Abstract
Background
Mosquito control is needed to prevent dengue fever, which is mainly spread by Aedes albopictus in China. Application of insecticides is one of the main mosquito control methods; however, this approach can fail due to the knockdown resistance (kdr) gene mutation that causes decreased sensitivity to insecticides in Ae. albopictus. The kdr mutation patterns among different regions in China differ significantly. However, the underlying mechanism and factors that influence kdr mutation remain unclear. To explore the potential influence of genetic background on the development of insecticide resistance in Ae. albopictus, we analyzed the genetic structure of Ae. albopictus populations in China and its correlation with major kdr mutations.
Methods
We collected Ae. albopictus from 17 sites in 11 provinces (municipalities) across China from 2016 to 2021 and extracted the genomic DNA from individual adult mosquitoes. We selected eight microsatellite loci for genotyping, and based on microsatellite scores, we estimated intraspecific genetic diversity, population structure, and effective population size. The association between the intrapopulation genetic variation and F1534 mutation rate was evaluated by the Pearson correlation coefficient.
Results
Based on variation analysis of the microsatellite loci of 453 mosquitoes representing 17 populations throughout China, more than 90% of the variation occurred within individuals, whereas only about 9% of the variation occurred among populations, indicating that field populations of Ae. albopictus are highly polymorphic. The northern populations tended to belong to gene pool I (BJFT 60.4%, SXXA 58.4%, SDJN 56.1%, SXYC 46.8%), the eastern populations tended to belong to pool III (SH 49.5%, JZHZ 48.1%), and the southern populations tended to belong to three different gene pools. Moreover, we observed that the greater the fixation index (FST), the lower the wild-type frequency of F1534 of VSGC.
Conclusions
The degree of genetic differentiation among Ae. albopictus populations in China was low. These populations were divided into three gene pools, in which the northern and eastern pools are relatively homogeneous, while the southern gene pool is heterogeneous. The possible correlation between its genetic variations and kdr mutations is also noteworthy.
Graphical Abstract

Similar content being viewed by others
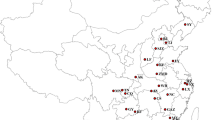
Background
Dengue fever has become a public health emergency in China. In 2019, over 22,000 dengue cases were reported, and since then, the distribution of the disease has been significantly expanding [1, 2]. Aedes albopictus is widely distributed in China, where it can be found from Liaoning Province in the north to Hainan Province in the south, an area that includes tropical, subtropical, and temperate climate zones [3]. Ae. albopictus is the main vector of dengue fever in China due to its abundance and wide distribution [4].
Mosquito control is an important component of the integrated programs designed to prevent dengue fever transmission, and insecticide spraying is one of the most commonly used methods of mosquito control. This method is simple and quickly effective, thus it has been a critical component of emergency control of mosquito-borne diseases, including dengue fever. Because of its importance in the prevention and control of mosquito-borne infectious diseases, the development of insecticide resistance in mosquitoes has become a focal issue. The mechanisms of insecticide resistance in mosquitoes include behavioral resistance, target insensitivity, and metabolic detoxification, with target insensitivity being considered the main mechanism of insecticide resistance in Ae. albopictus. The well-known knockdown resistance (kdr) is caused by mutations in the voltage-gated sodium cannel (VGSC) gene, which encodes the target site of pyrethroids [5]. The types of kdr mutations found in field populations of Ae. albopictus include F1534S, F1534C, I1532T, and V1016G. In China, the mutation rate of kdr in field populations of Ae. albopictus is extremely high, indicating that it undergoes rapid evolution.
The origin of insecticide resistance in mosquitoes has long been a topic of interest. There is evidence indicating that mosquito population structure and insecticide resistance are two mutually influencing factors contributing to insecticide resistance. Chang et al. demonstrated the impact of insecticide selection factors on the population structure of Anopheles sinensis in China [6]. Rahman et al. also observed prevalent kdr mutations, low genetic architecture, and frequent migration in Ae. aegypti populations in Rio de Janeiro, Brazil [7]. In our previous study, we found that the kdr mutation pattern of the Ae. albopictus population in China is correlated with the average annual temperature at the collection site [8]. The research showed that Ae. albopictus populations in tropical, subtropical, and temperate regions of China have different genetic structure characteristics [9]. These studies have given rise to our interest in exploring the association between population genetic structure and insecticide resistance in Ae. albopictus.
Previous research on the molecular population genetic structure of Ae. albopictus has mainly focused on its invasion and migration routes [10,11,12,13]. These studies have applied the following molecular markers: allozyme, random amplified polymorphic DNA [14], NADH dehydrogenase subunit 5 [15,16,17], cytochrome c oxidase subunit I (COI) [18,19,20,21,22], cytochrome B [23] of mitochondrial DNA, ribosomal DNA ITS2 [24], and microsatellite DNA [9, 10, 25,26,27,28]. Analysis of COI sequences and nuclear microsatellites in Ae. albopictus populations found substantial intermixing between Papua New Guinea’s southern Fly region and Torres Strait Island, which has been attributed to human sea traffic shuttling this mosquito between the two sites; and this extensive movement may well compromise Ae. albopictus eradication attempts in this region [11]. In Vietnam, the COI haplotype grouping of Ae. albopictus found geographically distributed populations, resulting in a distinct population structure in which northeastern populations and the remaining populations were genetically differentiated [20]. Microsatellite DNA analysis of Ae. albopictus populations in East Asia, the Indian Ocean, the Mediterranean Basin, the Pacific Ocean, and North America suggests that independent and transcontinental introductions may have facilitated the rapid establishment of adventive populations through the admixture of unrelated genomes. This study found a high level of intrapopulation variability, and this variability may extend to the genetic mechanisms controlling vector competence [10].
