Abstract
Multiple myeloma (MM) is a hematological malignancy characterized by clonal proliferation of plasma cells. Although therapeutic advances have been made to improve clinical outcomes and to prolong patients’ survival in the past two decades, MM remains largely incurable. Single-cell sequencing (SCS) is a powerful method to dissect the cellular and molecular landscape at single-cell resolution, instead of providing averaged results. The application of single-cell technologies promises to address outstanding questions in myeloma biology and has revolutionized our understanding of the inter- and intra-tumor heterogeneity, tumor microenvironment, and mechanisms of therapeutic resistance in MM. In this review, we summarize the recently developed SCS methodologies and latest MM research progress achieved by single-cell profiling, including information regarding the cancer and immune cell landscapes, tumor heterogeneities, underlying mechanisms and biomarkers associated with therapeutic response and resistance. We also discuss future directions of applying transformative SCS approaches with contribution to clinical translation.
Similar content being viewed by others
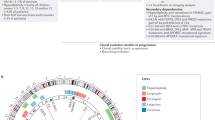
Introduction
MM is a hematological cancer characterized by uncontrolled proliferation of malignant plasma cells (PCs) in the bone marrow (BM) which remains largely incurable [1]. MM accounts for around 10% of hematological cancers with about 155,688 patients to be diagnosed worldwide per year [2]. The survival in MM patients has significantly improved over the past decade [3], owing to the introduction of effective novel regimens including proteasome inhibitors (PIs), monoclonal antibodies and immunomodulatory drugs (IMiDs). However, these patients ultimately develop disease relapse, thus further treatment is required [4]. Clonal evolution and diversity of MM cells and BM microenvironment (BME) changes are the major causes of the disease relapse and poor response rate, and these diverse alterations pose both challenges and opportunities for myeloma therapy [5, 6]. With the development of the high throughput next generation sequencing (NGS), we have gained greater insights into MM biology by exploring its intricate genomic landscape [7,8,9,10]. Integrated examination of bulk genomic, transcriptomic and exome sequencing has provided valuable information regarding disease drivers including translocations, copy number alterations, somatic mutations, and altered gene expression. Besides, at cellular level, active interactions between myeloma cells and their microenvironment, including bone marrow stromal cells (BMSCs) and immune cells have been extensively discussed [11, 12]. The molecular mechanisms underlying the progression of this malignancy are driven by signals coming from the BME and immune surveillance failure [13, 14]. An increasing number of evidences suggests that the impairments of immunological processes contribute to myeloma evasion from immune surveillance and resistance to effector cells mediated cytotoxicity, resulting in an immune suppressive BME of myeloma [15, 16].
Despite the impressive progress in understanding the molecular pathogenesis of MM, in developing new therapies and improving in transplant technology [17], many important questions have yet to be addressed, leading to several confronting issues and challenges in the MM field (Fig. 1). One of the key questions is that the identity of the MM cell origin remains controversial. Researchers revealed that cancer stem cell might be responsible for the development of MM, although there is ongoing debate regarding the identity of the MM stem cells (MMSCs) [18,19,20]. Several markers such as side population (SP) [21], ALDH1+ [22] and CD24+ [23] have been used to identify MMSCs. Verifying the origin of myeloma cells represents a significant effort to achieve effective cancer treatment. Clonal evolution is also a key topic in MM research field which drives tumor progression, chemoresistance and relapse in myeloma [9, 10, 24,25,26]. NGS studies have demonstrated different types of clonal changes over the course of disease, which are categorized into stable, linear, and branching evolution of myeloma clones [27, 28]. The major queries are how these subclones arise and how they are selected. As a consequence of current bulk sequencing methods, the answer is certainly equivocal and more powerful technologies are needed. BM myeloma cells are highly dependent on neighboring cell signals for survival which allows them to grow and proliferate. The BME consists of multiple cellular compartments, including mesenchymal stromal cells (MSCs), immune cells, endothelial cells, osteoblasts and osteoclasts, creating a distinct milieu that supports immune escape and promotes progression of myeloma cells. The mechanisms by which the BM niche contributes to MM pathogenesis remain largely unexplored. Despite landmark therapeutic advancements to treat MM, one of the major challenges remains that the majority of patients eventually relapse and become refractory to anti-myeloma drugs. Additionally, there are no reliable biomarkers available for the accurate prediction of responses to specific drug classes, limiting our ability to select a more personalized treatment strategy. Therefore, it is becoming increasingly obvious that there is a prominent need to develop and exploit more powerful and precise approaches to fully dissect the molecular and cellular landscape during disease pathogenesis, and to discover new biomarkers for predicting drug efficacy or resistance, thus providing opportunities for precision medicine of MM.
Unresolved questions in the field of myeloma biology research. Studying MM biology in both myeloma intrinsic and extrinsic contexts will advance understanding of how the complex cross-talk between myeloma cells and surrounding non-cancer cells results in the successful growth of malignant subclones, with impacts on clonal revolution and resistance to therapies. MM: multiple myeloma; MMSCs: multiple myeloma stem cells; TME: tumor microenvironment; BM: bone marrow
Traditional microarrays and NGS assays require bulk DNA or RNA from large number of cells, and they are limited to providing average information of a population of cells. Furthermore, rare cell populations or unique cellular states could be critical in tumor transformation and pathogenesis, such as cancer stem cells (CSCs) or immune cell subsets, which might not be detected in bulk analyses [29]. The advent of single-cell sequencing (SCS) has overcome these limitations through revealing the genomic/transcriptomic profile of each cell within given samples at high resolution and throughput [30,31,32]. SCS has been widely applied in the field of myeloma research, providing the analysis of cellular heterogeneity [33, 34], identifying new cell subtypes [35], distinct cellular states [36], and elucidating dynamic cellular transitions during tumour evolution and microenvironment remodeling [37,38,39]. These multi-faceted, high-dimensional dissections at genomic, epigenomic, transcriptomic, and proteomic levels in tumour cells and the related immune or stromal cells allow the in-depth characterization of cancer biology, the intricate interactions between cancer cells and surrounding compartments in BME, and details of the clonal evolution in each MM tumour. In this review, we summarize recent progress in the SCS workflow and techniques that have been used in MM research and further discuss the findings of clonal revolution, cancer cellular heterogeneity, stromal cells and immune microenvironment explored by SCS. We further discuss the growing applications of single-cell approaches for answering important research questions and their implications in clinical translation.
Emerging single-cell sequencing technologies
Breakthroughs in single-cell capture, sequencing technologies, and analytical bioinformatics have led to rapid progress in SCS analysis methodologies which have been reviewed extensively elsewhere [40,41,42,43]. The success of single-cell approaches benefits from concurrent improvements in methodological pipeline and analytics, such as the isolation of single cells, high-dimensional reduction, unsupervised clustering, evolutionary modelling, multiple datasets integration, lineage tracing and ligand-receptor interaction predicting [43,44,45]. Single-cell approaches have the unique potential to help answer many important questions that lie in cancer research, including: the roles of cancer and immune cells heterogeneities; the relations between tumor cell clonotype and phenotype; the network of ligand-receptor interactions present in the tumor microenvironment (TME); as well as the spatio-temporal crosstalk between cancer cells and immune populations. With the rapid advancements of SCS technologies, numerous methods have been developed, offering unprecedented opportunities not only to profile DNA, mRNA, chromatin and proteins through different single-cell omics, but also to measure multiple modalities one cell at a time by various multi-omics tools (Table 1). In this part, we will discuss the most important single-cell approaches and platforms that have been utilized in disease research (Fig. 2).
Single-cell approaches utilized in disease research. Single-cell approaches are developed to profile the genome, epigenome, transcriptome/spatial transcriptome and proteome, and single-cell multi-omics approaches are built by combining different SCS methods, which have been widely used in disease research. SCS: single-cell sequencing; scRNA-seq: single-cell RNA sequencing; scDNA-seq: single-cell DNA sequencing; CNV: copy number variation; scATAC-seq: single-cell assay for transposase-accessible chromatin by sequencing; scChIP-seq: single-cell chromatin immunoprecipitation followed by sequencing; NGS: next generation sequencing; CITE-seq: cellular indexing of transcriptomes and epitopes by sequencing
Single-cell transcriptomics
Single-cell RNA sequencing (scRNA-seq) is a procedure that enables non-targeted quantification of transcripts in individual cells [86]. This technique is largely independent of previous biology knowledge, and it allows for the detailed description of tissue subtypes and cell states. Multiple efficient high-throughput protocols for single-cell separation have been developed (for example, the Fluidigm C1 platform [87], droplet microfluidics [46] and microwells [88]). And various scRNA-seq approaches such as Drop-seq [47], Smart-seq2 [89], CEL-seq2 [52], MARS-seq [54], CytoSeq [50], sci-RNA-seq [53] have contributed to the discovery of novel and rare cell types, cellular heterogeneity within complex tissues, and biological mechanisms in healthy and disease conditions. A high-throughput sequencing method for mRNA transcriptomics at a single-cell level was first reported in 2009 [90]. Islam et al. developed single-cell tagged reverse transcription sequencing method in 2011, allowing for detecting mixed cell samples including highly heterogeneous tumor samples on a large scale [91]. In 2012, Smart-seq was introduced to facilitate the measurement of full-length transcripts [51]. By enhancing transcriptomic reading coverage, this technology enables accurate analysis of alternative splicing and detection of single nucleotide polymorphisms (SNP) and other genomic mutations. A modified Smart-seq2 was created later by Picelli et al., which improved accuracy, sensitivity and coverage in full-length [92].
In recent years, a number of droplet-based platforms for high-throughput scRNA sequencing have attracted attention, including 10X Chromium Genomics, inDrop and Drop-seq [46, 47, 93]. These technologies share similar strategies in generating droplets, isolating single cells through on-bead primers with barcodes, and correcting bias by applying unique molecular identifiers (UMIs) [94]. However, these technologies differ in cost, time, cell capture efficiency, and sensitivity due to different manufacturing methods for beads, barcoding, and cDNA amplification [94, 95]. In a recent study comparing 10X Genomics and Smart-seq2 sequencing data from CD45+ cell samples [96] showed that Smart-seq2 was able to capture more features within a single cell with high sensitivity, in particular capable to detect those cells with low-abundance transcripts and alternative spliced transcripts [96]. Although 10X Genomics method showed higher dropout rates and noise in lowly expressed genes, this tool can detect more genes owing to its preferable coverage of abundant cells, which therefore enable the detection of rare cell populations. In another report, Smart-Seq2 and 10X Genomics were combined to help elucidate the landscape of immune cells and revealed the dynamic status features in hepatocellular carcinoma [97].
Single-cell spatial transcriptome
Single-cell spatially resolved transcriptome technologies have been developed and improved rapidly in recent years, with a unique capability of capturing cellular spatial distribution and revealing local networks of intercellular communication acting in situ, which cannot be achieved by scRNA-seq. Although there are numerous spatial transcriptomic (ST) methods, current ST protocols can be generally divided into two major groups according to their detection strategies. Most ST techniques use either a spatial indexing or imaging-based approaches to measure and quantify mRNA molecules in situ. In spatial indexing approaches such as 10X Visium and Slide-seq, barcodes are locally hybridized to RNA molecules, then gene expression profiles are quantified by NGS. This approach relies on polyA hybridization, which pose a challenge in fresh-frozen and formalin-fixed paraffin-embedded (FFPE) tissues as mRNA integrity varies in these samples. Further solution on this issue is desirable as clinical samples are often stored in FFPE blocks. Furthermore, achieving single-cell resolution remains a technical and computational challenge. Imaging-based approaches use fluorescent tagging of mRNA molecules in situ, and high-resolution fluorescence microscopy to detect and differentiate between single mRNA transcripts. These methods can achieve single-cell resolution but are still constrained by limitations on probe design, and low in situ mRNA abundance and degradation. Despite these challenges, ST technologies have added a new dimension to single-cell omics and extensively broadened our understanding of cancer biology.
The ST profiling and temporal lineage tracing enable multi-faceted investigations into the surrounding environment and molecular dynamics within a single cell [31], thus add another layer of tumor heterogeneity which might be critical for disease diagnosis, monitoring and treatment in cancer research [98]. Attaching spatial barcodes allows encoding and retrieving location information of single cells, providing important and useful information in research and disease diagnosis. For instance, by positioning histological sections on spatially barcoded microarrays, researchers were able to visualize and quantify the transcriptome with spatial resolution in tissues of mouse brain and human breast cancer [55]. By using this spatial-resolved method, the researchers found prominent tumor heterogeneity within a tumor biopsy section, reflecting different subclones with varying genes expression patterns located in the same area [55]. In addition, a recent study integrated scRNA-seq and microarray-based spatial transcriptomics data from pancreatic ductal adenocarcinomas (PDAC) samples, and revealed that cancer cells with high expression of the stress module colocalized with inflammatory fibroblasts, which may contribute to therapy resistance [99]. Tumor progression is a complex and dynamic process involving multiple steps evolving from initiation, progression to the emergence of therapeutics resistance [100]. Definition of the molecular and temporal nature during these processes is crucial to understand tumor biology and develop effective therapeutics strategies. Lineage tracing by nuclease-activated editing of ubiquitous sequences (LINNAEUS) is a newly developed tool for lineage tracing and has successfully applied to reconstruct lineage trees in zebrafish [101]. CRISPR-Cas9 coupled single-cell analysis was utilized in a KRAS mutant mouse model to decipher a comprehensive spectrum of cancer cells [101]. This approach improved the sensitivity of low mutation, and showed the ability to illustrate the detailed changing of tumor subtypes, and allow tracking the spread pattern of lung cancer cells [102]. These findings suggest that in patients with KRAS mutations, targeted therapy may be developed and clinical management could be improved through these approaches [102]. Another study combining high-confidence clonal tracing and scRNA-seq led to a detailed dissection of leukemic stem cells, providing novel insights into the understanding of leukemia oncogenesis and therapeutics [103].
Single-cell genomics
Single-cell genomics aims to extend our understanding of genetics by bringing the research of genomes to the cellular level. Thus, rare and unique mutations and copy-number variations (CNVs) can be detected faithfully to reveal the clonal heterogeneity and evolution, which may be involved in disease processes. Sequencing an entire genome of a cell requires whole-genome amplification (WGA) by three major methods including degenerate oligonucleotide primed PCR (DOP-PCR), multiple displacement amplification (MDA) and multiple annealing and looping based amplification cycles (MALBAC) [104]. DOP-PCR often yields low genome coverage, which is pertinent to the exponential amplification of PCR. MDA is considered to be the most suitable method for detecting single nucleotide variants (SNVs) and insertions/deletions at the genome-wide level, owing to its capacity to amplify the majority of a human genome with a high-fidelity polymerase. MALBAC achieves more accuracy for CNV detection and a low false negative rate for SNV detection [104, 105]. High-throughput single-cell DNA sequencing (scDNA-seq) using different sing-cell isolation strategies have been developed by several commercial platforms [106, 107], thus providing higher scalability, allowing cell selection with lower costs, and offering more flexibility for customized chemistry steps. At present, CNV profiling is one of the most common applications of scDNA-seq. Despite the high throughput (up to 10 K cells) achieved by microdroplets (10X Genomics) and combinatorial indexing for single-cell CNV profiling, these methods face challenges of lower data quality and limited genomic resolution. By contrast, FACS, nanowells and microfluidic platforms utilizing tagmentation chemistry [108, 109] are able to provide much high-quality CNV data at single-cell resolution, but provide modest throughput (hundreds to 1,000 cells). Mutation detection presents another major application of scDNA-seq, which requires in-depth coverage of a certain mutation site. While early studies carried out whole-genome or exome sequencing of single cells [110, 111], high cost prevented these studies from profiling large number of cells. In order to increase throughput and reduce costs, later approaches aimed to profile targeted regions of the genome, such as specific cancer gene panels [112, 113]. A microdroplets approach have been developed and commercialized for scaling up scDNA-seq [107], which conducts PCR amplification of single cells at hundreds of targeted genomic regions. ScDNAseq approaches provide a reliable resolution in examining clonal substructure and reconstructing clonal lineages during cancer evolution in the context of premalignant status, metastasis and drug resistance. Technologies for scDNA-seq are particularly helpful to resolve mutual exclusivity and mutation co-occurrence in different clonal subpopulations, which are difficult to identified by bulk sequencing data [113].
