]. It is believed that increased vagal function in hypothyroid patients leads to hyperexcitability and the loss of normal coordination and regularity of the smooth muscles of the gastrointestinal tract. The experimental findings of Khraisha in patients with chronic atrophic gastritis and gastroparesis also support the therapeutic effects of thyroxine on gastric motility disorders [41].
This study used multiple machine learning algorithms to construct a model for predicting postoperative gastroparesis and identified relevant high-risk factors. These findings can serve as a standard to assist in preoperative patient assessment. If the assessment indicates that a patient is at high risk, corrective actions can be taken for the identified risk factors and special attention can be given during postoperative care. Specific measures include timely imaging examinations and implementing appropriate interventions to prevent the occurrence of postoperative gastroparesis.
The present investigation conducted a comprehensive evaluation of the model in terms of discrimination, calibration, and clinical utility. Nonetheless, it is imperative to acknowledge certain limitations within this study. While multiple facets of risk factors were taken into account, the inclusion of imaging as one of these factors was regrettably omitted. It is our firm belief that future research endeavors on predictive models should place emphasis on amalgamating imaging data with patient clinical indicators to achieve enhanced disease prediction capabilities. Moreover, although the machine learning algorithms were more accurate, their models were more intricate and less transparent. The entire computational and decision-making process of the model was executed in a black box, which is less intuitive and less apparent than the logistic regression model [42]. On the other hand, the present study is a retrospective study with certain drawbacks, such as selection bias, distribution bias, and retrospective bias. Therefore, there is a need to incorporate multicenter prospective studies in future research to enhance the reliability of the outcomes.
5 Conclusion
This study developed a model using the XGBoost machine learning algorithm to predict the risk of postoperative gastroparesis after CME. The model demonstrated good predictive accuracy and clinical utility, enabling surgeons to diagnose patients promptly. The model highlighted that gastroparesis remains a significant challenge for patients with colon cancer after surgery and is strongly linked to advanced age, longer operation time, higher intraoperative bleeding, surgical approach, hypoproteinemia, anemia, history of diabetes mellitus, and history of hypothyroidism.
Data availability
The datasets used in this study are available from the corresponding author on reasonable request.
References
Alexander DD, Weed DL, Miller PE, Mohamed MA. Red meat and colorectal cancer: a quantitative update on the state of the epidemiologic science. J Am Coll Nutr. 2015;34(6):521–43. https://doi.org/10.1080/07315724.2014.992553.
Feng B, Sun J, Ling TL, Lu AG, Wang ML, Chen XY, et al. Laparoscopic complete mesocolic excision (Cme) with medial access for right-hemi colon cancer: feasibility and technical strategies. Surg Endosc. 2012;26(12):3669–75. https://doi.org/10.1007/s00464-012-2435-9.
Pedrazzani C, Lauka L, Sforza S, Ruzzenente A, Nifosì F, Delaini G, et al. Management of nodal disease from colon cancer in the laparoscopic era. Int J Colorectal Dis. 2015;30(3):303–14. https://doi.org/10.1007/s00384-014-2075-8.
Egboh SC, Abere S. Gastroparesis: a multidisciplinary approach to management. Cureus. 2022;14(1): e21295. https://doi.org/10.7759/cureus.21295.
Baia M, Conti L, Pasquali S, Sarre-Lazcano C, Abatini C, Cioffi SPB, et al. Delayed gastric emptying after multivisceral resection for retroperitoneal sarcoma. Ann Surg Oncol. 2022;29(5):3264–70. https://doi.org/10.1245/s10434-021-11154-z.
Maehata T, Sato Y, Nakamoto Y, Kato M, Kawashima A, Kiyokawa H, et al. Updates in the field of submucosal endoscopy. Life (Basel). 2022. https://doi.org/10.3390/life13010104.
Tack J, Janssen P. Gastroduodenal motility. Curr Opin Gastroenterol. 2010;26(6):647–55. https://doi.org/10.1097/MOG.0b013e32833ece1e.
