Abstract
Educational studies are often focused on growth in student performance and background variables that can explain developmental differences across examinees. To study educational progress, a flexible latent variable model is required to model individual differences in growth given longitudinal item response data, while accounting for time-heterogenous dependencies between measurements of student performance. Therefore, an item response theory model, to measure time-specific latent traits, is extended to model growth using the latent variable technology. Following Muthén (Learn Individ Differ 10:73–101, 1998) and Azevedo et al. (Comput Stat Data Anal 56:4399–4412, 2012b), among others, the mean structure of the model represents developmental change in student achievement. Restricted covariance pattern models are proposed to model the variance–covariance structure of the student achievements. The main advantage of the extension is its ability to describe and explain the type of time-heterogenous dependency between student achievements. An efficient MCMC algorithm is given that can handle identification rules and restricted parametric covariance structures. A reparameterization technique is used, where unrestricted model parameters are sampled and transformed to obtain MCMC samples under the implied restrictions. The study is motivated by a large-scale longitudinal research program of the Brazilian Federal government to improve the teaching quality and general structure of schools for primary education. It is shown that the growth in math achievement can be accurately measured when accounting for complex dependencies over grades using time-heterogenous covariances structures.






Similar content being viewed by others
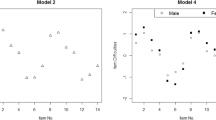
References
Albert, J.: Bayesian estimation of normal ogive item response curves using Gibbs sampling. J. Educ. Behav. Stat. 17, 251–269 (1992)
Albert, J.A., Chib, S.: Bayesian analysis of binary and polychotomous response data. J. Am. Stat. Assoc. 88, 669–679 (1993)
Albert, J.A., Chib, S.: Bayesian residual analysis for binary response regression models. Biometrika 82, 747–769 (1995)
Ando, T.: Bayesian predictive information criterion for the evaluation of hierarchical bayesian and empirical Bayes models. Biometrika 94, 443–458 (2007)
Andrade, D.F., Tavares, H.R.: Item response theory for longitudinal data: population parameter estimation. J. Multivar. Anal. 95, 1–22 (2005)
Azevedo, C.L.N.: Multilevel multiple group longitudinal models in item response theory: estimation methods and structural selection under a Bayesian perspective. unpublished PhD thesis, in Portuguese (2008)
Azevedo, C.L.N., Andrade, D.F.: An estimation method for latent trait and population parameters in nominal response model. Braz. J. Probab. Stat. 24, 415–433 (2010)
Azevedo, C.L.N., Bolfarine, H., Andrade, D.F.: Bayesian inference for a skew-normal IRT model under the centred parameterization. Comput. Stat. Data Anal. 55, 353–365 (2011)
Azevedo, C.L.N., Andrade, D.F., Fox, J.-P.: A Bayesian generalized multiple group IRT model with model-fit assessment tools. Comput. Stat. Data Anal. 56, 4399–4412 (2012b)
Azevedo, C.L.N., Bolfarine, H., Andrade, D.F.: Parameter recovery for a skew-normal IRT model under a bayesian approach: hierarchical framework, prior and kernel sensitivity and sample size. J. Stat. Comput. Simul. 82, 1679–1699 (2012a)
