Abstract
A higher-order dual for a non-differentiable minimax fractional programming problem is formulated. Using the generalized higher-order η-convexity assumptions on the functions involved, weak, strong and strict converse duality theorems are established in order to relate the primal and dual problems. Results obtained in this paper naturally unify and extend some previously known results on non-differentiable minimax fractional programming in the literature.
MSC:26A51, 90C32, 49N15.
Similar content being viewed by others
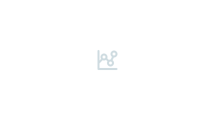
1 Introduction
The problem to be considered in the present analysis is the following nondifferentiable minimax fractional problem:
where Y is a compact subset of , , , and are continuously differentiable functions. B and C are positive semidefinite symmetric matrices. It is assumed that for each in , and .
In an earlier work, Schmittendorf [1] established necessary and sufficient optimality conditions for the following minimax programming problem:

where Y is a compact subset of , the functions , and are in .
Tanimoto [2] applied the necessary conditions in [1] to formulate a dual problem and discussed the duality results, which were extended to fractional analogue of (P1) by several authors [3–12]. Liu [13] proposed the second-order duality theorems for (P1) under generalized second-order B-invex functions. Mishra and Rueda [14] and Ahmad, Husain and Sarita [15] discussed the second-order duality results for the following nondifferentiable minimax programming problems:

where Y is a compact subset of , , and are twice differentiable functions. B is an positive semidefinite symmetric matrix. Ahmad, Husain and Sharma [16] formulated a unified higher-order dual of (P2) and established weak, strong and strict converse duality theorems under higher-order -Type I assumptions. Recently, Jayswal and Stancu-Minasian [17] obtained higher-order duality results for (P2).
In this paper, we formulate a higher-order dual of (P) and establish weak, strong and strict converse duality theorems under generalized higher-order η-convexity assumptions. More precisely, this paper is an extension of second-order duality results of Hu, Chen and Jian [18] to a class of higher-order duality and it also presents an answer to a question raised in [17].
2 Notation and preliminaries
Let denote the set of all feasible solutions of (NFP). Any point is called a feasible point of (NFP). For each , we define
such that for each ,
For each , we define
where

Since f and g are continuously differentiable and Y is compact in , it follows that for each , , and for any , we have a positive constant
Lemma 2.1 (Generalized Schwarz inequality)
Let A be a positive-semidefinite matrix of order n. Then, for all ,
The equality holds for some . Clearly, if , we have
We will use the following definitions.
Let and be differentiable functions.
Definition 2.1 [19]
A function ϕ is said to be higher-order η-convex if there exists a certain mapping such that for all , we have
Definition 2.2 [19]
A function ϕ is said to be higher-order (strictly) η-pseudoconvex if there exists a certain mapping such that for all , we have

Definition 2.3 [19]
A function ϕ is said to be higher-order (strictly) η-quasiconvex if there exists a certain mapping such that for all , we have

3 Higher-order duality model
In this section, we formulate the higher-order dual for (NFP) and derive duality results.
where denotes the set of all subject to




where , with and if . If for a triplet , the set , then we define the supremum over it to be ∞.
Remark 3.1 (i) Let , , , and , . Then (NMD) reduces to the second-order dual in [18, 20]. If, in addition, , then we get the dual formulated by Ahmad, Gupta, Kailey and Agarwal [5].
(ii) If , then the above dual becomes the dual formulated in [21].
Theorem 3.1 (Weak duality)
Let x and be feasible solutions of (NFP) and (NMD) respectively. Assume that
-
(i)
is higher-order η-pseudoconvex at z,
-
(ii)
, , is higher-order η-quasiconvex at z.
Then
Proof Suppose to the contrary that
Then we have
It follows from , , that
with at least one strict inequality since . Taking summation over i and using , we have
It follows from the generalized Schwarz inequality and (3.4) that
By the feasibility of x for (NFP)and , we obtain
The above inequality with (3.5) gives
Now, the feasibility of x for (NFP), and (3.3) yields
The inequality (3.8) and Hypothesis (ii) gives
From (3.1) and (3.9), we have

which by virtue of Hypothesis (i) yields

This contradicts (3.7). □
Theorem 3.2 (Strong duality)
Let be an optimal solution of (NFP) and let , be linearly independent. Assume that

Then there exist and such that is a feasible solution of (NMD) and the two objectives have the same values. Furthermore, if the assumptions of weak duality (Theorem 3.1) hold for all feasible solutions of (NFP) and (NMD), then is an optimal solution of (NMD).
Proof Since is an optimal solution of (NFP) and , are linearly independent, by the necessary conditions obtained in [13], there exist and such that is a feasible solution of (NMD) and the problems (NFP) and (NMD) have the same objectives values and
□
Theorem 3.3 (Strict converse duality)
Let and be the optimal solutions of (NFP) and (NMD), respectively. Assume that
-
(i)
, are linearly independent,
-
(ii)
is higher-order strictly η-pseudoconvex at ,
-
(iii)
, , is higher-order η-quasiconvex at .
Then, ; that is, is an optimal solution of (NFP).
Proof We will assume that and reach a contradiction. From the strong duality theorem (Theorem 3.2), it follows that
Now, proceeding as in Theorem 3.1, we get
and

