Abstract
In this paper, the required models and methods to analyze and quantify the potential demand for urban air mobility (UAM) complementing public transport and possible impacts were defined and applied to the Munich Metropolitan region. An existing agent-based transport model of the study area were used and extended to cover socio-demographic changes up to the year 2030 and intermodal UAM services. An incremental logit model for UAM was derived to simulate demand for this new mode. An airport access model was developed as well. Three different UAM networks with different numbers of vertiports were defined. Sensitivity studies of ticket fare and structure, flying vehicle cruise speed, passenger process times at vertiports and different Urban Air Mobility networks sizes were performed. For the reference case, UAM accounts for a modal share of 0.5%. The absolute UAM demand is concentrated on very short routes; hence, UAM vehicle flight speed variation shows low UAM demand impacts. Kilometer-based fare, number of UAM vehicles per vertiport and passenger process times at vertiports show a significant impact on UAM demand.
Similar content being viewed by others
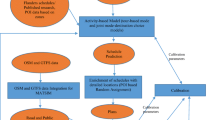
Avoid common mistakes on your manuscript.
1 Introduction
Due to the current developments in the field of Urban Air Mobility (UAM) and the Initiative European Innovation Platform Smart Cities and Communities (EIP-SCC), the city of Ingolstadt and the Munich metropolitan region have been among the model cities that explore Urban Air Mobility as a complement to existing transport systems. Despite the short-term support of local UAM manufacturers and the provision of airspace and ground infrastructure to test vehicle operations and navigation technologies, the long-term application potential for this region is still unclear. The Munich metropolitan area is of interest to UAM applications as Munich is Germany´s most congested metropolitan area today; seven out of the top ten municipalities with the highest buying power in Germany are located in this region and Bavaria is the most visited federal state in Germany with more than 19 million foreign tourists per year. This paper will, therefore, provide consolidated and integrated project results by investigating the long-term application potential of Urban Air Mobility as a complement to local public transport for the Munich metropolitan area.
1.1 Research questions and methodological approach
To be able to quantify the long-term application potential of urban air mobility to complement public transport for the selected study area of Munich metropolitan region, various methodological approaches from different partners have been applied and combined:
-
1.
Methods to quantify current and future mobility demand on household and detailed origin–destination levels for a respective, relevant study area
-
2.
Development of a mode-choice model for urban air mobility for both commuters and other potential users (e.g. airport passengers) which includes all other relevant alternative modes of transport
-
3.
Approach to precisely define vertiport locations to capture all positive and negative effects of integration with other modes of transport
-
4.
Development of an operational concept including first operating cost estimation to be able to define a consistent simulation plan
-
5.
Development of an assessment framework to be able to evaluate potential impacts of urban air mobility operations regarding transport-business related, socio-economic and environmental changes
1.2 Study area
The study area is polycentric and is composed of five core cities (Munich, Augsburg, Ingolstadt, Landshut and Rosenheim) and their commuting catchment areas. Catchment areas were defined as municipalities with at least 25% commute to any of the five core cities. The studied area includes a population of 4.5 million distributed among 2.1 million households. A total of 444 municipalities form the Greater Munich Metropolitan area (Fig. 1).
Greater Munich Metropolitan Area as study area [1]
After delineating the study area, municipalities are divided in Traffic Analysis Zones (TAZs) using a gradual raster-based zone system [1]. The method creates raster cell zones that are smaller in denser areas and larger in rural areas. Thereby, more zonal detail is given in areas where most travel is happening. The total number of TAZs is 4950.
2 Simulation model
2.1 Overview
Based on the Microcensus data and control totals at the municipality and county levels, a synthetic population was generated for the Munich Metropolitan area [2]. The travel demand was modeled by the agent-based and trip-based model MITO, or Microscopic Transportation Orchestrator [3].
The model was applied to generate transport demand to be expected around the year 2030. Accordingly, a synthetic population for this year was generated using the land-use model SILO (Simple Land Use Orchestrator) [4]. SILO simulates the changes in the synthetic population from 2011 to 2030 (e.g. demography, real estate, and household relocation). SILO inputs include population and employment control totals for the entire study area for 2030 [5]. A flowchart of this model is shown in Fig. 2. First, the number of trips was generated for each household. These trips were segmented as mandatory work and education trips, for which the work or school place was already defined by the synthetic population, and discretionary trips, for which the travel time budget is calculated for each household.
MITO-MATSim flowchart [3]
Destinations were selected by a multinominal logit model that takes the remaining travel time budget into account. Logit models consider a utility factor, e.g. a certain quantified value of an alternative to estimate the probability of selecting that alternative. The utility factor takes into account both individual and alternative characteristics [6]. A nested logit model is used for mode choice, where private and public transport modes were grouped in individual nests. The time-of-day choice model selected a preferred arrival time for trips and calculated the trip departure time based on the expected travel time by the selected mode. The trip assignment was simulated in the multi-agent travel-based model, MATSim [7].
2.2 Airport access
The model MITO, as described above, does not include the travel demand to the airport. Given the importance of the airport located in the study area (Munich international airport), and the potential of UAM for trips that connect the airport with the rest of the study area, MITO was improved to add this demand [8]. The airport access model was developed using counts of passengers by mode and postal code of origins that arrived at the airport by ground transportation. These data were obtained from an annual survey carried out by the Munich Airport (Flughafen München Gesellschaft-FMG).
The model distributes trips to the airport among the zones of the study area based on population and employment density, and on the level of service to access the airport (transport supply). The model also adds inbound trips from the airport to the end destination of passengers. The number of simulated trips matched the forecasts of FMG. For each trip, a mode was selected based on its generalized costs using a multinomial logit choice model. Individual departure times were selected to replicate the distribution of take-offs and landings over the course of the day.
