Abstract
This study investigates the robust stability problem in the presence of uncertain parameters for a class of stochastic neutral-type systems with mixed time-varying delays, where external disturbance and nonlinearity are considered together. The nonlinear function is assumed to satisfy the one-sided Lipschitz condition and the quadratic inner-boundedness condition. By constructing a modified Lyapunov–Krasovskii functional and using the free-weighting matrix technique, some new delay-dependent criteria for the stability of the problem are presented. In particular, the derivatives of the time-varying delays are no longer limited to being less than one. Finally, numerical examples are given to illustrate the effectiveness of the derived results.
Similar content being viewed by others
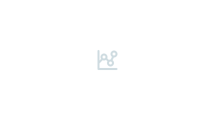
1 Introduction
The stability analysis and stabilization of time-delay systems have been tackled over because time delays occur in many practical systems, such as those in the fields of aeronautics, chemistry, and mechanics [1]. There are many valuable results regarding the stability analysis and stabilization of time delay systems [2,3,4]. Generally speaking, delay-dependent stability conditions are less conservative than delay-independent ones, especially when the time delays are relatively small. Thus, the present study is focused on delay-dependent stability [5].
Systems in many branches of science and industry are often subject to various types of noise and uncertainty [6]. Stochastic systems governed by Itô stochastic differential equations have attracted considerable attention, this being where the noise is described by Brownian motion [7, 8]. A great number of results have been successfully extended to stochastic time delay systems (e.g. [9,10,11,12]). Zhou et al. investigated the problem of stability analysis of a class of delayed genetic regulatory networks with stochastic disturbances, where the delays are assumed to be time-varying and bounded. Based on Itô’s differential formula and free-weighting matrix method [13], delay-range-dependent and rate-dependent (or independent) stability criteria are obtained [14]. By constructing a generalized free-weighting-matrix approach, Zhang et al. investigated the delay-dependent stability problem of continuous neural networks with a bounded time-varying delay. The developed approach can estimate the single integral term arising in the derivative of the Lyapunov–Krasovskii functional more accurately [15].
Neutral-type stochastic differential equations, depend on the delays of state and state derivative simultaneously, are often encountered in various fields, such as automatic control, aircraft stabilization, lossless transmission lines, and turbojet engines [16,17,18,19,20,21,22,23]. Cheng et al. investigated the problem of robust stability criteria delay-dependent for neutral systems with interval time-varying delays and nonlinear perturbation [24]. Basic on a piecewise delay method, the authors obtained some new sufficient conditions to guarantee the asymptotic stability for neutral time-delay systems. Kao et al. have probed the problem of \(H_{\infty }\) sliding mode control for nonlinear uncertain neutral stochastic systems with Markovian switching parameters. By utilizing a sliding mode control strategy, they got some criteria on asymptotic stability of the error system and sliding mode dynamics [23]. In [25], Yao et al. investigated the problem of robust adaptive sliding mode control for uncertain neutral Markovian jump systems with unknown nonlinearity and unmeasured states. To the authors’ best knowledge, the problem of delay-dependent stability criteria for uncertain neutral-type with mixed time-varying delays has not been well probed yet, which still is an open problem. This being because the neutral item and the nonlinearity complicate the problem.
Motivated by the aforementioned discussion, the present paper investigates the problem of robust stability for a class of stochastic nonlinear neutral-type systems with mixed time-varying delays. The main contributions of the present work are as follows.
-
i.
Some delay-dependent sufficient conditions are proposed by constructing an appropriate Lyapunov–Krasovskii functional and using the free-weighting matrix method.
-
ii.
The derivatives of the time-varying delays no longer must be less than one, thereby generalizing the existing results.
-
iii.
Some free-weighting matrices are introduced to avoid using any inequality to deal with the cross terms. Therefore, our results are less conservative.
The remainder of the paper is organized as follows. Section 2 outlines the required mathematical preliminaries. The main results are presented in Sect. 3. In Sect. 4, two practical examples are provided to demonstrate the effectiveness of the proposed methods. Finally, Sect. 5 concludes the paper.
