ABSTRACT
BACKGROUND
The range of hospital charges for similar diagnoses show tremendous variability across U.S. hospitals. This charge variability remains unexplained.
OBJECTIVE
We aimed to describe hospital charge variability in the U.S. and examine its relationship to local health factors.
DESIGN
This was a descriptive study of the 2011 Medicare Inpatient Charge data summarizing inpatient hospital charges billed to Medicare. This data was evaluated using 29 county-level measures of health status, health behavior, clinical access and quality, built environment, and socioeconomic status in a clustered, multivariate linear regression.
PARTICIPANTS
2871 U.S. hospitals registered with Medicare and with at least ten discharges for diagnosis-related groups (DRGs) of six common inpatient conditions.
MAIN MEASURE
Inpatient hospital charges were assessed.
KEY RESULTS
No community health measures were associated with hospital charges. The one notable exception associated with higher charges was higher rates of uninsured status ($344.84 higher charges for every one-percentage point increase in prevalence (p < 0.001)). One variable was associated with lower hospital charges: the percentage of children living in poverty [$309.30 lower charges for every one-percentage point increase in prevalence (p < 0.001)].
CONCLUSIONS
Overall, hospital charges lacked an association with population health measures, and their variability remains largely unexplained. However, the association of higher charges with uninsured status raises concerns about hospitals’ price-setting strategies, such as price discrimination and cost-shifting strategies that expose vulnerable populations to great financial risks.
Similar content being viewed by others
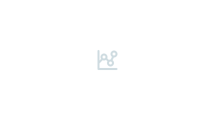
Recent, unprecedented media attention has focused on high health care charges in the United States, particularly by acute care hospitals. These reports note hospital charges to be highly variable across the country and several magnitudes higher than charges in other countries.1,2 Despite this anecdotal evidence, there is little empirical evidence to explain this variability, raising concerns about the lack of transparency in hospital pricing.
High health care charges may be a symptom of the larger problem of rising health care costs in the U.S. For decades, hospitals have increased the charged prices of their services in response to lower reimbursements rates and climbing costs of providing uncompensated care.3 This trend of escalating health care costs may make health care unaffordable for many. In contrast, hospital administrators and some policy makers have minimized the importance of hospital charges (or the chargemaster price list). Some suggest that charges have little relationship to the actual costs of or reimbursement for care. In addition, it is often noted that few individuals are ever exposed to the totality of a hospital’s charges.1,2
In fact, hospital charges are important for multiple health system stakeholders. First, uninsured individuals (who are also often low income) are often responsible for the billed hospital charges. Such bills are financially taxing, particularly to low-income individuals, and are cited as the most common cause of personal bankruptcy in this country.4 Second, hospital charges are often used as a proxy for the value of hospital care. For example, Medicare uses hospital charges to calculate “outlier” payments for complex cases, and hospitals use listed charges to calculate the value of their charity services. Lastly, charges are at times used to determine payment for care, with private insurance companies negotiating payments based on such charges.5,6
If hospital charges are not simply fictitious, then the factors that drive variation in hospital charges are unknown. One concern is that local market factors, such as community heath factors, may influence hospital charges. Hospitals serving a high proportion of unhealthy patients may charge higher prices in an effort to obtain higher reimbursements for care of costly patients.5,6 Similarly, hospitals serving a high proportion of uninsured patients may charge higher prices to private insurers in an effort to increase private-payment rates and cross subsidize the care of the uninsured. This strategy may have the unintended consequence of increasing the bills faced by uninsured patients. While the Affordable Care Act has reduced the uninsured rate, many individuals remain uninsured and potentially exposed to exorbitant, hospital-based charges. A better understanding of how hospital charges are determined is key to ensuring fair pricing practices.
In an effort to increase public awareness of hospital charges, the Center for Medicare and Medicaid Services (CMS) released previously undisclosed information about the prices hospitals charge Medicare. It provides a key opportunity to understand the hospital industry’s price-setting practices. This study aims to advance our understanding of the hospital industry’s price-setting practices. To the best of our knowledge, this is the first study of first to use Medicare data and systematically analyze charges. We examine the relationship between hospital charges for inpatient services and community factors such as socioeconomic factors and population health.