2.3 UAM stated preference survey and mode choice model development
Aiming at gaining insights into the travel behavior impacts of autonomous transportation modes, especially UAM, Fu et al. [9] conducted a preliminary research by deriving measures for transportation service attributes and identifying characteristics of potential users who might adopt autonomous transportation services, particularly the services of UAM. A stated preference questionnaire was designed and the responses were randomly collected in Munich metropolitan region and further selected through adequately calculated weights and expansion factors based on ages and employment status. Based on appropriately represented demographics, a multinomial logit mode choice model was developed including four transport alternatives: private car, public transportation (PT), autonomous taxis, and autonomous flying taxis (as an example of UAM service). The results suggest that travel time, travel cost, and safety may be critical determinants in adopting autonomous transportation modes. The potential travelers indicate a high willingness to pay for using autonomous transportation modes, especially the service of UAM. Among different market segments, younger individuals, as well as high-income households, are more likely to accept UAM [9].
The potential mode share of UAM was simulated using both an incremental logit model [10] and a stated preference survey from Fu et al. as described above. The principle of an incremental logit model is to select a reference mode for UAM and to define the changes in utility provided by the new mode. In this research, the incremental approach is built on the existing mode choice model that is part of MITO. Given the similarity in terms of un-included attributes of UAM and other existing modes, UAM was located in the transit nest (Fig. 3) and train was selected as the reference mode. It could be argued that UAM resembles a public transport mode in the mobility system; similar to the mode train, it is designed to travel fast and to serve as a station-to-station travel option.
To define the changes in utilities for UAM, the results from the stated preference survey of Fu et al. were used. As no observed data were available, travel time, travel cost, and the value of time were assumed to be the only different variables between train and UAM. All other socio-demographic variables used in the mode choice model remained unchanged for UAM. The results of the stated preference survey presented a lower weight for the costs of UAM compared to the costs for train, while the travel time for UAM was found to be more sensitive than that for train. Based on the survey results, the UAM cost coefficient were modified by a factor of 0.454 and the time coefficient by a factor of 1.475.
2.4 Traffic assignment in MATSim and UAM extension
As previously mentioned, the results of an initial travel demand generation via MITO was fed into the agent-based transport simulation MATSim which has been extended to enable the simultaneous simulation of ground- and aerial-based transportation modes [11,12,13]. Additionally to ground-based transportation (e.g. car, public transport, walking, and cycling), aerial on-demand mobility can be specified by providing a number of UAM stations and their locations, UAM vehicles with speeds and capacities, and an aerial flight network—which is connected to the ground-based networks via stations links, as depicted in Fig. 4.
Illustration of the UAM network encoding in MATSim [11]
For the integration of UAM-enabled MATSim with MITO, changes and feature additions have been made to the UAM MATSim extension. First, an application program interface (API) has been set up for MITO to control UAM MATSim directly and be able to integrate its usage within the MITO loop (see Fig. 5). Furthermore, utility functions have been added to facilitate handling of UAM station data, as this information must be transferable between MITO and UAM MATSim. Lastly, an additional UAM routing strategy has been created to ensure that the chosen UAM route, which consists of an access mode, a departure/origin station, an arrival/destination station, and an egress mode, are consistent between MITO and the simulated traffic in MATSim. Using this predefined strategy, rather than for instance a total travel time minimizing strategy, was expected to reduce computation time since UAM routes from MITO would not be recalculated based on MATSim’s more granular network—trading runtime decrease against model simplifications.
Simulating UAM via the UAM extension for MATSim would allow detailed analyses of UAM operations and the creation of a feedback loop to enable agents to refine their mode choices, which incorporate UAM’s operational properties such as vehicle speed and availability, as well as infrastructure accessibility and capacity.
The mode choice model generated demand for UAM based on utilities that reflect price, travel time and other attributes of the UAM service which are further discussed by Pukhova et al. [14]. It is perceivable that the mode choice model generates more demand for UAM than the available fleet of UAM vehicles could handle. Particularly in scenarios where the price is relatively low, it was expected that substantially more travelers would select UAM in the mode choice model than could be handled by the UAM system. In contrast to transit, where capacity constraints are softer constraints, each UAM vehicle has a strict capacity limit, and each vertiport has a strict limit of how many UAM vehicles can be processed throughout the day. Figure 5 shows this feedback loop that has been implemented to avoid very long waiting times for a UAM ride.
After the first assignment was simulated, agents choosing UAM evaluated the waiting time for the UAM boarding. The new individual waiting time was fed into MITO’s mode choice model, making UAM less attractive than in MITO’s initial travel demand. This reduced the number of passengers waiting for UAM, though some passengers decided to wait despite long waiting times, because the alternative modes were substantially less attractive.
3 Simulation plan
3.1 UAM network architypes
One essential part for the modeling and assessment of UAM is the development of a vertiport network and related routes along which the UAM vehicles fly. The routes might not be able to follow the beeline between two vertiports due to safety, privacy, and noise concerns. One approach is to have routes that follow train tracks or major roads to minimize additional perceived noise, minimize risk in case of UAM malfunctions and to minimize visual impacts.
To identify potential vertiport locations within the study area, four workshops with representatives from Munich Airport, Chamber of Industry and Commerce of Upper Bavaria and the Cities of Munich and Ingolstadt have been conducted. This helped to gain an understanding of the need for vertiport infrastructure at the different locations; all workshops started by identifying potential trip purposes relevant for UAM. Besides commuting and business trips, the experts emphasized the market potential for tourism and leisure trips. Additionally, enabling accessibility to badly connected and rural regions was highlighted. The experts were then asked to identify relevant locations for each of the mentioned purposes.
