Abstract
This paper presents a method to predict short-term trends in financial time series data found in the foreign exchange market. Trends in the Forex market appear with similar chart patterns. We approach the chart patterns in the financial markets from a discovery of motifs in a time series perspective. Our method uses a modified Zigzag technical indicator to segment the data and discover motifs, expectation maximization to cluster the motifs and support vector machines to classify the motifs and predict accurate trading parameters for the identified motifs. The available input data are adapted to each trading time frame with a sliding window. The accuracy of the prediction models is tested across several different currency pairs, spanning 5 years of historical data from 2010 to 2015. The experimental results suggest that using the Zigzag technical indicator to discover motifs that identify short-term trends in financial data results in a high prediction accuracy and trade profits.













Similar content being viewed by others
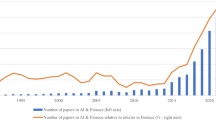
Notes
Please refer to the “Appendix A.1” for the definitions of Forex terms take profit and stop loss.
References
Ahmed NK, Atiya AF, Gayar NE, El-Shishiny H (2010) An empirical comparison of machine learning models for time series forecasting. Econom Rev 29(5–6):594–621
Aminikhanghahi S, Cook DJ (2017) A survey of methods for time series change point detection. Knowl Inf Syst 51(2):339–367
Bolton AH (1976) The Elliott wave principle: a critical appraisal. Monetary Research Limited, Hamilton
Brown DP, Jennings RH (1989) On technical analysis. Rev Financ Stud 2(4):527–551
Chakraborti A, Toke IM, Patriarca M, Abergel F (2011) Econophysics review: I. Empirical facts. Quant Finance 11(7):991–1012
Cont R (2005) Long range dependence in financial markets. In: Lutton E, Levy Vehel J (eds) Fractals in engineering. Springer, Berlin, pp 159–179
Cortes C, Vapnik V (1995) Support-vector networks. Mach Learn 20(3):273–297
Danıelsson J, Payne R (2002) Real trading patterns and prices in spot foreign exchange markets. J Int Money Finance 21(2):203–222
Dempster AP, Laird NM, Rubin DB (1977) Maximum likelihood from incomplete data via the EM algorithm. J R Stat Soc Series B (Methodol) 39(1):1–38
Denton AM, Besemann CA, Dorr DH (2009) Pattern-based time-series subsequence clustering using radial distribution functions. Knowl Inf Syst 18(1):1–27
Esling P, Agon C (2012) Time-series data mining. ACM Comput Surv (CSUR) 45(1):12
Faloutsos C, Ranganathan M, Manolopoulos Y (1994) Fast subsequence matching in time-series databases, vol 23. ACM, New York
Gaucan V et al (2011) How to use Fibonacci retracement to predict forex market. J Knowl Manag Econ Inf Technol Econ 2(2):1
Gopikrishnan P, Plerou V, Amaral LAN, Meyer M, Stanley HE (1999) Scaling of the distribution of fluctuations of financial market indices. Phys Rev E 60(5):5305
Hochreiter S, Schmidhuber J (1997) Long short-term memory. Neural Comput 9(8):1735–1780
Huang C-J, Yang D-X, Chuang Y-T (2008) Application of wrapper approach and composite classifier to the stock trend prediction. Expert Syst Appl 34(4):2870–2878
Kampouridis M, Otero FE (2017) Heuristic procedures for improving the predictability of a genetic programming financial forecasting algorithm. Soft Comput 21(2):295–310
Kamruzzaman J, Sarker RA (2003) Forecasting of currency exchange rates using ANN: a case study. In: Proceedings of the 2003 international conference on neural networks and signal processing, 2003, vol 1. IEEE, pp 793–797
Keogh E, Chu S, Hart D, Pazzani M (2004) Segmenting time series: a survey and novel approach. In: Data mining in time series databases, World Scientific, pp 1–21
Khemchandani R, Chandra S et al (2009) Regularized least squares fuzzy support vector regression for financial time series forecasting. Expert Syst Appl 36(1):132–138
Kirkpatrick CD II, Dahlquist JA (2010) Technical analysis: the complete resource for financial market technicians. FT Press, Upper Saddle River
Korol T (2014) A fuzzy logic model for forecasting exchange rates. Knowl Based Syst 67:49–60
Lee M-C (2009) Using support vector machine with a hybrid feature selection method to the stock trend prediction. Expert Syst Appl 36(8):10896–10904
Leitch G, Tanner JE (1991) Economic forecast evaluation: profits versus the conventional error measures. Am Econ Rev 81:580–590
Lo AW (1989) Long-term memory in stock market prices, Technical report, National Bureau of Economic Research
Loglisci C, Malerba D (2017) Leveraging temporal autocorrelation of historical data for improving accuracy in network regression. Stat Anal Data Min ASA Data Sci J 10(1):40–53
Lu C-J, Lee T-S, Chiu C-C (2009) Financial time series forecasting using independent component analysis and support vector regression. Decis Supp Syst 47(2):115–125
Major Forex Currencies (n.d.). http://www.investopedia.com/university/forex-currencies/. Accessed 17 Aug 2015
Moosa I, Burns K (2014) The unbeatable random walk in exchange rate forecasting: Reality or myth? J Macroecon 40:69–81
Mueen A (2013) Enumeration of time series motifs of all lengths. In: 2013 IEEE 13th international conference on data mining (ICDM), IEEE, pp 547–556
Mueen A, Chavoshi N, Abu-El-Rub N, Hamooni H, Minnich A (2016) Awarp: fast warping distance for sparse time series. In: 2016 IEEE 16th international conference on data mining (ICDM), IEEE, pp 350–359
JV Neumann, Morgenstern O et al (1944) Theory of games and economic behavior. Princeton University Press, Princeton
Nguyen H-L, Ng W-K, Woon Y-K (2014) Closed motifs for streaming time series classification. Knowl Inf Syst 41(1):101–125