METHODS
Our goal was to describe variation in hospital charges across all U.S. acute-care hospitals and assess its relationship to community-level socioeconomic factors and health status. We examined charges for six common, inpatient conditions: cellulitis, chronic obstructive pulmonary disease (COPD), congestive heart failure (CHF), myocardial infarction (MI), orthopedic surgeries, and pneumonia. These six conditions were chosen to represent a broad range of diagnoses that frequently require hospitalization. Each condition was defined by specific diagnosis-related groups (DRGs) (described in online supplemental Table 1).
Outcome Variable
Hospital charges were taken from the 2011 CMS Inpatient Charge data for the top 100 DRGs billed to Medicare. This data set included 3337 U.S. acute care hospitals registered with Medicare and had at least ten discharges for any given DRG. For each hospital, we calculated a condition-aggregated charge by averaging the charges across conditions and weighting the charge by the hospital’s proportion of discharges per DRG. Forty-three hospitals did not have charge data for any of the conditions used for this study and were dropped from the sample, leaving 3294 hospitals with charge data.
Explanatory Variables
Our explanatory variables were primarily derived from the Robert Wood Johnson Foundation County Health Rankings data, which uses a collection of county-level health and socioeconomic factors from validated data sources to model and measure community health.7 We followed the County Health Rankings conceptual model using explanatory variables in the areas of health status, health behavior, access and quality of care, and physical environment that might explain population health. We hypothesized that these factors may contribute to poor population health, which may increase hospital charges through increased costs of care for sicker patients. We also included the County Health Ranking’s measures of socioeconomic status, hypothesizing that low socioeconomic status may increase hospital charges in an effort to cross-subsidize charity care. Below we describe each explanatory variable. Online supplemental Table 2 lists the data sources for each of these variables.
Health Status
We hypothesized that population disease burden contributes to population health and that poorer health is associated with higher hospital charges. We used the following measures of population health status: age-adjusted years of potential life lost per 100,000 population, percent of adults who report fair or poor health, percent days reported as physically unhealthy per month, percent days reported as mentally unhealthy per month, obesity prevalence, and percent of live births less than 2,500 grams.
Health Behavior
Certain health behaviors are known to result in worse health. For example, tobacco use is a known risk factor for the development of COPD and physical inactivity for cardiovascular disease. We hypothesized that such behaviors would lead to poorer health and higher hospital charges. We used the prevalence of smoking, the percentage of adults reporting no leisure time activity, the prevalence of heavy alcohol drinking, motor vehicle crash mortality rate, chlamydia rate, teen birth rate between the ages of 15 and 19 years as markers of detrimental health behaviors.
Access to and Quality of Outpatient Care
The next set of variables reflects an individual’s ability to access outpatient care and the quality of care received. We hypothesized that reduced access to health care, as reflected by the number of primary care physicians and dentists per 100,000 population, could lead to poorer health status and ultimately higher hospital charges. In addition, we hypothesized that higher quality outpatient care could lead to better health and lower hospital charges. The quality of outpatient care was measured using the rate of preventable hospitalizations of ambulatory care sensitive conditions, the percent of diabetic patients who received a hemoglobin A1c test, and the percent of women who received screening mammography.
Physical Environment
The neighborhoods in which individuals live may impact health, depending on the environmental quality (air and water quality) or local built environment (access to healthy food options or outdoor recreational areas). We used the average daily fine particulate matter level and the percent of the population exposed to water sources exceeding violation limits as measures of environmental quality. In addition, we used the number of accessible recreational facilities per 100,000 population, percent of the population that lives in poverty and within 1 to 10 miles from a grocery store, and the percent of all restaurants that are fast food as measures of the built environment.
Socioeconomic Status
We hypothesized that lower socioeconomic status would result in higher hospital charges. This could happen both through higher hospital charges to cross-subsidize uncompensated care or the known adverse health consequences of poverty, unemployment, lower educational attainment, crime, and poor social support.8–11 To measure socioeconomic status, we used the percent of individuals who are uninsured, percent of a 9th grade cohort that graduates from high school in four years, percent of adults aged 25–44 years with some college education, prevalence of unemployment, percent of children living in poverty, percent of adults with inadequate social support, percent of children living in single-parent homes, and violent crime rate per 100,000 population.
ANALYSIS
We merged the hospital charge data with data containing hospital and community-level variables. Of the original cohort of 3294 hospitals, 423 had missing data on one or more community or hospital characteristics (< 2 % missing for all variables except five, which had<5 % missing). Hospitals with missing data were dropped, leaving 2871 hospitals in our final sample.