Yet, to create a business case for UAM, networks cannot be separated by trip purpose but rather need to capture all demand within one network (Fig. 6). Therefore, possible vertiport locations based on these four trip purposes have been merged to form a high-density network that covers all relevant trip purposes and thus aims at capturing demand stemming from various target groups. This network includes 130 vertiports within the study area and enables connections between rural regions and cities as well as within cities. Assuming costs per vertiport are high, as indicated before, this might not be profitable and smaller and less dense networks might have advantages. To assess this, two additional networks have been developed. The low-density network included 24 vertiports and covered larger agglomerations, employment centers, transportation hubs and densely populated areas with a large share of high incomes. The medium density network included all vertiports presented in the low-density network and increased coverage by adding the terminus of main subway lines and suburban trains as well as all major employment centers in the study area.
Low (left), medium (middle) and high-density networks (right) for the study area with 24, 74 and 130 vertiports respectively [28]
As shown in Fig. 7, the number of possible UAM routes significantly increases with number of vertiports. Especially, for the high-density network, a large number of possible UAM routes can be seen for distances lower than 20 km as well as between 70 and 90 km.
3.2 Infrastructure requirements
For the construction of inner city vertiports, regulations and basic requirements for the construction and operation of aerodromes must be complied with and considered. As there are no explicit regulations for vertiports yet, the requirements of heliports were used for the basic requirements of vertiports and would be supplemented [15]. Basic requirements stemmed from § 6 Luftverkehrsgesetz (LuftVG) and § 44 et seq. Luftverkehrs-Zulassungs-Ordnung (LuftVZO). The facilities must be designed in accordance with the specifications of the UAVs. In addition, the provisions of Annex 14 Vol II—Airports—of the ICAO Convention are to be applied, insofar as this is not contrary to German law. In the following, the different areas of necessary infrastructure are described.
Communication facilities These include active data networks (LAN/WLAN/WAN). Local offices of the German Air traffic control, meteorological service, rescue service and fire brigade need to guarantee their availability at any time.
Statics and construction Statics and construction play a central role in structural design. In case the vertiports are placed on the rooftop of buildings, various loads would have an effect on them. Additionally, the weight of the structure itself, traffic-, wind- and snow loads, water pressure and gravity, vehicle weight, temperature and dynamic loads (shocks, vibrations, and oscillations) are considered. As part of public transport, the facility should be barrier free and the size of the vertiport must be demand oriented to the customers’ needs. The commercial areas can be used for additional service like gastronomy or parcel service stations.
With regards to the construction, it is important to note that the electric installations have to be integrated into the platform and to be protected from environmental hazards. Devices for fire and noise protection and charging stations must be available for professionals like firefighter and technical staff who need to work with it and can be adapted to the design of the vertiport. Areas for parking of eVTOLs and maintenance should be provided [16]. A further consideration for future development can be to design the platform to be easily expandable and upward compatible, demountable and reusable for sustainability purposes.
Vertiport placement Depending on local conditions, vertiports can be placed at different locations in the urban area. Thus, multi-storey car parks, company roofs or free floor areas can be used. A seamless public transport connection or addition is crucial to ensure time and comfort disutilities perceived by future users.
Vertiport space requirements Current vertiport designs follow basic design principles and regulations (e.g. ICAO Annex 14, Volume 2) of heliports. The required space for vertiports consists of landing pads, taxiways, parking gates to service the vehicle and space for passenger processes. Depending on the level of service and recharging strategy, additional space for vehicle maintenance or battery recharging has to be considered. The required dimension of a vertiport depends on a large number of parameters. If similar legal conditions are assumed as for heliports, the size of a landing side is determined as a FATO (Final Approach and Take-off Area) and safety area of at least 2 D. In Annex 14 Vol II—Airports, D is defined as the largest overall dimension of the aircraft when rotors are turning. In this study, maximum vehicle dimensions of 10 m were assumed, resulting in a FATO and safety area of 400 m2. For parking pads, a space requirement of 16 m multiplied 16 m was assumed. Vertiports space requirements also take into account parked vehicles during possible night curfews or operational disruptions. For a small vertiport for 10 vehicles and a passenger throughput of 5400 passengers a day, a vertiport space requirement of 4160 m2 was estimated. For large vertiports for 50 vehicles and daily passenger throughputs of 130,000 passengers a day, more than 20,000 m2 of vertiport space was estimated. Therefore, future vertiport designs have to be efficient in terms of space requirements, operational costs and operational reliability.
Vertiport passenger process The passenger process describes the process from the time passengers arrive at the Vertiport until they board to the eVTOL and from the time they get to the designated vertiport until they deboard. Infrastructure operators such as Fraport assume that a security check is not necessary for a UAM offer; however, the final legal framework still needs to be established [17]. In this paper, it is also assumed that there is no need for a security check similar to the taxi service by car or helicopter service. Therefore, the duration of the passenger process can be significantly reduced.
Due to limited space in urban regions, vertiports will have a limited number of landing pads forcing handling processes be designed to allow a high, constant, reliable and cost-effective throughput, e.g. assembly line, relocation [18].
To improve public transport connections, vertiports must be established at locations in the immediate vicinity of main stations or at termini of the urban rail network.
3.3 Operational and business models concepts
To generate a distinct motivation for the use of urban air mobility from the meta-motive mobility, the critical needs of time saving, stress reduction and attraction feeling were identified. To satisfy these needs, autonomously flying eVTOLs have a utilization of 12 h per day in average. The vehicles can be booked on-line on demand similarly to current public transport offers. Due to the different routes, different aircraft concepts are used depending on the application e.g. multicopters, tilt wings and Hybrid wings. Especially in inner city areas, the distances between the vertiports are usually too short and urban air mobility should not be used as a substitute for public transport, but as a supplement to it. While aircraft manufacturers themselves act as operators during the implementation phase, this is successively transferred to external public service providers who are able to offer mobility as a full service, which is the basis for the elaboration of the business models [19]. This means transparent ticketing for all means of transport, including billing and compensation. The goal is to support mobility users sustainably through intelligent route planning, booking, travel and flight as well as payment processes. Social acceptance is a key success factor for the comprehensive use of UAM [20]. This is primarily achieved through seamless and safe processes. Furthermore, the customer commitment is achieved through customer-oriented communication strategies, such as social media, events, and feedback systems.
