Functional Linear Partial Quantile Regression with Guaranteed Convergence for Neuroimaging Data Analysis
Authors (first, second and last of 6)
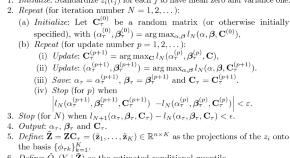
Collection
The last few years have seen a huge data collection increase in biological and biomedical research fields, such as biobanks, neuroimaging, spatial transcriptomics, single cell genomics, cancer genomics, and microbiomes. These biomedical data present numerous challenges and pitfalls for machine learning methods due to the complexity of data structures, the high dimensionality of biomarkers, and the heterogeneity of data resources. Novel statistical machine learning methods are demanded to address those problems in the context of translating clinical data into knowledge and practice. To join the force to improve data collection, quality control, and analysis in a wide range of biomedical fields, we plan to publish in this special issue manuscripts on new statistical machine learning methods that target on emerging questions in biomedical data analyses, such as sampling bias, biomarker discovery, high-dimension data analysis, streaming and online data analysis, domain adaptation, dimensional reduction methods, data integration techniques, data privacy, missing data methods, predictive models, transfer learning, causal and mediation inference, and statistical diversity and generalizability.
Call for Papers Flyer: Machine Learning in Biomedical Sciences
Dehan Kong is currently an assistant professor in the Department of Statistical Sciences and Department of Mathematical and Computational Sciences at the University of Toronto.
Bingxin Zhao is an assistant professor in the Wharton Statistics and Data Science Department at the University of Pennsylvania. He obtained his Ph.D. in Biostatistics from the University of North Carolina at Chapel Hill in 2020. Before joining the University of Pennsylvania, he was an assistant professor in the Department of Statistics at Purdue University.