Notations
Throughout this paper, let \((\varOmega ,\mathcal{F}, \mathcal{P})\) be a complete probability space with a filtration \(\{\mathcal{F}_{t}\}_{t\geq 0}\) satisfying the usual conditions. \(B(t)\) is a one-dimensional Brownian motion defined on the probability space adapted to the filtration. \(\mathbb{R}^{n}\) and \(\mathbb{R}^{m \times n}\) denote the n-dimensional Euclidean space and the set of all \(m\times n\) real matrix, respectively. \(\|\cdot \|\) is the usual Euclidean norm in \(\mathbb{R}^{n}\). The inner product of vectors x and y in \(\mathbb{R}^{n}\) is denoted by \(\langle x,y\rangle \) or \(x^{T}y\). Let \(C^{2,1}(\mathbb{R}^{n}\times \mathbb{R}_{+}; \mathbb{R}_{+})\) denote the family of all real-valued functions \(V(x(t),t)\) defined on \(\mathbb{R}^{n}\times \mathbb{R}_{+}\) such that they are continuously twice differentiable in x and once in t. \(C([- {r},0]; \mathbb{R}^{n})\) denotes the space of all continuous \(\mathbb{R}^{n}\)-valued functions φ defined on \([- {r}, 0]\) with a norm \(\|\varphi \|=\) sup\(_{- {r} \leq \theta \leq 0}|\varphi (\theta )|\). For a real symmetric matrix X, \(X>0\) (\(X \geq 0\)) means that X is positive definite (positive semi-definite). The asterisk ∗ denotes a matrix that can be inferred by symmetry and the superscript T represents the transpose of a matrix or a vector. In a matrix, \((i,j)\) denotes an \((i,j)\)-block element of the matrix. The notation \(\mathbf{E}\{\cdot \}\) represents the mathematical expectation operator. I denotes the identity matrix of compatible dimension. \(e_{i}\) denotes index matrices that consist of the unit matrix on the ith position and zero blocks on other positions.
2 Preliminaries
In this section, several basic assumptions and conclusions are offered that are of use regarding the main results. These basic facts can be found in any introductory book on stochastic differential equations (e.g. [5, 6, 26,27,28,29]).
Assumption 2.1
(One-sided Lipschitz condition [5])
The nonlinear function \(f(x,y)\) is said to be one-sided Lipschitz if there exist \(\alpha _{1}, \alpha _{2}\in \mathbb{R}\) satisfying
for \(\forall x,y \in \mathbb{R}^{n}\), where constant \(\alpha _{1}\) and \(\alpha _{2}\) are positive, zero, or even negative, and they are called one-sided Lipschitz constants for \(f(x,y)\) with respect to x and y.
Assumption 2.2
(Quadratic inner-boundedness condition [5])
The nonlinear function \(f(x,y)\) is called quadratic inner-boundedness in the region \(\mathcal{C}\), if there exist constants \(\beta _{1}\), \(\beta _{2}\), and κ such that
For stochastic systems, Itô’s formula plays an important role in the stability analysis. We cite the following result here.
Lemma 2.1
(Itô’s formula [6])
Let \(x(t)\) be an n-dimensional Itô process on \(t\geq 0\) with the stochastic differential
where \(f(t)\in \mathbb{R}^{n}\) and \(g(t)\in \mathbb{R}^{n\times m}\). Let \(V(x(t),t)\in \mathcal{C}^{2,1}(\mathbb{R}^{n}\times \mathbb{R}^{+}; \mathbb{R}^{+})\). Then \(V(x(t),t)\) is a real-valued Itô process with its stochastic differential given by
where \(\mathcal{C}^{2,1}(\mathbb{R}^{n}\times \mathbb{R}^{+}; \mathbb{R}^{+})\) denotes the family of all real-valued functions V(x(t),t) such that they are continuously twice differentiable in x and t. If \(V(x(t),t)\in \mathcal{C}^{2,1}(\mathbb{R}^{n}\times \mathbb{R} ^{+}; \mathbb{R}^{+})\), we set
Lemma 2.2
(S-procedure [29])
Let \(\mathcal{Z}\) be a linear vector space, and \(\mathcal{F}(z)\), \(y_{1}(z)\), \(y_{1}(z)\), …, \(y_{k}(z)\) be some real-valued functionals over \(\mathcal{Z}\). Furthermore, define the domain \(\mathcal{D}\) as follows:
and the two following conditions:
-
(1)
\(\mathcal{F}(z)>0\), \(\forall z\in \mathcal{D}\),
-
(2)
∃ scalars \(\varepsilon _{1}\geq 0,\varepsilon _{2}\geq 0,\ldots , \varepsilon _{k}\geq 0\) such that
$$ \mathcal{S}(\varepsilon ,z)=\mathcal{F}(z)-\sum_{i=1}^{k} \varepsilon _{i} y_{i}(z)>0, \quad \forall z\in \mathcal{Z}. $$
Then (2) implies (1). The procedure of replacing (1) by (2) is called the S-procedure.