The population genetic structure of Ae. albopictus in some areas of China has been studied using molecular markers [9, 10, 29], and COI analysis indicates that gene flow occurs commonly among 15 populations in Guangzhou, Guangdong; moreover, the analysis found no genetic differentiation nor differences in genetic diversities among populations [30, 31]. In contrast, there was an obvious genetic differentiation between Ae. albopictus in Hainan and a few samples collected from 10 provinces in China [32]. These studies indicate the need to more fully study the genetic structure of Ae. albopictus populations in China, and the relationship between genetic structure and insecticide resistance development also needs to be investigated.
In this study, we analyzed the genetic structure of Ae. albopictus in China by collecting samples from 17 field populations located in regions ranging from temperate to tropical. We analyzed the population genetic structure of Ae. albopictus based on based on 8 polymorphic microsatellite loci. We then analyzed the correlation between genetic variations within Ae. albopictus populations and kdr mutations. The main objectives of this study were to estimate the degree of genetic differences among Ae. albopictus populations in China; and to analyze the factors influencing insecticide resistance for the development of vector control strategies.
Methods
Sample collection and species identification
To encompass the distribution range of Ae. albopictus in China, we selected 17 sample collection sites within 11 provinces (municipalities) situated from the north to south of China. These sites include temperate, subtropical, and tropical climate regions. Samples were collected from 2016 to 2021 (Additional file 1: Table S1) by scooping larvae and pupae in breeding sites (more than 8 breeding sites in a collection site), and then bringing these back to the laboratory for rearing to adults under standard conditions [26 ± 1 °C, 65 ± 5% relative humidity, photoperiod of 12/12 h (light/dark)]. Adult mosquitoes in outdoor environments were collected using aspirators, BGtraps (Biogents AG, Regensburg, Germany), or light traps (Houji Dianzi, Dongguan, China). Details on sample collection are summarized in Additional file 1: Table S1 and Figure S2. Species of adult Aedes mosquitoes were identified based on morphological characteristics [3] and confirmed by analysis of molecular markers [33].
Microsatellite genotyping
Genomic DNA was extracted from individual adult mosquitoes using the DNAzol reagent (Invitrogen, Thermo Fisher Scientific, Waltham, MA, USA) according to the manufacturer’s instructions. Nineteen microsatellite loci were initially selected from the literature [11, 34], and eight microsatellite loci were finally selected based on results of preliminary experiments on amplification efficiency and site polymorphism, and they consisted of Alb-di-4 (GenBank accession number: KF146971), Alb-di-6 (KF146972), Alb-tri-6 (KF146974), Alb-tri-41 (KF146983), Alb-tri-46 (KF146975), AealbA9 (DQ366022), AealbB52 (DQ366024), and AealbF3 (DQ366027) (Additional file 1: Table S2). Microsatellite loci were amplified according to detailed protocols described by Schuelke [34], with each PCR reaction containing three primers: a sequence-specific forward primer with an M13(-21) adapter (5′- TGT AAA ACG ACG GCC AGT -3′) conjugated to its 5′ end, a sequence-specific reverse primer, and a universal FAM-labeled M13(-21) primer. Amplified fragments were separated by capillary electrophoresis in an automatic sequencer (ABI 3770, Applied Biosystems, Foster City, CA) and fragment sizes were scored using GENOTYPER 3.7 software (Applied Biosystems, Foster City, CA).
Analysis of genetic differences within a population
Genetic variation within the population in each locality was estimated in terms of average numbers of alleles (na), the number of private alleles (np), and frequency of private alleles (Ap), which were obtained using GenAlEx 6.5 [35]. The average number of alleles and private alleles were also calculated at the individual level from the expressions na/n and np/n, respectively. The inbreeding index (FIS) was obtained using FSTAT V.2.9.3.2 [36]. Observed and expected heterozygosity and pairwise-FST [37] values were computed using Microsatellite Analyser V.4.05 [38]. The statistical significance of each FST value was assessed by comparing the observed value to the values obtained from 10,000 matrix permutations, followed by Bonferroni corrections. Linkage disequilibrium between pairs of loci in each sample (number of dememorizations 1000, 100 batches, 1000 iterations per batch) and deviations from Hardy–Weinberg equilibrium (HWE) at each locus/sample combination were examined with GENEPOP V. 4.2 [39], and the statistical significance was assessed following Bonferroni corrections [40]. The allelic polymorphic information content and null allele frequencies (An) for each locus were estimated using CERVUS [41]. The significance of the regression examining the relationship between genetic differences and the geographic distance between sample pairs was tested using a Mantel test [42] with 100,000 permutations in GENEPOP V. 4.2 [39].
