Abstract
In this study, we develop a novel moving average forecasting approach based on fuzzy time series data set. The main objective of applying this moving average approach in develop method is to provide better results and enhance the accuracy in forecasted output by reducing the fluctuation in time series data set. The developed method is to define the universe of discourse and partition into equal length of intervals which is based on the average-length method. Further, triangular fuzzy sets are defined and obtain a membership grade of each moving average historical datum rather than actual datum of historical fuzzy time series data. Here, the fuzzification process of moving average historical data to their maximum membership grades obtained into corresponding triangular fuzzy sets. The general suitability of developed model has been examined by implementing in the forecast of student enrollments data at the University of Alabama. Further, the market price of State Bank of India share at Bombay Stock Exchange, India, has also been implemented in the forecast. The developed method of moving average fuzzy time series provides an improved forecasted output with least root mean square error and average forecasting errors which shows that our developed method is more superior than other existing models available in the literature based on fuzzy time series data.



Similar content being viewed by others
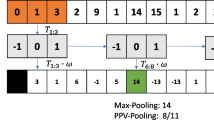
References
Abhishekh, Bharati, S. K., & Singh, S. R. (2019). A novel approach to handle forecasting problems based on moving average two-factor fuzzy time series. In J. C. Bansal, K. N. Das, A. Nagar, K. Deep, & A. K. Ojha Soft computing for problem solving; Advances in intelligent systems and computing (Vol. 816). Singapore: Springer.
Abhishekh, Gautam, S. S., & Singh, S. R. (2017). A refined weighted for forecasting based on type 2 fuzzy time series. International Journal of Modelling and Simulation, 38, 180–188.
Abhishekh, Gautam, S. S., & Singh, S. R. (2018a). A score function based method of forecasting using intuitionistic fuzzy time series. New Mathematics and Natural Computation, 14(1), 91–111.
Abhishekh, Gautam, S. S., & Singh, S. R. (2018b). A refined method of forecasting based on high-order intuitionistic fuzzy time series data. Progress in Artificial Intelligence, 7(4), 339–350.
Abhishekh, & Kumar, S. (2017). A computational method for rice production forecasting based on high-order fuzzy time series. International Journal of Fuzzy Mathematical Archive, 13(2), 145–157.
Aladag, C. H., Basaran, M. A., Egrioglu, E., Yolcu, U., & Uslu, V. R. (2009). Forecasting in high order fuzzy time series by using neural networks to define fuzzy relations. Expert Systems with Applications, 36, 4228–4231.
Aladag, C. H., Yolcu, U., Egrioglu, E., & Dalar, A. Z. (2012). A new time invariant fuzzy time series forecasting method based on particle swarm optimization. Applied Soft Computing, 12(10), 3291–3299.
Bisht, K., & Kumar, S. (2016). Fuzzy time series forecasting method based on hesitant fuzzy sets. Expert Systems with Applications, 64, 557–568.
Chang, X. H., Li, Z. M., & Park, J. H. (2017a). Fuzzy generalized H2 filtering for nonlinear discrete-time systems with measurements quantization. IEEE Transactions on Systems, Man, and Cybernetics: Systems, 99, 1–12.
Chang, X. H., Park, J. H., & Shi, P. (2017b). Fuzzy resilient energy-to-peak filtering for continuous-time nonlinear systems. IEEE Transactions on Fuzzy Systems, 25(6), 1576–1588.
Chang, X. H., & Wang, Y. M. (2018). Peak to peak filtering for networked nonlinear DC motor systems with quantization. IEEE Transactions on Industrial Informatics, 14(12), 5378–5388.
Chen, S. M. (1996). Forecasting enrollments based on fuzzy time series. Fuzzy Sets and Systems, 81(3), 311–319.
Chen, S. M. (2002). Forecasting enrollments based on high-order fuzzy time series. Cybernetics and Systems, 33(1), 1–16.
Chen, S. M., & Chung, N. Y. (2006). Forecasting enrollments using high-order fuzzy time series and genetic algorithms. International Journal of Intelligent Systems, 21(5), 485–501.
Chen, S. M., & Hsu, C. C. (2004). A new method to forecast enrollments using fuzzy time series. International Journal of Applied Science and Engineering, 2(3), 234–244.
Chen, S. M., & Kao, P. Y. (2013). TAIEX forecasting based on fuzzy time series, particle swarm optimization techniques and support vector machines. Information Sciences, 247, 62–71.
Chen, S. M., & Tanuwijaya, K. (2011). Fuzzy forecasting based on high-order fuzzy logical relationships and automatic clustering techniques. Expert Systems with Applications, 38, 15425–15437.
Chen, S. M., Wang, N. Y., & Pan, J. S. (2009). Forecasting enrollments using automatic clustering techniques and fuzzy logical relationships. Expert Systems with Applications, 36(8), 11070–11076.
Eǧrioǧlu, E. (2012). A new time-invariant fuzzy time series forecasting method based on genetic algorithm. Advances in Fuzzy Systems, 2012, 785709. https://doi.org/10.1155/2012/785709.
Fraccaroli, F., Peruffo, A., & Zorzi, M. A. (2015). A new recursive least-squares method with multiple forgetting schemes. In: 2015 54th IEEE conference on decision and control (CDC) (pp. 3367–3372).
Gangwar, S. S., & Kumar, S. (2012). Partitions based computational method for high-order fuzzy time series forecasting. Expert Systems with Applications, 39(15), 12158–12164.
Gangwar, S. S., & Kumar, S. (2014). Probabilistic and intuitionistic fuzzy sets-based method for fuzzy time series forecasting. Cybernetics and Systems, 45(4), 349–361.
