Abstract
The 2030 Agenda has among its key objectives the poverty eradication through increasing the level of education. A good level of education and investment in culture of a country is in fact necessary to guarantee a sustainable economy, in which coexists satisfactory levels of quality of life and an equitable distribution of income. There is a lack of studies in particular on the relations between some significant dimensions, such as education, culture and poverty, considering time lags for the measurement of impacts. Therefore, this study aims to fill this gap by focusing on the relationship between education, culture and poverty based on a panel of data from 34 European countries, over a 5-year period, 2015–2019. For this purpose, after applying principal component analysis to avoid multicollinearity problems, the authors applied three different approaches: pooled-ordinary least squares model, fixed effect model and random effect model. Fixed-effects estimator was selected as the optimal and most appropriate model. The results highlight that increasing education and culture levels in these countries reduce poverty. This opens space to new research paths and policy strategies that can start from this connection to implement concrete actions aimed at widening and improving educational and cultural offer.
Similar content being viewed by others
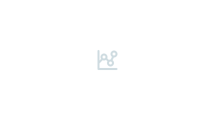
Avoid common mistakes on your manuscript.
1 Introduction
Poverty eradication has been the key objective for spans in many countries since that has been recognized as the greatest hostile issues ‘jeopardising balanced society socio-economic development’ (Balvociute, 2020). Poverty can be considered one of the core features of unsustainable socio-economic development and as a persistent phenomenon that can have upsetting effect on peoples’ lives (Bossert et al., 2022). For this reason, the extreme poverty removal, as well as the fight against inequalities and injustices, have been placed at the center, with climate change, of the 2030 Sustainable Development Goals. The nature of poverty is multidimensional and inequalities within and among countries is an obstinate origin for concern (Fund, 2015; Alvaredo et al., 2017; Alkire & Seth, 2015; Kwadzo, 2015). For its interpretation and measurement, the literature has added to the monetary approach of material deprivation, the social and subjective dimension of the human being (Bellani & D’Ambrosio, 2014; Maggino, 2015). As stated by Kwadzo (2015), it is possible to define three poverty measurements: monetary poverty, social exclusion, and capability poverty. Similarly, there are a lot of indicators measuring well-being and quality of life: Index of Happiness, Human Poverty Index and Human Development Index (Senasu et al., 2019; Spada et al., 2020; UNDP, 1990; Veenhoven, 2012; Watkins, 2007). All these indicators focus and start from education. For example, the Human Poverty Index (HPI) was introduced by the United Nations to complement the Human Development Index (HDI) and used, for the first time, in the 1997 Human Development Report. In 2010, it was replaced by the Multidimensional Poverty Index. The HPI focuses on the deprivation of three essential parameters of human life, already taken into account by the Human Development Index: life expectancy, education and standard of living (Alkire et al., 2015; UNDP, 1990).
Previous studies shown that education indicators have a large impact on a country’s poverty (Bakhtiari & Meisami, 2010; UNDP, 1990; Watkins, 2007) and that investing in health and education is a way to reduce income inequality and poverty. In addition, studies highlight that increasing equality and the quality of education is essential to combat economic and gender inequality within society (Walker et al., 2019). However, few studies provide empirical evidence on how education impacts on income inequality (Liu et al., 2021; Santos, 2011; Walker et al., 2019) and most of these studies analyses the poverty phenomenon neglecting the combined effect of various variables. Different dimensions of poverty have also empirically demonstrated a high degree of correlation (Kwadzo, 2015). In addition, the literature review analysis highlighted a gap in quantitative studies, especially on the paths between some relevant dimensions, such as education, culture and poverty, considering time lags for the measurement of impacts. In light of this, the main objectives of this study are: (i) To identify over the five-year period considered (2015–2019), with what delay and with what magnitude and sign, the poverty is influenced by some indicators representative of the educational and cultural dimension; and (ii) Consequently, better calibrate education policies in European countries, in order to achieve a reduction in the poverty rate in the short term, in compliance with the objectives of the 2030 Agenda.
The rest of the paper is organized as follows. A literature review regarding the relation between poverty, education and inequalities is presented in Sect. 2. The Sect. 3 enlightens research gaps linked to the aims of this study and hypothesis to corroborate. Section 4 defines data and summarizes the methodological approach used to reach the work’s aims. Results are presented and discussed in Sect. 5. Finally, the last section sets out our main conclusions by highlighting limitations of the study and future directions.