Bock, R.D., Aitkin, M.: Marginal maximum likelihood estimation of item parameters: an application of an EM algorithm. Psychometrika 46, 317–328 (1981)
Chib, S., Greenberg, E.: Analysis of multivariate probit models. Biometrics 85, 347–361 (1998)
Chib, S., Carlin, B.P.: On MCMC sampling in hierarchical longitudinal models. Stat. Comput. 9, 17–26 (1999)
Congdon, P.: Applied Bayesian Modelling. Wiley, Chichester (2003)
Conoway, M.R.: A: random effects model for binary data. Biometrics 46(1990), 317–328 (1990)
De Ayala, R., Sava-Bolesta, M.: Item parameter recovery for the nominal response model. Appl. Psychol. Meas. 23, 3–19 (1999)
DeMars, C.E.: Sample size and the recovery of nominal response model item parameters. Appl. Psychol. Meas. 27, 275288 (2003)
Douglas, J.A.: Item response models for longitudinal quality of life data in clinical trials. Stat. Med. 18, 2917–2931 (1999)
Dunson, D.B.: Dynamic latent trait models for multidimensional longitudinal data. J. Am. Stat. Assoc. 98, 555–563 (2003)
Eid, M.: Longitudinal confirmatory factor analysis for polytomous item responses : model definition and model selection on the basis of stochastic measurement theory. Methods Psychol. Res. Online 1, 65–85 (1996)
Fitzmaurice, G., Davidian, M., Verbeke, D., Molenberghs, G.: Longitudinal Data Analysis, 1st edn. Chapman & Hall/CRC, London (2008)
Fox, J.-P.: Multilevel IRT assessment. In: van der Ark, M., Sijtsma, K. (eds.) New Developments in Categorical Data Analysis for the Social and Behavioral Sciences, pp. 227–252. Lawrence Erlbaum Associates, Inc., London (2004)
Fox, J.-P., Glas, C.A.W.: Bayesian modification indices for IRT models. Stat. Neerl. 59, 95–106 (2005)
Fox, J.-P.: Bayesian Item Response Modeling: Theory and Applications, 1st edn. Springer, New York (2010)
Gamerman, D., Lopes, H.: Markov Chain Monte Carlo : Stochastic Simulation for Bayesian Inference, 2nd edn. Chapman & Hall/CRC, London (2006)
Gelman, A., Carlin, J.B., Stern, H.S., Rubin, D.B.: Bayesian Data Analysis, 2nd edn. Chapman & Hall/CRC, London (2004)
Gelman, A.: Prior distribution for variance parameters in hierarchical models. Bayesian Anal. 1, 515–533 (2006)
Green, P.J.: Reversible jump Markov chain Monte Carlo computation and Bayesian model determination. Biometrika 82, 711–732 (1995)
Hedeker, D., Gibbons, R.D.: Longitudinal Data Analysis, 1st edn. Wiley Series, New York (2006)
Imai, K., van Dyk, D.A.: A Bayesian analysis of the multinomial probit model using marginal data augmentation. J. Econom. 124, 311–334 (2005)
Jennrich, R.I., Schluchter, M.D.: Unbalanced repeated-measures models with structured covariance matrices. Biometrics 42, 805–820 (1986)
Kass, R.E., Raftery, A.E.: Bayes factors. J. Am. Stat. Assoc. 90, 773–795 (1995)
Liu, L.C., Hedeker, D.: A mixed-effects regression model for longitudinal multivariate ordinal data. Biometrics 62, 261–268 (2006)
McCulloh, R., Polson, N.G., Rossi, P.E.: A Bayesian analysis of the multinomial probit model with fully identified parameters. J. Econom. 99, 173–193 (2000)
Muthén, B.O.: Longitudinal studies of achievement growth using latent variable modeling. Learn. Individ. Differ. 10, 73–101 (1998)
Nunez-Anton, V., Zimmerman, D.L.: Modelinng nonstationary longitudinal data. Biometrics 56, 699–705 (2000)
Rencher, R.C.: Methods of Multivariate Analysis, 1st edn. Wiley Series, New York (2002)