Population genetic structure and demographic inference
The relationships among populations were assessed using Principal Coordinate Analysis in GenAlEx 6.5 [35]. Bayesian clustering analysis in STRUCTURE V 2.3.2 [43,44,45] was used to infer population genetic structure using the admixture model and assuming independent allele frequencies. The burn-in was set to 1,000,000 steps, followed by 1,000,000 Markov Chain Monte Carlo replications. All runs were repeated 20 times for each number of possible clusters (K), set between 1 and 16 (i.e., the number of samples). The appropriate number of genetic clusters was determined by plotting the log probability [L(K)] and ΔK across multiple runs [46] as implemented in STRUCTURE HARVESTER [47]. Finally, the programs CLUMPP [48] and DISTRUCT [49] were used to average replicate runs and to generate bar graphs of structure results, respectively. An analysis of molecular variance (AMOVA) was used to examine the distribution of genetic variation using GenAlEx 6.5. The longterm effective population size (Ne) was estimated using NEESTIMATOR 2.1 [50] based on the heterozygote excess and linkage disequilibrium methods.
Correlation between genetic variation within a population and kdr mutation
The FST value, representing the intrapopulation genetic variation, was estimated by GENEPOP V. 4.2[39]. Mutant F1534S is the most common type of kdr mutation in Ae. albopictus in China, and it is significantly associated with the pyrethroid resistance phenotype [51]. The frequency of F1534S was obtained from our previous publication [8], which studied the same populations as those in this study. The correlations between the frequency of F1534 wild-type and FST within 14 Ae. albopictus populations were evaluated by Pearson correlation analysis. The linear regression was implemented using the “ggpmisc” and “ggplot2” package of R 4.2.2 (R Foundation for Statistical Computing, Vienna, Austria. URL https://www.R-project.org).
Results
Intraspecific variation
The scores obtained from eight microsatellite loci in 453 mosquitoes collected from 17 localities across China, displayed different levels of polymorphism in terms of the number of alleles, which ranged from 7 (AealbB52) to 27 (AealbA9) alleles. The number of alleles per locus in each population is provided in Additional file 1: Table S3. Analysis of these loci yields a mean polymorphic information content of 0.57, suggesting that they are sufficiently informative for assessing the degree of population variability and structuring of the populations [52]. The results of Fisher's exact tests after sequential Bonferroni corrections reveal significant departures from HWE in 14 out of 120 population/locus combinations. However, these departures were not concentrated at any one locus or in any population. We observed no consistent pattern of linkage disequilibrium between any particular pair of loci; therefore, the 8 selected loci are independent and their variability likely reflects genome-wide patterns across populations.
These eight loci were considerably variable across the 17 populations (Table 1), and the GDST population displayed the highest level of variation with an HO of 0.57. The maximum mean number of alleles/individual was observed in the GDGZ population (na/n = 0.58). Private alleles were detected in all populations, except for the SH, JZHZ, GDST, and SXYC populations, with the highest proportion of private alleles found in HNSS (np/n = 0.31). Analysis of the variability distribution in and among the populations (AMOVA) showed that most variation (91%) occurred within individuals, whereas only about 9% of the total variation was detected among populations, indicating that the field populations of Ae. albopictus are highly polymorphic.
Population genetic structure
In the analysis of population genetic structure, GDGZ was excluded due to the low number of individuals (n = 10). Therefore, a total of 443 individuals in 16 populations were analyzed for population genetic diversity. Pairwise comparisons indicate FST values were significantly different from zero, with the lowest value (0.012) found between SH with JZHZ, while the highest value (0.167) was between GDST with STWS (Table 2). We found no correlation between genetic differentiation (FST) and geographical distance, which was confirmed by an R2 value of 0.029 (P = 0.785). All FST values within the population were greater than 0.1, except for that of GDST (0.087), with the range level from 0.271 (HNSS) to 0.582 (SH) (Table 2).
Results of Bayesian analysis implemented in STRUCTURE show that the proportion of individuals sharing coancestry in the 16 populations was not consistent with their geographic proximity. The natural logarithm of the likelihood of the data, i.e., ln (P(X/K)), increased from K = 1 to K = 3 and then gradually reached a plateau toward 16, suggesting that the genetic clustering of the 16 populations was 3 (Additional file 1: Figure S1). Individual mosquitoes from the 16 populations were then assigned to each of the 3 clusters with a certain probability value (Additional file 1: Figure S1, Table S4), and the pairwise and within cluster genetic differences (FST) were calculated. The FST values between cluster I and clusters II and III were 0.110 and 0.114, respectively, while that between clusters II and III was 0.027. The FST values within the three clusters were 0.124, 0.048, and 0.080, respectively.