Single-cell epigenomics
Research into epigenetic regulation at the single-cell level has helped to define epigenetic landscape by profiling DNA modifications, chromatin accessibility and histone modifications. A variety of epigenomic sequencing approaches at single-cell level have been developed, such as single-cell reduced-representation bisulfite sequencing (scRRBS) to measure DNA methylation [114], single-cell chromatin immunoprecipitation followed by sequencing (scChIP-seq) to measure histone modifications [72] and protein-DNA interactions [115], and single-cell assay for transposase accessible chromatin sequencing (scATAC-seq) to measure chromatin accessibility [69, 70, 116]. A major advantage of scATAC-seq compared with scRNA-seq is that it offers greater insights into gene regulation and transcription along with cell lineage and identity information [117]. Transcript-indexed ATAC-seq (T-ATAC-seq) approach was built by combining scATAC-seq with sequencing of the T cell receptor (TCR) repertoire, which allows for studying both the epigenomic state and the TCR specificity simultaneously at the single-cell level [118]. Apart from mapping chromatin accessibility, investigating diverse chromatin modifications may provide further insights into epigenomic states. Recently, a new method named single-cell cleavage under targets and tagmentation (CUT&Tag) technology was established to profile multiple histone modifications and DNA-protein interactions [73]. This novel technology generates high-quality data with ultra-low cell inputs compared to traditional ChIP-seq, and helps to study the comprehensive histone modifications and dynamic regulatory interactions with high throughput and sensitivity within each single cell [73].
For better understanding of genomic organization, in situ genome sequencing (IGS) has been used by researchers for simultaneous in situ imaging and sequencing of the genomes within the same single cell [119]. This process includes the construction of in situ genomics DNA libraries, in situ sequencing of amplicons and spatially localized sequences, amplicon dissociation, PCR and ex situ sequencing of amplicons, revealing the accurate localization of distinct DNA sequences. It is clear that IGS presents a valuable mean of addressing biological questions involving the relationships between genomic architecture and disease [119]. Furthermore, integrated high-resolution multiple annealing and looping-based amplify cycles was applied by another study to analyze transcriptomic dynamics and define the three-dimensional genomic architecture at single-cell level [120]. Using this method, researchers can specifically unravel the roles of transcriptomic and genomic architecture during oncogenesis, as well as the interplay among anatomy, function, transcription, and cell types along with cancer progression [120].
Single-cell proteomics
Proteins are essential macromolecules that are responsible for the main functional machinery within a cell, including regulations of gene expression, signaling pathway and catalytic reaction. Proteome measurements based on mass spectrometry (MS) have historically been limited to bulk samples containing thousands or millions of cells. The rise of single-cell proteomics leads to an in-depth and unbiased profile of protein expression within single cells. This emerging technology is mainly based on two methods: MS-based method, in which the proteomic content of the cell is digested and analyzed; and antibody-based method, which typically target a certain number of predefined proteins [75]. The use of MS is the basis for detecting and quantifying proteomes, but it is only useful for identifying the most abundant proteins. By improving protein preparation and isolation procedures, researchers have been able to decrease protein loss and perform quantitative proteomics sequences at a single-cell resolution. By combining the principles of MS with flow cytometry, mass cytometry by time of flight (CyTOF) uses metal isotope-labeled antibodies conjugated with specific molecules on the cell surface or inside cells, allowing for the examination of 100 specific proteins in single cells [121]. Based on immunohistochemistry with metal-labeled antibodies and CyTOF, imaging mass cytometry (IMC) has been developed [122]. Through IMC, up to 40 protein markers can be analyzed simultaneously, along with their spatial architecture and interactions, which would be lost with traditional lysis of tissue to single cells [123]. Furthermore, IMC can be performed on paraffin-embedded tissue sections, making it useful for retrospective analysis of patient cohorts with known outcomes, ultimately benefitting individualized medicine. Liquid chromatography-mass spectrometry (LC-MS) is another technology for single-cell proteomics analysis [124], which is a bioanalytical method for analyzing proteins quantitatively and is widely used in drug development and drug toxicology research. Furthermore, a multiplexed single-cell proteomics (SCoPE-MS) method has been developed to increase throughput by multiplexing, and enhance peptide sequence identification using an isobaric carrier [125]. As expected, the application of single-cell proteomic sequencing will revolutionize the pathological investigation, particularly when combined with multi-omics platforms, such as transcriptomic and genomic profiling at the single-cell resolution.
Single-cell multi-omics
Multi-omics single-cell methods interrogate multiple levels of molecular information from a single cell, such as DNA & RNA, RNA & protein, or three layers combined [126]. By combining these methods, it is feasible to gain deeper insight into genotype-phenotype relationships and how genomics affects gene expression and protein level. For instance, the transcriptome and targeted genomic regions can be simultaneously profiled within a single cell, which allows to provide better coverage of the targeted genomic loci for detecting SNVs, deletion mutations as well as CNVs, which are related to drug resistance in lung cancer [127].Quantification of the transcriptome and DNA-protein interactome simultaneously in a single cell will lead to a better understanding of the transcriptional changes during the process of DNA binding to a specific protein, and such quantification has been applied by scDam&T-seq [128]. Furthermore, a new single-cell method named scM&T-Seq was developed for parallel sequencing of DNA methylome and transcriptome in one cell, enabling in-depth analyses of how epigenetic heterogeneity relates to gene expression output at each locus [79]. Moreover, the transcriptome and proteome can also be delineated simultaneously using several technologies, such as CITE-seq [83], REAP-seq [82] and RAID [84]. In a recent study, an integrative pipeline using 3D imaging and the rapid clearing agent FUnGI were used in breast cancer and demonstrated that tumor clones were significantly reduced during oncogenesis, with the luminal progenitor serving as the key cells of origin [129]. In conjunction with multicolor lineage tracing and molecular analysis, LSR-3D imaging offers crucial visual and spatial information within the tumor to describe biological processes, highlighting the nature of tumors with inherent plasticity. Future developments of these multi-omic SCS methods hold promise for overcoming technical hurdles, thereby allowing their widespread adoption in translational research in the near future.
Applications of single‐cell sequencing in MM biology and pathogenesis
A growing number of studies have begun to investigate the genomic, epigenetic, and transcriptional landscape in MM at the single-cell level, as summarized in Table 2. Herein we summarized the recent SCS studies in MM research and discussed the insights they have provided, which can be roughly categorized into three main aspects: (1) clonal evolution and heterogeneity, (2) reprogramming of the BM microenvironment, (3) response or resistant to anti-myeloma therapy (Fig. 3).
Applications of SCS in myeloma research. SCS has impacted various areas of cancer research and improved our understanding of clonal evolution, tumor heterogeneity, tumor microenvironment, therapeutic resistance in MM, which offers a profound opportunity to improve the diagnosis and identify biomarkers for precision medicine of MM. SCS: single-cell sequencing; MM: multiple myeloma; MGUS: monoclonal gammopathy of undetermined significance; SMM: smoldering multiple myeloma; RRMM: relapsed refractory multiple myeloma
Understanding the heterogeneity of MM
MM is a clonal disease with strong inter- and intra-tumoral heterogeneity, and these heterogeneities are reflected genetically, epigenetically, and phenotypically [5, 7, 143]. Intra-tumoural heterogeneity occurs when different tumour cells within a patient display different genetic or phenotypic characteristics. On the other hand, inter-tumoural heterogeneity indicates genomic and biological variations across different MM individuals. In the search for potential target therapies, gene expression profiling is a widely used method to characterize tumor phenotype in individual patients, however, most cancers display intra-tumoral heterogeneity, which may have impacts on drug response to specific therapeutics and clinical outcomes. With SCS technologies, tumor heterogeneity in myeloma can be assessed at a single-cell resolution, enabling researchers to unravel the complex biological nature of MM.
In the initial studies of scRNA-seq, samples were taken from patients at multiple time points in order to trace the dynamic tumoral evolution. In an early single-cell whole-exome sequencing (WES) study of six patients with t(11;14) MM, it was found that there were two to six major subclones present at the time of the diagnosis of MM and the existence of clones with both linear and branching evolution patterns [144]. As a result of subsequent single-cell genomic efforts, SCS have been applied to pursued molecular profiling of MM and its premalignant stages, with higher resolution than conventional NGS method. Recently, Liu et al. analyzed 14 MM patients throughout the disease progression by multi-omic combining bulk DNA and scRNA sequencing, and identified three patterns of clonal evolution, including stability (from premalignancy to diagnosis), and gain or loss (from diagnosis to relapse) [37]. Notably, by mapping PCs together with B cell lineage, they found “B cell-like” plasma cell subpopulations that cluster closely with B cells. This study provides an important guide to delineate plasma/myeloma cells origin, and motivates us go deeper into investigating myeloma cells development and its implications in MM malignancy.
By examining scRNA-seq data from patients with MGUS, SMM, MM, and light chain amyloidosis, the authors found that myeloma heterogeneity persisted throughout these different disease stages [33]. In addition, a recent scRNA-seq study of plasma cells from 26 patients at different disease stages revealed comprehensive intratumor heterogeneity by capturing shared transcriptional programs across patients, and demonstrated that scRNA-seq is sensitive enough to detect latent cytogenetic alterations in patients at asymptomatic stages with low tumor burden [145]. These findings emphasize the feasibility of applying scRNA-seq to detect changes in BM myeloma cells earlier than methods implemented currently in the clinic. Apart from human myeloma studies, a model of disease progression in Vκ*MYC mice was subjected to longitudinal single-cell depiction, and revealed that tumors at early stage contained subclonal CNVs which persisted throughout the disease progression [146]. More importantly, researchers identified a tumoral program involving GCN2 stress response that progressively activated during myeloma progression, and indicated the potential role of GCN2 as a promising therapeutic target [146]. These studies together highlight the application of single-cell technologies to profile the genetic and transcriptomic heterogeneity of tumor cells, and emphasize the necessity for their combination in studies aimed to explore disease progression of MM.
Multiple relapses and drug resistance are hallmarks of MM [147]. In recent studies, scRNA-seq was performed to extensively dissect MM resistance in refractory/relapsed patients. Through single-cell transcriptomic and chromatin accessibility profiling, Frede et al. demonstrated coexisting transcriptional programs in single tumor cells of relapsed refractory MM (RRMM) [36]. They detected six distinct expression programs across RRMM patients including cell cycle, different cell signaling pathways, including KRAS-MAPK, IL-6-STAT3 and IL-2-STAT5 pathways, as well as the interferon response. More importantly, pseudotime trajectories showed that myeloma cells exhibited a higher developmental potential than normal plasma cells, suggesting their lineage infidelity and plasticity. They further demonstrated that standard treatment can reduce differential potential while increase regulon activity, and CXCR4 may represent an attractive therapeutic target, since clinical trials have already tested CXCR4 antagonist for relapsed myeloma [148] and use for other clinical applications [149]. In another study published recently, scRNA-seq and variable-diversity-joining regions-targeted sequencing (scVDJ-seq) were performed to showed the transcriptomic and clonal diversity in newly diagnosed MM (NDMM) and RRMM patients [34]. Similarly, they observed universal intra-tumoral heterogeneity after identifying 8 meta-programs covered diverse biological functions, including the cell cycle that have been identified previously [36]. Comparing to NDMM, RRMM-specific myeloma cell clusters showed upregulations of the expression signatures of several immature progenitors (e.g. MMP and CLP) and representative target genes including STMN1, TUBB, TYMS, TUBA1B and HMGN2, which may contribute to relapse risk and dismal overall survival (OS).
In MM, clonal PCs accumulate in the BM, resulting in bone destruction and focal lesions [143]. The number of focal lesions served as a prognostic factor due to its correlation with the disease progression [150]. BM samples are collected mainly from the posterior iliac crest or from the sternum. Limited by this invasive procedure, repeated samplings are restricted. Nonetheless, Rasche et al. elegantly revealed genomic heterogeneity spatially, which was found in most of the patients at both chromosomal and mutational levels [150]. For instance, del(17p) exhibited spatial variation in two out of six MM patients. Progression events are frequently restricted to focal events, however initiating events are uniformly spread. High-risk disease distribution can be heterogeneous, and taking a "snapshot" at diagnosis at only one locus misses important information [151, 152]. Despite limited evidence of spatial heterogeneity from whole-exome sequencing, a recent report used scRNA-seq might provide potential mechanisms by which myeloma cells induce osteolytic lesions (OL) in one region, whereas other regions show no indications of bone destruction despite subtotal infiltration. Beyond the characterization of inter- and intra-tumor heterogeneity, the study identified OL-specific differentially expressed genes like DKK1, HGF and TIMP-1 compared to PCs from BM, and contributed to the understanding of mechanisms underlying myeloma bone disease [135].
Collectively, by integrated analysis of single-cell sequencing, these studies elucidated multiple layers of complexity regarding myeloma heterogeneity, including inter- and intra-patient heterogeneity across disease progression from precursor stages to active MM and relapse status, as well as additional spatial assessment in bone lesions. These data have proved fruitfully in resolving intricate molecular dynamics of MM pathogenesis and disease relapse by SCS technologies.
Uncovering the mechanisms of drug resistance
The main goals of anti-myeloma therapy are to achieve greater depth of response and improve outcome of MM patients. Drug-induced changes possess the potential to reveal important insights into the molecular mechanisms in action of chemotherapeutics. A better understanding of the specific molecular signatures changed during the course of treatment is key to understand the detailed mechanisms behind drug response or resistance. With the capacity to investigate tumour-intrinsic transcriptome profiles, and tumour heterogeneity, scRNA-seq analysis combined with dynamic sampling and detailed genetic profiling could largely support the identification of drug-response genes and the understanding of drug-induced changes in the clonal dynamics. A recent scRNA-seq analysis of BM cells derived from 20 RRMM patients revealed that MM consists of diverse genetic clones with marked transcriptional changes and clonal dynamics before and after treatment [38]. Gain/amplification of chromosome 1q (1q +) region has been shown to be associated with poor outcomes of patients with MM [153, 154]. Using scRNA-seq tool, Tirier et al. detected rare subclones harboring 1q + that comprised ~ 2% of the cells, and these cells cannot be separated into a certain transcriptional cluster, demonstrating the sensitivity of SCS in detecting rare subclones in MM [38]. In clone dynamics during treatment in RRMM, they found that 1q + clones often showed expansion or remained stable and therefore displayed a compelling robustness anti-myeloma drug in RRMM. Interestingly, by screening the upregulated genes located in 1q, they found 18 genes were exclusively detected by scRNA-seq, which were not found in bulk RNA-seq, and these genes were associated with various biological processes, such as apoptosis, proteasome, and signaling from BME, which may explain mechanisms of drug resistance in different treatment strategies. Additionally, the 1q + specific tumor program can be used to faithfully detected 1q + subclones with low abundant before treatment, which could be exploited in other scRNA-seq data sets of MM.