Lemma 2.3
(Schur complement [28])
For a given symmetric matrix , the following conditions are equivalent:
-
(1)
\(S<0\);
-
(2)
\(S_{11}<0\), \(S_{22}-S_{12}^{T}S_{11}^{-1}S_{12}<0\);
-
(3)
\(S_{22}<0\), \(S_{11}-S_{12}S_{22}^{-1}S_{12}^{T}<0\).
Lemma 2.4
(Matrix inequality [30])
Let E, G and F be real matrices of appropriate dimensions with \(F^{T}F\leq I\), then we have, for any scalar \(\varepsilon >0\),
3 Robust stability analysis
Consider the following uncertain neutral stochastic mixed time-varying delays neutral-type system described in Itô’s form:
where \(x(t)\in \mathbb{R}^{n}\) is the state vector, \(\tau (t)\) is the unknown time-varying delay satisfying \(0\leq \tau (t)< {\tau _{1}}\), \(0\leq \lambda (t)< {\lambda _{1}}\), \(\dot{\tau } (t) \leq d\) and \(\dot{\lambda } (t)\leq \mu \) with real constants \({\tau _{1}, \lambda _{1}}\), d and μ. \(f(x(t),x(t-\tau (t)))\in \mathbb{R}^{n}\) is a nonlinear function with respect to the state \(x(t)\) and the delayed state \(x(t-\tau (t))\), \(f(0,0)=0\). \({\varphi }(t)\in C([ {-r} ,0];\mathbb{R}^{n})\) is a vector valued continuous function, and \(w(t)\) is the standard one-dimensional Brownian motion satisfying
Here \(A\in \mathbb{R}^{n\times n}\), \(A_{\tau }\in \mathbb{R}^{n\times n}\), \(D\in \mathbb{R}^{n\times n}\), \(H\in \mathbb{R}^{n\times n}\) and \(H_{\tau }\in \mathbb{R}^{n\times n}\). Moreover, \(\Delta A(t)\), \(\Delta A_{\tau }(t)\), \(\Delta H(t)\) and \(\Delta H_{\tau }(t)\) are unknown matrices representing time-varying parameter uncertainties and are assumed to be of the form
where \(E_{1}\), \(E_{2}\), \(G_{1}\) and \(G_{2}\) are known real constant matrices of appropriate dimensions, and \(F(t)\) is an unknown time-varying matrix function satisfying
The parameter uncertainties \(\Delta A(t)\), \(\Delta A_{\tau }(t)\), \(\Delta H(t)\) and \(\Delta H_{\tau }(t)\) are said to be admissible if both (4) and (5) hold.
Let
System (3) can be rewritten as follows:
In this section, we will solve the problem of robust stability for uncertain stochastic time-delay system (6) by constructing the appropriate Lyapunov–Krasovskii functional and introducing free-weighting matrix. A new delay-dependent stability criteria is derived.
