Abstract
Thyroid carcinoma (THCA) is the most common endocrine malignancy, and its incidence is increasing worldwide. Several studies have explored whether the tumor immune microenvironment and immune-related genes (IRGs) influence the prognosis of patients with THCA and can be used to predict the response to immune checkpoint inhibitors (ICIs). We developed an IRG prognostic/risk signature using a bioinformatics method, and its predictive capacity was validated in patients in the test set and the total set. Subsequently, we analyzed the correlation between this IRG prognostic signature and tumor-infiltrating immune cells, tumor mutation burden (TMB), and immune checkpoint protein expression in patients with THCA. With a multivariate analysis, the IRG prognostic signature, which comprised eight IRGs, was identified as an independent prognostic factor. High-risk patients had poor overall survival compared with low-risk patients. Plasma cells, monocytes, and dendritic cells infiltrated differently according to the IRG prognostic signature. The low-risk group had a higher TMB and immunophenoscore (IPS), which indicated a better response to ICIs. The qRT-PCR validated eight IRGs with differential expression in thyroid cancer and normal tissues. We conclude that the IRG prognostic signature may be a useful tool to predict survival and response to ICIs. However, further testing is required to assess the predictive capacity of this IRG prognostic signature.








Similar content being viewed by others
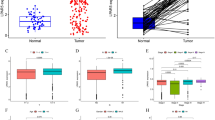
Data availability
Gene expression profiles, clinical information, and mutation data of THCA in this study are available from the public database (TCGA, https://portal.gdc.cancer.gov/). The IPS values are downloaded from The Cancer Immunome Atlas (TCIA, https://tcia.at/home). The immune- related genes are acquired from the Immunology Database and Analysis Portal database (ImmPort, https://immport.niaid.nih.gov).
References
Coelho M et al (2020) The potential of metabolomics in the diagnosis of thyroid cancer. Int J Mol Sci. https://doi.org/10.3390/ijms21155272
Bray F et al (2018) Global cancer statistics 2018: GLOBOCAN estimates of incidence and mortality worldwide for 36 cancers in 185 countries. CA Cancer J Clin 68:394–424. https://doi.org/10.3322/caac.21492
Cabanillas ME, McFadden DG, Durante C (2016) Thyroid cancer. The Lancet 388:2783–2795. https://doi.org/10.1016/s0140-6736(16)30172-6
Schneider DF, Chen H (2013) New developments in the diagnosis and treatment of thyroid cancer. CA Cancer J Clin 63:374–394. https://doi.org/10.3322/caac.21195
Gupta S et al (2020) International trends in the incidence of cancer among adolescents and young adults. J Natl Cancer Inst 112:1105–1117. https://doi.org/10.1093/jnci/djaa007
Morris LG, Tuttle RM, Davies L (2016) Changing trends in the incidence of thyroid cancer in the United States. JAMA Otolaryngol Head Neck Surg 142:709–711. https://doi.org/10.1001/jamaoto.2016.0230
Xing M (2013) Molecular pathogenesis and mechanisms of thyroid cancer. Nat Rev Cancer 13:184–199. https://doi.org/10.1038/nrc3431
Albero A, Lopez JE, Torres A, de la Cruz L, Martin T (2016) Effectiveness of chemotherapy in advanced differentiated thyroid cancer: a systematic review. Endocr Relat Cancer 23:R71-84. https://doi.org/10.1530/ERC-15-0194
Schachter J et al (2017) Pembrolizumab versus ipilimumab for advanced melanoma: final overall survival results of a multicentre, randomised, open-label phase 3 study (KEYNOTE-006). Lancet 390:1853–1862. https://doi.org/10.1016/S0140-6736(17)31601-X