Lacy BE, Crowell MD, Mathis C, Bauer D, Heinberg LJ. Gastroparesis: quality of life and health care utilization. J Clin Gastroenterol. 2018;52(1):20–4. https://doi.org/10.1097/mcg.0000000000000728.
Blackett JW, Benvenuto L, Leiva-Juarez MM, D’Ovidio F, Arcasoy S, Jodorkovsky D. Risk factors and outcomes for gastroparesis after lung transplantation. Dig Dis Sci. 2022;67(6):2385–94. https://doi.org/10.1007/s10620-021-07249-y.
Jiang T, Gradus JL, Rosellini AJ. Supervised machine learning: a brief primer. Behav Ther. 2020;51(5):675–87. https://doi.org/10.1016/j.beth.2020.05.002.
Zhang L, Cui Y, Mei J, Zhang Z, Zhang P. Exploring cellular diversity in lung adenocarcinoma epithelium: advancing prognostic methods and immunotherapeutic strategies. Cell Prolif. 2024. https://doi.org/10.1111/cpr.13703.
Zhang P, Pei S, Zhou G, Zhang M, Zhang L, Zhang Z. Purine metabolism in lung adenocarcinoma: a single-cell analysis revealing prognostic and immunotherapeutic insights. J Cell Mol Med. 2024;28(8): e18284. https://doi.org/10.1111/jcmm.18284.
Yue S, Li S, Huang X, Liu J, Hou X, Zhao Y, et al. Machine learning for the prediction of acute kidney injury in patients with sepsis. J Transl Med. 2022;20(1):215. https://doi.org/10.1186/s12967-022-03364-0.
Ye B, Ji H, Zhu M, Wang A, Tang J, Liang Y, et al. Single-cell sequencing reveals novel proliferative cell type: a key player in renal cell carcinoma prognosis and therapeutic response. Clin Exp Med. 2024;24(1):167. https://doi.org/10.1007/s10238-024-01424-x.
Ye B, Hongting G, Zhuang W, Chen C, Yi S, Tang X, et al. Deciphering lung adenocarcinoma prognosis and immunotherapy response through an Ai-driven stemness-related gene signature. J Cell Mol Med. 2024;28(14): e18564. https://doi.org/10.1111/jcmm.18564.
Sullivan A, Temperley L, Ruban A. Pathophysiology, aetiology and treatment of gastroparesis. Dig Dis Sci. 2020;65(6):1615–31. https://doi.org/10.1007/s10620-020-06287-2.
Zheng T, Camilleri M. Management of gastroparesis. Gastroenterol Hepatol (N Y). 2021;17(11):515–25.
Arezzo F, Cormio G, Mongelli M, Cazzato G, Silvestris E, Kardhashi A, et al. Machine learning applied to MRI evaluation for the detection of lymph node metastasis in patients with locally advanced cervical cancer treated with neoadjuvant chemotherapy. Arch Gynecol Obstet. 2022. https://doi.org/10.1007/s00404-022-06824-6.
Melinte-Popescu AS, Vasilache IA, Socolov D, Melinte-Popescu M. Predictive performance of machine learning-based methods for the prediction of preeclampsia—a prospective study. J Clin Med. 2023. https://doi.org/10.3390/jcm12020418.
Ebrahimi M, Aghagolzadeh P, Shamabadi N, Tahmasebi A, Alsharifi M, Adelson DL, et al. Understanding the undelaying mechanism of Ha-subtyping in the level of physic-chemical characteristics of protein. PLoS One. 2014;9(5): e96984. https://doi.org/10.1371/journal.pone.0096984.
Lin Z, Chou WC. Machine learning and artificial intelligence in toxicological sciences. Toxicol Sci. 2022;189(1):7–19. https://doi.org/10.1093/toxsci/kfac075.
Zhang P, Wu X, Wang D, Zhang M, Zhang B, Zhang Z. Unraveling the role of low-density lipoprotein-related genes in lung adenocarcinoma: insights into tumor microenvironment and clinical prognosis. Environ Toxicol. 2024. https://doi.org/10.1002/tox.24230.