2 Theoretical Framework
2.1 The Core Role of Education
Over the last decades it is possible to individuate in the EU-28 a quickly growing portion of the population having income below 60% of the median disposable income. In addition, there is a share of the population has been becoming more impoverished (Balvociute, 2020; EUROSTAT Statistic Explained, 2019). In same way, it is possible to speak about “poverty trap”, a mechanisms whereby countries are poor and persist poor: existing poverty appears a straight cause of poverty in the future (Knight et al., 2009; Kraay & McKenzie, 2014). Aspects such as accommodation, education, medical and material services are considered essential. In particular, an increasing number of empirical studies have supported the positive effects of education on the creation of wealth by individuals and on promoting economic effective and fair development (UNESCO & Global Education Monitoring Report, 2017; Walker et al., 2019; Xu, 2016; Zhang, 2020). A research note by European Commission (2015) shows that individuals with primary education remain the most vulnerable in all EU countries (with a risk of poverty ranging from 13%—Netherlands—to 56% Romania). Even the Millennium Development Goals (MDGs), the Poverty Reduction Strategy Papers (PRSP) endorsed by the World Bank and ‘Education for All’ program (UNESCO, 2007) emphases the significant role of education (Awan et al., 2011). A diverse balance can be possible and policy efforts to interrupt the poverty trap might have long-term effects. In this framework, the model proposed by Santos (2011) shows that a policy oriented towards aligning the quality of education would reduce initial inequalities. In light of this, Shi & Qamruzzaman, (2022) in a recent work, study, by means of numerous econometrical methods, the tie between investments in education, financial inclusion, and poverty decrease for the period 1995–2018 in 68 nations, underlining the role of education-backed poverty mitigation public policies that need to be more targeted. Several studies demonstrate that level of poverty and education are strictly related. For instance, Bossert et al. (2022) by focusing on Atkinson-Kolm-Sen index, that measures the percentage income gap of the poor that can be attributed to inequality among the poor (Sen, 1973, 1976), emphasized the close relation between poverty and inequality. Consistent with previous studies, Lenzi and Perruca (2022) demonstrate that tertiary educated people report higher ranks of life satisfaction. This link is even more marked in rural territories where education is recognised as an important tool for reducing poverty as it allows the acquisition of skills and productive knowledges which increase people’s productivity and their earnings (Tilak, 2002). A recent report of the United Nations (2021) underlines how the reduced access to educational and health services in rural areas becomes a barrier, determining the difficulty of people living in these areas to found employment in well-paid professions contributing to economic growth (Chmelewska and Zegar, 2018). However, as Liu and colleagues (2021) find, different levels of education have distinct effects on poverty in rural areas of China and that the latter is driven not only by factors within the region but also by the level of poverty in the surrounding regions. In addition, numerous empirical evidences reveal a link between educational level and income inequalities in several geopolitical contexts. Bakhtiari and Meisami (2010), in a work of over 10 years ago, makes use of a panel data set of 37 Islamic countries (eight time periods) to study income inequality along with a model of poverty, with the main variables as income level, health status, education and savings. Findings show that enhancing the health and education can reduce income inequality and poverty. Likewise, as Arafat and Khan (2022) underline the high level of education not only contributes to reducing the degree of poverty but improves the conditions of mental, social and emotional well-being compared to poorly educated families. After about 10 years, similar works by Wani and Dhami (2021) and Sabir and Aziz (2018) reach the same results investigating the SAARC (South Asian Association for Regional Cooperation) countries and 31 developing countries (by employing the System Generalized Method of Moments). In several cases, and especially in rural areas, poverty is linked to the lower level of household income compared to urban areas, resulting in differences in access to basic goods and services to meet personal needs (Chmelewska and Zegar, 2018). In this territories household income level is directly associated with food security, in fact, an increase in the level of income reduces food insecurity (Chegini et al., 2021). However, as evidenced by other authors (Kirkpatrick et al., 2020; Kusio & Fiore, 2022), access to education can help to overcome the migration of young people and geographical isolation and inaccessibility that characterize the poor areas (Kvedaraite et al., 2011). In turn, young, educated people affect entrepreneurial attitudes. Walker et al. (2019) in the recent report ‘The Power of Education to Fight Inequality. How increasing educational equality and quality is crucial to fighting economic and gender inequality’ show how education can be emancipating for individuals, and it can play the role of a ‘leveler and equalizer within society’. Education interrupts obstinate and rising inequality by promoting the development of more decent work, rising incomes for the poorest people: it can aid to endorse long-lasting, wide-ranging economic growth and social cohesion.