Rochon, J.: Arma covariance structures with time heterocedasticity for repeated measures experiments. J. Am. Stat. Assoc. 87, 777–784 (1992)
Sahu, S.K.: Bayesian estimation and model choice in item response models. J. Stat. Comput. Simul. 72, 217–232 (2002)
Singer, J.M., Andrade, D.F.: On the choice of appropriate error terms in profile analysis. Statistician 43, 259–266 (1994)
Singer, J.M., Andrade, D.F.: Analysis of longitudinal data. In: Sen, P.K., Rao, C.R. (eds.) Handbook of Statistics 18, Bioenvironmental and Public Health Statistics, pp. 115–160. Elsevier, Amsterdam (2000)
Sinharay, S.: A Bayesian item fit analysis for unidimensional item response theory models. Br. J. Math. Stat. Psychol. 59, 429–449 (2006)
Sinharay, S., Johnson, M.S., Stern, H.: Posterior predictive assessment of item response theory models. Appl. Psychol. Meas. 30, 298–321 (2006)
Spiegelhalter, D.J., Best, N.G., Carlin, B.P., van der Linde, A.: Bayesian measures of model complexity and fit. J. R. Stat. Soc. Ser. B 64, 583–639 (2002)
Stern, H.S., Sinharay, S.: Bayesian model checking and model diagnostics. In: Dey, D.D., Rao, C.R. (eds.) Handbook of Statistics 25, Bayesian Modelling, Thinking and Computation, pp. 171–192. Elsevier, Amsterdam (2005)
Tavares, H.R., Andrade, D.F.: Item response theory for longitudinal data: item and population ability parameters estimation. Test 15, 97–123 (2006)
Tiao, G.C., Zellner, A.: On the Bayesian estimation of multivariate regression. J. R. Stat. Soc. B 26, 277–285 (1964)
Acknowledgments
The authors are thankfull to CNPq (Conselho Nacional de Desenvolvimento Científico e Tecnológico) from Brazil, for the financial support through a Doctoral Sandwich Scholarship granted to the first author under the guidance of the two others
Author information
Authors and Affiliations
Corresponding author
Appendix
Appendix
-
Step 1: Simulate the augmented data using \(Z_{ijt}|(.)\), according to Eq. (22).
-
Step 2: Simulate the latent traits using
$$\begin{aligned} \varvec{\theta }_{j.} |(.) \sim N_T(\widehat{\varvec{\varPsi }}_{\varvec{\theta }_j}\widehat{\varvec{\theta }}_j,\widehat{\varvec{\varPsi }}_{\varvec{\theta }_j}) \end{aligned}$$where
$$\begin{aligned}&\widehat{\varvec{\theta }}_j = \sum _{i \mid I_{ijt}=1} a_i b_i \varvec{1}_{T} + \sum _{i \mid I_{ijt}=1} a_i \varvec{z}_{ij.} + \varvec{\varPsi }_{\varvec{\theta }}^{-1}\varvec{\mu }_{\varvec{\theta }}\,,\nonumber \\ \widehat{\varvec{\varPsi }}_{\varvec{\theta }_j}&= \left( \sum _{i \mid I_{ijt}=1}a_i^2 \varvec{I}_{T} + \varvec{\varPsi }_{\varvec{\theta }}^{-1}\right) ^{-1}\,, \end{aligned}$$where \(\varvec{z}_{ij.} = (z_{ij1},\ldots ,z_{ijT})^{t}\).
-
Step 3: Simulate the item parameters by using \(\varvec{\zeta }_i|(.) \sim N(\widehat{\varvec{\varPsi }}_{\varvec{\zeta }_i}\widehat{\varvec{\zeta }}_i,\widehat{\varvec{\varPsi }}_{\varvec{\zeta }_i})\), mutually indepedently, where
$$\begin{aligned}&\widehat{\varvec{\zeta }}_i = \varvec{H}_{i..}^{t}\varvec{z}_{i..} + \varvec{\varPsi }_{\varvec{\zeta }}^{-1}\varvec{\mu }_{\varvec{\zeta }}\,,\nonumber \\&\widehat{\varvec{\varPsi }}_{\varvec{\zeta }_i} = \left( \varvec{H}_{i..}^{t}\varvec{H}_{i..} + \varvec{\varPsi }_{\varvec{\zeta }}^{-1}\right) ^{-1}\,,\nonumber \\&\varvec{H}_{i..} = [\varvec{\theta }\,\,\, -\varvec{1}]\bullet \mathbf {I}_{i}\,, \end{aligned}$$(30)where \(\mathbf {I}_i\) is the indicator vector of item \(i\), which indicates the subjects responding to item \(i\) and “\(\bullet \)” is the Hadamard product.