Longitudinal monitoring during treatment and remission allowed the researchers to evaluate disease status, drug response and provide real-time notification of clonal emergence or reduction for clinicians. ScRNA-seq analysis can be incorporated with longitudinal sampling and intensive genetic and transcriptional profiling, which would bring a deeper understanding of treatment-induced alterations in the clonal dynamics and identification of drug-response genes. Masuda et al. performed scRNA-seq on MM cells from a patient with relapsed MM by temporal sampling during multiple anti-MM drugs [139]. Although only one sample was assessed, they identified a distinct cell cluster which arose after lenalidomide treatment and eliminated after PI treatment. Further investigation identified a drug-responsive gene, PELI2, which may confer PI sensitivity, and in vitro experiments and computational analysis further confirmed that PELI2 presents a predictive biomarker of the PI responsiveness in MM patients. This study highlights the advantages of SCS in detecting myeloma cell population with low abundance during therapy and examining drug-induced cellular changes. These findings warrant further research on more MM patients by utilizing SCS approaches. In another recent study assessing drug response in a larger sample size, scRNA-seq data were collected from optimal responders (ORs) who achieved the best response in CR or VGPR and suboptimal responders (SORs) with best response of PR, MR, SD, or PD. By comparing ORs with SORs, the authors identified a 24-gene signature that upregulated in SORs, and further validated its association with bortezomib responsiveness and poor prognosis [140]. Additionally, our recent study that performed scRNA-seq on MM samples during anti-myeloma treatment, revealed dynamic changes in both tumoral and microenvironmental programs, and highlighted the SCS derived-benefits for comprehensively understanding overall and detailed features in myeloma BM ecosystems during therapy [155].
Based on the capability of predicting drug response, scRNA-seq can be used in clinical trials to predict resistance and guide therapy development for resensitizing tumors to treatment [131]. In this study, the authors applied massively parallel RNA SCS framework (MARS-Seq) on BM samples from a cohort of 41 individuals with primary refractory MM (PRMM), who experienced primary resistance or early relapse after a first-line bortezomib-based agent [131]. These patients were enrolled in the KYDAR trial and treated with a combination agent: daratumumab, carfilzomib, lenalidomide, and dexamethasone (DARA-KRD). Through exploring drug resistant mechanism, the authors found 66 differential expressed genes that could be clustered into 3 modules with capacity to distinguish PRMM from NDMM, and integrating both ≥ double hit and module 1-high in multivariate analyses was found to increase the prediction power of examining patients’ outcomes. Moreover, by comparing responders and non-responders during DARA-KRD treatment, they generated a resistance signature, which contained substantial overlap genes with module 1 and suggested that this newly discovered resistant signatures may be relevant throughout the course of several lines of therapy. Clinical utility of these signatures might facilitate to predict the risk of drug resistance in a group of MM patients. This approach could be implemented using routine methods such as qPCR and also by machine learning-based method. Another implication of this study is that by combining longitudinal scRNA-seq and clonal analysis using B-cell receptor sequencing (BCR-seq), they were able to score a single clone in every tumor and detected small resistant clones that appeared during treatment. Thus, this study presents a framework for developing a drug-resistance atlas that could revolutionize clinical practice in the future.
Dissecting bone marrow microenvironment
The interplay between myeloma cells and the BME is crucial for tumor development, treatment and disease progression [11, 156,157,158]. Several cell types in BME including stromal cells [159, 160], osteoblasts [156, 161], osteoclasts [161], immune cells [13, 162, 163] (e.g., B cells, T cells, natural killer cells, monocytes, myeloid-derived suppressor cells) and endothelial cells [156, 164] present in the BM and generate a unique milieu that favors MM cells immune evasion, bone disease and promotes drug resistance. Reciprocal interactions between the various compartments in BME and the MM cells are essential to regulate differentiation, migration, growth and survival of the malignant plasma cells. In-depth understanding of the tumour ecosystem and cell-cell interactions could faithfully provide insights for developing novel and more effective therapeutics for MM patients. One limitation of traditional genomic studies is that they are focused on the myeloma cells only and thus ignore the complex interactions with microenvironment. Single-cell technology is a powerful approach to investigate the sophisticated TME and can be exploited for precision medicine and overcoming treatment resistance. Here, we briefly introduce several key studies performing SCS approaches to explore TME in MM.
T cells
T cells play central roles in anti-tumor immunity. In recent years, a variety of reports have revealed quantitative and functional T cell abnormalities in MM patients [165,166,167,168]. Early study on T cell subsets revealed three major abnormalities of T cells in myeloma, including (1) the emergence of cytotoxic T cell harboring the senescence-associated secretory phenotype (SASP) [166, 167]; (2) increase in the ratio between the regulatory T (Treg) cell and T helper 17 (Th17) [169, 170]. (3) the acquired Treg leading to immunosuppression [169, 171]. Single-cell profiling has allowed high-resolution mapping and comprehensive analysis of T cell heterogeneity and function. In an early study of MM that utilized scRNA-seq on patient-derived BME samples (CD138− or CD45+ cell populations) from patients with MGUS, low-risk or high-risk SMM, and active MM, the authors revealed early alterations in immune cell composition at the precursor stages, with substantial T cells enrichment in particular [39]. This study demonstrated a shift from the manifestation of less-mature cytotoxic memory T cells with high GZMK expressing in non-malignant and MGUS patients, to an enrichment in more-mature cytotoxic memory T cells with GZMB expression at the SMM and MM stages. Later in vivo experiments using C57BL/KaLwRij mice with 5TGM1 cells injection revealed that this transition may reflect a mechanism that drives immune evasion during disease progression of MM. In regard to dissect T cell atlas and function in precursor stages, another SCS study of T cells from MGUS, SMM to active MM was conducted [172]. To increase the sample size, the authors combined their in-house data with samples from a published SCS data set [39] to perform a meta-analysis. They found that the anti-tumor immune response showed a decline from MGUS, SMM to MM, evident by a general decrease in naïve and memory CD4+ T cells and an increase of Treg together with CD8+ Eff 2 cells with an inhibitory phenotype. These two single-cell studies jointly demonstrated that early alterations of T cell populations have arisen in asymptomatic conditions of MGUS and SMM stages [39, 172]. Identifying the early immune events that promote MM progression by single-cell technologies will help to stratify patients based on their risk of progression, and offer therapeutic opportunities for early intervention.
Except for these quantitative changes, MM patients also exhibited relevant defects of T cell functions. Early studies showed that under the influence of TGF-β, released by regulatory immune cells and myeloma cells, T cells presented a remarkable reduction in IL-2-mediated autocrine proliferation [167], and diverse signaling impairment, including the downregulation of CD28, CD152, p56lck, ZAP-70, and PI3K, which were found in both CD4+ and CD8+ T cells in MM patients with advanced-stage [173]. A combined analysis of scRNA-seq and mass cytometric assay on immune cells in the MGUS and MM showed that during disease progression, T cell clusters with stem-like and tissue-resident signature, were depleted during MGUS-to-MM progression [174]. In particular, more substantial T cell exhaustion and senescence at the tumor site (BM) were observed than peripheral blood (PB) by expressing multiple molecules related to exhaustion (PD-1, CD160, 2B4, CTLA-4) and senescence (CD57, lack of CD28) [166]. Additionally, these T cells displayed reduced proliferative capability, defective cytotoxicity, and disability in IFN-γ production after antigen stimulation. In another report with in-depth immune checkpoints phenotyping of BM T cells in MM using mass cytometry-based single-cell analysis, Wang et al. confirmed higher expression of these conventional immune checkpoints in T cells and ligands in myeloma cells from MM patients compared with healthy donors (HDs), as well as several newly identified immune checkpoints including LAG3, Tim-3, and TIGIT [175]. Notably, a recent scRNA-seq study refined the molecular features of T cell by comparing MM patients with different bortezomib treatment responses [140], and the authors found that dysfunctional T cells expressing exhausting genes (PD-1, TIGIT, TIM3, CD244, LAG3, CTLA4 and TOX) were enriched in SORs compared to the ORs patients. Moreover, an upregulation of LAG3, KLRG1, CD47 and IFNG which have been previously linked to dysfunctional T cells in RRMM patients, pointing to the key roles of T cell dysfunction in drug resistance. In the immune cell populations that play roles in the tumor microenvironment, innate-like T cells expressing T cell receptors composed of γ and δ chains (γδ T cells) are of particular interest, and previous reports have demonstrated their impaired immune function during MM disease progression [176]. Interestingly, Tirier et al. showed that among all T cell populations, the highest expression of the exhaustion signature was detected in γδ T cells of RRMM patients by upregulating expressions of multiple inhibitory receptors, including LAG3, KLRG1, VSIR and TIGIT [38]. And in some patients, this exhaustion signature showed increased after treatment. By exerting antigen-driven cytotoxicity against target cells in an MHC-independent fashion, γδ T cells can recognize a broad variety of antigens to exert their potent and broad anti-tumour activity [177]. The findings of exhaustion of γδ T in RRMM and other infiltrated tumor tissues [178,179,180] suggest that these cells have a similar ability to respond to immune checkpoint inhibitors (ICIs) as traditional effector T cells. Thus, we can expect that reinvigorating γδ T cells by targeting inhibitory molecules might be beneficial for developing novel effective immunotherapies.
Recent studies have illustrated that defective metabolic flexibility related to cancer cells can lead to an inefficacy in anti-tumor immune response and linked to cancer progression [181,182,183]. The impaired energetic metabolism also involved with the myeloma oncogenesis and clinical outcomes of MM patients [184]. In a recent study, Lv et al. performed scRNA-seq on immune cells from NDMM and HD, and found that CD8+ T effector cells and natural killing (NK) cells with high tumor infiltration exhibited distinct metabolic features with comparison to those with low tumor infiltration and HD [185]. The abnormal metabolism was reflected by impaired metabolism amino acid including arginine, proline, glycine, serine metabolism and enhanced glycolysis/gluconeogenesis, oxidative phosphorylation and lipid metabolism in CD8-XCL2 memory T cells and CD8-GNLY effector T cells. The finding of defective metabolism was dependent on pathway analysis, further functional investigation would be needed to confirm the observations and dissect the detailed underlying mechanisms. The authors also revealed an upregulation of PIM kinases in effector CD8 T cells, supporting that targeting PIM kinases served as a feasible strategy to restore the immune cells function through metabolism regulation.
Chimeric antigen receptor (CAR) T-cell therapies have achieved impressive efficacy in MM but also bring its own set of challenges including durability of CAR-T cells, toxicities and therapeutic resistance [186,187,188]. A recent study performed single-cell genomic on temporal samples from a MM patient who relapsed after initial anti-BCMA CAR-T cell treatment [137]. The authors reported a clone selection with acquired deletion of BCMA, which resulted in lack of CAR-T cell proliferation following the second infusion, supporting the notion that antigen escape is one of the possible causes of CAR-T therapy failure [186, 189]. Interestingly, a recent study demonstrated that CAR level played a critical role in CAR-T cell activity with notable influence on clinical response [136]. To better understand the heterogeneity of CAR-T cells and the influence of CAR density on the transcriptional profile, the authors performed scRNA-seq and identified 23 clusters of CAR-T cell populations and observed that CARHigh T cells mainly localized within activated CD4+ cells. Furthermore, in the CD8+ T cell subset, CARHigh T cells showed a pre-exhausted feature. They then demonstrated that CAR density was associated with differential activation of regulatory networks, evident by activation of transcription factors NR4A1 and MAF, low active SATB1 regulon in CARHigh T cells, which could explain their exhausted phenotype. Therefore, single-cell omics enables the high-resolution molecular characterization of the activation and functional states of T cells, thus holding great potential for understanding CAR-T cell behavior. Given the very active clinical research on CAR-T cells in MM ongoing currently [188, 190, 191], we expect that in the near future, SCS could become a standard tool for clinical monitoring cell states and developing more effective CAR-T cell therapies.
NK cells
NK cells have been identified as the primary effector cells of innate immunity [192,193,194]. Numerous studies have demonstrated the significance roles of NK cells in killing cancer cells or in tumor progression regulation [193, 195, 196]. Impairments of NK cell maturation, chemotaxis, cytokine production, and expression of effector molecules have been described in the context of MM [197, 198]. Therefore, restoring or enhancing the cytotoxicity of NK cells for MM treatment has become one of the key topics in recent years. In the first scRNA-seq study analyzing the alterations in immune microenvironment along MM progression, the authors identified 3 major NK subpopulations including CXCR4+ NK, CX3CR1+ NK and a less frequent CD56bright NK population, and showed that NK cell proportion is frequently increased in the precursor stages indicating an early onset of an immune response [39]. Another recent study applied scRNA-seq to profile CD56+ NK cells from NDMM BM samples, have also acknowledged the CD56bright NK population [133]. They further revealed three novel NK cell populations at single-cell level: adaptive NK-cell cluster characterized by high expression of KLRC2 and low expression of FCER1G, the terminal NK cluster expressed ZEB2 and B3GAT1 genes, a NK-HSP cluster characterized by the high expression of genes for heat shock protein (HSP), which was considered as stressed NK cells due to sample freezing and thawing. The uncovered population of adaptive NK was also identified in a recent study utilized scRNA-seq to dissect TME of MM patients receiving bortezomib-based treatment [140]. Their further investigation demonstrated that unlike conventional NK cells, adaptive NK cells displayed distinct immunophenotypic features with low expression of inhibitory receptors such as killer cell immunoglobulin like receptor (KIR), TIGIT and NKG2A. More importantly, with daratumumab treatment, adaptive NK cells from NDMM patients exhibited enhanced cytokine production and increased degranulation compared with conventional NK cells, suggesting that adaptive NK cells serve as an important mediator of antibody-dependent cellular cytotoxicity (ADCC) in MM.
Through conventional measuring methods, such as flow cytometry and bulk RNA-seq, the exhausted phenotype of NK cells in MM has been described in previous studies, reflected by expressions of immunosuppressive molecules, such as PD-1 [199, 200]. However, the detailed mechanisms driving NK exhaustion in myeloma have not been completely elucidated. Innovative studies are needed to dissect molecular mechanisms involving NK cell dysfunction. In order to characterize potential mediators of NK-cell exhaustion, our previous study performed single-cell transcriptome profiling on NK cells from MM patients and HD, and identified a distinct NK subset that enriched in MM patients [35]. This MM-specific NK cluster exhibited exhaustion phenotypes by upregulating inhibitory receptors including LAG3, KLRG1, and KIR. More importantly, we further identified a vital transcription factor ZNF683 that might be responsible for the NK-cell exhaustion. Mechanistically, ZNF683 can directly suppress SH2D1B (EAT-2) expression, thus disrupting EAT-2/SLAMF7-mediated activating signals and eventually promoting NK cell exhaustion. Likewise, a ZNF683+ NK cell subset was also found in nonkeratinizing nasopharyngeal carcinoma exhibiting similar exhausted phenotypes with upregulated LAG3 and TIGIT expressions [201]. These consistent findings lead to a confirmation of ZNF683+ NK cell present in cancer context and highlight potential roles of ZNF683 in regulating NK cell function. These findings were uncovered mainly based on scRNA-seq profiling on NK cells in both studies, and we believe it may largely rely on the sensitivity of single-cell technology in capturing low abundant cells that conventional methods cannot provide.
Monocytes/macrophages
Among the most important regulators of inflammation, monocytes and macrophages play important roles in cancer-associated inflammation [202, 203]. Their critical roles in tumor progression have been extensively described in solid tumors and hematological malignancies, including MM [204, 205]. Indeed, it has been proposed that within the BME, tumor-associated monocytes and macrophages (TAMs) are able to protect MM cells from treatment-induced apoptosis, promote angiogenesis and immune evasion [206]. In addition, several studies confirmed the roles of TAM in contributing resistance to common anti-MM regimens such as melphalan or bortezomib [207, 208].