Gradstein and Justman (2002) underlined the role of education in shaping the social cohesion that can assure equality between individuals. Universal free education enhances people’s earning power, and can bring them out of poverty. Low levels of education hamper economic growth, which in turn slows down poverty reduction (UNESCO, 2017; Global Education Monitoring Report, 2019) estimates that each year of schooling raises earnings by around 10%;53 this figure is even higher for women. In Tanzania, having a secondary education reduces the chances of being poor as a working adult by almost 60%. According to a study by UNESCO and the Global Education Monitoring Report (2019), if all adults finished secondary school, 420 million individuals would be lifted out of poverty. The convergence of crises deriving first from COVID-19 then from climate change, and conflicts, are generating extra impacts above all on poverty, nutrition, health and education affecting all the Sustainable Development Goals (SDGs).
Equilience, a synchratic neologism composed of Equity + Resilience, that is resilient systems in respect of equity as a balancing of the different interests of the parties. Recent research (Berbés-Blázquez et al., 2021; Williams et al., 2020; Contò and Fiore, 2020) highlight the crucial importance to promote the ‘marriage’ between equity and resilience.
2.2 Aims of Study and Hypothesis
This research is potentially the first study to investigate the relationship between educational, cultural factors and poverty in European countries.
The main research directions are as follows: (i) To assess the impact of education and culture (expressed by the following indicators: Cultural employment, Total educational expenditure, Graduates in tertiary education, Number of enterprises in the cultural sectors, Tertiary educational attainment) upon poverty (indicated by Persons at risk of poverty or social); (ii) To compare the strength and direction of the relationships between the variables considered in two temporal situations, i.e. with zero lag, and with lag equal to one year. The data cover the period 2015–2019 and were extracted from the Eurostat database.
In the light of the above discussion, of the literature review analysis, and of the theoretical frameworks examined this study explores the following research hypotheses with regard to the European context:
H1
Education and culture have an inverse impact on the levels of poverty.
Our second hypothesis states:
H2
The association between cultural, educational variables and poverty, in the short term is more intense if we consider a delay of one-year.
2.3 Data
The dataset is a balanced panel of annual observations for 34 European countries and covers the period from 2015 to 2019. On the basis of literature findings, our analysis focused on the following dimensions: education, income inequality and poverty.
Thereby, the variables considered for our investigation are as follows:
-
Poverty indicator: Persons at risk of poverty or social exclusion (% of population, thousand persons; hereinafter labelled with PRP);
-
Education and cultural indicators: Cultural employment (thousand persons); Total educational expenditure (million euros); Graduates in tertiary education (‰ of population;); Number of enterprises in the cultural sectors(number) Tertiary educational attainment (‰ of population). Respectively, hereinafter they will be labelled with CE, TEE, GTE, NEC and TEA.
The indicators have been extracted from the Eurostat database. The summary statistics are reported in Table 1. In the selected time period, Iceland is the country that shows the lowest values with respect PRP (12.08%). Instead, the country showing the worst performance is Romania (PRP = 41.60%). With regard to the education indicators, Germany holds the highest values for both CE (81,661.48 thousand persons) and TEE (30.588.86 million euros), highlighting great attention to education issues. Instead, in Eastern Europe (Montenegro, Romania, and Hungary) the indicators pertaining to the education area take on more penalized values. Italy is the country that boasts the largest number of enterprises in the cultural sector (NEC = 179,136.8), thanks also to the artistic beauties of which this country is rich. As far as the tertiary education level is concerned, the highest value of is held by Cyprus while the lowest by Romania (respectively TEA = 57.34 and TEA = 25.26). For subsequent processing, since the variables considered are both in the form of ratios and counts, all data were converted to natural logarithms.
3 Methodology
The methodological approach used is based on linear panel data models including the simple Pooled Ordinary Least Square (pooled OLS) model, the Fixed Effects (FE) model and the Random Effects (RE) model. Before proceeding with the application of the linear models, the correlation matrix between the variables taken into consideration was performed and subsequently, to avoid multicollinearity problems and distorted estimates, the study, based on the principal component analysis (PCA), used two indicators related to education and culture. According to Jolliffe and Cadima (2016), through PCA starting from a set of correlated variables, a set of uncorrelated variables is obtained, known as Principal Components (PC). In PCA, only common factors that have an eigenvalue greater than one or greater than the mean should be kept (Jolliffe, 2002; Kaiser, 1974). In this study PCA allowed to obtain the following indicators: EDU1, which includes CE, NEC, TEE, and EDU2, composed of TEA and GTE. These indicators have been incorporated into the panel data models, replacing the original variables.