Using this sample, for each condition, we calculated summary statistics for hospital charges and the total number of discharges across all hospitals. We also calculated the average hospital charge across all six conditions for each hospital. These condition-aggregated, average hospital charges were stratified into quartiles, and summary statistics for each quartile were calculated.
Linear regressions were run at the hospital level, using the condition-aggregated, average charge as the dependent variable and including all county-level variables as independent variables. Analyses using the condition-specific, average hospital charge were performed for each condition and produced qualitatively similar results (see online supplemental table 3). Due to missing data from the original data set, we assessed its impact using mean imputation for missing data. This analysis produced qualitatively similar results as the final analysis. Hence, we present the result using the final sample of 2871 hospitals.
All analyses included covariates for hospital and market factors that may be correlated with both hospital charges and explanatory variables: the percent of the population over the age of 65 (to correct for charge variation related to an aging population), hospital wage index (to correct for charge variation related to labor costs), hospital cost-to-charge ratio (to correct for charge variation that stems from variation in costs), county-level Herfindahl-Hirschman Index (to correct for price variation related to market competition within the county), hospital case mix index (to correct for charge variation that stems from case severity), and for-profit status (to correct for charge variation that stems from hospital operations). Because more than one hospital could be located in a single county, the regression was clustered by county to generate the robust standard errors using the Huber-White sandwich estimators.
Because the distribution of hospital charges is right-skewed, we tested the sensitivity of our results to our choice of a linear model by repeating the analysis using log-transformed charges. We also used a generalized linear model, which directly characterizes how the expectation on the original scale is related linearly to the predictors. This model contrasts the use of transformation of the outcome variable to deal with issues of skewness. Both analyses did not qualitatively change our results. We present only the results using the non-transformed charge variable.
Due to concerns of multicollinearity, we performed an analysis to assess for its presence. First, we conducted a regression using a single, representative variable from each category and found the coefficient and significance remained unchanged from the full regression analysis. We also assessed the variance inflation factor for each explanatory variable. Only one variable—age-adjusted years of potential life lost per 100,000 population—was above the threshold of ten and removed from the model.
All statistical tests were two-sided and deemed significant with p values<0.002 using the Bonferroni method to address multiple comparisons. Analyses were performed using STATA 13.1. The study was exempt from institutional review board approval by the Rutgers University Institutional Review Board.
RESULTS
The summary of hospital charges for each condition is displayed in Table 1. The median hospital charge ranged from $17,389 for cellulitis to $48,039 for orthopedic surgeries. Hospital charges were highly variable within each condition and across conditions. After averaging each hospital’s charges across the six conditions, the median hospital charge was $29,551.
Table 2 summarizes the condition-aggregated, average hospital charge stratified into quartiles. The highest quartile of hospitals demonstrated the greatest variability in hospital charges, which resulted in the significantly, right-skewed distribution.
Hospital charges varied significantly across the country. Figure 1 displays the nationwide distribution of average hospital charges for each county. Areas with the highest hospital charges were clustered in the Southwest (Arizona and California), the mid-Atlantic (New Jersey, eastern Pennsylvania and southern New York), and Florida. Counties with high hospital charges tended to be in metropolitan areas.
In multivariate regression, the only variable to explain higher hospital charges was the prevalence of uninsured status. The prevalence of uninsured status was associated with $344.84 higher hospital charges for every 1-percentage point increase in its prevalence (p<0.001). Health-related factors explained none of the charge variation (see Table 3).
One variable was associated with lower hospital charges: the percentage of children living in poverty. Hospital charges were $309.30 lower for every 1-percentage point increase in poverty status (p<0.001).
DISCUSSION
This descriptive study focuses on the variability of hospital charges and its relationship to local population factors. We hypothesized that higher inpatient charges would be associated with worse population health status; however, no health status factors were associated with higher charges. Of the socioeconomic factors, only the rate of uninsurance was associated with higher hospital charges. These findings suggest that hospital charges remain largely unexplained, while also raising concern for regressive price-setting practices that expose vulnerable populations to higher charges.
Based on our study, hospital charges lacked a relationship with population health indicators. This finding may be surprising since health status is generally associated with illness severity, which can drive hospital charges. For example, tobacco use is a known risk factor for developing COPD or coronary artery disease and may impact hospitalizations and its charges for such conditions. In fact, alcohol abuse, smoking, and obesity—all of which are variables in our regression model—have been shown to contribute to increased inpatient health care expenditures,12 yet did not have an association with higher charges in our study. The lack of a relationship between health status and charges reinforces prior concerns of arbitrary price setting by hospitals and supports the need for price transparency of medical services.13
Higher hospital charges were associated with uninsured status. The reasons behind the association between rates of uninsurance and hospital charges are not clear. One possible explanation is that uninsured individuals tend to have worse health status, which may result in greater inpatient resource utilization.14,15 However, our model was adjusted for case mix severity, yet uninsured status remained significant. Also, health status variables were not associated with hospital charges, but may vary for uninsured people in ways we did not observe in our data.
