Abstract
Objectives
To develop and validate a general radiomics nomogram capable of identifying EGFR mutation status in non-small cell lung cancer (NSCLC) patients, regardless of patient with either contrast-enhanced CT (CE-CT) or non-contrast-enhanced CT (NE-CT).
Methods
A total of 412 NSCLC patients were retrospectively enrolled in this study. Patients’ radiomics features not significantly different between NE-CT and CE-CT were defined as general features, and were further used to construct the general radiomics signature. Fivefold cross-validation was used to select the best machine learning algorithm. Finally, a general radiomics nomogram was developed using general radiomics signature, and clinical and radiological characteristics. Two groups of data collected at different time periods were used as two test sets to access the discrimination and clinical usefulness. Area under the receiver operating characteristic curve (ROC-AUC) was applied to performance evaluation.
Result
The general radiomics signature yielded the highest AUC of 0.756 and 0.739 in the two test sets, respectively. When applying to same type of CT, the performance of general radiomics signature was always similar to or higher than that of models built using only NE-CT or CE-CT features. The general radiomics nomogram combining general radiomics signature, smoking history, emphysema, and ILD achieved higher performance whether applying to NE-CT or CE-CT (test set 1, AUC = 0.833 and 0.842; test set 2, AUC = 0.839 and 0.850).
Conclusions
Our work demonstrated that using general features to construct radiomics signature and nomogram could help identify EGFR mutation status of NSCLC patients and expand its scope of clinical application.
Key Points
• General features were proposed to construct general radiomics signature using different types of CT of different patients at the same time to identify EGFR mutation status of NSCLC patients.
• The general radiomics nomogram based on general radiomics signature, and clinical and radiological characteristics could identify EGFR mutation status of patients with NSCLC and outperformed the general radiomics signature.
• The general radiomics nomogram had a wider scope of clinical application; no matter which of NE-CT and CE-CT the patient has, its EGFR mutation status could be predicted.
Similar content being viewed by others
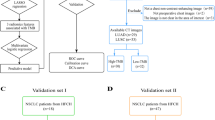
Introduction
Considering the growing insight into the molecular mechanisms of lung cancer, the treatment of non-small-cell lung cancer (NSCLC) has shifted its focus to determining oncogenic driver mutation subtypes. The most common gene mutation in NSCLC is epidermal growth factor receptor (EGFR) mutation [1, 2]. A recent study showed that the patient who received third-generation EGFR-TKI of osimertinib even had a longer overall survival [3]. Therefore, the testing of EGFR mutation status before treatment is very important.
The detection of EGFR mutant status relies on tumor tissue from surgical or tissue biopsy, which is an invasive sampling method. Inspired by genomics and tumor heterogeneity, radiomics approach transforms any type of medical images into quantitative data to guide clinical decisions [4, 5]. Studies have shown that either non-contrast-enhanced CT (NE-CT) or contrast-enhanced CT (CE-CT) can be used to build radiomics models to predict EGFR mutation status [6,7,8,9]. CE-CT showed superior diagnosis abilities over NE-CT in identifying the type of EGFR mutant [10]. Radiomics comparison study showed that some radiomics features were not significantly different between NE-CT and CE-CT [11]. However, whether radiomic features extracted from NE-CT and CE-CT can be used together to build EGFR mutation status prediction model remains unknown.
The purpose of this study is to develop a method to construct radiomics signature and nomogram using different types of CT from different patients simultaneously, so that it cannot only identify EGFR mutation status of NSCLC patients, but also can be applied to different types of CT.
Materials and methods
Study population
This study was approved by our institutional review board. Informed consent from the patients was waived for this retrospective study. The study comprises two sets of patients from different study time. The first set included a total of 784 patients with pathological confirmed NSCLC from January of 2017 to June 2019. The second set is from June of 2020 to December of 2020, when a total of 44 patients with NSCLC and who underwent paired NE-CT and CE-CT before surgery were retrospectively enrolled. The exclusion criteria were as follows: (I) without molecular testing of EGFR mutation; (II) without CT before surgery; (III) with a history of other malignant tumors; (IV) with therapy before detection of EGFR mutations. The EGFR mutant status was detected with a polymerase chain reaction and confirmed by direct sequencing [12]. The clinical information including age, gender, smoking history, pulmonary function tests, pathological type, and the results of molecular testing were collected from the personal medical charts.