Currently, a number of surface markers have been used to characterize TAMs (including CD14, CD68, CD163 and CD206), and two major functional macrophage states have been identified: M1 (inflammatory or “classically activated”), activating during infections and M2 (suppressive, “alternative pathway”) involving in wound healing and angiogenesis. Notably, M1 and M2 macrophages should be considered as two extremes instead of a continuum, however TAMs often exhibit a mixed transcriptional profile [209,210,211]. Consistently, a recent study applied scRNA-seq to dissect TME during disease progression of myeloma, and found that reprogrammed TAMs displayed a mixed phenotype with both M1 and M2 features. They also identified two TAM clusters exclusively emerged in the MM stage and exhibited higher M2 scores, suggesting higher pro-myeloma activity of these TAMs clusters [142]. Besides, a similar polarization pattern has also been observed in monocytes. In particular, three major subsets are recognized including classical (CD14+CD16−), intermediate (CD14+/− CD16low), and non-classical monocytes (CD14−CD16+), and the latter subset is considered to harbor a tumor-promoting phenotype [212]. In the absence of standard detection tool, the TAMs percentage within BM of MM patients has been found to be highly variable (ranging from near 0 up to 25%). TAMs frequency displays an increase during the development from MGUS to MM, and patients with a high CD163+ and CD206+ TAM infiltration are associated with worse prognosis [205, 213]. A recent scRNA-seq study reported that even though reduced in MGUS compared to advanced stages, mature monocytes/macrophages are already impaired, presenting a phenotypic transition resulting in the loss of MHC class II surface representation affecting their antigen-presenting capability [39]. Another scRNA-seq study on RRMM patient samples also revealed 3 distinct TAM clusters, in which TAM cluster 3 showed a unique profile with specific expression of immunosuppressive genes (e.g., CD84 and VSIG4) [38]. Additional immune cell interaction networks showed that TAMs presented as nodes of high connectivity with other immune cells in TME, and IL18R1/IL18RAP was identified as an immunosuppressive interaction between TAM3 and NKbright cells [38].
Dendritic cells
Dendritic cells (DCs) are vital antigen-presenting cells (APCs), which serve as a bridge between innate and adaptive immunity in response to various pathogens [214]. These cells can be distinguished into two subgroups based on their function: conventional DC (cDC) and plasmacytoid DC (pDC). DCs have been found playing crucial roles in the pathogenesis and disease progression of MM [215, 216]. There is a significant difference between MM patients and HDs, with a near 50% reduction in myeloid DCs and pDCs in PB [216, 217]. Interestingly, the evolution from MGUS to MM is linked to increase in both cDCs and pDCs from the BME. As a consequence of MM, DCs also display a significantly altered immunophenotypic profile [215]. The expression levels of CCR5, CCR7 and DEC205 down-regulated on DC subtypes in MM compared to those from healthy individuals [217]. Downregulation of CCR5 and CCR7 impairs DC migration to inflammation sites, whereas reduced DEC-205 expression dampens antigen uptake. Recent studies in transcriptomics have moved beyond expression arrays of bulk populations to single-cell profiling, helping to identify novel surface markers, reveal heterogeneity within subpopulations and to identify rare but crucial DC subclusters. A recent single-cell study identified four DC subclusters in BM samples from NDMM and HDs, in which cDC2 showed a reduction in MM patients compared with HD samples. And cDC1 population showed higher expressions of MHC I/II molecules and inflammatory cytokines and chemokines in MM with low tumor infiltration than MM with high tumor infiltration group [185]. These findings imply that antigen presentation still can be triggered by cDC1 in the context of low myeloma cell infiltration, but suppressed with the increased myeloma cell infiltration. Further pathway analysis suggested that the metabolic pattern of cDC1 was affected by high level of myeloma cells. pDC is a unique DC subset defined by its essential properties of secretory plasmacytoid morphology and abundant endoplasmic reticulum, and key roles in antiviral responses by producing type I interferon (IFN) [218]. This “non-canonical” DC subset have been reported to accumulate in the BM of MM patients, and these pDC failed to stimulate T-cell proliferation but supported malignant cell growth and survival by direct contact with myeloma cells [219]. Recently, Tirier et al. generated scRNA-seq profiles from 20 RRMM tumour samples to analyze the impacts of treatments on BME cell type abundance and phenotype [38]. They found pDCs showed an expansion upon IMiD-based treatment, as well as exhibited upregulation of inhibitory receptors including LGALS9, CLEC4A and CD300A in pDCs from RRMM. These data suggested that a remodeling pro-tumorigenic phenotype of pDC contributes to RRMM immunosuppression and resistance to IMiD-based treatment. Therefore, these studies confirm that targeting pDC-MM interplay offers a promising therapeutic strategy for overcoming drug resistance in MM.
Collectively, the DC impairment in MM leads to dysfunctional capability of antigen presentation and pro-tumor activity. Through improved understanding of the interactions between myeloma cell, cDC and pDC via single-cell methodologies, targeting the crosstalk between these cells may pave the way to successful DC-targeting immunotherapies in the future.
Mesenchymal stromal cells
As an important component of the nonimmune microenvironment, mesenchymal stromal cell (MSC) has been reported to promote MM proliferation and induce drug resistance [160, 220]. Despite great enthusiasm in exploring the roles of MSCs in MM progression, the low frequency of MSCs in BM aspirates has hampered detailed investigations into the roles these cells. A recent scRNA-seq report conducted by de Jong et al. presents the first study using scRNA-seq to profile MSCs, as well as immune and myeloma cells, from patients with MM and healthy individuals to comprehensively dissect the microenvironment crosstalk in MM [132]. Using samples with over 200 million mononuclear cells and MSC enrichment allowed the authors to profile ~ 1,000-1,500 MSCs per patient. They identified total five MSC subsets, two of which (MSC1 and MSC2) were termed as iMSCs and were nearly exclusive to the MM samples. These cells were characterized by expressing inflammatory cytokines and chemokines, including several MSC regulators reported previously, such as IL6, CXCL8, CXCL2, PTGS2 and VEGFA, proteins involving in the tumor necrosis factor (TNF) pathway and CCL2, which has been shown to support myeloma cell migration via interacting with CCR2 [220,221,222]. Additionally, these iMSC subpopulations also expressed genes encoding CXCL5, CXCL3 and CD44, and the later one has been suggested as an iMSC marker in flow cytometry analysis. De Jong et al. demonstrated that IL-1β and TNF receptors were identified as key phenotypic mediators of iMSCs, which was further confirmed by the IL-1β and TNF induced activation of MSCs in vitro. The authors further investigated the intercellular crosstalk between MSC and immune or myeloma cells, and they found that TNF and IL-1β were mainly expressed by cytotoxic T cells, NK cells and monocytes respectively. Aside from communicating with myeloid subsets, iMSCs committed with proliferating MM cells via the CCL2-CCR2 signaling pathway. More importantly, they demonstrated that stromal inflammation in myeloma BME induced by immune cells persisted even achieving successful induction therapy, indicating a potential effect of iMSCs in myeloma relapse.
Taken together, high-throughput SCS technologies enhance the ability of researchers to comprehensively characterize the cellular heterogeneity, temporal/spatial evolution of tumor cells and infiltrated immune and stromal cells in MM. These approaches provide insights into the mechanisms of drug resistance, immune suppression, and disease relapse in individual MM patients, thereby contributing to develop more effective and personalized anti-myeloma therapeutics.
Future perspectives and directions
Our understanding of myeloma biology has been improved dramatically, owing to the rapid development and application of single-cell technologies. Some remained fundamental questions in myeloma research can be further explored by SCS. First, MM is an extremely complex disease whose origin and inheritability remain controversial and require detailed investigation. To explore myeloma origin, a comprehensive strategy for the identification of MMSCs could be aided by advanced SCS techniques. Owing to its ability to capture rare cell population with high-purity, and to unravel initiating point along the differential trajectory, SCS holds promise to detected the MMSCs population and identify its real markers. Indeed, scRNA-seq have been utilized to unravel the hierarchies of tumors and their progenitor cancer stem cells (CSCs) in many cancer types [223,224,225,226,227]. In addition to application in deciphering tumor origin, scRNA-seq can also be used to characterize unknown features of CSC subtypes, such as its heterogeneity, unique stem/stem-like features [103, 228, 229], which will provide novel insights into the underlying mechanisms of CSC mediated self-renewal and drug resistance. Second, single-cell profiling of myeloma cells and the surrounding immune/stromal cells enables the characterization of the sophisticated BM ecosystem, specially the complex crosstalk inferred from scRNA-seq data via predicting interactions of ligand-receptor pairs between different cell subsets. Novel approaches are also being developed to allow the investigation of physical intercellular interactions, especially the spatially resolved scRNA-seq methods. These attempts will bring a better understanding of the intricately cellular interactions within the BME. Besides, single-cell T cell receptor sequencing (scTCR-seq), with the capability to track each T-cell clone through paired sequencing of the T-cell receptor genes, can be integrated with high-dimensional single-cell spatial analysis, which has become particularly relevant to immuno-oncology [29]. And combination of scRNA-seq and scTCR-seq offers an effective method to analyze the phenotypic and functional characteristics of immune cells during disease progression or over the course of treatment. Third, high throughput spatial technologies are particularly suitable for BM and extramedullary exploration of MM, which presents a spatially divergent disease with multi-region genomic heterogeneity [135, 150]. Combined with imaging modalities, spatial transcriptomic techniques will be able to add important information on cellular location within the bone niche, contributing to a better understanding of myeloma bone disease. Extramedullary disease (EMD) presents an aggressive form of MM, which is linked to high-risk genomic alterations, increased proliferation and poor survival [230]. Innovative technologies recently revealed a complex spatial architecture of solid tumors [231,232,233,234] marked by multicellular niches supporting tumor growth or preventing immune cell infiltration. However, whether these observations hold true for EMD is largely unknown. Thus, investigating the EMD microenvironment in a spatial context using spatial omics technologies will provide a deeper understanding of ecosystems outside the BM and underlying mechanisms involving extramedullary spread.
SCS technologies also offer powerful tools for unbiased discovery of novel drug targets for MM treatment. For example, Frede et al. showed that in RRMM patients, treatment induced significant changes in chromatin accessibility by scATAC-seq, and they identified a surface protein CXCR4 as an attractive candidate as surface markers that can be targeted by immunotherapy [36]. Furthermore, another study that paves a way for incorporating scRNA-seq in clinical trials, identified PPIA as a potent therapeutic target to overcome resistance [131]. A multi-omics tool called scDAb-seq was generated [235] by combining of scDNA-seq with surface protein mapping (AB-seq [236]), which enables the characterization of both genotypic and phenotypic features. Using this elegant assay, it’s possible to determine the proteogenomic profile of clones at specific stages. Additionally, this approach allows researchers to determine the vast cell-surface proteome or “surfaceome” that regulates direct or indirect cell-cell interactions, ligand-receptor induced signaling, presentation of MM-specific antigens for immunotherapy. Impressive progresses have been achieved on determining myeloma cell surface proteome and enable unbiased discovery of novel therapeutic targets [237, 238]. CyTOF offers a new cytometric method for deep profiling single-cell protein on myeloma cells [37, 239] and immune populations [141, 240, 241]. Given the great success in immunotherapy in myeloma, we believe that application of single-cell proteomic sequencing will revolutionize the research in cancer pathology, especially when combined with multi-omic platforms, such as genetic and transcriptomic data at a single-cell resolution, and more importantly, dramatically contribute to identify novel targets for MM immunotherapy.
Preclinical models including human-derived cell lines [242] and mouse models [243] represent solid platforms for drug testing and investigation of disease mechanisms. Owing to technological advances, single-cell omics have been employed in numerous studies using clinical samples and preclinical models to investigate various pathologic conditions [244,245,246,247]. Inter-patient heterogeneity remains a key barrier to transform the preclinical findings into the clinic, with some of the patients failed to benefit from new drugs in clinical trials. With the power to dissect tumor heterogeneity, SCS can be applied in different model systems and provides rich information related to genomic, phenotypic heterogeneity and tumor evolution, thus promoting precision medicine. In the condition of MM with genetical diversity, it’s hard to recapitulate all the clinical queries by preclinical models. A recent study represents an excellent example of preclinical research by establishing fifteen genetically engineered mouse models that covered the keys factors during MM pathogenesis, including diverse genetic heterogeneity, disease progression and BM microenvironment changes [248]. Their integrative results from coupled scRNA-seq and TCR-seq highlighted the values of applying high throughput SCS on mouse and patient samples to test and predict response to immunotherapy drug combinations. Therefore, with innovations in unprecedented resolution and unbiased insights from SCS, preclinical models will accelerate our ability to answer fundamental and pivotal questions from bench to bedside, and to reproducibly apply these technologies across cancer patient populations.
Beyond being exploratory tools, SCS technologies can undoubtedly achieve more values when translated and adopted into clinical practice. Regarding the clinical utility, in our view, SCS assessments should be conducted based on the clinical needs, thus help to resolve problems encountered in the clinic. For instance, accurately monitoring measurable residual disease (MRD), identifying high risk patients and overcoming drug resistance represent three of the major unmet medical needs in MM. For the MRD measurement, a recent study has developed a single-cell MRD (scMRD) assay by combining flow cytometric enrichment of the targeted precursor/blast population in AML samples with integrated scDNA-seq and immunophenotyping, and achieved significantly high sensitivity [249]. This attempt encourages us to test single-cell based MRD monitoring on MM patients, which would be particularly helpful in disease monitoring. High-risk MM (HRMM) patients have been shown to have poor prognosis, thus accurately identifying high-risk features is a critical issue in MM. Genomic characterization of functional HRMM patients was proposed in a recent report [250], suggesting that factors outside of the myeloma cells may be critical for us to recognize the real HRMM. Thus, we can expect that comprehensively profiling both tumor cells and immune/stromal cells by single-cell approaches holds a great potential for this purpose. RRMM patients remain hard to treat, mainly due to drug resistance. The great power in predicting drug resistance in MM patients by scRNA-seq has been shown in a recent multicenter clinical trial [131], which offers a blueprint for applying SCS in clinical trials to build a drug-resistance atlas, and to promote biomarkers discovery for novel therapeutics [251].
However, applications of current SCS approaches outlined in this review are confronting with costs and technological challenges, which impeding our next step on the road to the clinic. By far the most expensive part of SCS is the sequencing itself. With the rapid advancements in sequencing technologies and exploitation of cost-reduction strategies, we believe that the sequencing costs will continue to reduce. Besides, there are several important frameworks and infrastructure are required for clinical use, including: (1) introducing standardized analytic pipelines and quality-controlled workflows when dealing with large amount of SCS data. In particular, batch effects should be carefully examined and corrected to bring reliable clinical results; (2) controlling false positive or negative rates. ScRNA-seq and scDNA-seq data would generate false negative or positive results due to the data sparsity [252], coverage nonuniformity and allelic dropout events [253]. Thus, it’s necessary to select computational methods to reduce potential false-positive/negative errors. (3) establishing clear biospecimen collection and processing procedures based on the experimental design and goals, such as what types and formats (fresh, snap-frozen and/or FFPE) of samples should be collected, optimal timepoints to collect and how sample materials should be stored; (4) developing devices or systems that automate these SCS technologies and are compatible with existing clinical laboratory workflows, thus saving time and reducing labor efforts; (5) specializing core facilities that enable secure big-data storage and enhancing collaboration between clinicians and translational scientists; Together, with the rapid advancements and further technical improvements of SCS technologies, we will be able to make single-cell assessments feasible for real-world applications in the coming years.