Finally, it is important to offer the urban air mobility service for an affordable price. Prices of today’s taxi services are envisaged with prices between 1.70 and 2.00€ per kilometer for Munich without base fare [21]. The following calculation was based on an average flight distance of 40 km and 300 flight days per year. The cost items include vertiport fees, staff, aircraft including depreciation, maintenance and repair, air traffic management (ATM), energy, taxes, insurance and costs for marketing activities and administration. The target profit margin was set to be 5%. The result is a price of 4.94€ per kilometer (including VAT). The composition of the costs is shown in Fig. 8.
To be able to offer comparable prices with taxi of around 2€ per kilometer certain parameters have been modified. The main cost drivers in the calculation are vertiport fees and the number of passengers. With the reduction of the vertiport/landing fees by 70% and increasing the number of passengers from two to four, a price of 1.75€ per kilometer and passenger could be achieved. Many options could be considered to achieve this objective. The reduction of vertiport fees could for example achieved by public subsidies, by reduction in infrastructure costs due to higher aircraft movements or other non-aeronautical revenue streams.
3.4 Urban air mobility vehicle requirements
Defining a set of UAM aircraft requirements as a basis for the design process requires a look into local conditions of the area, where the transport system shall be implemented. The nature of the station network, not only elevations, typical design of buildings and the structure of urban areas, local climatic conditions but also demographic composition, living standards and expected demand strongly influence the decision for one or the other aircraft type and final design choices.
In the subsequent sections, the design mission is systematically derived and precisely tailored to the study region and an advanced scenario with an already established UAM transport system. Additional general assumptions are defined, and design boundary conditions are outlined. The collected requirements and boundary conditions are then used to design appropriate urban air mobility vehicle configurations for the selected study area.
3.4.1 Take-off/landing capabilities, vehicle footprint, maximum take-off weight and energy source constraints
As many other published UAM scenarios, the project’s vision of an UAM transport system obviously requires aircraft capable of pure vertical take-off and landing (VTOL). Short (STOL) or extremely short take-off and landing aircraft (ESTOL) are not an option for operation from space limited inner city vertiports surrounded by other buildings.
Limited space on the vertiports further requires the limitation of the maximum vehicle footprint. The allowed footprint heavily affects the possible implementable VTOL rotor disk area and/or wingspan. On one hand, both design features are to be chosen as large as possible to increase the vehicle energy efficiency, a larger disk area additionally is crucial for reducing noise emissions via reducing rotor tip speed. On the other hand, large vehicle footprints lead to reduced throughput on the vertiports, since less aircraft can be dispatched concurrently. A detailed analysis of available space at vertiport locations with potentially high throughput is to be carried out as future work to find a reasonable maximum vehicle size. Until such an analysis is in place, a 10 × 10 m box is set as an initial assumption for the vehicle to fit in. This is roughly in line with the 50 ft (15.15 m) maximum vehicle dimension defined by Uber within their Elevate campaign [22]. To enable a certification within the EASA Special Condition VTOL Aircraft regulative [23], a maximum take-off weight of 3175 kg is not to be exceeded. Concerning vehicle energy source options, several options and combinations are considered, as shown in Fig. 9.
As a minimum requirement, VTOL, climb and descent, as well as cruise flight over urban areas must be flown with zero local emissions. From an infrastructure point-of-view, the most favorable solution clearly is a pure battery driven power train. Electric power lines and recharging infrastructure are much easier to handle on urban vertiports than any tanks for fuel or hydrogen. However, because of several reasons, hybrid power train concepts could be necessary to meet other crucial requirements. First, limited battery energy density could prevent the required design range of all-electric air taxis. Here, conventional fuel and a piston engine or hydrogen-powered fuel cells could supply power on longer cruise flights in a range extender configuration. Second, hybrid power trains could help meet noise emission targets. Because of the superior energy density of conventional fuel and hydrogen compared to batteries, the overall vehicle weight of hybrid-powered aircraft is potentially lower. This reduces disk loading during VTOL, which has a high impact on noise emissions.
An option to reduce the number of vertiports that must be equipped with fuel or hydrogen refueling infrastructure, the vehicles could take fuel/hydrogen for multiple trips with them. Since the required fuel weight for the considered distances is low, the weight penalty would be limited.
3.4.2 Design mission structure
The UAM design mission for the considered scenario, depicted in Fig. 10, consists of a single regular cruise leg and an additional diversion leg in case of unexpected incidents on the target vertiport at the time of scheduled landing. The single leg mission assumes that the battery can be rapidly recharged or swapped at every vertiport in the network. For take-off and landing maneuvers at the origin vertiport, the destination vertiport and the eventual diversion vertiport, a 30 s hover in ground effect (IGE) is estimated. After take-off, a steep or vertical climb to the minimum flight level Hmin is required for safety reasons, traffic separation and noise abatement. Subsequently, a transition phase is followed by an economic climb to the maximum flight level Hmax. After the cruise segment, an economic descent to Hmin leads to a possible 1 min holding hover phase out of ground effect (OGE). Although in an advanced ATM system all flights might be very well coordinated, the holding represents a buffer phase for operational uncertainties. Finally, the steep near vertical descent to the destination vertiport is followed by the 30 s landing hover IGE.