Gangwar, S. S., & Kumar, S. (2015). Computational method for high-order weighted fuzzy time series forecasting based on multiple partitions. In M. Chakraborty, A. Skowron, M. Maiti, & S. Kar (Eds.), Facets of uncertainties and applications (pp. 293–302). New Delhi: Springer.
Gautam, S. S., Abhishekh, & Singh, S. R. (2018a). An improved-based TOPSIS method in interval valued intuitionistic fuzzy environment. Life Cycle Reliability and Safety Engineering, 7, 81–88.
Gautam, S. S., Abhishekh, & Singh, S. R. (2018b). An intuitionistic fuzzy soft set theoretic approach to decisions making problems. MATEMATIKA, 34, 49–58.
Gautam, S. S., Abhishekh, & Singh, S. R. (2018c). A new high-order approach for forecasting fuzzy time series data. International Journal of Computational Intelligence and Applications, 17, 1850019.
Huarng, K. (2001). Effective lengths of intervals to improve forecasting in fuzzy time series. Fuzzy Sets and Systems, 123(3), 387–394.
Huarng, K., & Yu, T. H. (2006). Ratio-based lengths of intervals to improve fuzzy time series forecasting. IEEE Transactions on Systems, Man, and Cybernetics, Part B (Cybernetics), 36(2), 328–340.
Huo, X., Ma, L., Zhao, X., & Zong, G. (2019). Observer-based fuzzy adaptive stabilization of uncertain switched stochastic nonlinear systems with input quantization. Journal of the Franklin Institute, 356, 1789–1809.
Hwang, J. R., Chen, S. M., & Lee, C. H. (1998). Handling forecasting problems using fuzzy time series. Fuzzy Sets and Systems, 100(1–3), 217–228.
Jilani, T. A., & Burney, S. M. (2008). Multivariate stochastic fuzzy forecasting models. Expert Systems with Applications, 35(3), 691–700.
Lee, H. S., & Chou, M. T. (2004). Fuzzy forecasting based on fuzzy time series. International Journal of Computer Mathematics, 81(7), 781–789.
Lee, L. W., Wang, L. H., Chen, S. M., & Leu, Y. H. (2006). Handling forecasting problems based on two-factors high-order fuzzy time series. IEEE Transactions on Fuzzy Systems, 14(3), 468–477.
Li, S. T., & Cheng, Y. C. (2007). Deterministic fuzzy time series model for forecasting enrollments. Computers & Mathematics with Applications, 53(12), 1904–1920.
Pathak, H. K., & Singh, P. (2011). A new bandwidth interval based forecasting method for enrollments using fuzzy time series. Applied Mathematics, 2(04), 504.
Qiu, W., Liu, X., & Li, H. (2011). A generalized method for forecasting based on fuzzy time series. Expert Systems with Applications, 38(8), 10446–10453.
Singh, S. R. (2007a). A simple method of forecasting based on fuzzy time series. Applied Mathematics and Computation, 186(1), 330–339.
Singh, S. R. (2007b). A robust method of forecasting based on fuzzy time series. Applied Mathematics and Computation, 188(1), 472–484.
Song, Q. (2003). A note on fuzzy time series model selection with sample autocorrelation functions. Cybernetics & Systems, 34(2), 93–107.
Song, Q., & Chissom, B. S. (1993). Forecasting enrollments with fuzzy time series—Part I. Fuzzy Sets and Systems, 54(1), 1–9.
Song, Q., & Chissom, B. S. (1994). Forecasting enrollments with fuzzy time series—Part II. Fuzzy Sets and Systems, 62(1), 1–8.
Wang, N. Y., & Chen, S. M. (2009). Temperature prediction and TAIFEX forecasting based on automatic clustering techniques and two-factors high-order fuzzy time series. Expert Systems with Applications, 36(2), 2143–2154.
Wang, Y., Lei, Y., Fan, X., & Wang, Y. (2016). Intuitionistic fuzzy time series forecasting model based on intuitionistic fuzzy reasoning. Mathematical Problems in Engineering, 2016, 5035160. https://doi.org/10.1155/2016/5035160.
Wong, W. K., Bai, E., & Chu, A. W. (2010). Adaptive time-variant models for fuzzy time series forecasting. IEEE Transactions on Systems, Man, and Cybernetics, Part B (Cybernetics), 40(6), 1531–1542.
Yolcu, U., Egrioglu, E., Uslu, V. R., Basaran, M. A., & Aladag, C. H. (2009). A new approach for determining the length of intervals for fuzzy time series. Applied Soft Computing, 9(2), 647–651.
Yu, H. K. (2005). A refined fuzzy time-series model for forecasting. Physica A: Statistical Mechanics and Its Applications, 346(3), 657–681.
Zadeh, L. A. (1965). Fuzzy sets. Information and Control, 8(3), 338–353.
Zhao, X., Shi, P., & Zheng, X. (2016). Fuzzy adaptive control design and discretization for a class of nonlinear uncertain systems. IEEE Transactions on Cybernetics, 46(6), 1476–1483.
Acknowledgements
The authors are very thankful to the editor and the anonymous reviewers for their constructive suggestions and comments to enhance the quality of the study.
Author information
Authors and Affiliations
Corresponding author
Additional information
Publisher’s Note
Springer Nature remains neutral with regard to jurisdictional claims in published maps and institutional affiliations.
Rights and permissions
About this article
Cite this article
Gautam, S.S., Abhishekh A Novel Moving Average Forecasting Approach Using Fuzzy Time Series Data Set. J Control Autom Electr Syst 30, 532–544 (2019). https://doi.org/10.1007/s40313-019-00467-w
Received:
Revised:
Accepted:
Published:
Issue Date:
DOI: https://doi.org/10.1007/s40313-019-00467-w