As shown in Fig. 1, the enrolled patients were divided into three sets: (I) training set (n = 327), which consisted of patients whose scan time was before June of 2019 and only had one type of CT (167 NE-CT and 160 CE-CT); (II) test set 1 (n = 66), which consisted of patients whose scan time was before June of 2019 and had both NE-CT and CE-CT (66 NE-CT and 66 CE-CT); (III) test set 2 (n = 19), which consisted of patients whose scan time was during June of 2020 and December of 2020 and had both NE-CT and CE-CT (19 NE-CT and 19 CE-CT).
CT scan protocol
All patients who underwent chest CT scan used one of the two multidetector CT systems (Brilliance CT, Philips Healthcare; Toshiba CT). All the subjects were examined at full inspiration in the supine position with or without contrast material. The scanning parameters were as follows: 100–120 kVp, 100 mA, detector collimation of 64 × or 128 × 0.625 mm; field of view of 350 × 350 mm; and matrix of 512 × 512 using a reconstruction kernel for the lung. For CE-CT, after routine CT, a dose of 85 mL non-ionic iodinated contrast material (350 mg iodine/mL, Omnipaque, GE Healthcare) was injected into the antecubital vein at a rate of 3.0 mL/s using an automated injector (Ulrich CT Plus 150, Ulrich Medical). CT scanning was performed again with a 25-s delay after the injection. NE-CT and CE-CT of 5 mm were retrieved from the Picture Archiving and Communication System (PACS) workstation with format of DICOM.
CT evaluation
One chest radiologist with 15 years of experience interpreted the CT radiological features, including size, location, mass or nodules, morphology, opacity of tumor, interstitial changes of lung, bronchitis, bronchiectasis, emphysema, lymphadenopathy, pleural thickening, and pleural retraction. She described the location of tumor in the five lobes consisting of the upper lobes, middle lobe, and lower lobes. The radiological morphology features of tumor were subcategorized as lobulation, spiculation, cavitation, and pleural retraction. The opacity of tumor was classified into solid, part-solid, mostly part-solid, or ground-glass nodule [13]. Interstitial lung disease (ILD) diagnosis referred to the diagnostic criteria updated by the American Thoracic Society (ATS)/European Respiratory Society (ERS) in 2013 [14].
VOI segmentation and radiomics features extraction
Figure 2 showed the workflow of this study. The entire tumor on CT images was defined as the volume of interest (VOI). The same chest radiologist used a research platform (InferScholar; https://www.infer-vision.com/) to manually segment the VOI slice. the voxel size of VOI was resampled to 1*1*1 mm3 by cubic interpolation to reduce the variability of radiomic feature values due to different voxel sizes [15].
For each VOI, PyRadiomics [16] was used to automatically extract the quantitative radiomic features. Radiomic features extraction was performed on the VOI and the VOI converted by different filters. Specifically, six types of radiomics features were extracted, including first-order (18 features), gray-level co-occurrence matrix (GLCM, 22 features), gray-level size zone matrix (GLSZM, 16 features), gray-level run length matrix (GLRLM, 16 features), neighboring gray-tone difference matrix (NGTDM, 5 features), and gray-level dependence matrix (GLDM, 14 features). When calculating the texture features and two first-order features, the values of CT were discretized with a fixed bin width of 32 Hounsfield units (HU). Overall, 1092 quantitative radiomic features were extracted. Details of the radiomics features were provided in supplementary Table 1.
Feature selection in terms of reproducibility
To evaluate the reproductivity of the radiomic features, 1 month later, 50 patients were randomly selected from the training set and re-segmented by the previous radiologist. Intraclass correlation coefficient (ICC) was utilized to evaluate the intra-observer agreement between the two repeated segmented; values > 0.80 indicated good reproducibility, otherwise was excluded in the following feature selection process[8].