In the worst-case scenario, the destination vertiport is blocked or not operational on short notice. Then, the diversion segment must be flown according to the same mission layout. While the regular cruise segment is performed with cruise speed VCruise, the diversion leg is not time-critical and the vehicle can be operated at its respective maximum endurance speed, VMaxEndurance.
3.4.3 Vehicle range and diversion reserves
To define the range for the regular cruise mission, a closer look must be taken at the station networks.
To represent this, Fig. 11 shows the station connectivity in the high-density network with an assumed vehicle range of 110 km. Station distances were calculated as direct line between the vertiports multiplied by a de-tour factor of 1.2 [24]. The de-tour factor considered additional trip distance due to following specified flight routes. De-tour factor are intended to be replaced with distances of the actually defined routes in the future.
For a full point-to-point network, a vehicle range of 180 km is required. However, an air taxi designed for that long range will be burdened with a significantly lower efficiency at more frequently flown shorter missions. Reducing the design range to 110 km just reduces the connectivity of the stations at the border of the study area. While the vertiports at the area centre are still connected well, the border stations would loose about 20–30% of their connections (plus three outliers with less than 70% available connections).
Considering the coverage rate of the overall network for different vehicle ranges depicted in Fig. 11, a 110 km range enables about 95% of all potential connections (Fig. 12). The gain of higher ranges in terms of additional possible routes is relatively low.
In addition, due to the low population density at the borders of the study area the expected transport demand on the lost routes is low. Therefore, the selected 110 km design range seems to be an acceptable trade-off.
The relevant worst-case scenario for the diversion range is a balked landing in the city center of Munich, the by far largest urban area in Upper Bavaria. Local bad weather conditions or a blackout in parts or the whole city may prevent save landings on vertiports in this area. In this case, reserves for a 10 km diversion leg ensure a safe flight to city borders where enough space for dedicated emergency infrastructure is available.
3.4.4 Flight altitudes
Concerning cruise altitudes, very different assumptions can be observed in published aircraft requirements. Uber [22] requires a cruise at 1500 ft (~ 460 m) AGL. However, a steep or vertical climb is only required to 50 ft (~ 15 m) AGL [11]. This implicates a relatively long climb flight phase at very low altitudes. While this is advantageous from an energy consumption point of view, it may not be possible due to safety regulations and possibly excessive noise pollution in the overflown areas. Patterson et Al. [25] propose a significantly higher design cruise altitude of 4000 ft (~ 1000 m).
To provide an obstacle clearance as fast as possible, the aircraft were designed for a vertical climb to a minimum cruise altitude of Hmin = 100 m AGL for the project. Considering the currently prescribed minimum obstacle clearance for general aviation of 300 m, this seems to be a rather optimistic assumption. However, frequently updated databases with more accurate obstacle information in combination with a fine-grained air traffic management system and highly agile distributed electric propulsion vehicles may enable this reduced cruise altitude.
To increase the capacity of the airspace, three other flight levels were introduced on top of Hmin. Well-coordinated flight routes and the resulting considerable improvement of the information situation within the airspace may enable a vertical separation of 100 m of the flight levels. This leads to a maximum required cruise altitude of Hmax = 400 m AGL.
Finally, elevation data of the study area must be considered. Figure 13 shows the elevation map of Upper Bavaria.
The maximum difference in altitude between two station networks is 400 m. To overcome this difference in altitude, the design cruise altitude must be increased to Hmax = 800 m. Furthermore, the air taxis were designed to perform take-off and landing at the highest available vertiport at 700 m in Holzkirchen.
3.4.5 Weather and climate boundary conditions
To ensure operability of the UAM network almost all year round, the air taxis must be designed for the most extreme weather conditions that occur in the study region. Temperature-wise, all vehicle operations have to be possible at ISA + 30 conditions. Considering longer missions, head wind may not be neglected. Therefore, the average wind speeds per hour of 66 weather stations in the study region were evaluated. Figure 14 shows the data of the German Weather Service cumulated for the years 2016–2018.
To limit the downtime of the UAM transport system to 1% of the hours per year, the design mission must be able to be flown with a headwind of 20 m/s. As future work, the influence of the very low temperatures during winter in the study region on battery performance must be quantified and taken into account for battery sizing.
4 Simulation results and sensitivity analysis
4.1 Reference simulation and simulation plan
To systematically identify major demand drivers, a reference mission simulation was setup followed by a simulation plan (see Table 1) where different network densities, vehicle speeds, fare and fare structure, passenger process times where varied based on ceteris paribus assumptions. Passenger process times summarizes all time required to access the vertiport and vehicle plus egress of vehicle and vertiport from or to the respective access and egress mode.
The reference simulation case consists of 74 vertiports, an UAM vehicle with a cruise speed of 100 km/h, a fare system of a basis fare of 5€ plus 2€ fare per flown kilometer and passenger process time of 20 min.
4.2 Demand impact of fare and fare structure
A variation of a distance-dependent fare from 1€ per kilometer to 10€ per kilometer with a base fare of 5€ leads to significant changes (see Figs. 15, 16) both on absolute numbers of UAM passengers and UAM’s modal share. In absolute numbers, the majority of UAM demand was on routes of 20 km or less. On shorter distances, the impact of UAM on the overall modal share, yet, was minor. For trips of less than 10 km, UAM modal shares vary between 0.17 (10€/km) and 0.56% (1€/km). This is due to the massive overall number of trips on this distance.
For longer trips, modal shares were more sensitive to fare changes. For the reference case and trips exceeding 30 km, modal shares of 3% or higher were calculated. For very low fares of 1€ per kilometer, modal shares of up to 5–6% (41–110 km) were calculated. Fares of 5€ per kilometer and higher lead to a decrease of UAM’s modal share to 1–2% on routes between 21 and 120 km distance. Fares of 10 € per kilometers led to modal shares of around 0.5% on nearly all distances.