Theorem 3.1
Consider the neutral stochastic time-delay system (6). The nonlinear function \(f(x(t),x(t-\tau (t)))\) satisfies Assumptions 2.1 and 2.2. For given scalars \(\tau _{1}\), \(\lambda _{1}\), μ and d, if there exist symmetric positive definite matrices \(P\in \mathbb{R}^{n\times n}\), \(Q_{1}\in \mathbb{R}^{n\times n}\), \(Q_{2}\in \mathbb{R}^{n\times n}\), \(S_{1}\in \mathbb{R}^{n\times n}\), \(S_{2}\in \mathbb{R}^{n\times n}\), \(R_{1}\in \mathbb{R}^{n\times n}\), \(R_{2}\in \mathbb{R}^{n\times n}\), \(M _{i}>0\) (\(i=1,\ldots, 10\)) and \(N_{j}\) (\(j=1,\ldots, 8\)) of appropriate dimensions and scalars \(\varepsilon _{1}>0\), \(\varepsilon _{2}>0\) and \(\varepsilon _{3}>0\) satisfying the following LMIs:
with
then the null solution of the stochastic time-delay system (6) is asymptotically stable in the mean square.
Proof
Choose the following Lyapunov–Krasovskii functional:
with
Using Itô’s formula, Lemma 2.1, we obtain the stochastic differential as follows:
with
Substituting (16)–(21) into (15), one can obtain
Taking the expectation of both sides of (22), we have
Set
We can derive the following equations by using the Newton–Leibniz formula:
where \(N_{j}\) (\(j=1,\ldots ,4\)) are arbitrary matrices with appropriate dimensions. Adding the left-hand sides of (24), (25), (26) and (27) on to \(\mathcal{L}V (x(t), t)\), and noticing the properties of the stochastic integral, we have
Thus (23) is transformed to
where
Recalling that \(\dot{\tau } (t)\leq d\) and \(\dot{\lambda } (t) \leq \mu \), and subdividing the integration interval, we have
On the other hand, by using the one-sided Lipschitz (1) and the quadratically inner-bounded conditions (2), we obtain the following inequality:
Using the S-procedure Lemma 2.2 in (31), we can see that \(\mathcal{L}\tilde{V}(x(t),t)<0\) is implied if there exist positive scalars \(\varepsilon _{1}\), \(\varepsilon _{2}\) and \(\varepsilon _{3}\) satisfying
Moreover, the following formula holds for any positive definite matrices \(M _{i}\) (\(i=1,\ldots ,10\)) of appropriate dimensions:
Let
Combining the above formulas (35)–(44) and rearranging (34), if \(\varPsi _{11}<0\), we have the following inequality:
with \(\varPsi _{11}\), \(\varSigma _{1}\), \(\varSigma _{2}\), \(\varPi _{1}\) and \(\varPi _{2}\) being defined as in (8), (9), (10), (11) and (12). Utilizing the Schur complement Lemma 2.3, (45) is equivalent to the following LMI:
with \(\varPsi _{11}\), \(\varPsi _{12}\), \(\varPsi _{13}\), \(\varPsi _{14}\), \(\varPsi _{15}\), M̄ and \(\bar{\bar{M}}\) being defined as in (12) and (13).
Pre-and post-multiplying (46) by \(\operatorname{diag}\{\overbrace{ I,\ldots ,I,}^{11} R_{1},R_{2}, \overbrace{ I,\ldots ,I}^{10}\}\), we obtain LMI (7). Combining with LMIs (8), (9), (10) and (11), we find that \(\mathbf{E}\mathcal{L}\tilde{V }(\xi (t), t)<0\), i.e., it guarantees the asymptotic stability of system (6) in the mean square. □
Remark 3.1
In Theorem 3.1, we do not use any inequality techniques for the cross terms. Therefore, our result is less conservative.
Remark 3.2
In the proof of Theorem 3.1, the purpose of introducing \(M_{1},\ldots ,M_{10}\) is to expand the dimension of the matrices \(\varSigma _{1}\), \(\varSigma _{2}\), \(\varPi _{1}\) and \(\varPi _{2}\) so that we can solve them by LMIs technology.
If uncertain parameters \(\Delta A(t)\), \(\Delta A_{\tau }(t)\), \(\Delta H(t)\) and \(\Delta H_{\tau }(t)\) in system (6) are equal to zero, the system is simplified to the following deterministic stochastic system:
The following conclusion of the robust asymptotic stability is obtained by Theorem 3.1 for the deterministic stochastic system (47).
