Abstract
The ubiquitin–proteasome pathway (UPP) plays an important role in the degradation of cellular proteins and regulation of different cellular processes that include cell cycle control, proliferation, differentiation, and apoptosis. In this sense, the disruption of proteasome activity leads to different pathological states linked to clinical disorders such as inflammation, neurodegeneration, and cancer. The use of UPP inhibitors is one of the proposed approaches to manage these alterations. On other hand, the ChEMBL database contains >5,000 experimental outcomes for >2,000 compounds tested as possible proteasome inhibitors using a large number of pharmacological assay protocols. All these assays report a large number of experimental parameters of biological activity like \(EC_{50}, IC_{50}\), percent of inhibition, and many others that have been determined under many different conditions, targets, organisms, etc. Although this large amount of data offers new opportunities for the computational discovery of proteasome inhibitors, the complexity of these data represents a bottleneck for the development of predictive models. In this work, we used linear molecular indices calculated with the software TOMOCOMD-CARDD and Box–Jenkins moving average operators to develop a multi-output model that can predict outcomes for 20 experimental parameters in >450 assays carried out under different conditions. This generated multi-output model showed values of accuracy, sensitivity, and specificity above 70 % for training and validation series. Finally, this model is considered multi-target and multi-scale, because it predicts the inhibition of the UPP for drugs against 22 molecular or cellular targets of different organisms contained in the ChEMBL database.


Similar content being viewed by others
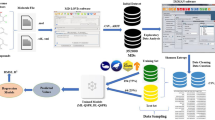
References
Ciechanover A (2005) Proteolysis: from the lysosome to ubiquitin and the proteasome. Nat Rev Mol Cell Biol 6:79–87. doi:10.1038/nrm1552
Tu Y, Chen C, Pan J, Xu J, Zhou ZG, Wang CY (2012) The ubiquitin proteasome pathway (UPP) in the regulation of cell cycle control and DNA damage repair and its implication in tumorigenesis. Int J Clin Exp Pathol 5:726–738
Zhang J, Wu P, Hu Y (2013) Clinical and marketed proteasome inhibitors for cancer treatment. Curr Med Chem 20:2537–2551. doi:10.2174/09298673113209990122
Pevzner Y, Metcalf R, Kantor M, Sagaro D, Daniel K (2013) Recent advances in proteasome inhibitor discovery. Expert Opin Drug Discov 8:537–568. doi:10.1517/17460441.2013.780020
Heikamp K, Bajorath J (2011) Large-scale similarity search profiling of ChEMBL compound data sets. J Chem Inf Model 51:1831–1839. doi:10.1021/ci200199u
Gaulton A, Bellis LJ, Bento AP, Chambers J, Davies M, Hersey A, Light Y, McGlinchey S, Michalovich D, Al-Lazikani B, Overington JP (2012) ChEMBL: a large-scale bioactivity database for drug discovery. Nucleic Acids Res 40:D1100–D1107. doi:10.1093/nar/gkr777
Mok NY, Brenk R (2011) Mining the ChEMBL database: an efficient chemoinformatics workflow for assembling an ion channel-focused screening library. J Chem Inf Model 51:2449–2454. doi:10.1021/ci200260t
Hu Y, Bajorath J (2010) Molecular scaffolds with high propensity to form multi-target activity cliffs. J Chem Inf Model 50:500–510. doi:10.1021/ci100059q
Erhan D, L’Heureux PJ, Yue SY, Bengio Y (2006) Collaborative filtering on a family of biological targets. J Chem Inf Model 46:626–635. doi:10.1021/ci050367t
