Abstract
Background
TSPAN7 is an important factor in tumor progression. However, the precise function of TSPAN7 and its role in pan-cancer are not clear.
Methods
Based on Xinhua cohort incorporating 370 patients with kidney neoplasm, we conducted differential expression analysis by immunohistochemistry between tumor and normal tissues, and explored correlations of TSPAN7 with patients’ survival. Subsequently, we conducted a pan-cancer study, and successively employed differential expression analysis, competing endogenous RNA (ceRNA) analysis, protein-protein interaction (PPI) analysis, correlation analysis of TSPAN7 with clinical characteristics, tumor purity, tumor genomics, tumor immunity, and drug sensitivity. Last but not least, gene set enrichment analysis was applied to identify enriched pathways of TSPAN7.
Results
In Xinhua cohort, TSPAN7 expression was significantly up-regulated (P-value = 0.0019) in tumor tissues of kidney neoplasm patients. High TSPAN7 expression was associated with decreases in overall survival (OS) (P-value = 0.009) and progression-free survival (P-value = 0.009), and it was further revealed as an independent risk factor for OS (P-value = 0.0326, HR = 5.66, 95%CI = 1.155–27.8). In pan-cancer analysis, TSPAN7 expression was down-regulated in most tumors, and it was associated with patients’ survival, tumor purity, tumor genomics, tumor immunity, and drug sensitivity. The ceRNA network and PPI network of TSPAN7 were also constructed. Last but not least, the top five enriched pathways of TSPAN7 in various tumors were identified.
Conclusion
TSPAN7 served as a promising biomarker of various tumors, especially kidney neoplasms, and it was closely associated with tumor purity, tumor genomics, tumor immunology, and drug sensitivity in pan-cancer level.
Similar content being viewed by others
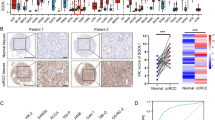
Introduction
Tetraspanins (TSPANs) are a protein family with 33 mammalian members that consists of four transmembrane segments, a large extracellular loop, a small extracellular loop, and a small intracellular loop [1,2,3]. Large amounts of evidence have demonstrated TSPANs as a facilitator of tumor development [4]. Runzhi Huang et al. discovered the important role of TSPANs in pan-cancer [5]. Notably, it has been found that TSPAN8 could increase tumor cell stemness through the activation of sonic Hedgehog pathway in breast cancer [6]. Apart from this, high expression of TSPAN13 could induce the CXCR4/CXCL12 signaling transduction, and increase the homing or migration of leukemia stem cells towards bone marrow niche [7]. In addition, TSPAN1 was related to the chemotherapy resistance of tumor cells [8]. Above all, TSPANs function in multiple steps of tumor development and tumor therapy.
TSPAN7 is a member of TSPANs located in chromosome Xp11.4 in humans, and exhibits a significant expression in the cerebral cortex and hippocampal region [9, 10]. Although other proteins of TSPAN family have been widely reported to be related to tumor development, there are relatively limited studies about TSPAN7’s roles in tumors. Marco Gerlinger et al. identified TSPAN7 as a predictive factor of poor cancer-specific survival in clear cell renal cell carcinoma [11]. Besides, in osteosarcoma, it has been discovered that TSPAN7 could stimulate the invasion of malignant cells by triggering FAK-Src-Ras-ERK1/2 signaling pathway and generating epithelial-mesenchymal transition (EMT) [12]. In glioma, the expressions of PD-1, PD-L1, and CTLA-4 were negatively correlated with the expression level of TSPAN7, suggesting it as a potential target of immunotherapy [13]. Although these studies preliminarily highlighted the pivotal role of TSPAN7 in a single kind of tumor, few studies have focused on the general function of TSPAN7 across various tumors.
Some previous studies have already identified certain genes as biomarkers in cancer progression at a pan-cancer level [14, 15]. Thus, we intended to carry out a pan-cancer study to comprehensively illustrate the complicated functions of TSPAN7 in tumors. First of all, based on a large retrospective cohort of kidney neoplasm, we discovered the differential expression level of TSPAN7 between tumor and normal tissues, and identified TSPAN7 as a promising prognostic biomarker for kidney neoplasm patients. Subsequently, we questioned if TSPAN7 also played a vital role in other tumors, and conducted a pan-cancer study. Based on The Cancer Genome Atlas (TCGA), we revealed the differential expression of TSPAN7 at a transcription level across 33 cancer types, and further constructed the competing endogenouse RNA (ceRNA) network and protein-protein interaction (PPI) network of TSPAN7. Then, we studied the clinical correlations of TSPAN7, as well as its relationships with tumor purity, tumor genomics, tumor immunity, and drug sensitivity. Last but not least, we conducted functional enrichment analysis of TSPAN7, to preliminarily reveal the molecular mechanism of TSPAN7’s role in tumor development.