Özorhan MO, Toroslu İH, Şehitoğlu OT (2016) A strength-biased prediction model for forecasting exchange rates using support vector machines and genetic algorithms. Soft Comput, 1–19
Pang S, Song L, Kasabov N (2011) Correlation-aided support vector regression for forex time series prediction. Neural Comput Appl 20(8):1193–1203
Paparrizos J, Gravano L (2015) k-shape: efficient and accurate clustering of time series. In: Proceedings of the 2015 ACM SIGMOD international conference on management of data, ACM, pp 1855–1870
Patel J, Shah S, Thakkar P, Kotecha K (2015) Predicting stock and stock price index movement using trend deterministic data preparation and machine learning techniques. Expert Syst Appl 42(1):259–268
Patel Pranav KEJ, Leonardi S (2002) Mining motifs in massive time series databases. In: Proceedings of the ICDM 2002, ICDM, pp 370–377
Poh K-L (2000) An intelligent decision support system for investment analysis. Knowl Inf Syst 2(3):340–358
Rakthanmanon T, Keogh EJ, Lonardi S, Evans S (2012) MDL-based time series clustering. Knowl Inf Syst 33(2):371–399
Risk/reward ratio (n.d.). http://www.investopedia.com/terms/r/riskrewardratio.asp. Accessed 13 Feb 2017
Shen F, Chao J, Zhao J (2015) Forecasting exchange rate using deep belief networks and conjugate gradient method. Neurocomputing 167:243–253
Smithsonian Agreement (n.d.). http://www.federalreservehistory.org/Events/DetailView/34. Accessed 17 Aug 2015
Son NT, Anh DT (2016) Discovery of time series k-motifs based on multidimensional index. Knowl Inf Syst 46(1):59–86
Tick-by-Tick Real-Time and Historical Market Rates (n.d.). http://www.truefx.com/. Accessed 13 Feb 2017
Villa S, Stella F (2014) A continuous time bayesian network classifier for intraday FX prediction. Quant Finance 14(12):2079–2092
Yao J, Tan CL (2000) A case study on using neural networks to perform technical forecasting of forex. Neurocomputing 34(1):79–98
Yuan C (2011) The exchange rate and macroeconomic determinants: time-varying transitional dynamics. North Am J Econ Finance 22(2):197–220
Zhang Liheng CA, Qi G-J (2017) Stock price prediction via discovering multi-frequency trading patterns. In: Proceedings of the 23rd ACM SIGKDD international conference on knowledge discovery and data mining, ACM, pp 2141–2149
Zhou Y, Lin S-C, Wang J-L (2018) Local and global temporal correlations for longitudinal data. J Multivar Anal 167:1–14
Author information
Authors and Affiliations
Corresponding author
Ethics declarations
Conflict of interest
The authors declare that they have no conflict of interest.
Ethical approval
This article does not contain any studies with human participants or animals performed by any of the authors.
Additional information
Publisher's Note
Springer Nature remains neutral with regard to jurisdictional claims in published maps and institutional affiliations.
A Appendix
A Appendix
1.1 A.1. Common forex terms
Definition A.1
(Take profit)—Take profit is a point in the time series where the trading system locks the profits and closes an open position. It is defined as a number of points from the initial price point.
For example, if a long transaction is expected to reach to a level 1.2500 at the end of the motif event horizon, that transaction can be terminated earlier if a level 1.3000 has been reached before arriving to the end of the event horizon, which gives even a better profit than the expected one. Notice that waiting for the end of the event horizon may produce better or worse result. The user may set the take profit level in terms of the expected value.
Definition A.2
(Stop loss)—Stop loss is a point in the time series where the trading system cuts the losses and closes an open position. Similar to the take profit, it is defined as a number of points from the initial price point.
This is the reverse of the take-profit value. Considering the same transaction, it can be terminated at level 1.2000 with a stop loss, accepting some loss before arriving to the event horizon. This decision can be made to prevent potentially higher losses. Notice that waiting for the event horizon may produce better or worse result. Also, the user sets the level in terms of the expected value.
Definition A.3
(Signal point)—Signal point is a point in the time series where the trading system opens a new position. The position can be a sell or buy position, depending on the underlying signal.
In our system, signal points are the last and completing points of detected motifs, which are all definitive Zigzag points. However, due to Zigzag’s backtracking mechanism, the last Zigzag point might be updated as long as it is not a signal point. The last Zigzag point in a motif becomes a signal point once the motif ends up in a cluster that has enough directional bias to be used for trading.
Consider a W-shaped motif where the 3rd peak is expected to be followed by an upward move. In real time, patterns are discovered in backward searches. So, at each time point, the data points must be searched, if this backward-searched sequence is similar to any of the motifs. For instance, if such a W-shaped motif has been found to be similar with the real data such that the current point corresponds to the last top point of W, this generates a signal for a long transaction to start (assuming 3rd peak is followed by and upward move).
Rights and permissions
About this article
Cite this article
Özorhan, M.O., Toroslu, İ.H. & Şehitoğlu, O.T. Short-term trend prediction in financial time series data. Knowl Inf Syst 61, 397–429 (2019). https://doi.org/10.1007/s10115-018-1303-x
Received:
Revised:
Accepted:
Published:
Issue Date:
DOI: https://doi.org/10.1007/s10115-018-1303-x