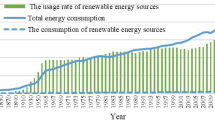
Overview
- Is the first textbook on load forecasting for the distribution network
- Brings together both statistical and machine learning topics
- Includes colorful illustrations and practical examples from many sectors and developing countries
- Is open access, which means that you have free and unlimited access
Buy print copy
Tax calculation will be finalised at checkout
About this book
This comprehensive open access book enables readers to discover the essential techniques for load forecasting in electricity networks, particularly for active distribution networks.
From statistical methods to deep learning and probabilistic approaches, the book covers a wide range of techniques and includes real-world applications and a worked examples using actual electricity data (including an example implemented through shared code). Advanced topics for further research are also included, as well as a detailed appendix on where to find data and additional reading. As the smart grid and low carbon economy continue to evolve, the proper development of forecasting methods is vital.
This book is a must-read for students, industry professionals, and anyone interested in forecasting for smart control applications, demand-side response, energy markets, and renewable utilization.
Similar content being viewed by others
Keywords
Table of contents (15 chapters)
Authors and Affiliations
About the authors
Stephen Haben has been working in forecasting and data analytics for over 15 years. His Ph.D. was in Data Assimilation for numerical weather prediction which was supported with the UK’s meteorological office (Met Office). From 2011, Stephen worked on and led the academic team on the Thames Valley Vision project, a low carbon network fund project where the aim was to investigate new technologies and solutions which could help support the future low carbon economy. This included investigating forecasting methods for low-voltage networks, integrating the forecasts within storage control algorithms, modelling consumer demand, simulating low-voltage network demand and producing long-term scenario forecasts to understand the impact of high uptakes of low carbon technologies such as electric vehicles, PV and heat pumps. Since 2018, Stephen has worked as a data scientist and then digital and data consultant at Energy Systems Catapult, a not-for-profit, independent technology and innovationcentre which supports innovators in the energy sector and aims to help bridge the gap between academia, industry and government. He continues to research low voltage forecasting methods and energy analytics as part of his visiting research position at the Mathematical Institute at the University of Oxford.
Professor William Holderbaum is a professor of Mathematics and Engineering at the University of Reading in the UK. He has played major leadership roles in research, while maintaining a very strong international reputation and an extensive list of industrial collaborations. Over the years, he has applied his control and mathematical modelling expertise to several applications and in particular energy transmission, storage for electrical systems and power systems. In 2011 he was involved in the Thames Valley Vision (TVV) project, a £30M low carbon network fund project. The aim of the projects is to assist distribution network operators in the UK to prepare for the future low carbon economy by testing various technological and operational solutions. Furthermore, he led a Climate/KIC EU-funded project “Susports: delivering sustainable energy solutions to ports” which had the aim of reducing greenhouse gases emissions in ports using data analysis and forecasts of energy flows to eventually develop a system which will reduce fuel consumption and will enable energy recovery using energy storage devices and innovative control techniques.
Marcus Voss has been working at the intersection of machine learning, climate change, the energy transition and sustainability for over 10 years. He is an AI Expert and Intelligence Architect at Birds on Mars, where he is responsible for AI applications for advancing sustainability. He serves on the board of directors at Climate Change AI, an international NPO catalysing impactful work at the intersection of climate change and machine learning. There he is the Community Lead for buildings and transportation. He has been anexternal lecturer on AI and data science, for instance, at TU Berlin, Leuphana University LĂĽneburg, and CODE University. Previously, Marcus Voss was a Research Associate at TU Berlin, where he led the Smart Energy Systems research group of the DAI-Labor, working on AI applications in the smart grid and the sustainable development of AI systems. In his doctoral research, he has been working on data analysis of low voltage-level smart meter data with a focus on load forecasting using machine learning methods.
Bibliographic Information
Book Title: Core Concepts and Methods in Load Forecasting
Book Subtitle: With Applications in Distribution Networks
Authors: Stephen Haben, Marcus Voss, William Holderbaum
DOI: https://doi.org/10.1007/978-3-031-27852-5
Publisher: Springer Cham
eBook Packages: Energy, Energy (R0)
Copyright Information: The Editor(s) (if applicable) and The Author(s) 2023
Hardcover ISBN: 978-3-031-27851-8Published: 01 May 2023
Softcover ISBN: 978-3-031-27854-9Published: 02 May 2024
eBook ISBN: 978-3-031-27852-5Published: 30 April 2023
Edition Number: 1
Number of Pages: XV, 331
Number of Illustrations: 50 b/w illustrations, 89 illustrations in colour
Topics: Energy Systems, Probability Theory and Stochastic Processes, Neurosciences, Energy Storage, Control, Robotics, Mechatronics, Control, Robotics, Mechatronics