Chi H, Huang J, Yan Y, Jiang C, Zhang S, Chen H, et al. Unraveling the role of disulfidptosis-related LncRNAs in colon cancer: a prognostic indicator for immunotherapy response, chemotherapy sensitivity, and insights into cell death mechanisms. Front Mol Biosci. 2023;10:1254232. https://doi.org/10.3389/fmolb.2023.1254232.
He R, Huang S, Lu J, Su L, Gao X, Chi H. Unveiling the immune symphony: decoding colorectal cancer metastasis through immune interactions. Front Immunol. 2024;15:1362709. https://doi.org/10.3389/fimmu.2024.1362709.
Lermite E, Pessaux P, Brehant O, Teyssedou C, Pelletier I, Etienne S, et al. Risk factors of pancreatic fistula and delayed gastric emptying after pancreaticoduodenectomy with pancreaticogastrostomy. J Am Coll Surg. 2007;204(4):588–96. https://doi.org/10.1016/j.jamcollsurg.2007.01.018.
Meng H, Zhou D, Jiang X, Ding W, Lu L. Incidence and risk factors for postsurgical gastroparesis syndrome after laparoscopic and open radical gastrectomy. World J Surg Oncol. 2013;11:144. https://doi.org/10.1186/1477-7819-11-144.
Al-Ani HH, Sims JL, Niederer RL. Cataract surgery in uveitis: risk factors, outcomes, and complications. Am J Ophthalmol. 2022;244:117–24. https://doi.org/10.1016/j.ajo.2022.08.014.
Crane J, Hamed M, Borucki JP, El-Hadi A, Shaikh I, Stearns AT. Complete mesocolic excision versus conventional surgery for colon cancer: a systematic review and meta-analysis. Colorectal Dis. 2021;23(7):1670–86. https://doi.org/10.1111/codi.15644.
Magistro C, Bertoglio CL, Giani A, Mazzola M, Rubicondo C, Maspero M, et al. Laparoscopic complete mesocolic excision versus conventional resection for right-sided colon cancer: a propensity score matching analysis of short-term outcomes. Surg Endosc. 2022;36(5):3049–58. https://doi.org/10.1007/s00464-021-08601-z.
Fukagawa T. Role of staging laparoscopy for gastric cancer patients. Ann Gastroenterol Surg. 2019;3(5):496–505. https://doi.org/10.1002/ags3.12283.
Yang DD, He K, Wu XL, Yang LK, Nie SF. Risk factors of gastroparesis syndrome after abdominal non-gastroduodenal operation and its prevention. Asian Pac J Trop Med. 2013;6(6):497–9. https://doi.org/10.1016/s1995-7645(13)60082-6.
van Berge Henegouwen MI, van Gulik TM, DeWit LT, Allema JH, Rauws EA, Obertop H, et al. Delayed gastric emptying after standard pancreaticoduodenectomy versus pylorus-preserving pancreaticoduodenectomy: an analysis of 200 consecutive patients. J Am Coll Surg. 1997;185(4):373–9. https://doi.org/10.1016/s1072-7515(97)00078-1.
Hayakawa T, Kaneko H, Konagaya T, Shinozaki K, Kasahara A, Funaki Y, et al. Enhanced somatostatin secretion into the gastric juice with recovery of basal acid output after Helicobacter pylori eradication in gastric ulcers. J Gastroenterol Hepatol. 2003;18(5):505–11. https://doi.org/10.1046/j.1440-1746.2003.03008.x.
Al-Saffar A, Lennernäs H, Hellström PM. Gastroparesis, metoclopramide, and tardive dyskinesia: risk revisited. Neurogastroenterol Motil. 2019;31(11): e13617. https://doi.org/10.1111/nmo.13617.
Petri M, Singh I, Baker C, Underkofler C, Rasouli N. Diabetic gastroparesis: an overview of pathogenesis, clinical presentation and novel therapies, with a focus on ghrelin receptor agonists. J Diabetes Complicat. 2021;35(2):107733. https://doi.org/10.1016/j.jdiacomp.2020.107733.