Conclusion
In closing, SCS technologies have already revolutionized many aspects of cancer translational research and are prepared to have a greater impact in clinic. As the last decade has seen NGS technologies transform modern oncology, we believe that single-cell methodologies will influence many areas of MM medicine in the same way, and will become a powerful tool that can be implemented for clinical practice. Over the coming years, emerging technologies such as spatial SCS and multi-omics approaches will further expand their utility in myeloma research, and bring benefits for MM patients on personalized medicine and precision prevention. Overall, we expect that the implementation of single-cell technologies in myeloma medicine over the next decade will bring huge improvements in the clinical translation and treatment of MM patients.
Availability of data and materials
Not applicable.
Abbreviations
- SCS:
-
Single-cell sequencing
- MM:
-
Multiple myeloma
- BM:
-
Bone marrow
- PIs:
-
Proteasome inhibitors
- IMiDs:
-
Immunomodulatory drugs
- BME:
-
Bone marrow microenvironment
- NGS:
-
Next generation sequencing
- CNVs:
-
Copy number variations
- WGA:
-
Whole-genome amplification
- DOP-PCR:
-
Degenerate oligonucleotide primed polymerase chain reaction
- MDA:
-
Multiple displacement amplification
- MALBAC:
-
Multiple annealing and looping based amplification cycles
- BMSCs:
-
Bone marrow stromal cells
- TME:
-
Tumor microenvironment
- MMSCs:
-
Multiple myeloma stem cells
- SP:
-
Side population
- scRNA-seq:
-
Single-cell RNA sequencing
- SNVs:
-
Single nucleotide variants
- UMIs:
-
Unique molecular identifiers
- PDAC:
-
Pancreatic ductal adenocarcinomas
- LINNAEUS:
-
Lineage tracing by nuclease-activated editing of ubiquitous sequences
- scDNA-seq:
-
Single-cell DNA sequencing
- ssRRBS:
-
Single-cell reduced-representation bisulfite sequencing
- ChIP-seq:
-
Chromatin immunoprecipitation sequencing
- scChIP-seq:
-
Single-cell chromatin immunoprecipitation followed by sequencing
- ATAC-seq:
-
Assay for transposase-accessible chromatin by sequencing
- scATAC-seq:
-
Single-cell assay for transposase-accessible chromatin by sequencing
- T-ATAC-seq:
-
Transcript-indexed assay for transposase-accessible chromatin by sequencing
- CUT&Tag:
-
Cleavage under targets and tagmentation
- IGS:
-
In situ genome sequencing
- MS:
-
Mass spectrometry
- CyTOF:
-
Cytometry by time of flight
- IMC:
-
Imaging mass cytometry
- LC-MS:
-
Liquid chromatography-mass spectrometry
- SCoPE-MS:
-
Single-cell proteomics by mass spectrometry
- scDam&T-seq:
-
Single-cell DNA adenine methyltransferase identification and transcriptome sequencing
- scM&T-Seq:
-
Single-cell genome-wide methylome and transcriptome sequencing
- CITE-seq:
-
Cellular indexing of transcriptomes and epitopes by sequencing
- REAP-seq:
-
RNA expression and protein sequencing assay
- RAID:
-
Single-cell RNA and immuno-detection
- WES:
-
Whole-exome sequencing
- MGUS:
-
Monoclonal gammopathy of undetermined significance
- SMM:
-
Smoldering multiple myeloma
- RRMM:
-
Relapsed refractory multiple myeloma
- IFN:
-
Interferon
- scVDJ-seq:
-
Single-cell variable-diversity-joining regions-targeted sequencing
- NDMM:
-
Newly diagnosed multiple myeloma
- MPP:
-
Multipotent progenitor
- CLP:
-
Common lymphoid progenitor
- OS:
-
Overall survival
- PCs:
-
Plasma cells
- OL:
-
Osteolytic lesions
- ORs:
-
Optimal responders
- SORs:
-
Suboptimal responders
- CR:
-
Complete response
- VGPR:
-
Very good partial response
- PR:
-
Partial response
- MR:
-
Minimal response
- SD:
-
Stable disease
- PD:
-
Progressive disease
- PRMM:
-
Primary refractory multiple myeloma
- SASP:
-
Senescence-associated secretory phenotype
- ICIs:
-
Immune checkpoint inhibitors
- CAR-T:
-
Chimeric antigen receptor-T
- HSP:
-
Heat shock protein
- KIR:
-
Killer cell immunoglobulin like receptor
- ADCC:
-
Antibody-dependent cellular cytotoxicity
- TF:
-
Transcription factor
- TAMs:
-
Tumor-associated macrophages
- DC:
-
Dendritic cell
- APCs:
-
Antigen-presenting cells
- cDC:
-
Conventional dendritic cell
- pDC:
-
Plasmacytoid dendritic cell
- MSC:
-
Mesenchymal stromal cell
- TNF:
-
Tumor necrosis factor
- CSCs:
-
Cancer stem cells
- scTCR-seq:
-
Single-cell T cell receptor sequencing
- EMD:
-
Extramedullary disease
- MRD:
-
Measurable residual disease
- scMRD:
-
Single-cell measurable residual disease
- HRMM:
-
High-risk multiple myeloma
- FFPE:
-
Formalin-fixed paraffin-embedded
References
Van de Donk N, Pawlyn C, Yong KL. Multiple myeloma. Lancet. 2021;397(10272):410–27.
Cowan AJ, Green DJ, Kwok M, Lee S, Coffey DG, Holmberg LA, et al. Diagnosis and management of multiple myeloma: a review. JAMA. 2022;327(5):464–77.
Kumar SK, Rajkumar SV, Dispenzieri A, Lacy MQ, Hayman SR, Buadi FK, et al. Improved survival in multiple myeloma and the impact of novel therapies. Blood. 2008;111(5):2516–20.
Dutta AK, Alberge JB, Sklavenitis-Pistofidis R, Lightbody ED, Getz G, Ghobrial IM. Single-cell profiling of tumour evolution in multiple myeloma - opportunities for precision medicine. Nat Rev Clin Oncol. 2022;19(4):223–36.
Herve AL, Florence M, Philippe M, Michel A, Thierry F, Kenneth A, et al. Molecular heterogeneity of multiple myeloma: pathogenesis, prognosis, and therapeutic implications. J Clin Oncol. 2011;29(14):1893–7.
Magrangeas F, Lode L, Wuilleme S, Minvielle S, Avet-Loiseau H. Genetic heterogeneity in multiple myeloma. Leukemia. 2005;19(2):191–4.
Manier S, Salem KZ, Park J, Landau DA, Getz G, Ghobrial IM. Genomic complexity of multiple myeloma and its clinical implications. Nat Rev Clin Oncol. 2017;14(2):100–13.
Chapman MA, Lawrence MS, Keats JJ, Cibulskis K, Sougnez C, Schinzel AC, et al. Initial genome sequencing and analysis of multiple myeloma. Nature. 2011;471(7339):467–72.
Walker BA, Boyle EM, Wardell CP, Murison A, Begum DB, Dahir NM, et al. Mutational spectrum, copy number changes, and outcome: results of a sequencing study of patients with newly diagnosed myeloma. J Clin Oncol. 2015;33(33):3911–20.
Bolli N, Avet-Loiseau H, Wedge DC, Van Loo P, Alexandrov LB, Martincorena I, et al. Heterogeneity of genomic evolution and mutational profiles in multiple myeloma. Nat Commun. 2014;5:2997.
Hideshima T, Mitsiades C, Tonon G, Richardson PG, Anderson KC. Understanding multiple myeloma pathogenesis in the bone marrow to identify new therapeutic targets. Nat Rev Cancer. 2007;7(8):585–98.
Lomas OC, Tahri S, Ghobrial IM. The microenvironment in myeloma. Curr Opin Oncol. 2020;32(2):170–5.
Kawano Y, Roccaro AM, Ghobrial IM, Azzi J. Multiple myeloma and the immune microenvironment. Curr Cancer Drug Targets. 2017;17(9):806–18.
Dosani T, Mailankody S, Korde N, Manasanch E, Bhutani M, Tageja N, et al. Host-related immunodeficiency in the development of multiple myeloma. Leuk Lymphoma. 2018;59(5):1127–32.
Swamydas M, Murphy EV, Ignatz-Hoover JJ, Malek E, Driscoll JJ. Deciphering mechanisms of immune escape to inform immunotherapeutic strategies in multiple myeloma. J Hematol Oncol. 2022;15(1):17.
Lopes R, Caetano J, Ferreira B, Barahona F, Carneiro EA, Joao C. The immune microenvironment in multiple myeloma: friend or foe? Cancers (Basel). 2021;13(4):625.
Cornell RF, Kassim AA. Evolving paradigms in the treatment of relapsed/refractory multiple myeloma: increased options and increased complexity. Bone Marrow Transplant. 2016;51(4):479–91.
Matsui W, Huff CA, Wang Q, Malehorn MT, Barber J, Tanhehco Y, et al. Characterization of clonogenic multiple myeloma cells. Blood. 2004;103(6):2332–6.
Huff CA, Matsui W. Multiple myeloma cancer stem cells. J Clin Oncol. 2008;26(17):2895–900.
Matsui W, Wang Q, Barber JP, Brennan S, Smith BD, Borrello I, et al. Clonogenic multiple myeloma progenitors, stem cell properties, and drug resistance. Cancer Res. 2008;68(1):190–7.
Wu C, Alman BA. Side population cells in human cancers. Cancer Lett. 2008;268(1):1–9.
Zhou W, Yang Y, Gu Z, Wang H, Xia J, Wu X, et al. ALDH1 activity identifies tumor-initiating cells and links to chromosomal instability signatures in multiple myeloma. Leukemia. 2014;28(5):1155–8.
Gao M, Bai H, Jethava Y, Wu Y, Zhu Y, Yang Y, et al. Identification and characterization of tumor-initiating cells in multiple myeloma. J Natl Cancer Inst. 2020;112(5):507–15.
Van Nieuwenhuijzen N, Spaan I, Raymakers R, Peperzak V. From MGUS to multiple myeloma, a paradigm for clonal evolution of premalignant cells. Cancer Res. 2018;78(10):2449–56.
Bolli N, Maura F, Minvielle S, Gloznik D, Szalat R, Fullam A, et al. Genomic patterns of progression in smoldering multiple myeloma. Nat Commun. 2018;9(1):3363.
Lohr JG, Stojanov P, Carter SL, Cruz-Gordillo P, Lawrence MS, Auclair D, et al. Widespread genetic heterogeneity in multiple myeloma: implications for targeted therapy. Cancer Cell. 2014;25(1):91–101.
Keats JJ, Chesi M, Egan JB, Garbitt VM, Palmer SE, Braggio E, et al. Clonal competition with alternating dominance in multiple myeloma. Blood. 2012;120(5):1067–76.
Walker BA, Wardell CP, Melchor L, Hulkki S, Potter NE, Johnson DC, et al. Intraclonal heterogeneity and distinct molecular mechanisms characterize the development of t(4;14) and t(11;14) myeloma. Blood. 2012;120(5):1077–86.
Gohil SH, Iorgulescu JB, Braun DA, Keskin DB, Livak KJ. Applying high-dimensional single-cell technologies to the analysis of cancer immunotherapy. Nat Rev Clin Oncol. 2021;18(4):244–56.
Papalexi E, Satija R. Single-cell RNA sequencing to explore immune cell heterogeneity. Nat Rev Immunol. 2017;18(1):35–45.
Lei Y, Tang R, Xu J, Wang W, Zhang B, Liu J, et al. Applications of single-cell sequencing in cancer research: progress and perspectives. J Hematol Oncol. 2021;14(1):91.
Potter SS. Single-cell RNA sequencing for the study of development, physiology and disease. Nat Rev Nephrol. 2018;14(8):479–92.
Ledergor G, Weiner A, Zada M, Wang SY, Cohen YC, Gatt ME, et al. Single cell dissection of plasma cell heterogeneity in symptomatic and asymptomatic myeloma. Nat Med. 2018;24(12):1867–76.
He H, Li Z, Lu J, Qiang W, Jiang S, Xu Y, et al. Single-cell RNA-seq reveals clonal diversity and prognostic genes of relapsed multiple myeloma. Clin Transl Med. 2022;12(3): e757.
Li X, Chen M, Wan Y, Zhong L, Han X, Chen X, et al. Single-cell transcriptome profiling reveals the key role of ZNF683 in natural killer cell exhaustion in multiple myeloma. Clin Transl Med. 2022;12(10): e1065.
Frede J, Anand P, Sotudeh N, Pinto RA, Nair MS, Stuart H, et al. Dynamic transcriptional reprogramming leads to immunotherapeutic vulnerabilities in myeloma. Nat Cell Biol. 2021;23(11):1199–211.
Liu R, Gao Q, Foltz SM, Fowles JS, Yao L, Wang JT, et al. Co-evolution of tumor and immune cells during progression of multiple myeloma. Nat Commun. 2021;12(1):2559.
Tirier SM, Mallm JP, Steiger S, Poos AM, Awwad MHS, Giesen N, et al. Subclone-specific microenvironmental impact and drug response in refractory multiple myeloma revealed by single-cell transcriptomics. Nat Commun. 2021;12(1):6960.
Zavidij O, Haradhvala NJ, Mouhieddine TH, Sklavenitis-Pistofidis R, Cai S, Reidy M, et al. Single-cell RNA sequencing reveals compromised immune microenvironment in precursor stages of multiple myeloma. Nat Cancer. 2020;1(5):493–506.
Wang L, Livak KJ, Wu CJ. High-dimension single-cell analysis applied to cancer. Mol Aspects Med. 2018;59:70–84.
Gomes T, Teichmann SA, Talavera-Lopez C. Immunology driven by large-scale single-cell sequencing. Trends Immunol. 2019;40(11):1011–21.
Tang X, Huang Y, Lei J, Luo H, Zhu X. The single-cell sequencing: new developments and medical applications. Cell Biosci. 2019;9:53.
Qi R, Ma A, Ma Q, Zou Q. Clustering and classification methods for single-cell RNA-sequencing data. Brief Bioinform. 2020;21(4):1196–208.
Luecken MD, Theis FJ. Current best practices in single-cell RNA-seq analysis: a tutorial. Mol Syst Biol. 2019;15(6): e8746.
Saelens W, Cannoodt R, Todorov H, Saeys Y. A comparison of single-cell trajectory inference methods. Nat Biotechnol. 2019;37(5):547–54.
Zheng GX, Terry JM, Belgrader P, Ryvkin P, Bent ZW, Wilson R, et al. Massively parallel digital transcriptional profiling of single cells. Nat Commun. 2017;8:14049.
Macosko EZ, Basu A, Satija R, Nemesh J, Shekhar K, Goldman M, et al. Highly parallel genome-wide expression profiling of individual cells using nanoliter droplets. Cell. 2015;161(5):1202–14.
Zhu C, Yu M, Huang H, Juric I, Abnousi A, Hu R, Lucero J, Behrens MM, Hu M, Ren B. An ultra high-throughput method for single-cell joint analysis of open chromatin and transcriptome. Nat Struct Mol Biol. 2019;26(11):1063–70.
Gierahn TM, Wadsworth MH 2nd, Hughes TK, Bryson BD, Butler A, Satija R, et al. Seq-Well: portable, low-cost RNA sequencing of single cells at high throughput. Nat Methods. 2017;14(4):395–8.
Fan HC, Fu GK, Fodor SP. Expression profiling. combinatorial labeling of single cells for gene expression cytometry. Science. 2015;347(6222):1258367.
Ramskold D, Luo S, Wang YC, Li R, Deng Q, Faridani OR, et al. Full-length mRNA-Seq from single-cell levels of RNA and individual circulating tumor cells. Nat Biotechnol. 2012;30(8):777–82.
Hashimshony T, Senderovich N, Avital G, Klochendler A, de Leeuw Y, Anavy L, et al. CEL-Seq2: sensitive highly-multiplexed single-cell RNA-Seq. Genome Biol. 2016;17:77.