The coancestry of the populations in southern China were heterogeneous: i.e., populations in Hainan were tightly related to cluster II (green) (HNSY 95.8%, HNSS 76.6%) and cluster III (blue) (HNHK 57.7%), while populations in Guangdong were mainly related to cluster III (blue) (GDST 0.928) and cluster I (red) (STWS 79.7%, GDZJ 71.9%, SZSK 58.5%). Populations in the eastern part of China were weakly related to cluster III (SH 49.5%, JZHZ 48.1%), and populations in the northern part of China were mainly related to cluster I (BJFT 60.4%, SXXA 58.4%, SDJN 56.1%, SXYC 46.8%) (Additional file 1: Figure S2; Table S4).
Effective population size
Estimates of longterm Ne varied considerably, depending on the method of calculation used. Using the heterozygote excess method resulted in all Ne estimates of infinity. Meanwhile, the linkage disequilibrium method, which used the lowest allele frequency of 0.01, yielded diverse Ne values across populations (Table 3). The effective sizes of the GDZJ and SH populations were infinite. However, the Ne value of the GDST population was 2.3 and this population’s wild-type frequency of F1534 was 100%.
Correlation between the intrapopulation variation and knockdown resistance mutations
Pearson correlation analysis of FST and the frequency of F1534 wild-type within 14 Ae. albopictus populations yielded a correlation coefficient of − 0.39 (P = 0.17), indicating no significant correlation. However, we did observe the following trend: the greater the genetic difference (FST), the lower the wild-type frequency of F1534 (Additional file 1: Figure S3). In the GDST population, codon 1534 in VGSC was entirely wild-type (100%), and the population had the lowest FST (0.087). Meanwhile, the ZJHZ population had the highest (91.55%) F1534 mutant allele frequency, and its FST value was also large (0.516) (Table 2).
Discussion
Ae. albopictus has long been considered of secondary importance as a vector for dengue transmission. However, this species is the most important vector in China because it is widespread, while the distribution of the typical primary vector, Ae. aegypti, is limited. Moreover, recent dengue outbreaks in China have been caused by Ae. albopictus [2]. Therefore, more knowledge on the genetic diversity and genetic structure of this mosquito species will help researchers evaluate the risk of disease transmission and insecticide resistance spread, as well as identify the origins and frequencies of introductions.
In this study, we analyzed the population genetics of Ae. albopictus collected from the different climate regions in China based on the 8 polymorphic microsatellite loci. Most (91%) of the genetic variation occurred within individuals, whereas only about 9% of the total variation was detected among populations. We found no relationship between genetic distance and geographical distance, and populations in the southern part of China tended to be more heterogeneous. Within a population, we observed that the greater the genetic difference (FST), the lower the wild-type frequency of F1534 of VSGC.
Sampling strategy and geographic coverage greatly influence the analysis and interpretation of the data generated from the samples. In this study, Ae. albopictus mosquitoes were collected from 17 sites in 11 provinces (municipalities), encompassing the main distribution range of Ae. albopictus in China (i.e., from 16°N to 40°N [4]), which includes tropical, subtropical, and temperate climate zones. In addition, it is crucial to clearly distinguish between the type of collected samples (i.e., eggs, larvae, or flying adults), because Ae. albopictus larvae or eggs collected from the same breeding site are likely to belong to the same progeny. We avoided this potential drawback by collecting a few individuals per breeding site and setting multiple traps throughout the sampling site.
The following are key features of useful genetic markers: selective neutrality, ease of scoring in all specimens of the species, and sufficient variability to allow for measuring genetic differentiation and genetic clustering of individuals. Previous studies have reported on more than 10 microsatellite DNA loci for Ae. albopictus populations, and we found eight of them were highly polymorphic and amplified efficiently, and these were used for exploring the population genetic structure of Ae. albopictus in China. Although their chromosomal locations are unknown, we observed no consistent pattern of linkage disequilibrium between any particular pair of loci, suggesting that they are at least statistically independent and are likely distributed genome-wide. However, in the GDGZ population, we were unable to amplify some microsatellite loci in many individuals, resulting in invalid data. The high amplification failure rate in GDGZ was unanticipated and may be due to inherent genetic differences in Ae. albopictus samples, which have been suggested in other studies [53] and are thought to influence dengue virus susceptibility [54].
We observed high allelic diversity and heterozygosity in most of the populations (HO = 0.32–0.57), which is similar to the levels of diversity in the Ae. albopictus population at the coastal areas in southern China (HO = 0.384–0.641) [25], in 17 populations from 3 climatic regions of China (HO = 0.467–0.627) [9], in populations from 34 localities across China (HO = 0.551–0.633) [29], and in eight samples from Thailand, Réunion, and Northern Italy (HO = 0.22–0.28). In China, Ae. albopictus occurs in different climate zones, with populations undergoing marked seasonal variations in abundance, in which high densities are reached only during the summer months. The high levels of genetic diversity suggest that Ae. albopictus is able to maintain a relatively large effective population size, despite the seasonal changes in temperature.
