Abstract
This manuscript discusses the concurrent voltage and frequency control problem for an interconnected system having three areas, each containing thermal and diesel units in conjunction with renewable integration from a separate source. In the first case study, renewable sources like wind, solar, and geothermal have been incorporated using a static transfer function-based model without considering variability in wind and solar. In the second case study, the dynamic model of wind and solar has been considered after incorporating the deterministic drifts and stochastic fluctuations. The paper presents Harris hawks optimized model predictive controller (MPC-HHO) to curtail the frequency and voltage fluctuations caused due to the sudden change in loading pattern. Additionally, the authors have incorporated various dynamic energy storage devices like virtual inertia and redox flow battery (VI-RFB) in coordination with the MPC-HHO controller. From the obtained results, it has been validated that the transient response from the proposed MPC-HHO controller coordinated with VI-RFB has superior characteristics when compared with state-of-the-art methodologies available in the existing literature. Further, the most prevalent nonlinearities present in the realistic power system have been considered and their effects on the controller’s performance have been investigated and the robustness of the proposed MPC-HHO controller coordinated with VI-RFB has been successfully validated.

















Similar content being viewed by others
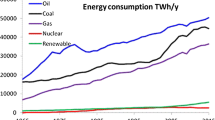
Abbreviations
- LFC:
-
Load frequency control
- AVR:
-
Automatic voltage regulator
- MPC:
-
Model predictive controller
- HHO:
-
Harris hawks optimization
- WOA:
-
Whale optimization algorithm
- GWO:
-
Grey wolf optimization
- PSO:
-
Particle swarm optimization
- AGC:
-
Automatic generation control
- SLP:
-
Step load perturbation
- EV:
-
Electric vehicle
- RFB:
-
Redox flow battery
- VI:
-
Virtual inertia
- MO:
-
Measured output
- MV:
-
Manipulated variable
- PO:
-
Peak overshoot
- PU:
-
Peak undershoot
- ST:
-
Settling time
- ACE:
-
Area control error
- STPP:
-
Solar thermal power plant
- RES:
-
Renewable energy sources
- \(\Delta f_{i}\) :
-
Incremental change in frequency in area i
- \(V_{i}\) :
-
Terminal voltage in area i
- \(f\) :
-
Nominal frequency of the system
- P(g):
-
Power generation output from RES
- \(T_{{{\text{pi}}}}\) :
-
Equivalent time constant of load model
- \(\eta\) :
-
Normalization constant 1 for RES modelling
- \(\partial\) :
-
Normalization constant 2 for RES modelling
- \(\beta\) :
-
Mean value of the generated power from RES
- \(\Gamma\) :
-
Time dependent switching signal
- \(B_{i}\) :
-
Frequency-bias coefficient of area i
- \(R_{i}\) :
-
Governor droop characteristic of area i
- G(s):
-
Low-pass transfer function
- \(K_{{{\text{VIi}}}}\) :
-
Gain of virtual inertia block
- \(T_{{{\text{VIi}}}}\) :
-
Time constant of virtual inertia block
- y1:
-
Coefficient involved with output variation in MPC objective function
- u1:
-
Coefficient involved with input variation in MPC objective function
- du1:
-
Coefficient involved with rate of change of input in MPC objective function
- \(K_{{{\text{RFBi}}}}\) :
-
Gain coefficient of RFB in area i
- \(T_{{{\text{RFBi}}}}\) :
-
Time constant of RFB in area i
- \({\text{ACE}}_{i}\) :
-
Area control error in area i
- \(\Delta T_{{{\text{pij}}}}\) :
-
Tie-line power error between area i and area j
References
Arya Y (2019) Impact of hydrogen aqua electrolyzer-fuel cell units on automatic generation control of power systems with a new optimal fuzzy TIDF-II controller. Renewable Energy 139:468–482
Arya Y (2020) Effect of electric vehicles on load frequency control in interconnected thermal and hydrothermal power systems utilising CF-FOIDF controller. IET Gener Transm Distrib 14(14):2666–2675
Bhookya J, Jatoth RK (2019) Optimal FOPID/PID controller parameters tuning for the AVR system based on sine–cosine-algorithm. Evol Intel 12:725–733
Bhuyan M, Barik AK, Das DC (2020) GOA optimised frequency control of solar-thermal/sea-wave/biodiesel generator based interconnected hybrid microgrids with DC link. Int J Sustain Energy 39(7):615–633
Dahiya P, Sharma V, Naresh R (2015) Solution approach to automatic generation control problem using hybridized gravitational algorithm optimized PID and FOPID controllers. Adv Electr Comput Eng 15:23–34
Dash P, Sakia LC, Sinha N (2015) Automatic generation control of multi area thermal system using bat algorithm optimized PD–PID cascade controller. Int J Electr Power Energy Syst 68:364–372
Elsisi M, Aboelela M, Soliman M, Mansour W (2018) Design of optimal model predictive controller for LFC of nonlinear multi-area power system with energy storage devices. Electr Power Compon Syst 46(11–12):1300–1311. https://doi.org/10.1080/15325008.2018.1469056
Heidari A, Mirjalili S, Faris H, Aljarah I, Mafarja M, Chen H (2019) Harris hawks optimization: algorithm and applications. Fut Gener Comput Syst 97:601–610