The base fare variation from zero to 10€ per kilometer had a minor overall impact on passenger demand (Figs. 17, 18). However, on very short distances of less than 10 km, a higher impact on overall UAM passengers was observed. Due to the high number of trips in that distance bin, the modal share impact was low.
4.3 Impact of vehicle flight speed and process times
UAM cruise speeds have been varied between 50 and 350 km/h. The impact on UAM passenger demand and modal share over distance is shown in Figs. 19 and 20. Overall change of demand between reference case (100 km/h), slowest (50 km/h) and fastest (350 km/h) vehicle cruise speed, varies only between − 9% and + 8% compared to the reference case. As the time benefit of higher flight speeds at short distances are low, also the demand impact was rather low. However, even on longer routes of 40 km and above where time savings are significant (~ 40 min between 50 and 350 km/h case), UAM demand did not change significantly. With the implemented UAM mode-choice model, demand was found to be more sensitive to price than travel time.
This can also be observed with the variation of passenger process times. On shorter trips, the impact on UAM overall demand (Fig. 21) was significant. An increase from 20 to 30 min showed a significant impact on UAM modal shares up to 100 km distances (Fig. 22).
4.4 Demand impact of network density
One of the last variables to investigate is the network density, where networks with 24, 74 and 130 vertiports have been simulated. The overall impact on UAM demand and modal shares were rather low (Fig. 23).
Comparing the demand at each vertiport for the different network sizes, a balancing shift towards each vertiports was observed. Therefore, a careful selection of vertiport locations without cannibalization effects between vertiports has to be ensured.
4.5 Impact of UAM fleet size
The number of vehicles per vertiport and hence the overall number of vehicles in the UAM transport network has a very high impact on the UAM demand (Fig. 24) and UAMs modal share (Fig. 25). When passenger demand exceeded passenger supply (e.g. number of available vehicles to serve the demand), additional passenger waiting times were observed. This additional waiting time significantly increased with decreasing fleet size and the impact was higher for shorter distances where overall travel times were lower.
Comparing the modal shares for different fleet sizes, only minor differences between fleets of 10 and 20 vehicles as well as between fleets of 100 and 1000 vehicles could be seen. Therefore, it can be assumed that the demand exceeds supply in the 10–20 vehicle per vertiport case whereas demand is nearly covered in the 100 vehicles per vertiport case. However, comparing the existing design with the ones from Uber, Volocopter or Airbus [6, 14, 23], it is clear that handling (de-boarding, cleaning, recharging) more than 20 vehicles simultaneously on ground per station might be challenging.
4.6 Summary of sensitivity analysis
For the reference case, 123,449 UAM trips per day have been calculated compared to the 14 million daily trips within the study area. Comparing effects of all parameter changes (number of vertiports, UAM vehicle cruise speed, number of vehicles per station, passenger process time, base and kilometer-based fare), it appears that fleet size and price per kilometer have the greatest influence (Fig. 26). The fleet size has a significant influence on agents’ waiting times. With an average number of ten vehicles per station, the demand for UAM trips clearly exceeds the supply. Resulting waiting times therefore become so long that the use of UAM is hardly worthwhile. The strong influence of the kilometer-dependent price is due to the resulting large differences in the overall price. The influence of the cruise speed, however, is small. Due to the relatively short average distance, travel time gains due to different speeds are marginal.
Additionally, simulation results showed that an extension of passenger processing times will mainly affect demand on shorter routes, where the process times take up a larger time of the overall trip time. The influence of the base fare was significantly less than that of the kilometer-based fare. A higher base fare mainly reduces demand on very short distances. The impact of fleet size is even stronger on short distances, where longer waiting times have a bigger impact on overall trip times. The influence of the network is rather small and more vertiports mainly distribute demand more evenly.
5 Multi-criteria assessment
In an aim to assess the potentials of UAM to complement public transport in the selected region of Upper Bavaria, the choice of an appropriate assessment tool is necessary. A multi-criteria analysis is therefore proposed due to its ability to evaluate alternatives at different levels; particularly, the multiple attribute utility theory (MAUT) was used. This method can be used with cardinal and qualitative data and one of its main assumptions lies in the linear property of its utility function [26]. This is of interest for indicators that are difficult to express in monetary units, as would be done in a cost–benefit analysis. In the scope of this project, an indicator system was developed to assess proposed UAM scenarios, following three main indicators: environmental, transport-business related, and socio-economic. For each main indicator, a set of sub-indicators was defined, with the corresponding desired outcome. The methodology followed to select and weigh the indicators has been described by Al Haddad et al. [27]. The selected indicators and sub-indicators for the assessment are summarized in Table 2 below:
The sub-indicators results obtained from the simulations were converted to utility points according to the utility theory. The overall utility for each alternative is then calculated, for each of the proposed alternatives (without UAM, with UAM in the different chosen set-ups). The alternative with the highest overall utility is then considered the most desirable.
6 Summary and research gaps
6.1 Summary and key results
In this paper, first results were presented to quantify the long-term application potential of urban air mobility to complement public transport for the Munich Metropolitan Region. To be able to estimate future UAM demand properly, current and future mobility demand on household and detailed origin–destination level must be known. Additionally, a specific mode-choice model for urban air mobility for both commuters and other potential users (e.g. airport passengers) including all other relevant alternative modes of transport (e.g. autonomous vehicles) have to be setup; this would require UAM related stated preference surveys upfront.