Notably, we observed the minimum FST value (0.087) in the GDST population, which carried no mutation in F1534 [8]. As this population is located close to seaside wharf, we speculate that the GDST population consists of mosquitoes that originated from ships and that colonized the local environment, which was suitable for the survival of Ae. albopictus. This population became abundant and because the time of colonization time may be recent or it may be derived from a single invasion, the genetic difference within the population is small, with 92.8% of the individuals belonging to cluster III. These attributes are consistent with the founder effect. Oral interviews revealed that almost no insecticides have been used in the GDST area, thus the alleles of F1534 are all wild-type. Similarly, HNSS was collected from a tropical island far from the Chinese mainland and with no source of fresh water. This population is likely to have been transported to the island by ships from Hainan Island, thus both the HNSS and HNSY populations belong to cluster II.
The results of STRUCTURE analysis show that these Ae. albopictus populations are divided into three clusters. Each cluster could be considered as a gene pool, the northern Chinese Ae. albopictus populations belong to gene pool I while the eastern populations belong to gene pool III. Meanwhile, the southern populations consist of three different gene pools. The genetic differences among the three gene pools are small. The FST values between cluster I and clusters II and III were 0.110 and 0.114, respectively, while that between clusters II and III was 0.027.
Ae. albopictus is also known as the Asian tiger mosquito, and it has been described as one of the 100 worst invasive species in the world. The species originated from South and East Asia, and it has spread throughout the world mostly since the second half of the twentieth century. It is now found on every continent except Antarctica [55]. Areas that have been colonized by Ae. albopictus include disparate environments such as tropical South America, Africa, and the most temperate areas of Northern America and Europe. In China, the species likely invaded the south first, colonizing the tropical and subtropical regions. From these regions, Ae. albopictus can colonize new areas and expand its population. In addition, Ae. albopictus populations from other places may have been able to invade many times; therefore, the genetic pools of the population in the southwest and south are relatively complex, as shown by STRUCTURE analysis.
Analysis of the likely migration route of Ae. albopictus in China indicates that the southern population of Ae. albopictus was introduced into the eastern and northern (the temperate regions) regions through the movement of people and goods. This describes a chaotic propagule distribution mediated by human activity, and it resulted in a relatively simple gene pool. Thus, Ae. albopictus populations in eastern and northern China should be temperate-diapausing, and there was sufficient gene flow with tropical nondiapausing populations. In particular, the photoperiodical diapause, which has a demonstrated genetic basis, seems to be an important component of climatic adaptation that favors the invasive success of Ae. albopictus. Conversely, anthropogenic activities also create new breeding and trophic niches of adaptation in close proximity to human living sites, which impacts the relationship between mosquitoes and humans.
Pearson correlation analysis found no correlation between kdr mutation and genetic structure. However, we did observe that low FST is associated with more wild-type, while high FST is associated with less wild-type. The mechanism of kdr mutation, which is closely related to insecticide resistance, is a very complex issue. This intrinsic interaction may be influenced by other important influencing factors, including climate variations, geographic distance, and the properties of insecticides used for mosquito control in different regions. Therefore, understanding the relationship between genetic structure and kdr mutations requires careful analysis, with consideration of case-specific factors. To clarify this issue, more samples need to be collected over a wider geographical area, ideally over multiple years, along with insecticide usage history. Perhaps in the future, more definite conclusions about the mechanism of kdr evolution in Ae. albopictus populations with different genetic backgrounds can be compared using a laboratory model under artificial insecticide pressure.
The main limitation of this study is the paucity of samples considering the large geographic area of China. Thus, the sampling areas should be expanded to obtain more detailed data. Therefore, we will continue to collect large numbers of samples of Ae. albopictus in the future to obtain more population data that can be used to further explore the correlation between resistance mutations and genetic background.
Conclusions
We observed a very low degree of genetic divergence among Ae. albopictus populations in China, and we found no relationship between genetic and geographical distance. These populations consist of three gene pools. The gene pools of the northern and eastern Chinese populations were relatively homogeneous, while the southern populations were heterogeneous. Greater genetic difference within a population tended to be associated with a higher mutation rate of knockdown resistance, which deserves further attention and exploration.
Availability of data and materials
Data supporting the conclusions are included within the article and additional files. The datasets used and/or analyzed during this study are available from the corresponding author upon reasonable request.
Abbreviations
- kdr :
-
Knockdown resistance
- VGSC :
-
Voltage-gated sodium channels
- F ST :
-
Fixation index
- COI:
-
Cytochrome c oxidase subunit I
References
Wu TT, Wu ZD, Li YP. Dengue fever and dengue virus in the People’s Republic of China. Rev Med Virol. 2022;32(1): e2245.
Liu QY. Dengue fever in China: new epidemical trend, challenges and strategies for prevention and control. Chin J Vector Biol Ctrl. 2020;31(1):1–6 (In Chinese).
Lu BL. Fauna Sinica, Insecta Vol. 9: Diptera, Culicidae I. Beijing: Science Press; 1997. p. 243–245 (In Chinese).
Yang S, Liu Q. Trend in global distribution and spread of Aedes albopictus. Chin J Vector Biol Ctrl. 2013;24(1):1–4 (In Chinese).