General feature
To explore whether the radiomic features obtained by NE-CT and CE-CT can be used simultaneously for analysis, we compared three data usage methods. Here, we proposed a general features approach, called G-NC (General NE-CT and CE-CT). The general features selection that contained three steps was applied in the training set. The first step was to divide the data into two groups according to their CT type (NE-CT or CE-CT). In the second step, the differences of radiomic features extracted from two groups were compared using Mann–Whitney U test. In the third step, the radiomic features showed significant differences between two groups (p < 0.05) were excluded, and the rest of the features were retained as general features. The second data usage method was called NE, which indicated the radiomic features extracted from NE-CT. The last data usage method was to use the radiomic features extracted from CE-CT, namely CE.
Selection of prediction factors and establishment of prediction model
We used a three-stage feature selection method for predicting factors selection. The first stage was to perform a Mann–Whitney U test between EGFR wild-type and the EGFR mutant patients for all radiomic features. The features with p < 0.05 were input to the second stage. The second stage was to calculate the Pearson correlation coefficient, defined as r, between each pair of input features. If there was a pair of features with |r|> 0.85, only the one with a smaller p value calculated in the first stage would be kept. The last stage was to evaluate all remaining features’ importance by a feature selection algorithm called Boruta. The algorithm was designed as a wrapper around Random Forest classification algorithm, which provided unbiased and stable selection of important and non-important attributes from an information system. After feature selection, a fivefold cross-validation strategy was used to choose the best machine learning method to construct a radiomic model [17]. The machine learning methods used included support vector machine (SVM), logistic regression (LR), random forest (RF), gradient boosting decision tree (GBDT), and naive Bayesian classification (NBC).
Nomogram construction
The radiomics signature was the prediction score of the radiomic model (details in supplementary). All clinical and radiological characteristics that differ significantly between EGFR wild-type and EGFR mutant in the training set, as well as the radiomics signature, were included in a multivariate logistic regression using forward stepwise selection to select independent predictors. Finally, a nomogram was contracted based on the independent predictors.
Statistical analysis
The differences of all features between EGFR wild-type and EGFR mutant were assessed using Mann–Whitney U test for continuous variables and Fisher’s exact test or chi-square test for categorical variables. The discrimination was evaluated by area under the receiver operating characteristic curve (ROC-AUC). Decision curve analysis (DCA) was used to evaluate clinical usefulness by quantifying the net benefits of the nomogram in both two test sets [18]. DeLong test [19, 20] was used for statistical comparisons of ROC curves. All statistical analyses were performed with R (version 3.5.0; http://www.Rpro-ject.org) and SPSS (version 22.0, IBM). A two-tailed p value was considered statistically significant if less than 0.05.
Results
Clinical and radiological characteristics
There were 176, 37, and 9 patients with EGFR mutation and 151, 29, and 10 with EGFR wild-type in the training set, test set 1, and test set 2, respectively. EGFR mutation rates were significantly higher in women than those in men, and in non-smokers than in smokers. Regarding other non-radiomics features, histology, COPD, ILD (p < 0.001), type of lesion (p = 0.008), size (p = 0.001), and emphysema (p < 0.001) were statistically different between the two groups. EGFR mutation was more likely to be found in groups with smaller size, adenocarcinoma and without emphysema, COPD, and ILD (Table.1).
Radiomics model analysis
The final feature counts of NE, CE, and G-NC were 5, 1, and 5, respectively (supplementary Table 2). The machine learning algorithms used for NE, CE, and G-NC were LR, LR, and RF (supplementary Table 3). The prediction performance of radiomics models was described in Table 2. Regardless of which test set, using CE-CT for prediction yielded higher performance. Compared with NE and CE, G-NC had the best prediction performance in both two test sets. In particular, when NE-CT was used for prediction in the test set 1, the performance improvement was significant (AUC: 0.656 vs. 0.730; p < 0.05).