The first linear panel data model adopted is the pooled OLS, which assumes no heterogeneity between countries, whose equation is as follows:
where lnPRP is the natural logarithm of the poverty indicator, α is the intercept, EDU is composed of the principal components extracted, ε is the error term, i denotes statistical units, in this case countries, and t denotes the time index.
The second model adopted is FE which controls for cross-country heterogeneity and is expressed as:
where \(\alpha_{i}\) is the regional specific parameter denoting the fixed effect. The basic intuition of the FE model is that \(\alpha_{i}\) does not change over time.
Finally, the third model is RE denoted as;
In the RE model, variations between units are assumed to be random and uncorrelated with the independent variables in the model.
To verify the two research hypotheses, for each of the three models (pooled OLS, FE and RE) two versions were calculated, with lag 0 and lag 1 year. In the model at lag 0 the variables are synchronous, while in the model at lag 1 principal components enter the equation with a one-year lag compared to PRP. The choice of the reference model between pooled OLS, FE and RE is based on several tests. In choosing between FE and pooled OLS, the study applies the F-test. A p-value of less than 5% indicates that there are important country effects that OLS fails to detect, and that thus neglecting unobserved heterogeneity in the model can lead to estimation errors and inconsistencies. The study also tests which is better between the OLS and RE model using the Breusch-Pagan (BP)-Langragian Multiplier (LM) test. The null hypothesis of the BP-LM test is that there is no substantial variance between regions. A probability value of less than 5% for the BP-LM test indicates that the RE model is appropriate and the OLS pool is not. Finally, the Hausman test χ2 is also performed to compare the FE model and the RE model. According to Algieri and Mannarino (2013), the Hausman test χ2 aims to identify a violation of the RE modelling hypothesis. In this test, the alternative hypothesis is that the FE model is preferable to the RE model, while the null hypothesis is that both models produce similar coefficients. A p-value greater than 5% denotes that both FE and RE are reliable, but the RE model is more efficient because it uses a lower degree of freedom. We also test for heteroskedasticity in the FE model using the modified Wald test developed by Lasker and King (1997). The null hypothesis of this test is that the variance of the error is similar for all countries (Amaz et al., 2012). All statistical analyses were conducted in Stata 17.0 (Stata Corp LP, College Station, Texas, USA). A critical value of p < 0.05 was specified a priori as the threshold of statistical significance for all analyses.
4 Results
The relationships between the variables, measured by Pearson’s linear correlation coefficient, is shown in Table 2. It is noted that the PRP variable is negatively correlated with all the other panel variables, albeit with modest correlations. Instead, TEE shows a high positive correlation with NEC (r = 0.963 and r = 0.903, respectively). There is also a high correlation between NEC and TEE (r = 0.857). Therefore, in the light of the results, to exclude the problem of multicollinearity between the covariates, we proceeded to analyse the principal components.
Table 3 shows the results of principal component analysis. On the basis of these results, the need to maintain the first two principal components is highlighted, since their eigenvalues are greater or very close to 1 and cumulatively represent the 84% of the information. They will be labelled as EDU1 and EDU2 respectively. EDU1 refers to TEE, CE and NEC, i.e. it refers to a cultural dimension of the country and therefore, even if not strictly connected to the school environment, with an important educational role, while the EDU2 component referring to GTE and TEA, is more closely related to the school.