Corollary 3.1
Consider the neutral stochastic time-delay system (47). The nonlinear function function \(f(x(t),x(t-\tau (t)))\) satisfies Assumptions 2.1 and 2.2. For given scalars \({\tau _{1}}\), \({\lambda _{1}}\), μ and d, if there exist symmetric positive definite matrices \(P\in \mathbb{R}^{n\times n}\), \(Q_{1}\in \mathbb{R}^{n\times n}\), \(Q_{2}\in \mathbb{R}^{n\times n}\), \(S_{1}\in \mathbb{R}^{n\times n}\), \(S_{2}\in \mathbb{R}^{n\times n}\), \(R_{1}\in \mathbb{R}^{n\times n}\), \(R_{2}\in \mathbb{R}^{n\times n}\), \(M _{i}>0\) (\(i=1,\ldots ,10\)) and \(N_{j}\) (\(j=1,\ldots ,8\)) of appropriate dimensions and scalars \(\varepsilon _{2}>0\) and \(\varepsilon _{3}>0\) satisfying the following LMI:
with
Combining with LMIs (8), (9), (10) and (11), then the null solution of the stochastic time-delay system (47) is asymptotically stable in the mean square.
4 Two illustrative numerical examples
In this section, we give some examples to demonstrate the effectiveness of the method proposed herein.
Example 4.1
Consider the neutral-type nonlinear mixed time-varying delays system (47). We choose the same system matrix as those in Refs. [31, 32] and nonlinear function as this in Ref. [9], i.e. \(\alpha _{1}=0.5\), \(\alpha _{2}=0.005\), \(\beta _{1}=-2.5\), \(\beta _{2}=-0.015\) and \(\kappa =5\). Let \(\lambda (t)=\tau (t)\) and give parameter matrix D. Table 1 lists the maximal allowable upper bound of the time delay for different parameter matrix D. From Table 1, it is easily seen that, when \(d>1\), our result still holds, that our proposed method is not constrained by the condition of \(0<\dot{\tau }(t)<1\). Therefore, we can conclude that our proposed method in this paper is a generalization of the existing results of neutral-type stochastic nonlinear time-varying delays systems.
Example 4.2
Consider the neutral-type stochastic mixed time-varying delays nonlinear system (6) with the same system matrix parameters as those in Refs. [33,34,35]:
Choosing the same nonlinear function parameter constants as those in Example 4.1. By using Theorem 3.1 with \(H=H_{\tau }=0\), we can obtain the maximal allowable upper bounds of the time delays in Tables 2 and 3. Tables 2 and 3 give the allowable upper bounds for \(\lambda _{1}\) and \({\tau _{1}}\) when \(d>1\) or \(\mu >1\), respectively. It can be seen that when d increases, the maximum allowable upper bounds \({\tau _{1}}\) descend with \(\mu =1.2\). By contrast, when \({\tau _{1}}\) descends, the maximum allowable upper bounds \(\lambda _{1}\) increase with \(d=0.5\).
Let
If we choose the time-varying delays as \({\tau _{1}} = 1.5\), \(d=0.5\), \(\mu =1.2\) and \({\lambda _{1}}=1.6596\) then according to Theorem 3.1 we can obtain a set of solutions as follows:
5 Conclusions
This paper provides sufficient findings for the stability criteria of uncertain neutral-type stochastic nonlinear systems with mixed time-varying delays. Some delay-dependent stability criteria are obtained by using a suitable Lyapunov–Krasovskii functional and linear matrix inequality (LMI) techniques. The derived outcome is expressed via LMIs that can be calculated through the MATLAB LMI Control Toolbox. Compared with some previous studies, the present study has two novel aspects. First, because no inequality technology is used to deal with the cross terms, our results are less conservative. Second, the derivatives of the time-varying delays are no longer limited to being less than one, thereby broadening the study scope. Numerical examples have been provided to illustrate the effectiveness of our main results.