Diagnostic performance measurements
As shown in Table 3 and Fig. 3, a multivariate logistic regression was used to develop an individualized prediction nomogram. Table 4 indicated the prediction performance of nomogram when different types of CT were used for prediction in the two test sets. In the test set 1, the AUCs of the nomogram were 0.833 and 0.842 (accuracy: 0.727 and 0.758; sensitivity: 0.784 and 0.784; specificity: 0.655 and 0.724) for NE-CT and CE-CT, respectively. In the test set 2, the AUCs of the nomogram were 0.839 and 0.850 on NE-CT and CE-CT, respectively. The accuracy, sensitivity, and specificity have the same values for NE-CT and CE-CT (accuracy: 0.842; sensitivity: 0.889; specificity: 0.800).
ROC curves of nomogram on the two test sets are shown in Fig. 4. On test set 1, when using NE-CT to predict, the nomogram is significantly outperformed than NE model (p = 0.013), and it is also significantly outperformed than G-NC model (p = 0.027). When using CE-CT to predict, there is no significant difference between the nomogram and the CE model, nor the G-NC model. On test set 2, no matter whether NE-CT or CE-CT is used for prediction, there is no significant difference between nomogram and other models. The correlation was used to evaluate the consistency of the nomogram in predicting NE-CT and CE-CT of the same subject. The correlation coefficients were 0.772 and 0.660 in the test set 1 and test set 2, respectively.
Clinical use
The decision curve shown in Fig. 5 was used to evaluate the benefits of the nomogram using NE-CT (blue line) to predict and using CE-CT to predict (orange line). On the test set 1, for threshold probabilities > 70%, nomogram using NE-CT to predict added most benefit. On test set 2, for threshold probabilities < 70%, nomogram using CE-CT to predict added most benefit. On both two test sets, at any given threshold probability, using nomogram for prediction added more or equal benefit than using the “All-EGFR-TKIs therapy” and “None-EGFR-TKIs therapy.”
Decision curve analysis (DCA). The y axis represents the net benefit, which was determined by calculating the difference between the expected benefit and the expected harm associated with each proposed model [net benefit = true-positive rate (TPR) – (false-positive rate (FPR) × weighting factor), where the weighting factor = threshold probability/ (1-threshold probability)]. The green line represents the assumption that all patients with EGFR-TKIs therapy. The dotted line represents the assumption that all patients without EGFR-TKIs therapy. a DCA for the nomogram using NE-CT and CE-CT in test set 1. For threshold probabilities > 70%, nomogram using NE-CT to predict added more benefit than using CE-CT. b DCA for the nomogram using NE-CT and CE-CT in test set 2. For threshold probabilities < 70%, nomogram using CE-CT to predict added more benefit than using NE-CT. On both two test sets, using nomogram for prediction added more or equal benefit than using the “all-EGFR-TKIs therapy” and “none-EGFR-TKIs therapy” at any given threshold probability
Discussion
Radiomics can transform any type of medical images into quantitative data to aid in diagnosis and treatment, such as identifying EGFR mutant status. Most radiomics signatures are based on a single type of medical image, which limits the scope of their use due to uncertain image type accessibility for patients in clinical practice. Moreover, using only one type of medical image for radiomics signature development cannot tap into the advantages of other existing types of data. In this study, we proposed a method to construct radiomics signature using multiple types of CT all at once. Both our proposed general radiomics signature and nomogram could be directly applied on patient’s NE-CT or CE-CT, which further expand its scope of clinical application. The detection of EGFR mutation status could potentially guide physicians to treat patients with distinct therapeutic strategies. Currently, radiomics features extracted from NE-CT or CE-CT are widely used in the literature. Previous studies revealed that radiomics signature based on NE-CT had good performance in EGFR mutation detection with AUC of 0.796 in the test set 1 and on CE-CT showed an accuracy of 0.755 and a sensitivity of 0.929 [9]. Another study showed the performance of the radiomics signature based on CE-CT was better than that based on NE-CT, but not statistically significant [10]. Inspired by Kakino R et al. [11], we hypothesized that both NE-CT and CE-CT-based radiomics signatures can detect EGFR mutation. The experimental results on two test sets showed that the general radiomics signature can be applied to NE-CT and CE-CT, and the performance was better than the radiomics signature based on a single type of CT (NE and CE). Especially for NE-CT, a significant performance improvement has been obtained.