For the reference case, 123,449 UAM passengers per day have been calculated compared to the 14 million daily trips within the study area, resulting in a share of around 1%. Only for longer distances, the modal share increases significantly to 3–4% for distances exceeding 30 km. Therefore, it can be assumed that urban air mobility will not significantly change the daily mobility situation in general, but the current transport offer could be complemented by a fast, flexible mode of transport. On short distances (< 10 km), UAM has a modal share of 0.5%. In absolute terms, UAM demand is concentrated (84%) on short distances under 40 km; 55% of demand is on routes < 10 km. It could be shown that passenger processing times at the vertiports of 30 min have a significant impact. The number of vehicles per vertiport has as well a very high impact, and hence the UAM overall transport supply.
6.2 Research gaps
Additional user groups Besides commuters and airport passenger, tourists as a potential customer group have been identified. However, it can be assumed that tourists have a different mobility pattern as well as a different mode-choice compared to commuters. Therefore, it would be crucial to investigate tourists in more detail, especially if a large number of vertiports are located at points of interest with high number of visitors.
Vertiport placement Besides the identification of possible vertiport locations by expert workshops, the ultimate goal would be to locate UAM stations using a rather automatic mechanism using relevant layers of population densities, job densities, average income distributions or major transport mode locations. Those layers can be overlaid, and the maximum coverage of those point layers will be calculated. As a result, the optimal locations for one or more vertiports that will service demand from the surrounding populations would be expected to be determined.
Vertiport preliminary sizing As the proposed vertiport locations do not consider any restrictions in size as well as all simulations were conducted without any capacity constraints (e.g. vehicle take-offs and landings per hour), a preliminary sizing of each vertiport might further limit provided overall transport capacity. Additionally, analyses should enable the design of the vertiports to be easily expandable and upward compatible, demountable and reusable for sustainability reasons.
Vehicle operations Several vehicle design requirements will be defined based on the transport system simulations results. To ensure an economically attractive cruise speed, VCruise and maximum payload, and transport system-wide sensitivity studies should be conducted. In addition, the demand on the different vertiport connections will be evaluated to reduce the required range if possible. Finally, the demand and required passenger flow rate at the stations during peak hours should be evaluated to set the requirements in terms of flight repeatability. This means that vehicles may have to be able to fly shorter missions for a few hours without being fully charged.
Once all requirements are in place, properly sized vehicles of promising aircraft types could be designed and design- and off-design performance for various relevant economic evaluation missions would be evaluated. Depending on the demand for shorter distance flights, a second short-range air taxi would be conceptualized and evaluated in the same way. Overall objectives include the identification of most promising air taxi types and corresponding appropriate application cases as well as the derivation of component requirements.
Noise impact and routing Specific focus has to be set to ensure minimal noise and visual impact on the ground. Therefore, low noise UAM trajectories or UAM trajectories with minimal additional noise on the ground have to be defined.
Role of security So far, it is assumed that similar to helicopter services, a security check is needed. Therefore, the duration of the passenger process can be significantly impacted. As some cities (like Shanghai, China) have security checks to enter public transport system, the impact on UAM must be analyzed.
Induced demand Empirical studies have observed that improvements of infrastructure capacity result in an increase of travel demand. These effects have been measured in many road enhancement projects and were also observed when a new mode is introduced. The new travel demand is called induced demand. Especially, an increase in accessibility might produce more discretionary trips, whereas mandatory trips (work and education trips), might not be affected by improved accessibility.
Welfare impacts This research is a first step in assessing the potential of UAM on inhabitants’ welfare. Yet, additional work is needed to evaluate the potential of UAM to increase connectivity to remote and rural regions as an equitable mode of transport and assess the impacts these changes can have.
Multi-modality Within this research, proximity of vertiports to other modes of transport has already been identified as a demand driver. Nevertheless, other questions in this context, including single-ticketing, service integration and data exchange remain unanswered.
Changes in travel behavior This research bases on large scale, national survey revealed preference data from 2008 as well as first stated preference passenger survey on urban air mobility conducted in 2018. Therefore, potential impacts due to external shocks like the COVID-19 crisis were not considered in this study. Therefore, future research is required to understand how external shocks like pandemics impact these fundamental assumptions within mobility research.