Cao J, Packer JS, Ramani V, Cusanovich DA, Huynh C, Daza R, et al. Comprehensive single-cell transcriptional profiling of a multicellular organism. Science. 2017;357(6352):661–7.
Jaitin DA, Kenigsberg E, Keren-Shaul H, Elefant N, Paul F, Zaretsky I, et al. Massively parallel single-cell RNA-seq for marker-free decomposition of tissues into cell types. Science. 2014;343(6172):776–9.
Stahl PL, Salmen F, Vickovic S, Lundmark A, Navarro JF, Magnusson J, et al. Visualization and analysis of gene expression in tissue sections by spatial transcriptomics. Science. 2016;353(6294):78–82.
Rodriques SG, Stickels RR, Goeva A, Martin CA, Murray E, Vanderburg CR, et al. Slide-seq: a scalable technology for measuring genome-wide expression at high spatial resolution. Science. 2019;363(6434):1463–7.
Wei X, Fu S, Li H, Liu Y, Wang S, Feng W, et al. Single-cell Stereo-seq reveals induced progenitor cells involved in axolotl brain regeneration. Science. 2022;377(6610):eabp9444.
Liu Y, Yang M, Deng Y, Su G, Enninful A, Guo CC, et al. High-spatial-resolution multi-omics sequencing via deterministic barcoding in tissue. Cell. 2020;183(6):1665-81 e18.
Chen KH, Boettiger AN, Moffitt JR, Wang S, Zhuang X. RNA imaging. spatially resolved, highly multiplexed RNA profiling in single cells. Science. 2015;348(6233):aaa6090.
Lubeck E, Coskun AF, Zhiyentayev T, Ahmad M, Cai L. Single-cell in situ RNA profiling by sequential hybridization. Nat Methods. 2014;11(4):360–1.
Wang X, Allen WE, Wright MA, Sylwestrak EL, Samusik N, Vesuna S, et al. Three-dimensional intact-tissue sequencing of single-cell transcriptional states. Science. 2018;361(6400):eaat5691.
Telenius H, Carter NP, Bebb CE, Nordenskjold M, Ponder BA, Tunnacliffe A. Degenerate oligonucleotide-primed PCR: general amplification of target DNA by a single degenerate primer. Genomics. 1992;13(3):718–25.
Baslan T, Kendall J, Rodgers L, Cox H, Riggs M, Stepansky A, et al. Genome-wide copy number analysis of single cells. Nat Protoc. 2012;7(6):1024–41.
Dean FB, Hosono S, Fang L, Wu X, Faruqi AF, Bray-Ward P, et al. Comprehensive human genome amplification using multiple displacement amplification. Proc Natl Acad Sci U S A. 2002;99(8):5261–6.
Zong C, Lu S, Chapman AR, Xie XS. Genome-wide detection of single-nucleotide and copy-number variations of a single human cell. Science. 2012;338(6114):1622–6.
Guo H, Zhu P, Wu X, Li X, Wen L, Tang F. Single-cell methylome landscapes of mouse embryonic stem cells and early embryos analyzed using reduced representation bisulfite sequencing. Genome Res. 2013;23(12):2126–35.
Farlik M, Sheffield NC, Nuzzo A, Datlinger P, Schonegger A, Klughammer J, et al. Single-cell DNA methylome sequencing and bioinformatic inference of epigenomic cell-state dynamics. Cell Rep. 2015;10(8):1386–97.
Han L, Wu HJ, Zhu H, Kim KY, Marjani SL, Riester M, et al. Bisulfite-independent analysis of CpG island methylation enables genome-scale stratification of single cells. Nucleic Acids Res. 2017;45(10): e77.
Buenrostro JD, Wu B, Litzenburger UM, Ruff D, Gonzales ML, Snyder MP, et al. Single-cell chromatin accessibility reveals principles of regulatory variation. Nature. 2015;523(7561):486–90.
Chen X, Miragaia RJ, Natarajan KN, Teichmann SA. A rapid and robust method for single cell chromatin accessibility profiling. Nat Commun. 2018;9(1):5345.
Satpathy AT, Granja JM, Yost KE, Qi Y, Meschi F, McDermott GP, et al. Massively parallel single-cell chromatin landscapes of human immune cell development and intratumoral T cell exhaustion. Nat Biotechnol. 2019;37(8):925–36.
Rotem A, Ram O, Shoresh N, Sperling RA, Goren A, Weitz DA, et al. Single-cell ChIP-seq reveals cell subpopulations defined by chromatin state. Nat Biotechnol. 2015;33(11):1165–72.
Bartosovic M, Kabbe M, Castelo-Branco G. Single-cell CUT&Tag profiles histone modifications and transcription factors in complex tissues. Nat Biotechnol. 2021;39(7):825–35.
Labib M, Kelley SO. Single-cell analysis targeting the proteome. Nat Rev Chem. 2020;4(3):143–58.
Bennett HM, Stephenson W, Rose CM, Darmanis S. Single-cell proteomics enabled by next-generation sequencing or mass spectrometry. Nat Methods. 2023;20(3):363–74.
Luo C, Liu H, Wang B-A, Bartlett A, Rivkin A, Nery JR, et al. Multi-omic profiling of transcriptome and DNA methylome in single nuclei with molecular partitioning. bioRxiv. 2018:434845.
Dey SS, Kester L, Spanjaard B, Bienko M, van Oudenaarden A. Integrated genome and transcriptome sequencing of the same cell. Nat Biotechnol. 2015;33(3):285–9.
Rodriguez-Meira A, Buck G, Clark SA, Povinelli BJ, Alcolea V, Louka E, et al. Unravelling Intratumoral Heterogeneity through High-Sensitivity Single-Cell Mutational Analysis and Parallel RNA Sequencing. Mol Cell. 2019;73(6):1292-305 e8.
Angermueller C, Clark SJ, Lee HJ, Macaulay IC, Teng MJ, Hu TX, et al. Parallel single-cell sequencing links transcriptional and epigenetic heterogeneity. Nat Methods. 2016;13(3):229–32.
Hou Y, Guo H, Cao C, Li X, Hu B, Zhu P, et al. Single-cell triple omics sequencing reveals genetic, epigenetic, and transcriptomic heterogeneity in hepatocellular carcinomas. Cell Res. 2016;26(3):304–19.
Cao J, Cusanovich DA, Ramani V, Aghamirzaie D, Pliner HA, Hill AJ, et al. Joint profiling of chromatin accessibility and gene expression in thousands of single cells. Science. 2018;361(6409):1380–5.
Stoeckius M, Hafemeister C, Stephenson W, Houck-Loomis B, Chattopadhyay PK, Swerdlow H, et al. Simultaneous epitope and transcriptome measurement in single cells. Nat Methods. 2017;14(9):865–8.
Peterson VM, Zhang KX, Kumar N, Wong J, Li L, Wilson DC, et al. Multiplexed quantification of proteins and transcripts in single cells. Nat Biotechnol. 2017;35(10):936–9.
Gerlach JP, van Buggenum JAG, Tanis SEJ, Hogeweg M, Heuts BMH, Muraro MJ, et al. Combined quantification of intracellular (phospho-)proteins and transcriptomics from fixed single cells. Sci Rep. 2019;9(1):1469.
Rooijers K, Markodimitraki CM, Rang FJ, de Vries SS, Chialastri A, de Luca KL, et al. Simultaneous quantification of protein-DNA contacts and transcriptomes in single cells. Nat Biotechnol. 2019;37(7):766–72.
Hwang B, Lee JH, Bang D. Single-cell RNA sequencing technologies and bioinformatics pipelines. Exp Mol Med. 2018;50(8):1–14.
Xin Y, Kim J, Ni M, Wei Y, Okamoto H, Lee J, et al. Use of the Fluidigm C1 platform for RNA sequencing of single mouse pancreatic islet cells. Proc Natl Acad Sci U S A. 2016;113(12):3293–8.
Wadsworth MH 2nd, Hughes TK, Shalek AK. Marrying microfluidics and microwells for parallel, high-throughput single-cell genomics. Genome Biol. 2015;16(1):129.
Picelli S, Bjorklund AK, Faridani OR, Sagasser S, Winberg G, Sandberg R. Smart-seq2 for sensitive full-length transcriptome profiling in single cells. Nat Methods. 2013;10(11):1096–8.
Tang F, Barbacioru C, Wang Y, Nordman E, Lee C, Xu N, et al. mRNA-Seq whole-transcriptome analysis of a single cell. Nat Methods. 2009;6(5):377–82.
Islam S, Kjallquist U, Moliner A, Zajac P, Fan JB, Lonnerberg P, et al. Characterization of the single-cell transcriptional landscape by highly multiplex RNA-seq. Genome Res. 2011;21(7):1160–7.
Picelli S, Faridani OR, Bjorklund AK, Winberg G, Sagasser S, Sandberg R. Full-length RNA-seq from single cells using Smart-seq2. Nat Protoc. 2014;9(1):171–81.
Klein AM, Mazutis L, Akartuna I, Tallapragada N, Veres A, Li V, et al. Droplet barcoding for single-cell transcriptomics applied to embryonic stem cells. Cell. 2015;161(5):1187–201.
Zhang X, Li T, Liu F, Chen Y, Yao J, Li Z, et al. Comparative analysis of droplet-based ultra-high-throughput single-cell RNA-Seq systems. Mol Cell. 2019;73(1):130-42 e5.
Ding J, Adiconis X, Simmons SK, Kowalczyk MS, Hession CC, Marjanovic ND, et al. Systematic comparison of single-cell and single-nucleus RNA-sequencing methods. Nat Biotechnol. 2020;38(6):737–46.
Wang X, He Y, Zhang Q, Ren X, Zhang Z. Direct comparative analyses of 10X genomics chromium and smart-seq2. Genomics Proteomics Bioinformatics. 2021;19(2):253–66.
Zhang Q, He Y, Luo N, Patel SJ, Han Y, Gao R, et al. Landscape and dynamics of single immune cells in hepatocellular carcinoma. Cell. 2019;179(4):829-45 e20.
Geng S, Wang J, Zhang X, Zhang JJ, Wu F, Pang Y, et al. Single-cell RNA sequencing reveals chemokine self-feeding of myeloma cells promotes extramedullary metastasis. FEBS Lett. 2020;594(3):452–65.
Moncada R, Barkley D, Wagner F, Chiodin M, Devlin JC, Baron M, et al. Integrating microarray-based spatial transcriptomics and single-cell RNA-seq reveals tissue architecture in pancreatic ductal adenocarcinomas. Nat Biotechnol. 2020;38(3):333–42.
Michor F, Iwasa Y, Nowak MA. Dynamics of cancer progression. Nat Rev Cancer. 2004;4(3):197–205.
Spanjaard B, Hu B, Mitic N, Olivares-Chauvet P, Janjuha S, Ninov N, et al. Simultaneous lineage tracing and cell-type identification using CRISPR-Cas9-induced genetic scars. Nat Biotechnol. 2018;36(5):469–73.
Quinn JJ, Jones MG, Okimoto RA, Nanjo S, Chan MM, Yosef N, et al. Single-cell lineages reveal the rates, routes, and drivers of metastasis in cancer xenografts. Science. 2021;371(6532):eabc1944.
Velten L, Story BA, Hernandez-Malmierca P, Raffel S, Leonce DR, Milbank J, et al. Identification of leukemic and pre-leukemic stem cells by clonal tracking from single-cell transcriptomics. Nat Commun. 2021;12(1):1366.
Gawad C, Koh W, Quake SR. Single-cell genome sequencing: current state of the science. Nat Rev Genet. 2016;17(3):175–88.
Mallory XF, Edrisi M, Navin N, Nakhleh L. Methods for copy number aberration detection from single-cell DNA-sequencing data. Genome Biol. 2020;21(1):208.
Andor N, Lau BT, Catalanotti C, Sathe A, Kubit M, Chen J, et al. Joint single cell DNA-seq and RNA-seq of gastric cancer cell lines reveals rules of in vitro evolution. NAR Genom Bioinform. 2020;2(2):lqaa016.
Lan F, Demaree B, Ahmed N, Abate AR. Single-cell genome sequencing at ultra-high-throughput with microfluidic droplet barcoding. Nat Biotechnol. 2017;35(7):640–6.
Laks E, McPherson A, Zahn H, Lai D, Steif A, Brimhall J, et al. Clonal decomposition and DNA replication states defined by scaled single-cell genome sequencing. Cell. 2019;179(5):1207-21 e22.
Zahn H, Steif A, Laks E, Eirew P, VanInsberghe M, Shah SP, et al. Scalable whole-genome single-cell library preparation without preamplification. Nat Methods. 2017;14(2):167–73.
Hou Y, Song L, Zhu P, Zhang B, Tao Y, Xu X, et al. Single-cell exome sequencing and monoclonal evolution of a JAK2-negative myeloproliferative neoplasm. Cell. 2012;148(5):873–85.
Wang Y, Waters J, Leung ML, Unruh A, Roh W, Shi X, et al. Clonal evolution in breast cancer revealed by single nucleus genome sequencing. Nature. 2014;512(7513):155–60.
Leung ML, Wang Y, Kim C, Gao R, Jiang J, Sei E, et al. Highly multiplexed targeted DNA sequencing from single nuclei. Nat Protoc. 2016;11(2):214–35.
Leung ML, Davis A, Gao R, Casasent A, Wang Y, Sei E, et al. Single-cell DNA sequencing reveals a late-dissemination model in metastatic colorectal cancer. Genome Res. 2017;27(8):1287–99.
Guo H, Zhu P, Guo F, Li X, Wu X, Fan X, et al. Profiling DNA methylome landscapes of mammalian cells with single-cell reduced-representation bisulfite sequencing. Nat Protoc. 2015;10(5):645–59.
Gutin J, Sadeh R, Bodenheimer N, Joseph-Strauss D, Klein-Brill A, Alajem A, et al. Fine-resolution mapping of TF binding and chromatin interactions. Cell Rep. 2018;22(10):2797–807.
Cusanovich DA, Daza R, Adey A, Pliner HA, Christiansen L, Gunderson KL, et al. Multiplex single cell profiling of chromatin accessibility by combinatorial cellular indexing. Science. 2015;348(6237):910–4.
Berest I, Tangherloni A. Integration of scATAC-Seq with scRNA-Seq Data. Methods Mol Biol. 2023;2584:293–310.
Satpathy AT, Saligrama N, Buenrostro JD, Wei Y, Wu B, Rubin AJ, et al. Transcript-indexed ATAC-seq for precision immune profiling. Nat Med. 2018;24(5):580–90.
Payne AC, Chiang ZD, Reginato PL, Mangiameli SM, Murray EM, Yao CC, et al. In situ genome sequencing resolves DNA sequence and structure in intact biological samples. Science. 2021;371(6532):eaay3446.
Tan L, Ma W, Wu H, Zheng Y, Xing D, Chen R, et al. Changes in genome architecture and transcriptional dynamics progress independently of sensory experience during post-natal brain development. Cell. 2021;184(3):741-58 e17.
Gonzalez VD, Huang YW, Fantl WJ. Mass cytometry for the characterization of individual cell types in ovarian solid tumors. Methods Mol Biol. 2022;2424:59–94.
Glasson Y, Chepeaux LA, Dume AS, Lafont V, Faget J, Bonnefoy N, et al. Single-cell high-dimensional imaging mass cytometry: one step beyond in oncology. Semin Immunopathol. 2023:1–12.
Veenstra J, Dimitrion P, Yao Y, Zhou L, Ozog D, Mi QS. Research techniques made simple: use of imaging mass cytometry for dermatological research and clinical applications. J Invest Dermatol. 2021;141(4):705-12 e1.