The data size of the training set for build general radiomics signature was twice the data size of training sets for building NE and CE, which may lead to incomparable performance. However, we argue that this is one of the benefits that our proposed general feature could bring, that is, we could utilize more data in a real-world clinical setting by merging different CT types, thereby improving the performance of the model. We conducted an ablation experiment to prove that the performance improvement was not only due to the increase of data size, but also because of the rational use of data (supplementary). On the one hand, directly mixing NE-CT and CE-CT for modeling did not lead to performance improvement. On the other hand, even under the same amount of data, general radiomics signature had the best performance.
Radiomics is not the only non-invasive detection method for EGFR mutations. Previously, physicians and radiologists tried to predict EGFR mutations through clinical and conventional CT characteristics, which were easily collected and would not be influenced by different CT types. Our findings are consistent with those of most existing studies that EGFR mutations were associated with female, non-smokers, and adenocarcinoma histology [21, 22]. Furthermore, the rate of EGFR mutations in NSCLC with COPD was significantly lower than that in NSCLC patients without COPD, in line with previous findings [23]. Past studies found that the radiological characteristics of maximum diameter, location, density, lymphadenopathy, spiculation, vacuole sign, air bronchograms, pleura retraction, emphysema, and fibrosis were associated with EGFR mutation status [24, 25]. Consistent with these studies, we found smaller size, GGO, bronchiectasis, lymphadenopathy, obstructive pneumonia and absence of emphysema, and fibrosis were more likely to be associated with tumors with EGFR mutations, despite insignificant difference in density, bronchiectasis, lymphadenopathy, and obstructive pneumonia. We also found that the frequency of EGFR mutations was higher in patients without emphysema or fibrosis than patients with emphysema or fibrosis, which is consistent with results of a prior study [26]. This indicates that CT findings of emphysema or fibrosis may be possible to predict the presence of EGFR mutations. Thus, a natural step forward is to combine radiomics signature and clinical or radiological characteristics to improve the accuracy of EGFR mutation status.
For this purpose, we constructed the general radiomics nomogram, which consists of general radiomics signature, smoking history, emphysema, and ILD. The result on the both two test sets indicated that regardless of whether NE-CT or CE-CT was used for prediction, the predictive performance of nomogram outperformed that of general radiomics signature and other models. The result of correlation matrix indicated that the nomogram predictions had good correlation for using CE-CT and NE-CT of the same subject on the test set 1 and test set 2. The decision curve analysis also showed that the nomogram added more or equal benefits than using the “All-EGFR-TKIs therapy” and “None-EGFR-TKIs therapy” at any given threshold probability.
There are several limitations in this study. First, this is a retrospective and single-center study. Although we constructed a time-based testing set in this study, external validation with more samples from other institutions is needed. Second, we divided all patients with both NE-CT and CE-CT into the test set, which may introduce sample selection bias. Third, our general feature selection method may filter out some radiomics features that may benefit the prediction of EGFR mutation status. In this study, we found that the feature groups used to establish NE and CE both contained wavelet-LHH_ngtdm_Strength. However, this feature was significantly different between NE-CT and CE-CT, and therefore excluded in general radiomics signature building process. It is necessary to develop a comprehensive method to use the radiomics features extracted from NE-CT and CE-CT more effectively in the future.
In conclusion, the general radiomics signature built jointly based on NE-CT and CE-CT has a good performance for EGFR mutation status prediction. Additionally, the nomogram consisting of radiomics, clinical, and radiological characteristics showed the most optimal predictive ability. Both general radiomics signature and nomogram are applicable to both NE-CT and CE-CT. Therefore, in clinical practice, the nomogram could be a potential non-invasive tool for EGFR mutation status detection.