Namasivayam V, Hu Y, Balfer J, Bajorath J (2013) Classification of compounds with distinct or overlapping multi-target activities and diverse molecular mechanisms using emerging chemical patterns. J Chem Inf Model 53:1272–1281. doi:10.1021/ci400186n
Tenorio-Borroto E, Garcia-Mera X, Penuelas-Rivas CG, Vasquez-Chagoyan JC, Prado-Prado FJ, Castanedo N, Gonzalez-Diaz H (2013) Entropy model for multiplex drug–target interaction endpoints of drug immunotoxicity. Curr Top Med Chem 13:1636–1649. doi:10.2174/15680266113139990114
Box GEP, Jenkins GM (1970) Time series analysis: forecasting and control. Holden-Day, San Francisco
Speck-Planche A, Kleandrova VV, Cordeiro MN (2013) Chemoinformatics for rational discovery of safe antibacterial drugs: simultaneous predictions of biological activity against streptococci and toxicological profiles in laboratory animals. Bioorg Med Chem 21:2727–2732. doi:10.1016/j.bmc.2013.03.015
Speck-Planche A, Kleandrova VV, Luan F, Cordeiro MN (2012) Chemoinformatics in multi-target drug discovery for anti-cancer therapy: in silico design of potent and versatile anti-brain tumor agents. Anti-Cancer Agent Med Chem 12:678–685. doi:10.2174/187152012800617722
Speck-Planche A, Kleandrova VV, Luan F, Cordeiro MN (2012) Chemoinformatics in anti-cancer chemotherapy: multi-target QSAR model for the in silico discovery of anti-breast cancer agents. Eur J Pharm Sci 47:273–279. doi:10.1016/j.ejps.2012.04.012
Marrero-Ponce Y, Valdés-Martini JR, Jacas CRG (2012) TOMOCOMD-CARDD. QuBiLS Software QUBILs-MAS. Version 1.0, CAMD-BIR Unit, Universidad Central “Marta Abreu” de Las Villas
Marrero-Ponce Y, Medina-Marrero R, Castillo-Garit JA, Romero-Zaldivar V, Torrens F, Castro EA (2005) Protein linear indices of the ‘macromolecular pseudograph alpha-carbon atom adjacency matrix’ in bioinformatics. Part 1: prediction of protein stability effects of a complete set of alanine substitutions in Arc repressor. Bioorg Med Chem 13:3003–3015. doi:10.1016/j.bmc.2005.01.062
Marrero-Ponce Y, Castillo-Garit JA, Torrens F, Romero-Zaldivar V, Castro E (2004) Atom, atom-type, and total linear indices of the “molecular pseudograph’s atom adjacency matrix”: application to QSPR/QSAR studies of organic compounds. Molecules 9:1100–1123. doi:10.3390/91201100
Marrero-Ponce Y, Medina-Marrero R, Martinez Y, Torrens F, Romero-Zaldivar V, Castro EA (2006) Non-stochastic and stochastic linear indices of the molecular pseudograph’s atom adjacency matrix: a novel approach for computational -in silico- screening and “rational” selection of new lead antibacterial agents. J Mol Mod 12:255–271. doi:10.1007/s00894-005-0024-8
Rescigno A, Casañola-Martin GM, Sanjust E, Zucca P, Marrero-Ponce Y (2011) Vanilloid derivatives as tyrosinase inhibitors driven by virtual screening-based QSAR models. Drug Test Anal 3:176–181. doi:10.1002/dta.187
Vega MC, Montero-Torres A, Marrero-Ponce Y, Rolón M, Gómez-Barrio A, Escario JA, Arán VJ, Nogal JJ, Meneses-Marcel A, Torrens F (2006) New ligand-based approach for the discovery of antitrypanosomal compounds. Bioorg Med Chem Lett 16:1898–1904. doi:10.1016/j.bmcl.2005.12.087
Brito-Sánchez Y, Castillo-Garit JA, Le-Thi-Thu H, González-Madariaga Y, Torrens F, Marrero-Ponce Y, Rodríguez-Borges JE (2013) Comparative study to predict toxic modes of action of phenols from molecular structures. SAR QSAR Environ Res 24:235–251. doi:10.1080/1062936x.2013.766260