Marx V. A dream of single-cell proteomics. Nat Methods. 2019;16(9):809–12.
Cheung TK, Lee CY, Bayer FP, McCoy A, Kuster B, Rose CM. Defining the carrier proteome limit for single-cell proteomics. Nat Methods. 2021;18(1):76–83.
Stuart T, Satija R. Integrative single-cell analysis. Nat Rev Genet. 2019;20(5):257–72.
Kong SL, Li H, Tai JA, Courtois ET, Poh HM, Lau DP, et al. Concurrent single-cell RNA and targeted DNA sequencing on an automated platform for comeasurement of genomic and transcriptomic signatures. Clin Chem. 2019;65(2):272–81.
Markodimitraki CM, Rang FJ, Rooijers K, de Vries SS, Chialastri A, de Luca KL, et al. Simultaneous quantification of protein-DNA interactions and transcriptomes in single cells with scDam&T-seq. Nat Protoc. 2020;15(6):1922–53.
Rios AC, Capaldo BD, Vaillant F, Pal B, van Ineveld R, Dawson CA, et al. Intraclonal plasticity in mammary tumors revealed through large-scale single-cell resolution 3D imaging. Cancer Cell. 2019;35(6):953.
Khoo WH, Ledergor G, Weiner A, Roden DL, Terry RL, McDonald MM, et al. A niche-dependent myeloid transcriptome signature defines dormant myeloma cells. Blood. 2019;134(1):30–43.
Cohen YC, Zada M, Wang SY, Bornstein C, David E, Moshe A, et al. Identification of resistance pathways and therapeutic targets in relapsed multiple myeloma patients through single-cell sequencing. Nat Med. 2021;27(3):491–503.
de Jong MME, Kellermayer Z, Papazian N, Tahri S, Hofste Op Bruinink D, Hoogenboezem R, et al. The multiple myeloma microenvironment is defined by an inflammatory stromal cell landscape. Nat Immunol. 2021;22(6):769–80.
Cho H, Kim KH, Lee H, Kim CG, Chung H, Choi YS, et al. Adaptive natural killer cells facilitate effector functions of daratumumab in multiple myeloma. Clin Cancer Res. 2021;27(10):2947–58.
Ryu D, Kim SJ, Hong Y, Jo A, Kim N, Kim HJ, et al. Alterations in the transcriptional programs of myeloma cells and the microenvironment during extramedullary progression affect proliferation and immune evasion. Clin Cancer Res. 2020;26(4):935–44.
Merz M, Merz AMA, Wang J, Wei L, Hu Q, Hutson N, et al. Deciphering spatial genomic heterogeneity at a single cell resolution in multiple myeloma. Nat Commun. 2022;13(1):807.
Rodriguez-Marquez P, Calleja-Cervantes ME, Serrano G, Oliver-Caldes A, Palacios-Berraquero ML, Martin-Mallo A, et al. CAR density influences antitumoral efficacy of BCMA CAR T cells and correlates with clinical outcome. Sci Adv. 2022;8(39):eabo0514.
Da Via MC, Dietrich O, Truger M, Arampatzi P, Duell J, Heidemeier A, et al. Homozygous BCMA gene deletion in response to anti-BCMA CAR T cells in a patient with multiple myeloma. Nat Med. 2021;27(4):616–9.
Liang Y, He H, Wang W, Wang H, Mo S, Fu R, et al. Malignant clonal evolution drives multiple myeloma cellular ecological diversity and microenvironment reprogramming. Mol Cancer. 2022;21(1):182.
Masuda T, Haji S, Nakashima Y, Tsuda M, Kimura D, Takamatsu A, et al. Identification of a drug-response gene in multiple myeloma through longitudinal single-cell transcriptome sequencing. iScience. 2022;25(8):104781.
Jung SH, Park SS, Lim JY, Sohn SY, Kim NY, Kim D, et al. Single-cell analysis of multiple myelomas refines the molecular features of bortezomib treatment responsiveness. Exp Mol Med. 2022;54(11):1967–78.
Yao L, Jayasinghe RG, Lee BH, Bhasin SS, Pilcher W, Doxie DB, et al. Comprehensive characterization of the multiple myeloma immune microenvironment using integrated scRNA-seq, CyTOF, and CITE-seq aAnalysis. Cancer Research Communications. 2022;2(10):1255–65.
Li J, Yang Y, Wang W, Xu J, Sun Y, Jiang J, et al. Single-cell atlas of the immune microenvironment reveals macrophage reprogramming and the potential dual macrophage-targeted strategy in multiple myeloma. Br J Haematol. 2023.
Schavgoulidze A, Cazaubiel T, Perrot A, Avet-Loiseau H, Corre J. Multiple myeloma: heterogeneous in every way. Cancers (Basel). 2021;13(6):1285.
Melchor L, Brioli A, Wardell CP, Murison A, Potter NE, Kaiser MF, et al. Single-cell genetic analysis reveals the composition of initiating clones and phylogenetic patterns of branching and parallel evolution in myeloma. Leukemia. 2014;28(8):1705–15.
Boiarsky R, Haradhvala NJ, Alberge JB, Sklavenitis-Pistofidis R, Mouhieddine TH, Zavidij O, et al. Single cell characterization of myeloma and its precursor conditions reveals transcriptional signatures of early tumorigenesis. Nat Commun. 2022;13(1):7040.
Croucher DC, Richards LM, Tsofack SP, Waller D, Li Z, Wei EN, et al. Longitudinal single-cell analysis of a myeloma mouse model identifies subclonal molecular programs associated with progression. Nat Commun. 2021;12(1):6322.
Bazarbachi AH, Al Hamed R, Malard F, Harousseau JL, Mohty M. Relapsed refractory multiple myeloma: a comprehensive overview. Leukemia. 2019;33(10):2343–57.
Ghobrial IM, Liu CJ, Redd RA, Perez RP, Baz R, Zavidij O, et al. A phase Ib/II trial of the first-in-class anti-CXCR4 antibody ulocuplumab in combination with lenalidomide or bortezomib plus dexamethasone in relapsed multiple myeloma. Clin Cancer Res. 2020;26(2):344–53.
Mandal M, Okoreeh MK, Kennedy DE, Maienschein-Cline M, Ai J, McLean KC, et al. CXCR4 signaling directs Igk recombination and the molecular mechanisms of late B lymphopoiesis. Nat Immunol. 2019;20(10):1393–403.
Rasche L, Chavan SS, Stephens OW, Patel PH, Tytarenko R, Ashby C, et al. Spatial genomic heterogeneity in multiple myeloma revealed by multi-region sequencing. Nat Commun. 2017;8(1):268.
Rasche L, Kortum KM, Raab MS, Weinhold N. The impact of tumor heterogeneity on diagnostics and novel therapeutic strategies in multiple myeloma. Int J Mol Sci. 2019;20(5):1248.
Zamagni E, Tacchetti P, Barbato S, Cavo M. Role of imaging in the evaluation of minimal residual disease in multiple myeloma patients. J Clin Med. 2020;9(11):3519.
Hu X, Wu CH, Cowan JM, Comenzo RL, Varga C. Outcomes of patients with multiple myeloma harboring chromosome 1q gain/amplification in the era of modern therapy. Ann Hematol. 2022;101(2):369–78.
Weinhold N, Salwender HJ, Cairns DA, Raab MS, Waldron G, Blau IW, et al. Chromosome 1q21 abnormalities refine outcome prediction in patients with multiple myeloma - a meta-analysis of 2,596 trial patients. Haematologica. 2021;106(10):2754–8.
Chen M, Wan Y, Li X, Xiang J, Chen X, Jiang J, et al. Dynamic single-cell RNA-seq analysis reveals distinct tumor program associated with microenvironmental remodeling and drug sensitivity in multiple myeloma. Cell Biosci. 2023;13(1):19.
Kawano Y, Moschetta M, Manier S, Glavey S, Gorgun GT, Roccaro AM, et al. Targeting the bone marrow microenvironment in multiple myeloma. Immunol Rev. 2015;263(1):160–72.
Giannakoulas N, Ntanasis-Stathopoulos I, Terpos E. The role of marrow microenvironment in the growth and development of malignant plasma cells in multiple myeloma. Int J Mol Sci. 2021;22(9):4462.
Andrews SW, Kabrah S, May JE, Donaldson C, Morse HR. Multiple myeloma: the bone marrow microenvironment and its relation to treatment. Br J Biomed Sci. 2013;70(3):110–20.
Maiso P, Mogollon P, Ocio EM, Garayoa M. Bone marrow mesenchymal stromal cells in multiple myeloma: their role as active contributors to myeloma progression. Cancers (Basel). 2021;13(11):2542.
Xu S, De Veirman K, De Becker A, Vanderkerken K, Van Riet I. Mesenchymal stem cells in multiple myeloma: a therapeutical tool or target? Leukemia. 2018;32(7):1500–14.
Terpos E, Ntanasis-Stathopoulos I, Gavriatopoulou M, Dimopoulos MA. Pathogenesis of bone disease in multiple myeloma: from bench to bedside. Blood Cancer J. 2018;8(1):7.
Nakamura K, Smyth MJ, Martinet L. Cancer immunoediting and immune dysregulation in multiple myeloma. Blood. 2020;136(24):2731–40.
Minnie SA, Hill GR. Immunotherapy of multiple myeloma. J Clin Invest. 2020;130(4):1565–75.
Jakob C, Sterz J, Zavrski I, Heider U, Kleeberg L, Fleissner C, et al. Angiogenesis in multiple myeloma. Eur J Cancer. 2006;42(11):1581–90.
Joshua D, Suen H, Brown R, Bryant C, Ho PJ, Hart D, et al. The T Cell in myeloma. Clin Lymphoma Myeloma Leuk. 2016;16(10):537–42.
Zelle-Rieser C, Thangavadivel S, Biedermann R, Brunner A, Stoitzner P, Willenbacher E, et al. T cells in multiple myeloma display features of exhaustion and senescence at the tumor site. J Hematol Oncol. 2016;9(1):116.
Suen H, Brown R, Yang S, Weatherburn C, Ho PJ, Woodland N, et al. Multiple myeloma causes clonal T-cell immunosenescence: identification of potential novel targets for promoting tumour immunity and implications for checkpoint blockade. Leukemia. 2016;30(8):1716–24.
Prabhala RH, Neri P, Bae JE, Tassone P, Shammas MA, Allam CK, et al. Dysfunctional T regulatory cells in multiple myeloma. Blood. 2006;107(1):301–4.
Favaloro J, Brown R, Aklilu E, Yang S, Suen H, Hart D, et al. Myeloma skews regulatory T and pro-inflammatory T helper 17 cell balance in favor of a suppressive state. Leuk Lymphoma. 2014;55(5):1090–8.
Prabhala RH, Pelluru D, Fulciniti M, Prabhala HK, Nanjappa P, Song W, et al. Elevated IL-17 produced by TH17 cells promotes myeloma cell growth and inhibits immune function in multiple myeloma. Blood. 2010;115(26):5385–92.
Brown R, Kabani K, Favaloro J, Yang S, Ho PJ, Gibson J, et al. CD86+ or HLA-G+ can be transferred via trogocytosis from myeloma cells to T cells and are associated with poor prognosis. Blood. 2012;120(10):2055–63.
Schinke C, Poos AM, Bauer M, John L, Johnson S, Deshpande S, et al. Characterizing the role of the immune microenvironment in multiple myeloma progression at a single-cell level. Blood Adv. 2022;6(22):5873–83.
Mozaffari F, Hansson L, Kiaii S, Ju X, Rossmann ED, Rabbani H, et al. Signalling molecules and cytokine production in T cells of multiple myeloma-increased abnormalities with advancing stage. Br J Haematol. 2004;124(3):315–24.
Bailur JK, McCachren SS, Doxie DB, Shrestha M, Pendleton K, Nooka AK, et al. Early alterations in stem-like/resident T cells, innate and myeloid cells in the bone marrow in preneoplastic gammopathy. JCI Insight. 2019;5(11):e127807.
Wang J, Zheng Y, Tu C, Zhang H, Vanderkerken K, Menu E, et al. Identification of the immune checkpoint signature of multiple myeloma using mass cytometry-based single-cell analysis. Clin Transl Immunology. 2020;9(5): e01132.
Castella B, Foglietta M, Riganti C, Massaia M. Vgamma9Vdelta2 T Cells in the bone marrow of myeloma patients: a paradigm of microenvironment-induced immune suppression. Front Immunol. 2018;9:1492.
Mensurado S, Blanco-Dominguez R, Silva-Santos B. The emerging roles of gammadelta T cells in cancer immunotherapy. Nat Rev Clin Oncol. 2023;20(3):178–91.
Rancan C, Arias-Badia M, Dogra P, Chen B, Aran D, Yang H, et al. Exhausted intratumoral Vdelta2(-) gammadelta T cells in human kidney cancer retain effector function. Nat Immunol. 2023;24(4):612–24.
Wu P, Wu D, Ni C, Ye J, Chen W, Hu G, et al. gammadeltaT17 cells promote the accumulation and expansion of myeloid-derived suppressor cells in human colorectal cancer. Immunity. 2014;40(5):785–800.
Weimer P, Wellbrock J, Sturmheit T, Oliveira-Ferrer L, Ding Y, Menzel S, et al. Tissue-specific expression of TIGIT, PD-1, TIM-3, and CD39 by gammadelta T Cells in Ovarian Cancer. Cells. 2022;11(6):964.
Vinay DS, Ryan EP, Pawelec G, Talib WH, Stagg J, Elkord E, et al. Immune evasion in cancer: mechanistic basis and therapeutic strategies. Semin Cancer Biol. 2015;35(Suppl):S185–98.
Reina-Campos M, Moscat J, Diaz-Meco M. Metabolism shapes the tumor microenvironment. Curr Opin Cell Biol. 2017;48:47–53.
Dey P, Kimmelman AC, DePinho RA. Metabolic codependencies in the tumor microenvironment. Cancer Discov. 2021;11(5):1067–81.
Evans LA, Anderson EA, Jessen E, Nandakumar B, Atilgan E, Jevremovic D, et al. Overexpression of the energy metabolism transcriptome within clonal plasma cells is associated with the pathogenesis and outcomes of patients with multiple myeloma. Am J Hematol. 2022;97(7):895–902.
Lv J, Sun H, Gong L, Wei X, He Y, Yu Z, et al. Aberrant metabolic processes promote the immunosuppressive microenvironment in multiple myeloma. Front Immunol. 2022;13:1077768.
Sterner RC, Sterner RM. CAR-T cell therapy: current limitations and potential strategies. Blood Cancer J. 2021;11(4):69.
Gagelmann N, Riecken K, Wolschke C, Berger C, Ayuk FA, Fehse B, et al. Development of CAR-T cell therapies for multiple myeloma. Leukemia. 2020;34(9):2317–32.
Teoh PJ, Chng WJ. CAR T-cell therapy in multiple myeloma: more room for improvement. Blood Cancer J. 2021;11(4):84.
Majzner RG, Mackall CL. Tumor antigen escape from CAR T-cell therapy. Cancer Discov. 2018;8(10):1219–26.
Lin Q, Zhao J, Song Y, Liu D. Recent updates on CAR T clinical trials for multiple myeloma. Mol Cancer. 2019;18(1):154.
Van de Donk N, Usmani SZ, Yong K. CAR T-cell therapy for multiple myeloma: state of the art and prospects. Lancet Haematol. 2021;8(6):e446–61.
Bjorkstrom NK, Strunz B, Ljunggren HG. Natural killer cells in antiviral immunity. Nat Rev Immunol. 2022;22(2):112–23.
Shimasaki N, Jain A, Campana D. NK cells for cancer immunotherapy. Nat Rev Drug Discov. 2020;19(3):200–18.