Materials and methods
Data source
Our study was approved by the Ethics Committee of Xinhua Hospital Affiliated to Shanghai Jiao Tong University School of Medicine. 370 patients with kidney neoplasm, who received surgery from 2016 to 2018 were selected to constitute a large retrospective cohort. We conducted follow-up on March, 2021. Tumor and normal tissues of these patients were extracted for immunohistochemical (IHC) staining and scoring to reveal the expression of TSPAN7 in kidney neoplasm at a proteomic level. The TSPAN7 expression data, the patients’ clinical data, the single nucleotide variation (SNV) data, the copy number variation (CNV) data, and the methylation data across 33 cancer types were obtained from TCGA database (https://tcga-data.nci.nih.gov/tcga/). The data of microRNAs (miRNAs) derived from DIANA-microT (https://dianalab.e-ce.uth.gr/microt_webserver/#/) [16], miRWalk (http://mirwalk.umm.uni-heidelberg.de/) [17], miRDB (https://mirdb.org/) [18], miRcode (http://www.mircode.org/) [19], and Encyclopedia of RNA Interactomes (ENCORI) (https://rnasysu.com/encori/) databases [20]. The data of long noncoding RNAs (lncRNAs) was from ENCORI database as well. The protein-protein interaction data came from STRING database (https://cn.string-db.org/) [21]. The microsatellite instability (MSI) scores derived from Sameek Roychowdhury et al.’s study [22]. The genetic alteration profiles of various pan-cancer studies were obtained from cBioPortal (https://www.cbioportal.org/) [23]. Besides, we also retrieved the immune cell infiltration data from Tumor Immune Estimation Resource 2.0 (TIMER2.0) (http://timer.cistrome.org/) [24], and searched for the data concerned with cytotoxic T-lymphocyte (CTL) infiltration and function from the Tumor Immune Dysfunction and Exclusion (TIDE) database (http://tide.dfci.harvard.edu/) [25]. The data concerned drug sensitivity was obtained from Genomics of Drug Sensitivity in Cancer (GDSC) (https://www.cancerrxgene.org/) [26], The Cancer Therapeutics Response Portal (CTRP) (https://pubmed.ncbi.nlm.nih.gov/23993102/) [27,28,29], and CellMiner database (https://discover.nci.nih.gov/cellminer/home.do) [30, 31]. Last but not least, the data concerning signaling pathways came from the Kyoto Encyclopedia of Genes and Genomes (KEGG) (https://www.genome.jp/kegg/) [32].
Immunohistochemical staining and scoring
Tumor and normal tissues of 370 patients with kidney neoplasm were preliminarily fixed, embedded in paraffin and then sliced into tissue sections. Then, the sections were dewaxed and rehydrated, and Ethylene Diamine Tetraacetic Acid (EDTA) (pH = 9.0) buffer was employed for the antigen-retrieval process. After that, the samples were treated with 3% hydrogen peroxide solution to block endogenous hydrogen peroxide. 5% goat serum was then added to prevent the non-specific antibody binding. Afterwards, we successively added primary antibodies (TSPAN7, proteintech, 18695-1-AP, 1: 50) and secondary antibodies marked with enzyme Horseradish Peroxidase (HRP). Then, Diaminobenzidine (DAB), the specific substrate for HRP, was then added for color development. Besides, haematoxylin was utilized to enhance nuclear contrast. Ultimately, a series of alcohol solution with graded concentrations were used to dehydrate the samples, and all tissue sections were observed under a light microscope. Two pathologists were invited to independently evaluate the slides. The conflicted results were carefully checked by a third pathologist. As TSPAN7 is mainly expressed in cytoplasm and membranes [4], the staining of these locations under the light microscope was regarded as positive staining. The staining intensity score was defined as: 0 for negative, 1 for weak, 2 for moderate, and 3 for intense. The score for the percentage of positive cells was defined as: 0 for 0%, 1 for 1–10%, 2 for 11–40%, 3 for 41–70%, and 4 for 71–100%. The IHC score was the product of the staining intensity score and score for the percentage of positive cells, ranging from 0 to 12.