By Hypothesis (ii), (3.2) and (3.13), we have
which contradicts (3.12). Hence the results. □
4 Concluding remarks
The notion of higher-order invexity is adopted, which includes many other generalized convexity concepts in mathematical programming as special cases. If we take , , , and , in Theorems 3.1-3.3, then we get Theorems 3.1-3.3 in [18].
The presented results in this paper can be further extended to the following related class of nondifferentiable minimax fractional programming problems:
where , for , . and are analytic with respect to ξ, W is a specified compact subset in , is a polyhedral cone in and is analytic. Also B, are positive semidefinite Hermitian matrices.
References
Schmitendorff WE: Necessary conditions and sufficient conditions for static minimax problems. J. Math. Anal. Appl. 1977, 57: 683–693. 10.1016/0022-247X(77)90255-4
Tanimoto S: Duality for a class of nondifferentiable mathematical programming problems. J. Math. Anal. Appl. 1981, 79: 283–294.
Ahmad I, Husain Z: Optimality conditions and duality in nondifferentiable minimax fractional programming with generalized convexity. J. Optim. Theory Appl. 2006, 129: 255–275. 10.1007/s10957-006-9057-0
Ahmad I, Husain Z: Duality in nondifferentiable minimax fractional programming with generalized convexity. Appl. Math. Comput. 2006, 176: 545–551. 10.1016/j.amc.2005.10.002
Ahmad I, Gupta SK, Kailey N, Agarwal RP:Duality in nondifferentiable minimax fractional programming with -invexity. J. Inequal. Appl. 2011, 2011: 75. 10.1186/1029-242X-2011-75
Chandra S, Kumar V: Duality in fractional minimax programming. J. Aust. Math. Soc. A 1995, 58: 376–386. 10.1017/S1446788700038362
Husain Z, Ahmad I, Sharma S: Second order duality for minimax fractional programming. Optim. Lett. 2009, 3: 277–286. 10.1007/s11590-008-0107-4
Lai HC, Liu JC, Tanaka K: Necessary and sufficient conditions for minimax fractional programming. J. Math. Anal. Appl. 1999, 230: 311–328. 10.1006/jmaa.1998.6204
Liu JC, Wu CS: On minimax fractional optimality conditions and invexity. J. Math. Anal. Appl. 1998, 219: 21–35. 10.1006/jmaa.1997.5786
Liu JC, Wu CS:On minimax fractional optimality conditions with -convexity. J. Math. Anal. Appl. 1998, 219: 36–51. 10.1006/jmaa.1997.5785
Yadav SR, Mukherjee RN: Duality in fractional minimax programming problems. J. Aust. Math. Soc. Series B, Appl. Math 1990, 31: 484–492. 10.1017/S0334270000006809
Yang XM, Hou SH: On minimax fractional optimality conditions and duality with generalized convexity. J. Glob. Optim. 2005, 31: 235–252. 10.1007/s10898-004-5698-4
Liu JC: Second-order duality for minimax programming. Util. Math. 1999, 56: 53–63.
Mishra SK, Rueda NG: Second order duality for nondifferentiable minimax programming involving generalized type I functions. J. Optim. Theory Appl. 2006, 130: 479–488. 10.1007/s10957-006-9113-9
Ahmad I, Husain Z, Sharma S: Second-order duality in nondifferentiable minimax programming involving type-I functions. J. Comput. Appl. Math. 2008, 215: 91–102. 10.1016/j.cam.2007.03.022
Ahmad I, Husain Z, Sharma S: Higher-order duality in nondifferentiable minimax programming with generalized type I functions. J. Optim. Theory Appl. 2009, 141: 1–12. 10.1007/s10957-008-9474-3
Jayswal A, Stancu-Minasian I: Higher-order duality in nondifferentiable minimax programming problem with generalized convexity. Nonlinear Anal., Theory Methods Appl. 2011, 74: 616–625. 10.1016/j.na.2010.09.016
Hu Q, Chen Y, Jian J: Second-order duality for nondifferentiable minimax fractional programming. Int. J. Comput. Math. 2012, 89: 11–16. 10.1080/00207160.2011.631529
Zhang, J: Generalized convexity and higher order duality for mathematical programming. Ph.D. Thesis, La Trobe University, Australia (1998)
Ahmad I: Second order nondifferentiable minimax fractional programming with square root terms. Filomat 2013, 27: 126–133.
Jin C, Ceng CZ: Higher-order duality for minimax fractional type programming involving symmetric matrices. Appl. Math. 2011, 2: 1387–1392. 10.4236/am.2011.211196
Acknowledgements
The research of the author is supported by the Internal Project No. IN111015 of King Fahd University of Petroleum and Minerals, Dhahran-31261, Saudi Arabia.
Author information
Authors and Affiliations
Corresponding author
Additional information
Competing interests
The author declares that he has no competing interest.
Rights and permissions
Open Access This article is distributed under the terms of the Creative Commons Attribution 2.0 International License (https://creativecommons.org/licenses/by/2.0), which permits unrestricted use, distribution, and reproduction in any medium, provided the original work is properly cited.
About this article
Cite this article
Ahmad, I. Higher-order duality in nondifferentiable minimax fractional programming involving generalized convexity. J Inequal Appl 2012, 306 (2012). https://doi.org/10.1186/1029-242X-2012-306
Received:
Accepted:
Published:
DOI: https://doi.org/10.1186/1029-242X-2012-306