Hemingway J, Ranson H. Insecticide resistance in insect vectors of human disease. Annu Rev Entomol. 2000;45:371–91.
Chang X, Zhong D, Lo E, Fang Q, Bonizzoni M, et al. Landscape genetic structure and evolutionary genetics of insecticide resistance gene mutations in Anopheles sinensis. Parasit Vectors. 2016;9:228.
Rahman RU, Cosme LV, Costa MM, Carrara L, Lima JBP, et al. Insecticide resistance and genetic structure of Aedes aegypti populations from Rio de Janeiro State, Brazil. PLoS Negl Trop Dis. 2021;15(2): e0008492.
Chen H, Zhou Q, Dong H, Yuan H, Bai J, et al. The pattern of kdr mutations correlated with the temperature in field populations of Aedes albopictus in China. Parasit Vectors. 2021;14(1):406.
Gao J, Zhang HD, Guo XX, Xing D, Dong YD, et al. Dispersal patterns and population genetic structure of Aedes albopictus (Diptera: Culicidae) in three different climatic regions of China. Parasit Vectors. 2021;14(1):12.
Manni M, Guglielmino CR, Scolari F, Vega-Rua A, Failloux AB, et al. Genetic evidence for a worldwide chaotic dispersion pattern of the arbovirus vector, Aedes albopictus. PLoS Negl Trop Dis. 2017;11(1): e0005332.
Beebe NW, Ambrose L, Hill LA, Davis JB, Hapgood G, et al. Tracing the tiger: population genetics provides valuable insights into the Aedes (Stegomyia) albopictus invasion of the Australasian Region. PLoS Negl Trop Dis. 2013;7: e2361.
Kotsakiozi P, Richardson JB, Pichler V, Favia G, Martins AJ, et al. Population genomics of the Asian tiger mosquito, Aedes albopictus: insights into the recent worldwide invasion. Ecol Evol. 2017;7(23):10143–57.
Goubert C, Minard G, Vieira C, Boulesteix M. Population genetics of the Asian tiger mosquito Aedes albopictus, an invasive vector of human diseases. Heredity (Edinb). 2016;117(3):125–34.
Li CX, Zhu LH, Dong YD, Zhao TY, Lu BL. Genetic differentiation of Aedes albopictus strains detected ty the random amplified polymorphism DNA polymerase chain reaction (RAPD-PCR). Chin J Vector Biol Ctrl. 2003;14(4):248–50 (In Chinese).
Adilah-Amrannudin N, Hamsidi M, Ismail NA, Ismail R, Dom NC, et al. Genetic polymorphism of Aedes albopictus population inferred from ND5 gene variabilities in Subang Jaya, Malaysia. J Am Mosq Control Assoc. 2016;32(4):265–72.
Maia RT, Scarpassa VM, Maciel-Litaiff LH, Tadei WP. Reduced levels of genetic variation in Aedes albopictus (Diptera: Culicidae) from Manaus, Amazonas State, Brazil, based on analysis of the mitochondrial DNA ND5 gene. Genet Mol Res. 2009;8(3):998–1007.
Usmani-Brown S, Cohnstaedt L, Munstermann LE. Population genetics of Aedes albopictus (Diptera: Culicidae) invading populations, using mitochondrial nicotinamide adenine dinucleotide dehydrogenase subunit 5 sequences. Ann Entomol Soc Am. 2009;102(1):144–50.
Ismail NA, Adilah-Amrannudin N, Hamsidi M, Ismail R, Dom NC, et al. The genetic diversity, haplotype analysis, and phylogenetic relationship of Aedes albopictus (Diptera: Culicidae) based on the cytochrome oxidase 1 marker: a Malaysian Scenario. J Med Entomol. 2017;54(6):1573–81.
Zawani MK, Abu HA, Sazaly AB, Zary SY, Darlina MN. Population genetic structure of Aedes albopictus in Penang. Malaysia Genet Mol Res. 2014;13(4):8184–96.
Duong CV, Kang JH, Nguyen VV, Bae YJ. Genetic diversity and population structure of the Asian tiger mosquito (Aedes albopictus) in Vietnam: evidence for genetic differentiation by climate region. Genes (Basel). 2021;12(10):1579.
Motoki MT, Fonseca DM, Miot EF, Demari-Silva B, Thammavong P, et al. Population genetics of Aedes albopictus (Diptera: Culicidae) in its native range in Lao People’s Democratic Republic. Parasit Vectors. 2019;12(1):477.
Md Naim D, Kamal NZM, Mahboob S. Population structure and genetic diversity of Aedes aegypti and Aedes albopictus in Penang as revealed by mitochondrial DNA cytochrome oxidase I. Saudi J Biol Sci. 2020;27(3):953–67.
Pech-May A, Moo-Llanes DA, Puerto-Avila MB, Casas M, Danis-Lozano R, et al. Population genetics and ecological niche of invasive Aedes albopictus in Mexico. Acta Trop. 2016;157:30–41.
Lin Y, Zhang SY, Lin YY, Xue LS, Xu LS, et al. Genetic polymorphism analysis on rDNA ITS2 from different geographical strains of Aedes albopictus in Fujian province. Chin J Zoonose. 2010;26(8):735–8 (In Chinese).