-
Step 4: Simulate the population mean vector by using
$$\begin{aligned}&\mu _{\theta _1}|(.)\sim N(\widetilde{\mu }_{\theta _{1}},\widehat{\psi }_{\mu })\,,\\&\varvec{\mu }_{\varvec{\theta }(1)}|(\mu _{\theta _1},(.)) \sim N_T(\widetilde{\varvec{\mu }}_{\varvec{\theta }(T-1)},\widehat{\varvec{\varPsi }}_{\varvec{\mu }_{(T-1)}})\,, \end{aligned}$$where
$$\begin{aligned}&\widehat{\varvec{\mu }}_{\varvec{\theta }} = \varvec{\varPsi }_{\varvec{\theta }}^{-1} \sum _{j=1}^n\varvec{\theta }_{j.} + \varvec{\varPsi }_{0}^{-1}\varvec{\mu }_{\varvec{\theta }}\\&\quad \quad =(\widehat{\mu }_{\theta _1},\widehat{\mu }_{\theta _2},\ldots ,\widehat{\mu }_{\theta _T})^{t}( \widehat{\mu }_{\theta _1}, \widehat{\varvec{\mu }}_{\varvec{\theta }}^{(T-1)})^{t}\,,\\&\widehat{\varvec{\varPsi }}_{\varvec{\mu }}= \left( n\varvec{\varPsi }_{\varvec{\theta }}^{-1} \!+\! \varvec{\varPsi }_{\varvec{\mu }}^{-1}\right) ^{-1} \!= \!\left[ \begin{array}{c@{\quad }c} \widehat{\psi }_{\mu } &{} \widehat{\varvec{\psi }}_{\varvec{\mu }}^{t\,(T-1)}\\ \widehat{\varvec{\psi }}_{\varvec{\mu }}^{(T-1)} &{} \widehat{\varvec{\varPsi }}_{\varvec{\mu }}^{(T-1)} \end{array}\right] \,,\\&\widetilde{\varvec{\mu }}_{\varvec{\theta }} = \widehat{\varvec{\varPsi }}_{\varvec{\mu }}\widehat{\varvec{\mu }}_{\varvec{\theta }} = (\widetilde{\mu }_{\theta _1},\widetilde{\mu }_{\theta _2},\ldots ,\widetilde{\mu }_{\theta _T})^{t}\\&\quad \quad = ( \widetilde{\mu }_{\theta _1}, \widetilde{\varvec{\mu }}_{\varvec{\theta }}^{(T-1)})^{t}\,,\\&\widetilde{\varvec{\mu }}_{\varvec{\theta }(T-1)} = \widetilde{\varvec{\mu }}_{\varvec{\theta }}^{(T-1)} + \widehat{\psi }_{\mu }^{-1}\widehat{\varvec{\psi }}_{\varvec{\mu }}^{(T-1)}(\mu _{\theta _1} - \widetilde{\mu }_{\theta _1})\,,\\&\widehat{\varvec{\varPsi }}_{\varvec{\mu }(T-1)} = \widehat{\varvec{\varPsi }}_{\varvec{\mu }}^{(T-1)} - \widehat{\psi }_{\mu }^{-1}\widehat{\varvec{\psi }}_{\varvec{\mu }}^{(T-1)}\widehat{\varvec{\psi }}_{\varvec{\mu }}^{t\,(T-1)}\,. \end{aligned}$$ -
Step 5: Simulate the first time point variance using \(\psi _{\theta _1} |(.) \sim IG(\widehat{\upsilon }_0,\widehat{\kappa }_0)\), where
$$\begin{aligned} \widehat{\upsilon }_1&= \frac{n + \upsilon _0}{2}\,,\\ \widehat{\kappa }_1&= \frac{\sum _{j=1}^{n}(\theta _{j1} - \mu _{\theta _1})^2 + \kappa _0}{2}\,. \end{aligned}$$ -