Clinical correlation analysis of TSPAN7 in kidney neoplasm
We collected the clinical data of 370 patients with kidney neoplasm in the retrospective cohort, and data concerning age, gender, histopathological classification, Fuhrman nuclear stage, TNM stage, clinical stage, progression after treatment, progression free survival (PFS), and overall survival (OS) were put into further analysis. The differential IHC scores of TSPAN7 in tumor and normal tissues were visualized in violin plot based on “ggviolin” function from “ggpubr” package (version 0.6.0, https://rpkgs.datanovia.com/ggpubr/). The “surv_cutpoint” function from “survminer” R package (version 0.4.9, https://rpkgs.datanovia.com/survminer/index.html) was applied to identify the cut-off value of the IHC score of TSPAN7, and divided the patients into two subgroups. Kaplan-Meier (K-M) survival analysis was then applied to explore the associations of TSPAN7 with the OS and PFS of patients with kidney neoplasm. Besides, we also used the method of Chi-square test to explore the relationships between TSPAN7 and other clinicopathological variables based on “chisq.test” function from “rstatix” package (version 0.7.2, https://rpkgs.datanovia.com/rstatix/) as well as “ggstatsplot” package (version 0.12.3, https://indrajeetpatil.github.io/ggstatsplot/, https://github.com/IndrajeetPatil/ggstatsplot). Subsequently, we incorporated demographic variables including “age”, “gender”, some important clinicopathological variables in the clinical practice of kidney neoplasms including “KIRC (yes or no)”, “Fuhrman nuclear stage”, “T stage”, “progression after treatment”, as well as “IHC score of TSPAN7 in tumor tissues” to conduct multivariable Cox regression analysis based on “coxph” function from “survival” package (version 3.7-0, https://github.com/therneau/survival). 76 patients lacking any of the above data were excluded, and 294 patients were ultimately included in the analysis. The forest plots and nomograms concerning the OS of patients with kidney neoplasm were then constructed, and the risk scores were calculated as follows:
Risk Score = β1 × Variable1 + β2 × Variable2 + …… + βn × Variablen.
β referred to the regression coefficient determined by the multivariable Cox regression model. n referred to the number of variables.
Based on the median value of risk scores, we divided the patients into the high- and low-risk subgroup, and visualized the OS of patients within the two subgroups, respectively, in the form of scatter plots and K-M survival curves. Finally, we employed residual analysis, calibration curve analysis, and Receiver Operating Characteristic (ROC) analysis to diagnose the multivariable Cox regression model (“survminer” package, “survival” package, and “timeROC” package (version 0.4, https://CRAN.R-project.org/package=timeROC)).
Differential expression analysis of TSPAN7 in pan-cancer
“ggpubr” R package was applied to reveal the differential expression of TSPAN7 between tumor and normal tissues across 33 cancer types in TCGA (Wilcox test). The expression of TSPAN7 was illustrated in the boxplot.
Competing endogenouse RNA (ceRNA) network analysis of TSPAN7 in pan-cancer
First of all, we identified miRNAs targeting TSPAN7 based on DIANA-microT, miRWalk, miRDB, and miRcode databases, and took the intersections of them as key miRNAs. Then, we explored the correlations between the expressions of key miRNAs and TSPAN7 in pan-cancer based on ENCORI database. Afterwards, we identified the target lncRNAs of key miRNAs as well, and constructed the ceRNA network of TSPAN7, visualized in the form of Cytoscape network and Sankey diagram.
Protein-protein interaction (PPI) analysis of TSPAN7 in pan-cancer
We investigated possible interactions of TSPAN7 with other proteins based on the STRING database, and exhibited the results in a protein-protein interaction (PPI) network.
Clinical correlation analysis of TSPAN7 in pan-cancer
Based on the data of TSPAN7 expression and patients’ survival data including OS, PFS, disease free survival (DFS), and disease specific survival (DSS), we successively conducted K-M survival analysis and univariable Cox regression analysis to explore the associations of TSPAN7 with patients’ survival in pan-cancer based on “survival” R package. Besides, we also displayed TSPAN7 expression in diverse stages of cancer, and used Wilcox test to reveal the correlations between TSPAN7 and clinical stages. Moreover, for four types of tumors that commonly metastasized to bones, including BLCA, BRCA, MESO, and PRAD, we conducted differential expression analysis between the primary and metastatic tumor to discover the potential role of TSPAN7 in tumor metastasis. Last but not least, we also exhibited the differential expression level of TSPAN7 across four different molecular subtypes of BRCA and the corresponding normal tissues, suggesting TSPAN7’s value in tumor heterogeneity.