Table 4 shows the results of the three econometric models (pooled OLS, FE, RE) on the link between education, culture and poverty. It is observed that all models converge in showing that poverty decreases with increasing education and culture. In particular, the EDU1 indicator always shows a negative coefficient, and this relationship is statistically significant in the model fixed at lag 0 and lag 1 (respectively b = − 0.3804, p < 0.001; b = − 0.3925, p < 0.001). Furthermore, for EDU1, in all three econometric models it can be noted that the coefficients are higher in absolute value passing from lag 0 to lag 1, highlighting that the impact between cultural and educational tools and poverty reduction occurs with a delay, perhaps necessary to have positive results. Also, the EDU2 indicator always shows a negative coefficient and this relationship is statistically significant in all three models, both at lag 0 and at lag 1 (for all p < 0.001). To discern the econometric model that best fits the data, as a first step the F-test allows you to choose between the OLS and FE models. The value F = 80.09 for lag 0 and F = 109.61for lag = 1, (for all p-value < 0.001), indicates in both cases that the FE model is more suitable than the pooled OLS. This demonstrates that in the relationships examined time plays an important role, which a simple OLS model may fail to capture, i.e. EDU1 and EDU2 have an effect on poverty decrease that changes over time. The choice between the RE model and the pooled OLS was instead based on the BP LM test, which suggests that the RE model is more suitable than the pooled OLS. Finally, the Hausman test χ2 allows to identify which between FE and RE is more suitable: The value χ2 = 15.95 at lag 0 and χ2 = 13.40 at lag = 1, (for all p-value < 0.001) suggests that the FE model is more suitable than the RE model, indicating the presence of non-random differences between countries or over time. The model that best fits the examined panel of data is therefore the FE model.
In light of these results, as supposed in hypothesis H1, it is evident that education and culture play a significant role in poverty reduction. Furthermore, as supposed by hypothesis H2 and based on the FE model which was found to be the most suitable, this impact is more intense if one considers a year of delay, above all for cultural and educational variables relating to a dimension that is not strictly scholastic.
5 Discussions and Conclusions
The present study analysed the relationship between education, culture and poverty for 34 countries, over the period 2015–2019. The findings indicate that rising education and culture levels in these nations reduce poverty. The model also highlighted that this relationship is weaker if we consider a contemporaneity of the values of the variables (at lag 0), while it is strengthened if we consider a time interval of one year.
As policy-makers regularly disclose the consequences of unfair development by identifying problems requiring solutions built on evidence-based guidelines, these results can have interesting and fruitful implications. By concluding, education appears, in line with other studies (Sabir & Aziz, 2018; Xu, 2016), one of the best effective methods to eradicate poverty. In line with the work by Walker et al., (2019), investing in universal-free-public education for all the persons can close different circles: the gap between rich and poor people, between women and men, between poor and rich areas within a country and among countries. In addition, education appears crucial to fight inequalities across the world. The results appear also consistent with the UN report (2021) that emphasizes the importance of the access to educational and health services in marginal poor areas to improve and contribute equal economic growth and reduce poverty (Chmelewska and Zegar, 2018; Bakhtiari & Meisami, 2010; Wani & Dhami, 2021). The same findings come from the work by Peng (2019) based on data from poor Chinese provinces showing that education has steady and positive impacts on farmers’ income, and the outcome of growing income in poor zones is higher than in other areas.
All in all, as evidenced by the European Commission (2015), the means to diminish the risk of poverty appears ‘straight-forward: go to school, get a job’. Clearly, these implications have to consider conditions and country environment. In line with previous research (Noper Ardi & Isnayanti, 2020; Walker et al., 2019), these results highlight that education can have an immediate impact on income inequalities and poverty; on the other hand, education (and public spending on it) has a longer-term impact on inequality through its effects in enhancing future salaries and chances. Indeed, as stated by some notable researchers (Kraay & McKenzie, 2014), the ‘more-likely poverty traps’ need action in less-traditional policy areas. The scholars have to further perfect the theoretical concepts and policy standards of poverty alleviation through education (Shi & Qamruzzaman, 2022).
This paper reinforces the conclusions deriving from other research (Mou and Xu, 2020; Assari et al., 2018; Batool and Batool, 2018) that are to give evidence of how education can forecast coming ‘Emotional Well-Being’ thus decreasing the inequalities by means of more generous policies and strategies. The latter can support international experience-based education (Xu, 2016).
In the following research phases, other variables can be inserted to improve the specifications of the model and also verify the existence of homogeneous groups of countries. In addition, a distinction between urban and rural areas to highlight the link between income, education and poverty and differences could enrich the literature and provide useful information to guide national policies in a targeted way. Regarding possible limitations of the paper, it is possible to notice a time period limited for missing data and health variables are missing.
The ‘dark’ side of this conclusions is considering the effects of the COVID19 pandemic that has increased on one hand the online teaching and training: on the other hand, education has become more difficult in remote, rural and/or marginal areas due to connections and hardware limitations.