Kerdphol T, Rahman FS, Mitani Y, Hongesombut K, Küfeoğlu S (2017) Virtual inertia control-based model predictive control for microgrid frequency stabilization considering high renewable energy integration. Sustainability 9(5):1–21
Khooban MH, Niknama T, Blaabjerg F, Dragicevic T (2017) A new load frequency control strategy for micro-grids with considering electrical vehicles. Electr Power Syst Res 143:585–598
Kumar V, Sharma V (2021) Electric vehicle integrated MPC scheme for concurrent control of voltage and frequency in power system network. Wind Eng. https://doi.org/10.1177/0309524X211036424
Kumar V, Sharma V, Naresh R (2021) HHO-based model predictive controller for combined voltage and frequency control problem including SMES. IETE J Res. https://doi.org/10.1080/03772063.2021.1908180
Kundur P (1994) Power system stability and control. Tata McGraw-Hill, New York
Lal DK, Barisal AK (2019) Combined load frequency and terminal voltage control of power systems using moth flame optimization algorithm. J Electr Syst Inf Technol 6(8):1–24. https://doi.org/10.1186/s43067-019-0010-3
Lalor G, Mullane A, O’Malley M (2005) Frequency control and wind turbine technologies. IEEE Trans Power Syst 20:1905–1913
Liu H, Hu Z, Song Y, Lin J (2013) Decentralized vehicle-to-grid control for primary frequency regulation considering charging demands. IEEE Trans Power Syst 28(3):3480–3489
Mohamed TH, Morel J, Bevrani H et al (2012) Model predictive based load frequency control-design concerning wind turbines. Int J Electr Power Energy Syst 43(1):859–867
Mohanty B, Panda S, Hota PK (2014) Controller parameters tuning of differential evolution algorithm and its application to load frequency control of multi-source power system. Int J Electr Power Energy Syst 54:77–85
Naga Sai Kalyan CH, Sambasiva Rao G (2020) Combined frequency and voltage stabilisation of multi-area multisource system by DE-AEFA optimised PID controller with coordinated performance of IPFC and RFBs. Int J Ambient Energy. https://doi.org/10.1080/01430750.2020.1860130
Oshnoei S, Oshnoei A, Mosallanejad A, Haghjoo F (2021) Novel load frequency control scheme for an interconnected two-area power system including wind turbine generation and redox flow battery. Int J Electr Power Energy Syst. https://doi.org/10.1016/j.ijepes.2021.107033
Pan I, Das S (2016) Fractional order fuzzy control of hybrid power system with renewable generation using chaotic PSO. ISA Trans 62:19–29
Rajbongshi R, Chandra Saikia L (2019) Performance of coordinated interline power flow controller and power system stabilizer in combined multiarea restructured ALFC and AVR system. Int Trans Electr Energy Syst. https://doi.org/10.1002/2050-7038.2822
Rajbongshi R, Saikia LC (2017) Combined control of voltage and frequency of multi area multi source system incorporating solar thermal power plant using lightning search algorithm optimized classical controllers. IET Gener Trans Distrib 11:2489–2498
Rajbongshi R, Saikia LC (2018) Performance of coordinated FACTS and energy storage devices in combined multi- area ALFC and AVR system. J Renew Sustain Energy 9(6):1–22
RakhshaniE, Rouzbehi K, Sadeh S (2009) A new combined model for simulation of mutual effects between LFC and AVR loops. In: Asia-Pacific power and energy engineering conference, Wuhan, pp. 1–5
Ramoji SK, Saikia LC (2021) Optimal coordinated frequency and voltage control of CCGT-thermal plants with TIDF controller. IETE J Res. https://doi.org/10.1080/03772063.2021.1959420
Saadat H (2004) Power system analysis. Tata McGraw-Hill, New York
Sahani AK, Raj U, Shankar R, Mandal RK (2019) Firefly optimization based control strategies for combined load frequency control and automatic voltage regulation for two-area interconnected power system. Int J Electr Eng Inform 11(4):1–12
Sahu RK, Panda S, Rout UK (2013) DE optimized parallel 2-DOF PID controller for load frequency control of power system with governor dead band nonlinearity. Int J Electr Power Energy Syst 49:19–33
Sharma Y, Saikia LC (2015) Automatic generation control of a multi-area ST: thermal power system using grey wolf optimizer algorithm based classical controllers. Int J Electr Power Energy Syst 73:853–862
Sikander A, Thakur P, Bansal RC, Rajasekar S (2018) A novel technique to design cuckoo search based FOPID controller for AVR in power systems. Comput Electr Eng 70:261–274
Singh A, Sharma V (2019) Salp swarm algorithm-based model predictive controller for frequency regulation of solar integrated power system. Neural Comput Appl 31:8859–8870
Vijaya Chandrakala KRM, Balamurugan S (2016) Simulated annealing based optimal frequency and terminal voltage control of multi-source multi area system. Int J Electr Power Energy Syst 78:823–829
Zafer B, Karahan O (2018) A novel performance criterion approach to optimum design of PID controller using cuckoo search algorithm for AVR system. J Frankl Inst 355(13):5534–5559
Acknowledgements
The presented study has been supported by H. P. Council for Science, Technology & Environment (HIMCOSTE), SCST & E (R&D)/2019-20, beneath project Grant No. STC/F(8)-6/2019(R&D 2019-20)-408, H. P., India, awarded to second author.