Marrero-Ponce Y, Castillo-Garit JA, Nodarse D (2005) Linear indices of the ‘macromolecular graph’s nucleotides adjacency matrix’ as a promising approach for bioinformatics studies. Part 1: prediction of paromomycin’s affinity constant with HIV-1 psi-RNA packaging region. Bioorg Med Chem 13:3397–3404. doi:10.1016/j.bmc.2005.03.010
Marrero-Ponce Y, Medina-Marrero R, Castillo-Garit JA, Romero-Zaldivar V, Torrens F, Castro EA (2005) Protein linear indices of the ‘macromolecular pseudograph \(\alpha \)-carbon atom adjacency matrix’ in bioinformatics. Part 1: prediction of protein stability effects of a complete set of alanine substitutions in Arc repressor. Bioorg Med Chem 13:3003–3015. doi:10.1016/j.bmc.2005.01.062
Luan F, Cordeiro MN, Alonso N, Garcia-Mera X, Caamano O, Romero-Duran FJ, Yanez M, Gonzalez-Diaz H (2013) TOPS-MODE model of multiplexing neuroprotective effects of drugs and experimental-theoretic study of new 1,3-rasagiline derivatives potentially useful in neurodegenerative diseases. Bioorg Med Chem 21:1870–1879. doi:10.1016/j.bmc.2013.01.035
Marzaro G, Chilin A, Guiotto A, Uriarte E, Brun P, Castagliuolo I, Tonus F, Gonzalez-Diaz H (2011) Using the TOPS-MODE approach to fit multi-target QSAR models for tyrosine kinases inhibitors. Eur J Med Chem 46:2185–2192. doi:10.1016/j.ejmech.2011.02.072
Alonso N, Caamano O, Romero-Duran FJ, Luan F, Dias Soeiro Cordeiro MN, Yanez M, Gonzalez-Diaz H, Garcia-Mera X (2013) Model for high-throughput screening of multi-target drugs in chemical neurosciences; synthesis, assay and theoretic study of rasagiline carbamates. ACS Chem Neurosci 4:1393–1403. doi:10.1021/cn400111n
Marrero-Ponce Y, Castillo-Garit JA, Olazabal E, Serrano HS, Morales A, Castanedo N, Ibarra-Velarde F, Huesca-Guillen A, Sanchez AM, Torrens F, Castro EA (2005) Atom, atom-type and total molecular linear indices as a promising approach for bioorganic and medicinal chemistry: theoretical and experimental assessment of a novel method for virtual screening and rational design of new lead anthelmintic. Bioorg Med Chem 13:1005–1020. doi:10.1016/j.bmc.2004.11.040
Marrero-Ponce Y, Machado-Tugores Y, Pereira DM, Escario JA, Barrio AG, Nogal-Ruiz JJ, Ochoa C, Aran VJ, Martinez-Fernandez AR, Sanchez RN, Montero-Torres A, Torrens F, Meneses-Marcel A (2005) A computer-based approach to the rational discovery of new trichomonacidal drugs by atom-type linear indices. Curr Drug Discov Technol 2:245–265. doi:10.2174/157016305775202955
Concu R, Dea-Ayuela MA, Perez-Montoto LG, Prado-Prado FJ, Uriarte E, Bolas-Fernandez F, Podda G, Pazos A, Munteanu CR, Ubeira FM, Gonzalez-Diaz H (2009) 3D entropy and moments prediction of enzyme classes and experimental–theoretic study of peptide fingerprints in Leishmania parasites. Biochim Biophys Acta 1794:1784–1794. doi:10.1016/j.bbapap.2009.08.020
Speck-Planche A, Kleandrova VV, Luan F, Cordeiro MN (2011) Multi-target drug discovery in anti-cancer therapy: fragment-based approach toward the design of potent and versatile anti-prostate cancer agents. Bioorg Med Chem 19:6239–6244. doi:10.1016/j.bmc.2011.09.015
Tenorio-Borroto E, Rivas CGP, Chagoyan JCV, Castanedo N, Prado-Prado FJ, Garcia-Mera X, Gonzalez-Diaz H (2012) ANN multiplexing model of drugs effect on macrophages; theoretical and flow cytometry study on the cytotoxicity of the anti-microbial drug G1 in spleen. Bioorg Med Chem. doi: 10.1016/j.bmc.2012.07.020
Hill T, Lewicki P (2006) Statistics: methods and applications: a comprehensive reference for science, industry and data mining. StatSoft, Tulsa