Step 6: Simulate the vector of covariances using \(\varvec{\psi }^* \sim N_{T-1}(\widehat{\varvec{\varPsi }}_{\varvec{\psi }}\widehat{\varvec{\psi }}_{\varvec{\psi }},\widehat{\varvec{\varPsi }}_{\varvec{\psi }})\), where
$$\begin{aligned} \widehat{\varvec{\psi }}_{\varvec{\psi }}&= \psi _{\theta _1}^{-1/2}\varvec{\varPsi }_{\varvec{\theta }}^{*\,-1}\sum _{j=1}^n\left( \varvec{\theta }_{j(1)}- \varvec{\mu }_{\varvec{\theta }(1)}\right) \left( \theta _{j1} - \mu _{\theta _1}\right) \\&+\,\, \varvec{\varPsi }_{\varvec{\psi }}^{-1}\varvec{\mu }_{\varvec{\psi }},\\ \widehat{\varvec{\varPsi }}_{\varvec{\psi }}&= \left( \psi _{\theta _1}^{-1}\varvec{\varPsi }_{\varvec{\theta }}^{*\,-1}\sum _{j=1}^n\left( \theta _{j1} - \mu _{\theta _1}\right) ^2 + \varvec{\varPsi }_{\varvec{\psi }}^{-1}\right) ^{-1}\,. \end{aligned}$$ -
Step 7: Simulate the covariance matrix \(\varvec{\varPsi }^* \sim IW_{T-1}\) \((\widehat{\nu }_{\varvec{\varPsi }},\widehat{\varvec{\varPsi }}_{\varvec{\varPsi }})\), where
$$\begin{aligned} \widehat{\nu }_{\varvec{\varPsi }}&= n + \nu _{\varvec{\varPsi }}\,,\\ \widehat{\varvec{\varPsi }}_{\varvec{\varPsi }}&= \varvec{\varPsi }_{\varvec{\varPsi }} + \sum _{j=1}^{n}\left( \varvec{\theta }_{j(1)} - \varvec{\mu }_{\varvec{\theta }}^*\right) \left( \varvec{\theta }_{j(1)} - \varvec{\mu }_{\varvec{\theta }}^*\right) ^{t}\,. \end{aligned}$$ -
Step 8: Calculate the original covariance matrix using (10) and \(\varvec{\varPsi }_{\varvec{\theta }(1)} = \varvec{\varPsi }^* + \varvec{\psi }^*\varvec{\psi }^{*^{t}}\).
-
Step 9: Calculate the population variances using
$$\begin{aligned} (\psi _{\theta _2},\ldots ,\psi _{\theta _T})^{t}= \varvec{\psi }_{\varvec{\theta }(1)}^* = Diag(\varvec{\varPsi }^* + \varvec{\psi }^*\varvec{\psi }^*{^{t}})\,, \end{aligned}$$(31)where \(Diag\) extracts the main diagonal of a square matrix.
-
Step 10: Depending on the restricted covariance structure of interest, transformations are defined for unrestricted parameters to facilitate draws of restricted model parameters. Below, in each subitem, the following notation is used: \(\varvec{\psi }_{\varvec{\theta }(1)}^*\) is given by (31), “\(\bullet \)” denotes the Hadamard product, \((.)^{-1/2}\) is an inverse-square-root pointwise operator, and \(\varvec{A}{[t]}\) and \(\varvec{A}{[t:]}\) denotes the \(t\)-th component and the remaining values of the vector \(\varvec{A}\), starting at \(t\), respectively.