Therefore, nowadays strategies, models and polices focusing on equi-lience (equity and resilience) processes can promote the creation of a different balance between the needs of sustainable growth and those of social, fair and environmental development (Fiore, 2022). Therefore, developing a strategy to convey a trained, skilled and well-supported workforce, investing in relevant and fair teaching resources, ensuring funds and building better liability mechanisms from national to local levels can be significant and fair paths to reduce poverty and inequalities. These strategies have to be aimed at developing national education plans that try to identify pre-education existing inequalities in order to arrange actions in poorer rural and marginalized districts or regions.
References
Alkire, S., Foster, J. E., Seth, S., Santos, M. E., Roche, J. M., & Ballon, P. (2015). Multidimensional poverty measurement and analysis. Oxford: Oxford University Press.
Alkire, S., & Seth, S. (2015). Multidimensional poverty reduction in india between 1999 and 2006: Where and how? World Development, 72(C), 93–108.
Algieri, B., & Mannarino, L. (2013). The role of credit conditions and local financial development on export performances: A focus on the Italian Regions, Universita della Calabria, Dipartimento di Economia e Statistica. Retrieved from http://www.ecostat.unical.it/WP2/WP/10_A%20panel%20analysis%20for%20the%20sectoral%20manufacturing%20exports.pdf
Alvaredo, F., Chancel, L. Piketty, T., Saez E. and G. Zucman. (2017). The world inequality Report 2018. World Inequality Lab. http://wir2018.wid.world/
Amaz, C., Gaume, V., & Lefevre, M. (2012). The impact of training on firm scrap rate: A study of panel data. Retrieved from http://www-perso.gate.cnrs.fr/polome/Pages2012_13/Panel/LEFEVRE_GAUME_AMAZ_rapport.pdf
Arafat, M., & Khan, M. (2022). Effect of education on poverty and wellbeing of rural households in District Hangu, Khyber Pakhtunkhwa. Journal of Managerial Sciences, 16(1), 63–92.
Ardi N. (2020) Structural Equation Modelling-Partial Least Square to Determine the Correlation of Factors Affecting Poverty in Indonesian Provinces In: IOP Conference Series: Materials Science and Engineering. 846: 012054 doi: https://doi.org/10.1088/1757-899X/846/1/012054.
Assari, S., Preiser, B., & Kelly, M. (2018). Education and income predict future emotional well-being of whites but not blacks: A Ten-Year cohort. Brain Sciences, 8, 122. https://doi.org/10.3390/brainsci8070122
Awan, M. S., Malik, N., Sarwar, H., & Waqas, M. (2011). Impact of education on poverty reduction.
Bakhtiari, S., & Meisami, H. (2010). An empirical investigation of the effects of health and education on income distribution and poverty in Islamic countries. International Journal of Social Economics.
Balvociute, R. (2020). Social assistance for poverty reduction in the EU Countries: An aspect of socio-economic sustainability. European Journal of Sustainable Development, 9(1), 469–469.
Batool, S. S., & Batool, S. S. (2018). Impact of education on women’s empowerment: mediational role of income and self-esteem. Journal of Research & Reflections in Education (JRRE), 12, 1.
Bellani, L., & D’Ambrosio, C. (2014). Deprivation and Social Exclusion in Europe. In A. C. Michalos (Ed.), Encyclopedia of Quality of Life and Well-Being Research. Dordrecht: Springer. https://doi.org/10.1007/978-94-007-0753-5_3711
Berbés-Blázquez, M., et al. (2021). Assessing future resilience, equity, and sustainability in scenario planning. In Z. A. Hamstead, D. M. Iwaniec, T. McPhearson, M. Berbes-Blazquez, E. M. Cook, & T. A. Munoz-Erickson (Eds.), Resilient urban futures. The urban book series. Cham: Springer. https://doi.org/10.1007/978-3-030-63131-4_8
Bossert, W., Cato, S., & Kamaga, K. (2022). Generalized Poverty-gap Orderings. Social Indicators Research, 164(1), 189–215.
Chegini, K. R., Pakravan-Charvadeh, M. R., Rahimian, M., & Gholamrezaie, S. (2021). Is there a linkage between household welfare and income inequality, and food security to achieve sustainable development goals? Journal of Cleaner Production, 326, 129390.
Chmielewska, B., & Zegar, J. S. (2018). Changes in rural poverty after Poland’s accession to the European Union. Journal of Agribusiness and Rural Development, (4 [50]).