References
Zheng, G., Polyakov, A., Levant, A.: Delay estimation via sliding mode for nonlinear time-delay systems. Automatica 89, 266–273 (2018)
Zhou, B., Luo, W.: Improved Razumikhin and Krasovskii stability criteria for time-varying stochastic time-delay systems. Automatica 89, 382–391 (2018)
Lee, T.H., Ju, H.P., Park, M.J., Kwon, O.M., Jung, H.Y.: On stability criteria for neural networks with time-varying delay using Wirtinger-based multiple integral inequality. J. Franklin Inst. 352(12), 5627–5645 (2015)
Gyurkovics, E., Takacs, T.: Multiple integral inequalities and stability analysis of time delay systems. Syst. Control Lett. 96, 72–80 (2016)
Ma, T., Li, L.: Stability criteria on delay-dependent robust stability for uncertain neutral stochastic nonlinear systems with time-delay. J. Inequal. Appl. 2018(1), 293 (2018). https://doi.org/10.1186/s13660-018-1886-5
Mao, X., Yuan, C.: Stochastic Differential Equations with Markovian Switching. Imperial College Press, London (2006)
Ito, K.: On stochastic differential equations. Mem. Am. Math. Soc. 4(4), 289–302 (1951)
Mao, X., Marion, G., Renshaw, E.: Environmental noise suppresses explosion in population dynamics. Stoch. Process. Appl. 97, 96–110 (2002)
Miao, X., Li, L.: New stability criteria for uncertain nonlinear stochastic time-delay systems. Circuits Syst. Signal Process. 34(8), 2441–2456 (2015)
Saravanakumar, R., Ali, M.S., Karimi, H.R.: Robust \(h_{\infty }\) control of uncertain stochastic Markovian jump systems with mixed time-varying delays. Int. J. Syst. Sci. 48(4), 862–872 (2017)
Wang, C., Shen, Y.: Delay-dependent non-fragile robust stabilization and \(h_{\infty }\) control of uncertain stochastic systems with time-varying delay and nonlinearity. J. Franklin Inst. 348(8), 2174–2190 (2011)
Li, H., Chen, B., Zhou, Q., Qian, W.: Robust stability for uncertain delayed fuzzy Hopfield neural networks with Markovian jumping parameters. IEEE Trans. Syst. Man Cybern., Part B, Cybern. 39(1), 94–102 (2009)
He, Y., Wang, Q.G., Xie, L., Lin, C.: Further improvement of free-weighting matrices technique for systems with time-varying delay. IEEE Trans. Autom. Control 52(2), 293–299 (2007)
Zhou, Q., Xu, S., Chen, B., Li, H., Chu, Y.: Stability analysis of delayed genetic regulatory networks with stochastic disturbances. Phys. Lett. A 373(41), 3715–3723 (2009)
Zhang, C., He, Y., Jiang, L., Lin, W., Wu, M.: Delay-dependent stability analysis of neural networks with time-varying delay: a generalized free-weighting-matrix approach. Appl. Math. Comput. 294, 102–120 (2017)
Samli, R., Arik, S.: New results for global stability of a class of neutral-type neural systems with time delays. Appl. Math. Comput. 210(2), 564–570 (2009)
Xia, Y., Boukas, E.K., Shi, P., Zhang, J.: Stability and stabilization of continuous-time singular hybrid systems. Automatica 45(6), 1504–1509 (2009)
Yue, D., Han, Q.L.: Robust \(h_{\infty }\) filter design of uncertain descriptor systems with discrete and distributed delays. IEEE Trans. Signal Process. 52(11), 3200–3212 (2004)
Gao, H., Lam, J., Wang, C.: Robust energy-to-peak filter design for stochastic time-delay systems. Syst. Control Lett. 55(2), 101–111 (2006)
Kolmanovskii, V., Myshkis, A.: Applied Theory of Functional Differential Equations. Kluwer Academic, Boston (1992)
Kao, Y., Wang, C.: Global stability analysis for stochastic coupled reaction-diffusion systems on networks. Nonlinear Anal., Real World Appl. 14(3), 1457–1465 (2013)
Niu, Y., Lam, J., Wang, X., Ho, D.W.C.: Neural adaptive sliding mode control for a class of nonlinear neutral delay systems. J. Dyn. Syst. Meas. Control 130(6), 758–767 (2008)
Kao, Y., Xie, J., Wang, C., Karimi, H.R.: A sliding mode approach to \(h_{\infty }\) non-fragile observer-based control design for uncertain Markovian neutral-type stochastic systems. Automatica 52, 218–226 (2015)