Abbreviations
- ATS:
-
American Thoracic Society
- CI:
-
95% Confidence interval
- CT:
-
Computed tomography
- CE-CT:
-
Contrast-enhanced CT
- COPD:
-
Chronic obstructive pulmonary disease
- DICOM:
-
Digital Imaging and Communications in Medicine
- EGFR:
-
Epidermal growth factor receptor
- EGFR-TKI:
-
EGFR tyrosine kinase inhibitors
- ERS:
-
European Respiratory Society
- GBDT:
-
Gradient boosting decision tree
- G-NC:
-
General NE-CT and CE-CT
- ICC:
-
Intraclass correlation coefficient
- ILD:
-
Interstitial lung disease
- LR:
-
Logistic regression
- NBC:
-
Naive Bayesian classification
- NE-CT:
-
Non-contrast-enhanced CT
- NSCLC:
-
Non-small-cell lung cancer
- PACS:
-
Picture Achiving and Communication System
- RF:
-
Random forest
- SVM:
-
Support Vector machine
- VOI:
-
Volume of interest
References
Minguet J, Smith KH, Bramlage P (2016) Targeted therapies for treatment of non-small cell lung cancer-recent advances and future perspectives. Int J Cancer 138(11):2549–2561
Roskoski R Jr (2019) Small molecule inhibitors targeting the EGFR/ErbB family of protein-tyrosine kinases in human cancers. Pharmacol Res 139:395–411
Ramalingam SS, Vansteenkiste J, Planchard D et al (2020) Overall survival with osimertinib in untreated, EGFR-mutated advanced NSCLC. N Engl J Med 382(1):41–50
Lambin P, Rios-Velazquez E, Leijenaar R et al (2012) Radiomics: extracting more information from medical images using advanced feature analysis. Eur J Cancer 48(4):441–446
Gillies RJ, Kinahan PE, Hricak H (2016) Radiomics: images are more than pictures, they are data. Radiology 278(2):563–577
Rossi G, Barabino E, Fedeli A et al (2021) Radiomic detection of EGFR mutations in NSCLC. Cancer Res 81(3):724–731
Zhang G, Cao Y, Zhang J et al (2021) Predicting EGFR mutation status in lung adenocarcinoma: development and validation of a computed tomography-based radiomics signature. Am J Cancer Res 11(2):546–560
Dang Y, Wang R, Qian K, Lu J, Zhang H, Zhang Y (2021) Clinical and radiological predictors of epidermal growth factor receptor mutation in nonsmall cell lung cancer. J Appl Clin Med Phys 22(1):271–280
Liu G, Xu Z, Ge Y et al (2020) 3D radiomics predicts EGFR mutation, exon-19 deletion and exon-21 L858R mutation in lung adenocarcinoma. Transl Lung Cancer Res 9(4):1212–1224
Hong D, Xu K, Zhang L, Wan X, Guo Y (2020) Radiomics signature as a predictive factor for EGFR mutations in advanced lung adenocarcinoma. Front Oncol 10:28
Kakino R, Nakamura M, Mitsuyoshi T et al (2020) Comparison of radiomic features in diagnostic CT images with and without contrast enhancement in the delayed phase for NSCLC patients. Phys Med 69:176–182
Asano H, Toyooka S, Tokumo M et al (2006) Detection of EGFR gene mutation in lung cancer by mutant-enriched polymerase chain reaction assay. Clin Cancer Res 12(1):43–48
Henschke CI, Yankelevitz DF, Mirtcheva R et al (2002) CT screening for lung cancer: frequency and significance of part-solid and nonsolid nodules. AJR Am J Roentgenol 178(5):1053–1057
Travis WD, Costabel U, Hansell DM et al (2013) An official American Thoracic Society/European Respiratory Society statement: update of the international multidisciplinary classification of the idiopathic interstitial pneumonias. Am J Respir Crit Care Med 188(6):733–748
Soufi M, Arimura H, Nagami N (2018) Identification of optimal mother wavelets in survival prediction of lung cancer patients using wavelet decomposition-based radiomic features. Med Phys 45(11):5116–5128
van Griethuysen JJM, Fedorov A, Parmar C et al (2017) Computational radiomics system to decode the radiographic phenotype. Cancer Res 77(21):e104–e107
Lu X, Li M, Zhang H et al (2020) A novel radiomic nomogram for predicting epidermal growth factor receptor mutation in peripheral lung adenocarcinoma. Phys Med Biol 65(5):055012
Vickers AJ, Elkin EB (2006) Decision curve analysis: a novel method for evaluating prediction models. Med Decis Making 26(6):565–574
DeLong ER, DeLong DM, Clarke-Pearson DL (1988) Comparing the areas under two or more correlated receiver operating characteristic curves: a nonparametric approach. Biometrics 44(3):837–845