StatSoft. Inc. (2002) STATISTICA (data analysis software system), version 6.0
Tabachnick BG, Fidell LS (1996) Using multivariate statistics. HarperCollins College, NewYork
Duart MJ, García-Domenech R, Anton-Fos GM, Galvez J (2001) Optimization of a mathematical topological pattern for the prediction of antihistaminic activity. J Comput Aided Mol Des 15:561–572. doi:10.1023/A:1011115824070
Prado-Prado FJ, Escobar M, García-Mera X (2013) Review of bioinformatics and theoretical studies of acetylcholinesterase inhibitors. Curr Bioinform 8:496–510. doi:10.2174/1574893611308040012
García-Domenech R, Zanni R, Galvez-Llompart M, De Julián-Ortiz JV (2013) Modeling anti-allergic natural compounds by molecular topology. Comb Chem High Throughput Screen 16:628–635. doi:10.2174/1386207311316080005
Helguera AM, Pérez-Garrido A, Gaspar A, Reis J, Cagide F, Vina D, Cordeiro MNDS, Borges F (2013) Combining QSAR classification models for predictive modeling of human monoamine oxidase inhibitors. Eur J Med Chem 59:75–90. doi:10.1016/j.ejmech.2012.10.035
Mai Q (2013) A review of discriminant analysis in high dimensions. Wiley Interdisciplin Rev Computat Statist 5:190–197. doi:10.1002/wics.1257
StatSoft Inc. (2001) STATISTICA (data analysis software system) vs 6.0. StatSoft Inc., Tulsa
Gerets HH, Dhalluin S, Atienzar FA (2011) Multiplexing cell viability assays. Methods Mol Biol 740:91–101. doi:10.1007/978-1-61779-108-6-11
Casañola-Martin GM, Le-Thi-Thu H, Marrero-Ponce Y, Castillo-Garit JA, Torrens F, Perez-Gimenez F, Abad C (2014) Analysis of proteasome inhibition prediction using atom-based quadratic indices enhanced by machine learning classification techniques. Lett Drug Des Discov 11:705–711. doi:10.2174/1570180811666140122001144
Oprea TI (2002) Current trends in lead discovery: are we looking for the appropiate properties? J Comput Aid Mol Des 16:325–334. doi:10.1023/A:1020877402759
Xu J, Hagler A (2002) Chemoinformatics and drug discovery. Molecules 7:566–700. doi:10.3390/70800566
Seifert HJM, Wolf K, Vitt D (2003) Virtual high-throughput in silico screening. Biosilico 1:143–149. doi:10.1016/S1478-5382(03)02359-X
Acknowledgments
Casañola-Martin, G. M. thanks the program Estades Temporals per a Investigadors Convidats for a fellowship to research at Valencia University (2013–2014). Le-Thi-Thu, H. gratefully acknowledges the support from the National Vietnam National University, Hanoi. Marrero-Ponce, Y. thanks the International Professor program for a fellowship to work at Cartagena University in the year 2013–2014. Also, thanks to Prof. Aroa Reguero from the Pontifical Catholic University of Ecuador in Esmeraldas (PUCESE) for her help in the review of the manuscript. Finally, the authors also thank the anonymous referees and editor for their useful comments that contributed to the improvement of this work.
Author information
Authors and Affiliations
Corresponding authors
Electronic supplementary material
Below is the link to the electronic supplementary material.
Rights and permissions
About this article
Cite this article
Casañola-Martin, G.M., Le-Thi-Thu, H., Pérez-Giménez, F. et al. Multi-output model with Box–Jenkins operators of linear indices to predict multi-target inhibitors of ubiquitin–proteasome pathway. Mol Divers 19, 347–356 (2015). https://doi.org/10.1007/s11030-015-9571-9
Received:
Accepted:
Published:
Issue Date:
DOI: https://doi.org/10.1007/s11030-015-9571-9