Guo S, Ling F, Wang JN, Wu YY, Hou J, et al. Genetic diversity of Aedes albopictus populations in the coastal areas of southern China, using the microsatellite markers. J Chin J Epidemic. 2019;40(8):992–6 (In Chinese).
Maynard AJ, Ambrose L, Cooper RD, Chow WK, Davis JB, et al. Tiger on the prowl: invasion history and spatio-temporal genetic structure of the Asian tiger mosquito Aedes albopictus (Skuse 1894) in the Indo-Pacific. PLoS Negl Trop Dis. 2017;11(4): e0005546.
Minard G, Tran FH, Van VT, Goubert C, Bellet C, et al. French invasive Asian tiger mosquito populations harbor reduced bacterial microbiota and genetic diversity compared to Vietnamese autochthonous relatives. Front Microbiol. 2015;6:970.
Rasic G, Schama R, Powell R, Maciel-de Freitas R, Endersby-Harshman NM, et al. Contrasting genetic structure between mitochondrial and nuclear markers in the dengue fever mosquito from Rio de Janeiro: implications for vector control. Evol Appl. 2015;8(9):901–15.
Wei Y, Wang J, Song Z, He Y, Zheng Z, et al. Patterns of spatial genetic structures in Aedes albopictus (Diptera: Culicidae) populations in China. Parasit Vectors. 2019;12(1):552.
Zhong D, Lo E, Hu R, Metzger ME, Cummings R, et al. Genetic analysis of invasive Aedes albopictus populations in Los Angeles County, California and its potential public health impact. PLoS ONE. 2013;8(7): e68586.
Zheng ZH, Wu SS, Wei Y, Zhong DB, Zheng XL. Analysis of the genetic diversity of 15 Aedes albopictus populations in Guangzhou based on the mitochondrial COI gene. Chin J Zoonose. 2021;37(11):985–94 (In Chinese).
Zhang RL, Yao GQ, Pan XQ, Ma DZ, Zhao AH, et al. Genetic diversities of different geographical populations of Aedes albopictus based on mitochondrial gene COI. Chin J Zoonose. 2017;33(4):316–20 (In Chinese).
Manni M, Gomulski LM, Aketarawong N, Tait G, Scolari F, et al. Molecular markers for analyses of intraspecific genetic diversity in the Asian Tiger mosquito, Aedes albopictus. Parasit Vectors. 2015;8:188.
Schuelke M. An economic method for the fluorescent labeling of PCR fragments. Nat Biotechnol. 2000;18(2):233–4.
Peakall R, Smouse PE. GenAlEx 6.5: genetic analysis in Excel. Population genetic software for teaching and research–an update. Bioinformatics. 2012;28(19):2537–9.
J. G. FSTAT, a program to estimate and test gene diversities and fixation indices (version 2.9.3).2001. http://www2.unil.ch/popgen/softwares/fstat.html.
Weir BSCC. Estimating F-statistics for the analysis of population structure. Evolution. 1984;38(6):1358–70.
Dieringer D, Schlötterer C. MICROSATELLITE ANALYSER (MSA): a platform independent analysis tool for large microsatellite data sets. Mol Ecol Notes. 2003;3(1):167–9.
Rousset F. genepop’007: a complete re-implementation of the genepop software for Windows and Linux. Mol Ecol Resour. 2008;8(1):103–6.
Rice WR. Analyzing tables of statistical tests. Evolution. 1989;43(1):223–5.
Kalinowski ST, Taper ML, Marshall TC. Revising how the computer program CERVUS accommodates genotyping error increases success in paternity assignment. Mol Ecol. 2007;16(5):1099–106.
Mantel N. The detection of disease clustering and a generalized regression approach. Cancer Res. 1967;27(2):209–20.
Falush D, Stephens M, Pritchard JK. Inference of population structure using multilocus genotype data: linked loci and correlated allele frequencies. Genetics. 2003;164(4):1567–87.
Falush D, Stephens M, Pritchard JK. Inference of population structure using multilocus genotype data: dominant markers and null alleles. Mol Ecol Notes. 2007;7(4):574–8.
Pritchard JK, Stephens M, Donnelly P. Inference of population structure using multilocus genotype data. Genetics. 2000;155(2):945–59.
Evanno G, Regnaut S, Goudet J. Detecting the number of clusters of individuals using the software STRUCTURE: a simulation study. Mol Ecol. 2005;14(8):2611–20.
Earl DAVB. STRUCTURE HARVESTER: a website and program for visualizing STRUCTURE output and implementing the Evanno method. Conserv Genet Resour. 2011;4(2):359–61.
Jakobsson M, Rosenberg NA. CLUMPP: a cluster matching and permutation program for dealing with label switching and multimodality in analysis of population structure. Bioinformatics. 2007;23(14):1801–6.
Rosenberg NA. DISTRUCT: a program for the graphical display of population structure. Mol Ecol Notes. 2004;4(1):137–8.