Mehnert JM et al (2019) Safety and antitumor activity of the anti-PD-1 antibody pembrolizumab in patients with advanced, PD-L1-positive papillary or follicular thyroid cancer. BMC Cancer 19:196. https://doi.org/10.1186/s12885-019-5380-3
Yi M et al (2021) Immune signature-based risk stratification and prediction of immune checkpoint inhibitor’s efficacy for lung adenocarcinoma. Cancer Immunol Immunother. https://doi.org/10.1007/s00262-020-02817-z
Xu Y, Wang Z, Li F (2021) Survival prediction and response to immune checkpoint inhibitors: a prognostic immune signature for hepatocellular carcinoma. Transl Oncol 14:100957. https://doi.org/10.1016/j.tranon.2020.100957
Liu J et al (2020) Exploration of a novel prognostic risk signatures and immune checkpoint molecules in endometrial carcinoma microenvironment. Genomics 112:3117–3134. https://doi.org/10.1016/j.ygeno.2020.05.022
Liu J et al (2020) Identification of a prognostic signature of epithelial ovarian cancer based on tumor immune microenvironment exploration. Genomics 112:4827–4841. https://doi.org/10.1016/j.ygeno.2020.08.027
Yang S et al (2019) Identification of a prognostic immune signature for cervical cancer to predict survival and response to immune checkpoint inhibitors. Oncoimmunology 8:e1659094. https://doi.org/10.1080/2162402X.2019.1659094
Colaprico A et al (2016) TCGAbiolinks: an R/Bioconductor package for integrative analysis of TCGA data. Nucleic Acids Res 44:e71. https://doi.org/10.1093/nar/gkv1507
Bhattacharya S et al (2014) ImmPort: disseminating data to the public for the future of immunology. Immunol Res 58:234–239. https://doi.org/10.1007/s12026-014-8516-1
Robinson MD, McCarthy DJ, Smyth GK (2010) edgeR: a Bioconductor package for differential expression analysis of digital gene expression data. Bioinformatics 26:139–140. https://doi.org/10.1093/bioinformatics/btp616
Yu G, Wang LG, Han Y, He QY (2012) clusterProfiler: an R package for comparing biological themes among gene clusters. OMICS 16:284–287. https://doi.org/10.1089/omi.2011.0118
Simon N, Friedman J, Hastie T, Tibshirani R (2011) Regularization paths for cox’s proportional hazards model via coordinate descent. J Stat Softw 39:1–13. https://doi.org/10.18637/jss.v039.i05
Newman AM et al (2015) Robust enumeration of cell subsets from tissue expression profiles. Nat Methods 12:453–457. https://doi.org/10.1038/nmeth.3337
Mayakonda A, Lin DC, Assenov Y, Plass C, Koeffler HP (2018) Maftools: efficient and comprehensive analysis of somatic variants in cancer. Genome Res 28:1747–1756. https://doi.org/10.1101/gr.239244.118
Robinson DR et al (2017) Integrative clinical genomics of metastatic cancer. Nature 548:297–303. https://doi.org/10.1038/nature23306
Charoentong P et al (2017) Pan-cancer immunogenomic analyses reveal genotype-immunophenotype relationships and predictors of response to checkpoint blockade. Cell Rep 18:248–262. https://doi.org/10.1016/j.celrep.2016.12.019
Yu J et al (2020) Hierarchical clustering of cutaneous melanoma based on immunogenomic profiling. Front Oncol 10:580029. https://doi.org/10.3389/fonc.2020.580029
Rodriguez-Cerdeira C et al (2017) Advances in immunotherapy for melanoma: a comprehensive review. Mediators Inflamm 2017:3264217. https://doi.org/10.1155/2017/3264217
Steven A, Fisher SA, Robinson BW (2016) Immunotherapy for lung cancer. Respirology 21:821–833. https://doi.org/10.1111/resp.12789
Riley RS, June CH, Langer R, Mitchell MJ (2019) Delivery technologies for cancer immunotherapy. Nat Rev Drug Discov 18:175–196. https://doi.org/10.1038/s41573-018-0006-z
Anania MC et al (2013) S100A11 overexpression contributes to the malignant phenotype of papillary thyroid carcinoma. J Clin Endocrinol Metab 98:E1591-1600. https://doi.org/10.1210/jc.2013-1652