Hamerman JA, Ogasawara K, Lanier LL. NK cells in innate immunity. Curr Opin Immunol. 2005;17(1):29–35.
Chiossone L, Dumas PY, Vienne M, Vivier E. Natural killer cells and other innate lymphoid cells in cancer. Nat Rev Immunol. 2018;18(11):671–88.
Wu SY, Fu T, Jiang YZ, Shao ZM. Natural killer cells in cancer biology and therapy. Mol Cancer. 2020;19(1):120.
Chan AC, Neeson P, Leeansyah E, Tainton K, Quach H, Prince HM, et al. Natural killer T cell defects in multiple myeloma and the impact of lenalidomide therapy. Clin Exp Immunol. 2014;175(1):49–58.
Venglar O, Bago JR, Motais B, Hajek R, Jelinek T. Natural killer cells in the malignant niche of multiple myeloma. Front Immunol. 2021;12: 816499.
Benson DM Jr, Bakan CE, Mishra A, Hofmeister CC, Efebera Y, Becknell B, et al. The PD-1/PD-L1 axis modulates the natural killer cell versus multiple myeloma effect: a therapeutic target for CT-011, a novel monoclonal anti-PD-1 antibody. Blood. 2010;116(13):2286–94.
Seymour F, Cavenagh JD, Mathews J, Gribben JG. NK cells CD56bright and CD56dim subset cytokine loss and exhaustion is associated with impaired survival in myeloma. Blood Adv. 2022;6(17):5152–9.
Chen C, Wang C, Pang R, Liu H, Yin W, Chen J, et al. Comprehensive single-cell transcriptomic and proteomic analysis reveals NK cell exhaustion and unique tumor cell evolutionary trajectory in non-keratinizing nasopharyngeal carcinoma. J Transl Med. 2023;21(1):278.
DeNardo DG, Ruffell B. Macrophages as regulators of tumour immunity and immunotherapy. Nat Rev Immunol. 2019;19(6):369–82.
Patysheva M, Frolova A, Larionova I, Afanas’ev S, Tarasova A, Cherdyntseva N, et al. Monocyte programming by cancer therapy. Front Immunol. 2022;13: 994319.
Sun J, Park C, Guenthner N, Gurley S, Zhang L, Lubben B, et al. Tumor-associated macrophages in multiple myeloma: advances in biology and therapy. J Immunother Cancer. 2022;10(4):e003975.
Panchabhai S, Kelemen K, Ahmann G, Sebastian S, Mantei J, Fonseca R. Tumor-associated macrophages and extracellular matrix metalloproteinase inducer in prognosis of multiple myeloma. Leukemia. 2016;30(4):951–4.
Leone P, Solimando AG, Malerba E, Fasano R, Buonavoglia A, Pappagallo F, et al. Actors on the scene: immune cells in the myeloma niche. Front Oncol. 2020;10: 599098.
Beyar-Katz O, Magidey K, Reiner-Benaim A, Barak N, Avivi I, Cohen Y, et al. Proinflammatory macrophages promote multiple myeloma resistance to bortezomib therapy. Mol Cancer Res. 2019;17(11):2331–40.
Zheng Y, Cai Z, Wang S, Zhang X, Qian J, Hong S, et al. Macrophages are an abundant component of myeloma microenvironment and protect myeloma cells from chemotherapy drug-induced apoptosis. Blood. 2009;114(17):3625–8.
Sacco A, Battaglia AM, Botta C, Aversa I, Mancuso S, Costanzo F, et al. Iron metabolism in the tumor microenvironment-implications for anti-cancer immune response. Cells. 2021;10(2):303.
Oshi M, Tokumaru Y, Asaoka M, Yan L, Satyananda V, Matsuyama R, et al. M1 Macrophage and M1/M2 ratio defined by transcriptomic signatures resemble only part of their conventional clinical characteristics in breast cancer. Sci Rep. 2020;10(1):16554.
Wu K, Lin K, Li X, Yuan X, Xu P, Ni P, et al. Redefining tumor-associated macrophage subpopulations and functions in the tumor microenvironment. Front Immunol. 2020;11:1731.
Damasceno D, Almeida J, Teodosio C, Sanoja-Flores L, Mayado A, Perez-Pons A, et al. Monocyte subsets and serum inflammatory and bone-associated markers in monoclonal gammopathy of undetermined significance and multiple myeloma. Cancers (Basel). 2021;13(6):1454.
Andersen MN, Andersen NF, Rodgaard-Hansen S, Hokland M, Abildgaard N, Moller HJ. The novel biomarker of alternative macrophage activation, soluble mannose receptor (sMR/sCD206): Implications in multiple myeloma. Leuk Res. 2015;39(9):971–5.
Banchereau J, Steinman RM. Dendritic cells and the control of immunity. Nature. 1998;392(6673):245–52.
Verheye E, Bravo Melgar J, Deschoemaeker S, Raes G, Maes A, De Bruyne E, et al. Dendritic Cell-Based Immunotherapy in Multiple Myeloma: Challenges, Opportunities, and Future Directions. Int J Mol Sci. 2022;23(2).
Ratta M, Fagnoni F, Curti A, Vescovini R, Sansoni P, Oliviero B, et al. Dendritic cells are functionally defective in multiple myeloma: the role of interleukin-6. Blood. 2002;100(1):230–7.
Brimnes MK, Svane IM, Johnsen HE. Impaired functionality and phenotypic profile of dendritic cells from patients with multiple myeloma. Clin Exp Immunol. 2006;144(1):76–84.
Reizis B. Plasmacytoid dendritic cells: development, regulation, and function. Immunity. 2019;50(1):37–50.
Chauhan D, Singh AV, Brahmandam M, Carrasco R, Bandi M, Hideshima T, et al. Functional interaction of plasmacytoid dendritic cells with multiple myeloma cells: a therapeutic target. Cancer Cell. 2009;16(4):309–23.
Reagan MR, Ghobrial IM. Multiple myeloma mesenchymal stem cells: characterization, origin, and tumor-promoting effects. Clin Cancer Res. 2012;18(2):342–9.
Vande Broek I, Asosingh K, Vanderkerken K, Straetmans N, Van Camp B, Van Riet I. Chemokine receptor CCR2 is expressed by human multiple myeloma cells and mediates migration to bone marrow stromal cell-produced monocyte chemotactic proteins MCP-1, -2 and -3. Br J Cancer. 2003;88(6):855–62.
Lemaitre L, DoSouto Ferreira L, Joubert MV, Avet-Loiseau H, Martinet L, Corre J, et al. Imprinting of mesenchymal stromal cell transcriptome persists even after treatment in patients with multiple myeloma. Int J Mol Sci. 2020;21(11):3854.
Young MD, Mitchell TJ, Vieira Braga FA, Tran MGB, Stewart BJ, Ferdinand JR, et al. Single-cell transcriptomes from human kidneys reveal the cellular identity of renal tumors. Science. 2018;361(6402):594–9.
Patel AP, Tirosh I, Trombetta JJ, Shalek AK, Gillespie SM, Wakimoto H, et al. Single-cell RNA-seq highlights intratumoral heterogeneity in primary glioblastoma. Science. 2014;344(6190):1396–401.
Zhou L, Yu KH, Wong TL, Zhang Z, Chan CH, Loong JH, et al. Lineage tracing and single-cell analysis reveal proliferative Prom1+ tumour-propagating cells and their dynamic cellular transition during liver cancer progression. Gut. 2022;71(8):1656–68.
Praktiknjo SD, Obermayer B, Zhu Q, Fang L, Liu H, Quinn H, et al. Tracing tumorigenesis in a solid tumor model at single-cell resolution. Nat Commun. 2020;11(1):991.
Xu K, Wang R, Xie H, Hu L, Wang C, Xu J, et al. Single-cell RNA sequencing reveals cell heterogeneity and transcriptome profile of breast cancer lymph node metastasis. Oncogenesis. 2021;10(10):66.
Savage P, Blanchet-Cohen A, Revil T, Badescu D, Saleh SMI, Wang YC, et al. A targetable EGFR-dependent tumor-initiating program in breast cancer. Cell Rep. 2017;21(5):1140–9.
Zheng H, Pomyen Y, Hernandez MO, Li C, Livak F, Tang W, et al. Single-cell analysis reveals cancer stem cell heterogeneity in hepatocellular carcinoma. Hepatology. 2018;68(1):127–40.
Berdeja JG, Madduri D, Usmani SZ, Jakubowiak A, Agha M, Cohen AD, et al. Ciltacabtagene autoleucel, a B-cell maturation antigen-directed chimeric antigen receptor T-cell therapy in patients with relapsed or refractory multiple myeloma (CARTITUDE-1): a phase 1b/2 open-label study. Lancet. 2021;398(10297):314–24.
Wu R, Guo W, Qiu X, Wang S, Sui C, Lian Q, et al. Comprehensive analysis of spatial architecture in primary liver cancer. Sci Adv. 2021;7(51):eabg3750.
Lewis SM, Asselin-Labat ML, Nguyen Q, Berthelet J, Tan X, Wimmer VC, et al. Spatial omics and multiplexed imaging to explore cancer biology. Nat Methods. 2021;18(9):997–1012.
Berglund E, Maaskola J, Schultz N, Friedrich S, Marklund M, Bergenstrahle J, et al. Spatial maps of prostate cancer transcriptomes reveal an unexplored landscape of heterogeneity. Nat Commun. 2018;9(1):2419.
Ji AL, Rubin AJ, Thrane K, Jiang S, Reynolds DL, Meyers RM, et al. Multimodal analysis of composition and spatial architecture in human squamous cell carcinoma. Cell. 2020;182(6):1661–2.
Demaree B, Delley CL, Vasudevan HN, Peretz CAC, Ruff D, Smith CC, et al. Joint profiling of DNA and proteins in single cells to dissect genotype-phenotype associations in leukemia. Nat Commun. 2021;12(1):1583.
Shahi P, Kim SC, Haliburton JR, Gartner ZJ, Abate AR. Abseq: Ultrahigh-throughput single cell protein profiling with droplet microfluidic barcoding. Sci Rep. 2017;7:44447.
Anderson GSF, Ballester-Beltran J, Giotopoulos G, Guerrero JA, Surget S, Williamson JC, et al. Unbiased cell surface proteomics identifies SEMA4A as an effective immunotherapy target for myeloma. Blood. 2022;139(16):2471–82.
Ferguson ID, Patino-Escobar B, Tuomivaara ST, Lin YT, Nix MA, Leung KK, et al. The surfaceome of multiple myeloma cells suggests potential immunotherapeutic strategies and protein markers of drug resistance. Nat Commun. 2022;13(1):4121.
Baughn LB, Sachs Z, Noble-Orcutt KE, Mitra A, Van Ness BG, Linden MA. Phenotypic and functional characterization of a bortezomib-resistant multiple myeloma cell line by flow and mass cytometry. Leuk Lymphoma. 2017;58(8):1931–40.
Smets T, Stevenaert F, Adams HC 3rd, Vanhoof G. Deep profiling of the immune system of multiple myeloma patients using cytometry by time-of-flight (CyTOF). Methods Mol Biol. 2018;1792:47–54.
Visram A, Dasari S, Anderson E, Kumar S, Kourelis TV. Relapsed multiple myeloma demonstrates distinct patterns of immune microenvironment and malignant cell-mediated immunosuppression. Blood Cancer J. 2021;11(3):45.
Stribbling SM, Ryan AJ. The cell-line-derived subcutaneous tumor model in preclinical cancer research. Nat Protoc. 2022;17(9):2108–28.
Chuprin J, Buettner H, Seedhom MO, Greiner DL, Keck JG, Ishikawa F, et al. Humanized mouse models for immuno-oncology research. Nat Rev Clin Oncol. 2023;20(3):192–206.
Stewart CA, Gay CM, Xi Y, Sivajothi S, Sivakamasundari V, Fujimoto J, et al. Single-cell analyses reveal increased intratumoral heterogeneity after the onset of therapy resistance in small-cell lung cancer. Nat Cancer. 2020;1:423–36.
Marjanovic ND, Hofree M, Chan JE, Canner D, Wu K, Trakala M, et al. Emergence of a high-plasticity cell state during lung cancer evolution. Cancer Cell. 2020;38(2):229-46 e13.
Krieger TG, Le Blanc S, Jabs J, Ten FW, Ishaque N, Jechow K, et al. Single-cell analysis of patient-derived PDAC organoids reveals cell state heterogeneity and a conserved developmental hierarchy. Nat Commun. 2021;12(1):5826.
Van de Sande B, Lee JS, Mutasa-Gottgens E, Naughton B, Bacon W, Manning J, et al. Applications of single-cell RNA sequencing in drug discovery and development. Nat Rev Drug Discov. 2023.
Larrayoz M, Garcia-Barchino MJ, Celay J, Etxebeste A, Jimenez M, Perez C, et al. Preclinical models for prediction of immunotherapy outcomes and immune evasion mechanisms in genetically heterogeneous multiple myeloma. Nat Med. 2023;29(3):632–45.
Robinson T, Bowman RL, Persaud S, Liu Y, Gao Q, Zhang J-P, et al. Single cell genotypic and phenotypic analysis of measurable residual disease in acute myeloid leukemia. Blood. 2022;140(Supplement 1):2275–7.
Soekojo CY, Chung TH, Furqan MS, Chng WJ. Genomic characterization of functional high-risk multiple myeloma patients. Blood Cancer J. 2022;12(1):24.
Sklavenitis-Pistofidis R, Getz G, Ghobrial I. Single-cell RNA sequencing: one step closer to the clinic. Nat Med. 2021;27(3):375–6.
Bouland GA, Mahfouz A, Reinders MJT. Consequences and opportunities arising due to sparser single-cell RNA-seq datasets. Genome Biol. 2023;24(1):86.
Wang Y, Navin NE. Advances and applications of single-cell sequencing technologies. Mol Cell. 2015;58(4):598–609.
Acknowledgements
Not applicable.
Funding
The work was supported by the National Natural Science Foundation of China (grant numbers: 81974006, 82000213); Shanghai Shenkang Hospital Development Center Funding (grant number: SHDC2020CR2070B); and the Interdisciplinary Program of Shanghai Jiao Tong University (project number: ZH2018ZDB02).
Author information
Ethics declarations
Ethics approval and consent to participate
Not applicable.
Consent for publication
Not applicable.
Competing interests
The authors declare that they have no competing interests.
Additional information
Publisher’s Note
Springer Nature remains neutral with regard to jurisdictional claims in published maps and institutional affiliations.
Rights and permissions
Open Access This article is licensed under a Creative Commons Attribution 4.0 International License, which permits use, sharing, adaptation, distribution and reproduction in any medium or format, as long as you give appropriate credit to the original author(s) and the source, provide a link to the Creative Commons licence, and indicate if changes were made. The images or other third party material in this article are included in the article's Creative Commons licence, unless indicated otherwise in a credit line to the material. If material is not included in the article's Creative Commons licence and your intended use is not permitted by statutory regulation or exceeds the permitted use, you will need to obtain permission directly from the copyright holder. To view a copy of this licence, visit http://creativecommons.org/licenses/by/4.0/. The Creative Commons Public Domain Dedication waiver (http://creativecommons.org/publicdomain/zero/1.0/) applies to the data made available in this article, unless otherwise stated in a credit line to the data.
About this article
Cite this article
Chen, M., Jiang, J. & Hou, J. Single-cell technologies in multiple myeloma: new insights into disease pathogenesis and translational implications. Biomark Res 11, 55 (2023). https://doi.org/10.1186/s40364-023-00502-8
Received:
Accepted:
Published:
DOI: https://doi.org/10.1186/s40364-023-00502-8