Contò F., Fiore M. (Eds.) (2020), Reasoning on Local Development: a ‘new’ reading of ‘ancient’ themes [Ragionando di Sviluppo Locale: una lettura ‘nuova’ di tematiche ‘antiche’, Franco Angeli, Milano, pp. 1–688, ISBN 978–88–351–0554–1, OPEN ACCESS at the following link: (https://www.francoangeli.it/Ricerca/Scheda_Libro.aspx?CodiceLibro=10365.39).
European Commission, (2015). Employment, education and other means of reducing poverty Research note no. 4/2015 Directorate-General for Employment, Social Affairs and Inclusion.
EUROSTAT Statistic Explained (2019). People at risk of poverty or social exclusion. Retrieved from https://ec.europa.eu/eurostat/statistics-explained/index.php/People_at_risk_of_poverty_or_social_exclusion
Friderichs, T., & Correa, F. M. (2022). Measuring human capital in South Africa across socioeconomic subgroups using a latent-variable approach. Social Indicators Research. https://doi.org/10.1007/s11205-022-02973-y
FUND, SDG (2015). Sustainable development goals. Available at this link: https://www.un.org/sustainabledevelopment/inequality/
Global Education Monitoring Report. (2019). Building bridges for gender equality: gender report 2019. UNESCO. https://en.unesco.org/gem-report/2019genderreport
Gradstein, M., & Justman, M. (2002). Education, social cohesion and economic growth. American Economic Review, 92(4), 1192–1204.
Jolliffe, I. (2002). Principal component analysis (2nd ed.). Springer.
Jolliffe, I. T., & Cadima, J. (2016). Principal component analysis: A review and recent developments. Philos Trans A Math Phys Eng Sci., 374(2065), 20150202. https://doi.org/10.1098/rsta.2015.0202. PMID:26953178;PMCID:PMC4792409.
Kaiser, P. (1974). An index of factorial simplicity. Psychometrika, 51(4), 804–821. https://doi.org/10.1007/BF02291575
Kirkpatrick, I. C. M., Horvat, T., & Bobek, V. (2020). Improving competitiveness between EU rural regions through access to tertiary ed-ucation and sources of innovation. International Journal of Diplomacy and Economy, 6, 26–40.
Knight, J., Shi, L., & Quheng, D. (2009). Education and the poverty trap in rural China: Setting the trap. Oxford Development Studies, 37(4), 311–332.
Kraay, A., & McKenzie, D. (2014). Do poverty traps exist? Assessing the evidence. Journal of Economic Perspectives, 28(3), 127–148. https://doi.org/10.1257/jep.28.3.127
Kusio, T., & Fiore, M. (2022). Which stakeholders’ sector matters in rural development? That is the problem. Energies, 15(2), 454. https://doi.org/10.3390/en15020454
Kvedaraite, N., Zvireliene, R., Glinskiene, R., Repechine, A., & Baksys, D. (2011). Research of social-economic causes of migration of higher school students and implications to the region. In W. Wereda & S. Starnawska (Eds.), Competitive and Cooperative Business Strategies for Efficient Outcomes in Different Markets-Internal Organizational Drivers (pp. 23–45). Siedlce University of Natural Sciences and Humanities.
Kwadzo, M. (2015). Choosing concepts and measurements of poverty: A comparison of three major poverty approaches. Journal of Poverty. https://doi.org/10.1080/10875549.2015.1015067
Lasker, M., & King, M. (1997). Modified wald test for regression disturbances. Economic Letters, 56(1), 5–11. https://doi.org/10.1016/S0165-1765(97)00128-6
Lenzi, C., & Perucca, G. (2022). No place for poor men: On the asymmetric effect of urbanization on life satisfaction. Social Indicators Research, 164(1), 165–187.
Liu, W., Li, J., & Zhao, R. (2021). The effects of rural education on poverty in China: a spatial econometric perspective. Journal of the Asia Pacific Economy, 8(1), 176–198.
Maggino, F. (2015). Assessing the subjective wellbeing of nations. In W. Glatzer, L. Camfield, V. Møller, & M. Rojas (Eds.), Global handbook of quality of life Exploration of well-being of nations and continents (pp. 803–822). Springer.
Mou, X., & Xu, F. (2020). Examining the factors influencing information poverty in western China. The Electronic Library, 38(5/6), 1115–1134. https://doi.org/10.1108/EL-04-2020-0095
Peng, N. (2019). How effective is the preference alleviation by supporting education? An empirical study based on the national provincial panel data. Educational Re-Search of Tsinghua University, 40(4), 90–97.