-
ARH and UH: Calculate the correlation coefficient using
$$\begin{aligned} \rho _{\theta }&= \frac{1}{T-1}\varvec{1}_{T-1}^{t}\left( \varvec{\psi }^*\bullet (\varvec{\psi }_{\varvec{\theta }(1)}^*)^{-1/2}\right) \,. \end{aligned}$$(32) -
HT: Calculate the correlation coefficient using
$$\begin{aligned} \rho _{\theta }&= \varvec{\psi }^*[1]\times (\varvec{\psi }_{\varvec{\theta }(1)}^*[1])^{-1/2}\,. \end{aligned}$$(33) -
HC: Calculate the covariance parameter using
$$\begin{aligned} \rho _{\theta }&= \frac{1}{T-1}\varvec{1}_{T-1}^{t}\left( \sqrt{\psi _{\theta _1}}\varvec{\psi }^*\right) \,. \end{aligned}$$(34) -
ARMAH: Calculate the moving average parameter (\(\gamma _{\theta }\)) using
$$\begin{aligned} \gamma _{\theta } = \varvec{\psi }^*[1]\times (\varvec{\psi }_{\varvec{\theta }(1)}^*[1])^{-1/2} \end{aligned}$$(35)and the correlation parameter (\(\rho _{\theta }\)) using
$$\begin{aligned} \rho _{\theta } \!=\!\frac{1}{T-2}\varvec{1}_{T\!-\!1}^{t}\left( \varvec{\psi }^*[T-2:]\bullet (\varvec{\psi }_{\varvec{\theta }(1)}^*[T-2:])^{\!-\!1/2}\right) .\nonumber \\ \end{aligned}$$(36) -
AD: Calculate the correlation parameter using
$$\begin{aligned} \rho _{\theta _1} = \varvec{\psi }^*[1]\times (\varvec{\psi }_{\varvec{\theta }(1)}^*[1])^{-1/2} \end{aligned}$$(37)and, for \(t=2,...,T-1\), using
$$\begin{aligned} \rho _{\theta _t} = \frac{\varvec{\psi }^*[t:]\times (\varvec{\psi }_{\varvec{\theta }(1)}^*[t:])^{-1/2}}{\prod _{t'=1}^{t-1}\rho _{\theta _{t'}}}. \end{aligned}$$(38) -
Step 11: A specific covariance pattern model is computed using the appropriate restriction on the free parameters sampled from their joint distribution. The computed restricted covariance matrix is used in the repeating MCMC Steps.
The unstructured covariance matrix is the least restrictive version, and assumes unique variance and covariance parameters for the measurements of theta over time. Each structured covariance pattern is a restricted version of the unrestricted covariance pattern. The parameter space defined by the unstructured covariance pattern model represents all possible combinations of the different parameters. Therefore, this parameter space will contain all possible combinations of parameters of each restricted covariance pattern model. This property is explicitly used in the present sampling procedure. That is; each restriction will be used to imply a relationship between the parameters sampled from their joint distribution. Each relationship is implied to restrict the free parameters, which are sampled from their joint distribution, where the restriction implies a common covariance or a function of the common covariance parameter, which is defined by the set of free covariance parameters.
By sampling parameters of the unrestricted covariance pattern, potentially all possible restricted versions can be drawn. In the procedure, a restricted version is computed from the unstructured sampled covariance parameters and the restricted set of parameters are considered to be the implied restricted sample from the unrestricted sample. Since all possible restricted samples are generated from all free possible combinations of parameters, the restricted sample is obtained from the parameter space of all possible combinations of the different parameters of the restricted covariance pattern model.
Rights and permissions
About this article
Cite this article
Azevedo, C.L.N., Fox, JP. & Andrade, D.F. Bayesian longitudinal item response modeling with restricted covariance pattern structures. Stat Comput 26, 443–460 (2016). https://doi.org/10.1007/s11222-014-9518-5
Received:
Accepted:
Published:
Issue Date:
DOI: https://doi.org/10.1007/s11222-014-9518-5