Wu J, Sun X, Wang J et al (2017) Identifying relations between imaging phenotypes and molecular subtypes of breast cancer: model discovery and external validation. J Magn Reson Imaging 46(4):1017–1027
Zou J, Lv T, Zhu S et al (2017) Computed tomography and clinical features associated with epidermal growth factor receptor mutation status in stage I/II lung adenocarcinoma. Thorac Cancer 8(3):260–270
Yotsukura M, Yasuda H, Shigenobu T et al (2017) Clinical and pathological characteristics of EGFR mutation in operable early-stage lung adenocarcinoma. Lung Cancer 109:45–51
Lim JU, Yeo CD, Rhee CK et al (2015) Chronic obstructive pulmonary disease-related non-small-cell lung cancer exhibits a low prevalence of EGFR and ALK driver mutations. PLoS One 10(11):e0142306
Shiri I, Maleki H, Hajianfar G et al (2020) Next-generation radiogenomics sequencing for prediction of EGFR and KRAS mutation status in NSCLC patients using multimodal imaging and machine learning algorithms. Mol Imaging Biol 22(4):1132–1148
Rizzo S, Petrella F, Buscarino V et al (2016) CT radiogenomic characterization of EGFR, K-RAS, and ALK mutations in non-small cell lung cancer. Eur Radiol 26(1):32–42
Usui K, Ushijima T, Tanaka Y et al (2011) The frequency of epidermal growth factor receptor mutation of nonsmall cell lung cancer according to the underlying pulmonary diseases. Pulm Med 2011:290132
Funding
This work is supported by Chinese Academy of Medical Sciences, Science and Technology Innovation in Medicine and Health Project (Major Collaborative Innovation Project 2018-I2M-1–0001), National Natural Science Foundation of China (No. 81870056, 81871328), and Beijing Science and Technology Commission Pharmaceutical and Technology Innovation Project Z181100001918034).
Author information
Authors and Affiliations
Corresponding authors
Ethics declarations
Guarantor
The scientific guarantor of this publication is Prof. Huaping Dai, MD.
Conflict of interest
The authors of this manuscript declare no relationships with any companies, whose products or services may be related to the subject matter of the article.
Statistics and biometry
No complex statistical methods were necessary for this paper.
Informed consent
Written informed consent was obtained from all patients or their family in this study.
Ethical approval
Institutional Review Board approval was obtained (Medical ethics number: 2019–123-K85-1).
Methodology
• Retrospective
• Diagnostic or prognostic study
• Performed at one institution
Additional information
Publisher's note
Springer Nature remains neutral with regard to jurisdictional claims in published maps and institutional affiliations.
Xiaoyan Yang and Min Liu contributed equally to this work.
Supplementary Information
Below is the link to the electronic supplementary material.
Rights and permissions
Open Access This article is licensed under a Creative Commons Attribution 4.0 International License, which permits use, sharing, adaptation, distribution and reproduction in any medium or format, as long as you give appropriate credit to the original author(s) and the source, provide a link to the Creative Commons licence, and indicate if changes were made. The images or other third party material in this article are included in the article’s Creative Commons licence, unless indicated otherwise in a credit line to the material. If material is not included in the article’s Creative Commons licence and your intended use is not permitted by statutory regulation or exceeds the permitted use, you will need to obtain permission directly from the copyright holder. To view a copy of this licence, visit http://creativecommons.org/licenses/by/4.0/.
About this article
Cite this article
Yang, X., Liu, M., Ren, Y. et al. Using contrast-enhanced CT and non-contrast-enhanced CT to predict EGFR mutation status in NSCLC patients—a radiomics nomogram analysis. Eur Radiol 32, 2693–2703 (2022). https://doi.org/10.1007/s00330-021-08366-y
Received:
Revised:
Accepted:
Published:
Issue Date:
DOI: https://doi.org/10.1007/s00330-021-08366-y