The association between uninsured status and higher charges may be due to price discrimination and cost-shifting strategies employed by hospitals. Prior research has demonstrated evidence of cost-shifting, where costs for under-reimbursed or unreimbursed care are shifted to other revenue sources.16–19 However, economists have questioned the existence of cost-shifting based on other empirical analyses that do not support such practices.20,21 Our findings provide additional evidence for cost-shifting. This regressive pricing strategy may preferentially target vulnerable populations who are self-pay and expose them to the highest prices of hospital care.3,5,6 In fact, research has demonstrated the financial benefit of pursuing collections from uninsured, self-pay individuals.22 Hospitals collected more charges from uninsured patients than from commercially insured patients, further legitimizing this concern for cost-shifting.16
The prevalence of children living in poverty was the only variable associated with lower charges. Our analysis support prior reports suggesting hospitals reduce charges for individuals below a certain income threshold. Alternatively, individuals living in poverty may be enrolled in Medicaid, and our results may reflect charges influenced by such enrollment. Ultimately, the relationship between poverty and lower charges remains unclear and in need of further research.
Based on the distribution of hospital charges in the U.S., a small portion of hospitals generated the wide variation in charges. While we did not examine the specific characteristics of this small group of hospitals, we noted their location in counties that cluster near highly populated, metropolitan areas. Such high charges could occur for a variety of reasons. First, urban environments are known to have a higher prevalence of poor health and uninsured individuals that could lead to greater inpatient health care utilization. Second, the higher cost of living in metropolitan areas may explain this association. However, our analysis corrects for labor cost variability through the wage index covariate. Another consideration may be the presence of academic medical centers, which are known to incur higher costs for hospitalizations in each of these areas.23,24 Further research is needed to elucidate the association between metropolitan areas and higher charges.
This study has several strengths and weaknesses. First, there is a lack of research in hospital pricing practices given the lack of regular access to pricing data. The studies that have focused on hospital pricing variability used charge data from a single state or claims data.16,25,26 To our knowledge, our study is the first to use Medicare data that encompasses charge data for nearly all U.S. hospitals. In addition, this study utilizes several, well-validated, nationally-representative data sets. Given the use of secondary data, this study is unable to assess a causal link between community factors such as uninsurance rates and hospital charges. Also, our analyses cannot distinguish whether explanatory variables deemed non-significant are truly unrelated to the dependent variable or just poor measures of the intended association. Lastly, our regression analysis may be limited by concerns for endogeneity. Despite a robust model of community health, there may be other unknown variables driving the statistical findings. However, our findings are limited to only two variables that were associated with hospital charges, and further work is needed to validate our findings.
Despite these limitations, these data provide important new insights into hospital pricing variability. Hospital charge variability generally lacked an association with population health status and remains largely unexplained. This finding raises concerns about the arbitrary nature of hospital charges. Despite the lack of explanation, our study did identify potential regressive pricing strategies that raise concerns about price discrimination and cost-shifting strategies that expose vulnerable populations to great financial risks.
REFERENCES
Brill S. Bitter pill: why medical bills are killing us. Time. 2013;4:16–55.
Rosenberg T. “Revealing a health care secret: the price”. The New York Times. 31 July 2013. Available at: http://opinionator.blogs.nytimes.com/2013//07/31/a-new-health-care-approach-dont-hide-the-price. Accessed August 2, 2013.
Tompkin CP, Altman SH, Eilat E. The precarious pricing system for hospital services. Health Aff (Millwood). 2006;25(1):45–56.
Himmelstein DU, Thorne D, Warren E, Woolhandler S. Medical bankruptcy in the United States, 2007: results of a national study. Am J Med. 2009;122(8):741–6.
Reinhardt UE. The pricing of U.S. Hospital services: chaos behind a veil of secrecy. Health Aff (Millwood). 2006;25(1):57–69.
Anderson GF. From ‘Soak the Rich’ to ‘Soak the Poor’: recent trends in hospital pricing. Health Aff (Millwood). 2007;26(3):780–9.