Sabir, S., & Aziz, N. (2018). Impact of health and education on income inequality: Evidence from selected developing countries. Business and Economic Review, 10(4), 83–102.
Santos, M. E. (2011). Human capital and the quality of education in a poverty trap model. Oxford Development Studies, 39(01), 25–47.
Sen, A. (1973). On economic inequality. Oxford University Press.
Sen, A. (1976). Poverty: An ordinal approach to measurement. Econometrica, 44, 219–231.
Senasu, K., Sakworawich, A., & Russ-Eft, D. F. (2019). Developing Thai happiness index. Social Indicators Research, 146(3), 425–448. https://doi.org/10.1007/s11205-019-02136-6
Shi, Z., & Qamruzzaman, M. (2022b). Re-visiting the role of education on poverty through the channel of financial inclusion: Evidence from lower-income and lower-middle-income countries. Frontiers in Environmental Science. https://doi.org/10.3389/fenvs.2022.873652
Spada, A., Rana, R. L., & Fiore, M. (2020). Investigating the evocative link among wine consumption, Human Development Index and geographical region. Studies in Agricultural Economics, 122, 66–76.
Tilak, J. B. G. (2002). Education and poverty. Journal of Human Development, 3(2), 191–207. https://doi.org/10.1080/14649880220147301
UN (2021). World social report 2021 Reconsidering Rural Development. Department of Economic and Social Affairs, United Nations.
UNDP. (1990). Human development Report 1990. Oxford University Press, USA.
UNESCO (2007) “Education For All Global Monitoring Report 2007”, www.unesco.org
UNESCO and Global Education Monitoring Report. (2017). Reducing global poverty through universal primary and secondary education, op. cit.
Veenhoven, R. (2012). Cross-national differences in happiness: Cultural measurement bias or effect of culture? International Journal of Wellbeing, 2(4), 333–353.
Walker, J., Pearce, C., Boe, K., & Lawson, M. (2019). The Power of Education to Fight Inequality: How increasing educational equality and quality is crucial to fighting economic and gender inequality. Oxfam.
Wani, N. U. H., & Dhami, J. K. (2021). An empirical investigation of the effects of health and education on income distribution and poverty in SAARC Countries. Management, 4(3), 1–15.
Watkins, K. (2007). Human development report 2007/8. Fighting climate change: Human solidarity in a divided world. Fighting Climate Change: Human Solidarity in a Divided World (November 27, 2007). UNDP-HDRO Human Development Report.
Williams, T. G., Guikema, S. D., Brown, D. G., & Agrawal, A. (2020). Resilience and equity: Quantifying the distributional effects of resilience-enhancing strategies in a smallholder agricultural system. Agricultural Systems. https://doi.org/10.1016/j.agsy.2020.102832
Xu, L. (2016) Education is the foundation of eradicating poverty. Study Times, pp 03–10.
Zhang, L. (2020). Overview of the poverty-alleviation by supporting education in China. Sci Insigt Edu Front, 6(2), 631–651.
Funding
Open access funding provided by Università di Foggia within the CRUI-CARE Agreement.
Author information
Authors and Affiliations
Corresponding author
Ethics declarations
Conflict of interest
Authors disclose financial or non-financial interests directly or indirectly related to the work submitted for publication.
Additional information
Publisher's Note
Springer Nature remains neutral with regard to jurisdictional claims in published maps and institutional affiliations.
Rights and permissions
Open Access This article is licensed under a Creative Commons Attribution 4.0 International License, which permits use, sharing, adaptation, distribution and reproduction in any medium or format, as long as you give appropriate credit to the original author(s) and the source, provide a link to the Creative Commons licence, and indicate if changes were made. The images or other third party material in this article are included in the article's Creative Commons licence, unless indicated otherwise in a credit line to the material. If material is not included in the article's Creative Commons licence and your intended use is not permitted by statutory regulation or exceeds the permitted use, you will need to obtain permission directly from the copyright holder. To view a copy of this licence, visit http://creativecommons.org/licenses/by/4.0/.
About this article
Cite this article
Spada, A., Fiore, M. & Galati, A. The Impact of Education and Culture on Poverty Reduction: Evidence from Panel Data of European Countries. Soc Indic Res (2023). https://doi.org/10.1007/s11205-023-03155-0
Accepted:
Published:
DOI: https://doi.org/10.1007/s11205-023-03155-0