Author information
Authors and Affiliations
Corresponding author
Ethics declarations
Conflict of interest
All the authors declare that there is no conflict of interest.
Ethical approval
This article does not contain any studies with human participants or animals performed by any of the authors.
Appendix
Appendix
i = 1, 2, and 3. T (Simulation Period) = 100 s. \(f\) = 60 Hz. SLP = 0.01 pu MW. AVR Model: \(K_{{{\text{Ai}}}}\) = 10; \(T_{{{\text{Ai}}}}\) = 0.1 s; \(K_{{{\text{Ei}}}}\) = 1; \(T_{{{\text{Ei}}}}\) = 0.4 s; \(K_{{{\text{Fi}}}}\) = 0.8; \(T_{{{\text{Fi}}}}\) = 1.4 s; \(K_{1}\) = 1; \(K_{2}\) = − 0.1; \(K_{3}\) = 0.5; \(K_{4}\) = 1.4; \(P_{s}\) = 0.145 \(K_{{{\text{Si}}}}\) = 1; \(T_{{{\text{Si}}}}\) = 0.05 s. LFC model: Geothermal System: \(T_{{{\text{gGeo}}}}\) = 0.1 s; \(T_{{{\text{tGeo}}}}\) = 0.1 s. STPP system: \(K_{s}\) = 1.0; \(T_{s}\) = 1 s; \(K_{{{\text{gs}}}}\) = 1.0; \(T_{{{\text{ts}}}}\) = 3.0 s. Wind System: \(T_{w1}\) = 0.6 s; \(T_{w2}\) = 0.041 s; \(K_{w1}\) = 1.25; \(K_{w2}\) = 1.4; \(G_{B}\) = 1. Thermal system: \(T_{{{\text{gi}}}}\) = 0.08 s; \(T_{{{\text{ti}}}}\) = 0.3 s; \(T_{{{\text{ri}}}}\) = 10 s; \(K_{{{\text{ri}}}}\) = 0.5 s. Diesel system: \(K_{{{\text{dii}}}}\) = 16.5. \(T_{{{\text{jk}}}} =\) 0.08 pu. \(R\) = 2.4 Hz/pu MW. B = 0.425 pu MW/Hz. \(H_{i}\) = 5 s. \(K_{{{\text{pi}}}}\) = 1/\(D_{i}\) = 120 Hz/pu MW. \(T_{{{\text{pi}}}}\) = \(2H_{i} /f_{i} D_{i}\) (= 20 s). \(f\) = Nominal frequency of the system = 60 Hz. RFB Model; \(T_{{{\text{RFBi}}}}\) = 0; \(K_{{{\text{RFBi}}}}\) = 1.8. Virtual Inertia: \(K_{{{\text{VI}}}}\) = 0.08; \(T_{VI}\) = 10. Stochastic Modelling of RES: Wind Model; \(\eta\) = 0.8; \(\beta\) = 10; \(\partial\) = 1. Solar Model: \(\eta\) = 0.7; \(\beta\) = 2; \(\partial\) = 0.1.
Rights and permissions
Springer Nature or its licensor (e.g. a society or other partner) holds exclusive rights to this article under a publishing agreement with the author(s) or other rightsholder(s); author self-archiving of the accepted manuscript version of this article is solely governed by the terms of such publishing agreement and applicable law.
About this article
Cite this article
Kumar, V., Sharma, V. & Naresh, R. Model Predictive Controller-Based Voltage and Frequency Regulation in Renewable Energy Integrated Power System Coordinated with Virtual Inertia and Redox Flow Battery. Iran J Sci Technol Trans Electr Eng 47, 159–176 (2023). https://doi.org/10.1007/s40998-022-00561-x
Received:
Accepted:
Published:
Issue Date:
DOI: https://doi.org/10.1007/s40998-022-00561-x