Robert Wood Johnson Foundation and the University of Wisconsin Population Health Institute. County Health Rankings and Roadmaps. 2013. Available at: http://www.countyhealthrankings.org. Accessed December 10, 2013.
Woolf SH, Braveman PA. Where health disparities begin: the role of social and economic determinants – and why current policies may make matters worse. Health Aff (Millwood). 2011;30(10):1852–9.
Braveman PA, Cubbin C, Egerter S, Williams DR, Pamuk E. Socioeconomic disparities in health in the United States: what the patterns tell us. Am J Public Health. 2010;100(S1):S186–96.
Woolf SH, Dekker MM, Byrne FR, Miller WD. Citizen-centered health promotion – building collaborations to facilitate healthy living. Am J Prev Med. 2011;40(1S1):S38–47.
Braveman PA, Egerter S, Williams DR. The social determinants of health: coming of age. Annu Rev Public Health. 2011;32:381–98.
Strum R. The effects of obesity, smoking, and drinking on medical problems and costs. Health Aff (Millwood). 2002;21(2):245–53.
FInker SA. The distinction between cost and charges. Ann Intern Med. 1982;96(1):102–9.
McWilliams JM, Meara E, Zaslavsky AM, Ayanian JZ. Health of previously uninsured adults after acquiring medicare coverage. JAMA. 2007;298(24):2886–94.
Ayanian JZ, Weissman JS, Schneider EC, Ginsburg JA, Zaslavsky AM. Unmet health needs of uninsured adults in the United States. JAMA. 2000;284(16):2061–9.
Melnick GA, Fonkych K. Hospital pricing and the uninsured: do the uninsured pay higher prices? Health Aff (Millwood). 2008;27(2):w116–22.
Zwanziger J, Bamezai A. Evidence of cost shifting in California Hospitals. Health Aff (Millwood). 2006;25(1):197–203.
Robinson J. Hospitals respond to medicare payment shortfalls by both shifting costs and cutting them, based on market concentration. Health Aff (Millwood). 2011;30(7):1265–71.
Ginsburg PB. Can hospitals and physician shift the effects of cuts in medicare reimbursements to private payers? Health Aff (Millwood). 2003;Suppl Web Exclusives:W3-472-479.
Frakt AB. How much do hospitals cost shift? A review of the evidence. Millbank Q. 2011;89(1):90–130.
White C. Contrary to cost-shift theory, lower medicare hospital payment rates for inpatient care lead to lower private payment rates. Health Aff (Millwood). 2013;32(5):935–43.
Lynk WJ, Alcain RF. The level of hospital charges and the income of the uninsured patient. Int J Health Care Finance Econ. 2008;8(1):53–72.
Mechanic R, Coleman K, Dobson A. Teaching hospital costs – implications for academic missions in a competitive market. JAMA. 1998;280(11):1015–9.
Koenig L, Dobson A, Ho S, Siegel JM, Blumenthal D, Weissman JS. Estimating the mission-related costs of teaching hospitals. Health Aff (Millwood). 2003;22(6):112–22.
Hsia RY, Antwi YA, Weber E. Analysis of variation in charges and prices paid for vaginal and cesarean sections births: a cross-sectional study. BMJ Open. 2014;4(1), e004017.
White C, Reschovsky JD, Bond AM. Understanding differences between high- and low-price hospitals: implications for efforts to rein in costs. Health Aff (Millwod). 2014;33(2):324–31.
Contributors
None.
Funders
This analysis was awarded second place in the Robert Wood Johnson Foundation Health Hospital Price Transparency Challenge, which had no role in the conduct of the study.
Prior Presentations
2014 Society of General Internal Medicine National Meeting, San Diego, California, 26 April 2014.
Conflict of Interest
None.
Author information
Authors and Affiliations
Corresponding author
Electronic supplementary material
Below is the link to the electronic supplementary material.
Supplemental Table 1
(DOCX 16 kb)
Supplemental Table 2
(DOCX 23 kb)
Supplemental Table 3
(DOCX 47 kb)
Rights and permissions
About this article
Cite this article
Park, J.D., Kim, E. & Werner, R.M. Inpatient Hospital Charge Variability of U.S. Hospitals. J GEN INTERN MED 30, 1627–1632 (2015). https://doi.org/10.1007/s11606-015-3352-0
Received:
Revised:
Accepted:
Published:
Issue Date:
DOI: https://doi.org/10.1007/s11606-015-3352-0