Sung AK, Lee KH (2018) HGF-mediated S100A11 overexpression enhances proliferation and invasion of gastric cancer. Am J Transl Res 10(11):3385
Lv XJ et al (2021) Aberrant expression of semaphorin 6B affects cell phenotypes in thyroid carcinoma by activating the Notch signalling pathway. Endokrynol Pol 72:29–36. https://doi.org/10.5603/EP.a2020.0072
Zheng J, Li H, Xu D, Zhu H (2017) Upregulation of tyrosine kinase fyn in human thyroid carcinoma: role in modulating tumor cell proliferation, invasion, and migration. Cancer Biother Radiopharm 32:320–326. https://doi.org/10.1089/cbr.2017.2218
Yu B et al (2020) FYN is required for ARHGEF16 to promote proliferation and migration in colon cancer cells. Cell Death Dis 11:652. https://doi.org/10.1038/s41419-020-02830-1
Yang Z et al (2015) Upregulation of heat shock proteins (HSPA12A, HSP90B1, HSPA4, HSPA5 and HSPA6) in tumour tissues is associated with poor outcomes from HBV-related early-stage hepatocellular carcinoma. Int J Med Sci 12:256–263. https://doi.org/10.7150/ijms.10735
Shin SS et al (2017) HSPA6 augments garlic extract-induced inhibition of proliferation, migration, and invasion of bladder cancer EJ cells; Implication for cell cycle dysregulation, signaling pathway alteration, and transcription factor-associated MMP-9 regulation. PLoS ONE 12:e0171860. https://doi.org/10.1371/journal.pone.0171860
Su C et al (2019) Identification of plasma RGS18 and PPBP mRNAs as potential biomarkers for gastric cancer using transcriptome arrays. Oncol Lett 17:247–255. https://doi.org/10.3892/ol.2018.9608
McCabe D, Cukierman T, Gabay JE (2002) Basic residues in azurocidin/HBP contribute to both heparin binding and antimicrobial activity. J Biol Chem 277:27477–27488. https://doi.org/10.1074/jbc.M201586200
Gao W et al (2021) IL20RA signaling enhances stemness and promotes the formation of an immunosuppressive microenvironment in breast cancer. Theranostics 11:2564–2580. https://doi.org/10.7150/thno.45280
Yi M et al (2018) Biomarkers for predicting efficacy of PD-1/PD-L1 inhibitors. Mol Cancer 17:129. https://doi.org/10.1186/s12943-018-0864-3
Acknowledgements
We appreciate the linguistic assistance provided by TopEdit (www.topeditsci.com) during the preparation of this manuscript.
Funding
This work was supported by the National Natural Science Foundation of China (81902726), China Postdoctoral Science Foundation (2018M641739).
Author information
Authors and Affiliations
Contributions
WP performed the data analysis and wrote the manuscript. SW and ZH reviewed and revised the manuscript. All authors read and approved the final manuscript.
Corresponding author
Ethics declarations
Conflict of interest
The authors declare that they have no conflict of interest.
Ethics approval
The research was approved by the Institutional Research Ethics Committees of the First Affiliated Hospital of China Medical University. Informed consent for publication was obtained from all patients for collection of tissue samples prior to the surgery.
Additional information
Publisher's Note
Springer Nature remains neutral with regard to jurisdictional claims in published maps and institutional affiliations.
Supplementary Information
Below is the link to the electronic supplementary material.
Rights and permissions
About this article
Cite this article
Wu, P., Sun, W. & Zhang, H. An immune-related prognostic signature for thyroid carcinoma to predict survival and response to immune checkpoint inhibitors. Cancer Immunol Immunother 71, 747–759 (2022). https://doi.org/10.1007/s00262-021-03020-4
Received:
Accepted:
Published:
Issue Date:
DOI: https://doi.org/10.1007/s00262-021-03020-4