Cheng, J., Zhu, H., Zhong, S., Li, G.: Novel delay-dependent robust stability criteria for neutral systems with mixed time-varying delays and nonlinear perturbations. Math. Pract. Theory 219(14), 7741–7753 (2013)
Yao, D., Liu, M., Li, H., Ma, H.: Robust adaptive sliding mode control for nonlinear uncertain neutral Markovian jump systems. Circuits Syst. Signal Process. 35(8), 1–21 (2016)
Mao, X.R.: Stochastic Differential Equations and Applications. Ellis Horwood, Chichester (2004)
Yue, D., Tian, E., Zhang, Y., Peng, C.: Delay-distribution-dependent robust stability of uncertain systems with time-varying delay. Int. J. Robust Nonlinear Control 19(4), 377–393 (2009)
Chen, H., Hu, P.: Further results on delay-dependent exponential stability for uncertain stochastic neural networks with mixed delays and Markovian jump parameters. Neural Comput. Appl. 22(1), 221–233 (2013)
Yakubovic, V.A.: S-procedure in Nonlinear Control Theory. Ser. Matematika, Vestnik Leningradskogo Universiteta (1997)
Niu, Y., Lam, J., Wang, X., Ho, D.W.C.: Observer-based sliding mode control for nonlinear state-delayed systems. Int. J. Syst. Sci. 35(2), 139–150 (2004)
Cao, Y., Lam, J.: Computation of robust stability bounds for time-delay systems with nonlinear time-varying perturbations. Int. J. Syst. Sci. 31(3), 359–365 (2000)
Zuo, Z., Wang, Y.: New stability criterion for a class of linear systems with time-varying delay and nonlinear perturbations. IEE Proc., Control Theory Appl. 153(5), 623–626 (2006)
Chen, H.: Brief paper: new results on stability analysis for neutral stochastic linear system. IET Control Theory Appl. 7(13), 1753–1764 (2013)
Yu, K.W., Lien, C.H.: Stability criteria for uncertain neutral systems with interval time-varying delays. Chaos Solitons Fractals 38(3), 650–657 (2008)
Zhang, Z., Guang, H.: Delay-dependent stability for systems with fast-varying neutral-type delays via a ptvd compensation. Acta Autom. Sin. 36(1), 147–152 (2010)
Acknowledgements
The authors would like to express their deep gratitude to the referee for his/her carefully reading and invaluable comments.
Availability of data and materials
Not applicable.
Funding
This work was supported by the National Natural Science Foundation of China (No. 61873071), the Fundamental Research Funds in Heilongjiang Provincial Universities (Nos. 135209246, 135209250, and 135209259), and the Science and Technology Planning Project Fund of Qiqihar (No. GYGG-201718).
Author information
Authors and Affiliations
Contributions
TM carried out the robust stability analysis. LL participated in the design and coordination and helped to analyze the manuscript. All authors read and approved the final manuscript.
Corresponding author
Ethics declarations
Competing interests
The authors declare that they have no competing interests.
Additional information
Publisher’s Note
Springer Nature remains neutral with regard to jurisdictional claims in published maps and institutional affiliations.
Rights and permissions
Open Access This article is distributed under the terms of the Creative Commons Attribution 4.0 International License (http://creativecommons.org/licenses/by/4.0/), which permits unrestricted use, distribution, and reproduction in any medium, provided you give appropriate credit to the original author(s) and the source, provide a link to the Creative Commons license, and indicate if changes were made.
About this article
Cite this article
Ma, T., Li, L. Stability analysis for uncertain neutral-type stochastic nonlinear systems with mixed time-varying delays. J Inequal Appl 2019, 153 (2019). https://doi.org/10.1186/s13660-019-2105-8
Received:
Accepted:
Published:
DOI: https://doi.org/10.1186/s13660-019-2105-8