Farrugia G. Interstitial cells of cajal in health and disease. Neurogastroenterol Motil. 2008;20(Suppl 1):54–63. https://doi.org/10.1111/j.1365-2982.2008.01109.x.
Hasler WL. Gastroparesis: pathogenesis, diagnosis and management. Nat Rev Gastroenterol Hepatol. 2011;8(8):438–53. https://doi.org/10.1038/nrgastro.2011.116.
Bharucha AE, Batey-Schaefer B, Cleary PA, Murray JA, Cowie C, Lorenzi G, et al. Delayed gastric emptying is associated with early and long-term hyperglycemia in type 1 diabetes mellitus. Gastroenterology. 2015;149(2):330–9. https://doi.org/10.1053/j.gastro.2015.05.007.
Nassar Y, Richter S. Gastroparesis in non-diabetics: associated conditions and possible risk factors. Gastroenterol Res. 2018;11(5):340–5. https://doi.org/10.14740/gr1060w.
Ghoshal UC, Bhut B, Misra A. Patients with specific gastrointestinal motility disorders are commonly diagnosed as functional Gi disorders in the early stage by community physicians due to lack of awareness. Turk J Gastroenterol. 2021;32(4):336–48. https://doi.org/10.5152/tjg.2021.20514.
Sk O, Ma M, Np A, Kp T. Gastroparesis—a novel cause of persistent thyroid stimulating hormone elevation in hypothyroidism. J La State Med Soc. 2015;167(2):47–9.
Aromolaran O, Aromolaran D, Isewon I, Oyelade J. Machine learning approach to gene essentiality prediction: a review. Brief Bioinform. 2021. https://doi.org/10.1093/bib/bbab128.
Funding
No funding.
Author information
Authors and Affiliations
Contributions
The study was conceived and designed by WW. Data collection was conducted by ZY. QZ performed the statistical analysis. The first draft of the manuscript was written by ZZ. The final approval of the submitted version was given by ZY and YJ. All authors contributed to the manuscript and approved the submitted version.
Corresponding authors
Ethics declarations
Ethics approval and consent to participate
This study is a retrospective analysis, and all data were obtained from patient records at The Affiliated Wuxi People's Hospital of Nanjing Medical University. The research protocol was reviewed and approved by the Ethics Committee of The Affiliated Wuxi People's Hospital of Nanjing Medical University. As this is a retrospective study and all patient identities have been anonymized, the Ethics Committee granted a waiver for individual informed consent. This study adheres to the ethical principles outlined in the Declaration of Helsinki and its subsequent amendments, ensuring the protection of patient privacy and data confidentiality.
Competing interests
The authors declare no competing interests.
Additional information
Publisher's Note
Springer Nature remains neutral with regard to jurisdictional claims in published maps and institutional affiliations.
Supplementary Information
Rights and permissions
Open Access This article is licensed under a Creative Commons Attribution-NonCommercial-NoDerivatives 4.0 International License, which permits any non-commercial use, sharing, distribution and reproduction in any medium or format, as long as you give appropriate credit to the original author(s) and the source, provide a link to the Creative Commons licence, and indicate if you modified the licensed material. You do not have permission under this licence to share adapted material derived from this article or parts of it. The images or other third party material in this article are included in the article’s Creative Commons licence, unless indicated otherwise in a credit line to the material. If material is not included in the article’s Creative Commons licence and your intended use is not permitted by statutory regulation or exceeds the permitted use, you will need to obtain permission directly from the copyright holder. To view a copy of this licence, visit http://creativecommons.org/licenses/by-nc-nd/4.0/.
About this article
Cite this article
Wang, W., Yan, Z., Zhang, Z. et al. Machine learning-based prediction of gastroparesis risk following complete mesocolic excision. Discov Onc 15, 483 (2024). https://doi.org/10.1007/s12672-024-01355-9
Received:
Accepted:
Published:
DOI: https://doi.org/10.1007/s12672-024-01355-9