Do C, Waples RS, Peel D, Macbeth GM, Tillett BJ, et al. NeEstimator v2: re-implementation of software for the estimation of contemporary effective population size (Ne) from genetic data. Mol Ecol Resour. 2014;14(1):209–14.
Gao JP, Chen HM, Shi H, Peng H, Ma YJ. Correlation between adult pyrethroid resistance and knockdown resistance (kdr) mutations in Aedes albopictus (Diptera: Culicidae) field populations in China. Infect Dis Poverty. 2018;7(1):86.
Serrote CML, Reiniger LRS, Silva KB, Rabaiolli SMDS, Stefanel CM. Determining the polymorphism information content of a molecular marker. Gene. 2020;726: 144175.
Guo Y, Song Z, Luo L, Wang Q, Zhou G, et al. Molecular evidence for new sympatric cryptic species of Aedes albopictus (Diptera: Culicidae) in China: a new threat from Aedes albopictus subgroup? Parasit Vectors. 2018;11(1):228.
Huang L, Luo X, Shao J, Yan H, Qiu Y, et al. Epidemiology and characteristics of the dengue outbreak in Guangdong, Southern China, in 2014. Eur J Clin Microbiol Infect Dis. 2016;35(2):269–77.
Kraemer MUSM, Duda KA, Mylne AQ, Shearer FM, Barker CM, et al. The global distribution of the arbovirus vectors Aedes aegypti and Ae. albopictus. Elife. 2015;4:e08347.
Acknowledgements
We are grateful to Jingpeng Gao, Pei-En Leng and Xiao-Qing Lin (SH), Li-Nong Yao (ZJHZ), Hai-Tao Huang, Jian-Bin Wu and Fang Cai (HNHK), Wuhan Liu (HNSS), Zhenzhou Yang (BJFT), Jinyong Jiang (YNJH), Li Zhang (SDJN), and Jingxia Cheng (SXYC/SXYQ), who provided field assistance in the study.
Funding
This work was supported by grants from the Natural Science Foundation of Shanghai (No. 19ZR1469600 to HP), National Natural Sciences Foundation of China (No. 31970445 to YM), National Key Research and Development Program of China (No. 2022YFC3103000 to YM) and Shanghai Industrial Collaborative Innovation Project (No. 2021-cyxt1-kj06 to HP).
Author information
Authors and Affiliations
Contributions
All authors contributed to the collection of mosquitoes. YM and HP designed the study. YM and HD identified specimens by morphological characteristics. HY, HC, HD, QZ, FT, HC, JB and FT extracted the genomic DNA and performed data analysis. WS, HY, HC, YM and HP did data analysis and wrote the manuscript. All authors have read and approved the final manuscript.
Corresponding authors
Ethics declarations
Ethics approval and consent to participate
Not applicable.
Consent for publication
Not applicable.
Competing interests
The authors declare that they have no competing interests.
Supplementary Information
Additional file 1: Figure S1.
Bayesian cluster analysis using STUCTURE. Graphical representation of the data set for the most likely K, where each color corresponds to a suggested cluster and each individual is represented by a vertical bar. The X-axis corresponds to the population codes, while the Y-axis represents the probability of assignment of an individual to each cluster. Figure S2. The collection sites for Aedes albopictus in China. The composition of the three clusters in each site is represented by a pie chart, with red, green, and blue representing clusters I, II, and III, respectively. The populations in the dotted circle belong to different clusters. Figure S3. Scatter plot of the Pearson correlation between F1534 wild-type frequency and FST value within 14 Ae. albopictus populations. Table S1. Sampling information of Aedes albopictus populations in China. Table S2. Primer sequences of 8 microsatellite loci of Aedes albopictus. Table S3. Number of alleles per population at each microsatellite locus. Table S4. Average coefficient of ancestry obtained from a STRUCTURE run with K = 3 for 443 individuals of Aedes albopictus from 16 samples collected in China.
Rights and permissions
Open Access This article is licensed under a Creative Commons Attribution 4.0 International License, which permits use, sharing, adaptation, distribution and reproduction in any medium or format, as long as you give appropriate credit to the original author(s) and the source, provide a link to the Creative Commons licence, and indicate if changes were made. The images or other third party material in this article are included in the article's Creative Commons licence, unless indicated otherwise in a credit line to the material. If material is not included in the article's Creative Commons licence and your intended use is not permitted by statutory regulation or exceeds the permitted use, you will need to obtain permission directly from the copyright holder. To view a copy of this licence, visit http://creativecommons.org/licenses/by/4.0/. The Creative Commons Public Domain Dedication waiver (http://creativecommons.org/publicdomain/zero/1.0/) applies to the data made available in this article, unless otherwise stated in a credit line to the data.
About this article
Cite this article
Shan, W., Yuan, H., Chen, H. et al. Genetic structure of Aedes albopictus (Diptera: Culicidae) populations in China and relationship with the knockdown resistance mutations. Infect Dis Poverty 12, 46 (2023). https://doi.org/10.1186/s40249-023-01096-x
Received:
Accepted:
Published:
DOI: https://doi.org/10.1186/s40249-023-01096-x