References
Molloy, J., Moeckel, R.: Automated design of gradual zone systems. Open Geospat. Data Softw. Stand. 2, 19 (2017)
Moreno, A.T., Moeckel, R.: Population synthesis handling three geographical resolutions. ISPRS Int. J. Geo-Inf. 7(5), 174 (2018)
Moeckel, Rolf, Kuehnel, Nico, Llorca, Carlos, Moreno, Ana Tsui, Rayaprolu, Hema: Agent-based simulation to improve policy sensitivity of trip-based models. J. Adv. Transp. (2020). https://doi.org/10.1155/2020/1902162
Moeckel, R.: Constraints in household relocation: modeling land-use/transport interactions that respect time and monetary budgets. J. Transp. Land Use 10, 1–18 (2017)
Bayerisches Landesamt für Statistik (2019). https://www.statistik.bayern.de/. Bundesministerium für Arbeit und Soziales (2019). Accessed 17 Mar 2020
Ben-Akiva, M., Lerman, S.R.: Discrete Choice Analysis. Theory and Application to Travel Demand, p. 9. MIT Press (MIT Press series in transportation studies), Cambridge (2006)
Horni, A., Nagel, K., Axhausen, K.W. (eds.): The Multi-Agent Transport Simulation MATSim. Ubiquity Press, London (2016)
Llorca, C., Zhang, Q., Moreno, A.T., Moeckel, R.: Airport access and egress trips in an agent-based travel demand model. In: Presented at the 8th Symposium of the European Association for Research in Transportation (hEART 2019), Budapest (2019)
Fu, M., Rothfeld, R., Antoniou, C.: Exploring preferences for transportation modes in an urban air mobility environment: Munich case study. Transp. Res. Rec. 2673(10), 427–442 (2019)
Koppelman, F.: Predicting transit ridership in response to transit service changes. J. Transp. Eng. 109, 548–564 (1983). https://doi.org/10.1061/(ASCE)0733-947X(1983)109:4(548)
Rothfeld, R. L.; Balac, M.; Ploetner, K. O.; Antoniou, C.: Agent-Based Simulation of Urban Air Mobility. In: AIAA AVIATION, Modeling and Simulation for Unmanned and Personal Aerial Vehicle Operations. American Institute of Aeronautics and Astronautics, Atlanta (2018)
Rothfeld, R.L., Balac, M., Ploetner, K.O., Antoniou, C.: Initial analysis of urban air mobility’s transport performance in sioux falls. In: AIAA AVIATION, Modeling and Simulation for Unmanned and Personal Aerial Vehicle Operations. American Institute of Aeronautics and Astronautics, Atlanta (2018)
Balac, M., Vetrella, A.R., Rothfeld, R., Schmid, B.: Demand estimation for aerial vehicles in urban settings. IEEE Intell. Transp. Syst. Mag. 11(3), 105–116 (2019). https://doi.org/10.1109/MITS.2019.2919500
Pukhova, A., Llorca, C., Moreno, A.T., Zhang, Q., Moeckel, R.: Urban air mobility: another disruptive technology or just an insignificant addition? In: Paper Presented at the European Transport Conference. Dublin, Ireland (2019)
Lineberger R., Hussain A., Metcalfe M., Rutgers V.: Infrastructure barriers to the elevated future of mobility. Deloitte Ser. Future Mobil. (2019)
Fraunhofer, I.B.P.: Integrationsstrategie UAM. Internal Report, Valley, Germany (2019)
Toepsch, F.: Interview with Felix Toepsch Fraport AG, Frankfurt, Germany (2019)
Volocopter: Infrastruktur für Lufttaxis in Großstädten [Youtube] (2018). Retrieved from https://www.youtube.com/watch?v=PPE6833gCz8
Becker A., Straubinger A., Plötner K., Michelmann J.: Urban air mobility 2030 + final presentation, Taufkirchen, Germany (2019)
Al Haddad, C., Chaniotakis, E., Straubinger, A., Plötner, K., Antoniou, C.: Factors affecting the adoption and use of urban air mobility. Transp. Res. Part A Policy Pract. 132, 696–712 (2020)
Taxi-München, E.G.: Tarif und Preise des Münchner Taxigewerbes (2019). http://www.taxi-muenchen.com/privatkunden/tarif-und-preise.html. Accessed 23 Sep 2019
Uber:. UberAir Vehicle Requirements and Missions (2019). Von https://s3.amazonaws.com/uber-static/elevate/Summary+Mission+and+Requirements.pdf. Accessed 23 Sep 2019
EASA-European Union Aviation Safety Agency: Special Condition for Small-Category VTOL Aircraft Report, Cologne, Germany (2019)
Rothfeld, R., Fu M., Antoniou, C.: Analysis of urban air mobility’s transport performance in munich metropolitan region. In: Presented at Mobil.TUM Conference 2019, Munich, Germany (2019)
Patterson, M.D., Antcliff, K.R., Kohlmann, L.W.: A proposed approach to studying urban air mobility missions including an initial exploration of mission requirements. In: VFS’s 75th Annual Forum and Technology Display. Phoenix, United States (2018)
Keeney, R.L., Raiffa, H.: Decision Analysis with Multiple Conflicting Objectives. Wiley, New York (1976)
Al Haddad, C., Fu, M., Straubinger, A., Plötner, K., Antoniou, C.: Multi-criteria analysis for assessing disruptive transport systems. A case study of urban air mobility in Germany. Eur. J. Transp. Infrastruct. Res. (2020) (Under Revision)
Straubinger, A., Fu, M.: Identification of Strategies How Urban Air Mobility Can Improve Existing Public Transport Networks. In: Poster presented at mobil.TUM 2019, Munich (2019)
Acknowledgements
The paper presents results from the research project OBUAM, which has been funded by the Bavarian Ministry of Economic Affairs, Regional Development and Energy (Grant no.: LABAY101A).
Funding
The paper publishes results from the research project OBUAM, which has been funded by the Bavarian Ministry of Economic Affairs, Regional Development and Energy.
Author information
Authors and Affiliations
Contributions
KOP: Sections 1, 1.1, 4, and 6. CAH: Section 5. CA: Section 5. FF: Sections 3.2 and 3.3. MF: Section 2.3. SK: Sections 3.2 and 3.3. CL: Section 2.2. RM: Sections 1.2, 2.2, and 2.3. ATM: Sections 2.1 and 2.3. AP: Section 2.1. RR: Section 2.4. MS: Section 3.4. AS: Section 4. HW: Sections 3.2 and 3.3. QZ: Section 2.3.
Corresponding author
Ethics declarations
Conflicts of interest
The author(s) declare that they have no competing interests
Code availability
SILO model: https://github.com/msmobility/silo. MITO model: https://github.com/msmobility/mito. MATSim UAM: https://bauhausluftfahrt.github.io/MATSim-UAM/.
Additional information
Publisher's Note
Springer Nature remains neutral with regard to jurisdictional claims in published maps and institutional affiliations.
Rights and permissions
About this article
Cite this article
Ploetner, K.O., Al Haddad, C., Antoniou, C. et al. Long-term application potential of urban air mobility complementing public transport: an upper Bavaria example. CEAS Aeronaut J 11, 991–1007 (2020). https://doi.org/10.1007/s13272-020-00468-5
Received:
Revised:
Accepted:
Published:
Issue Date:
DOI: https://doi.org/10.1007/s13272-020-00468-5