Estimation of STromal and Immune cells in MAlignant tumors using expression data (ESTIMATE) analysis
Tumors are usually consisted of tumor cells and the adjacent tumor microenvironment (TME), and TME is composed of stromal cells, immune cells, etc. [33, 34]. In our study, the ESTIMATE algorithm was employed to calculate the immune scores and stromal scores of 33 types of cancers in TCGA [35]. Subsequently, Spearman correlation analysis was conducted between TSPAN7 expression and immune scores or stromal scores. Generally speaking, more abundant immune and stromal components there were, lower the tumor purity was. Tumor purity was negatively correlated with immune scores and stromal scores. Similarly, the immune cell infiltration was also evaluated by the ESTIMATE algorithm, and correlations of TSPAN7 expression with immune infiltration were tested by Spearman correlation analysis as well.
Tumor mutation burden (TMB) analysis, microsatellite instability (MSI) analysis, cBioPortal analysis, and gene set cancer analysis (GSCA)
The TMB in 33 types of cancers was tested by “maftools” based on the TCGA Whole Exome Sequencing (WES) data [36], and the MSI scores of them came from Sameek Roychowdhury et al.’s study [22]. Subsequently, we used the method of Spearman correlation analysis to explore the relationships between TSPAN7 expression and TMB or MSI, visualizing the results in two radar maps. cBioPortal was applied to further reveal the genomic relevance of TSPAN7 (https://www.cbioportal.org/) [23]. We selected 10 pan-cancer studies as our database [37,38,39,40,41,42,43,44,45,46], and analyzed CNVs as well as mutation sites of TSPAN7. Afterwards, based on GSCA platform, we further identified the mutation profiles of TSPAN7 concerning SNV, CNV, and methylation, and explored the survival differences among patients with various TSPAN7 mutation types.
Co-expression analysis of TSPAN7 and immune gene in pan-cancer
Across 33 cancer types in TCGA, we used Spearman correlation analysis to analyze the correlations between TSPAN7 expression and 47 immune-related genes, including TNFRSF9, CD44, CD86, etc. The above results were illustrated in a heatmap, with the yellow triangle referring to the P-value, and the red or blue triangle referring to the correlation coefficient.
Immune infiltration analysis
TIMER2.0 used methods of CIBERSORT, TIMER, quanTIseq, xCell, MCP-counter, and EPIC algorithm to estimate the immune cell infiltration [24]. Subsequently, we used the method of Spearman correlation analysis to discover the associations between TSPAN7 and immune cell infiltration.
Cytotoxic T-lymphocyte infiltration and survival analysis
We used TIDE to explore correlations of TSPAN7 with CTLs and patients’ survival [25]. Based on the E-MTAB-179 (https://www.ebi.ac.uk/biostudies/arrayexpress/studies/E-MTAB-179), GSE12417_GPL570 [47], METABRIC [48], TCGA [49] database, we first exhibited the relevance of TSPAN7 with CTLs in the form of scatter plots and fitting curves. Then, we divided the samples into high- or low-TSPAN7 subgroups based on the median value of TSPAN7 expression, as well as separating the samples into high- or low-CTL subgroups based on the expressions of CTL biomarkers, including PRF1, CD8A, CD8B, GZMA, and GZMB. Finally, we obtained the K-M survival curves of these subgroups, to further reveal the impact of TSPAN7 on CTLs’ function.
Drug sensitivity prediction
We collected the half maximal inhibitory concentration (IC50) data and the corresponding mRNA expression of TSPAN7 in certain cell lines from GDSC, CTRP, and CellMiner database. For GDSC and CTRP database, Pearson correlation analysis was conducted between TSPAN7 expression and IC50 of certain small molecule drugs based on GSCA online platform. For CellMiner database, Pearson correlation analysis was conducted between TSPAN7 expression and Z scores of certain drugs based on “cor.test” function. Higher Z scores indicated higher drug sensitivity.
Gene set enrichment analysis (GSEA)
We applied GSEA to reveal the enriched KEGG pathways related to TSPAN7 expression across 33 cancer types in TCGA. Specifically, we first divided the pan-cancer samples from TCGA database into high- and low-TSPAN7 subgroups based on the median TSPAN7 expression, and re-ordered these samples according to the fold change of differential expressions. Then, we conducted functional enrichment analysis by comparing the gene expression profiles of certain KEGG pathways [32] with the gene expression profiles of our pan-cancer samples through the clusterProfiler R package (version 4.12.0, https://yulab-smu.top/biomedical-knowledge-mining-book/(docs)https://doi.org/10.1016/j.xinn.2021.100141(paper)) [50]. The cancer types with P-value < 0.05 were selected and the top five KEGG pathways with the highest enrichment scores of them were exhibited, respectively.
Statistical analysis
The statistical analysis was conducted with R version 4.3.2 software. Continuous variables having a normal distribution were described by mean ± standard deviation, while continuous variables with an abnormal distribution were described by median (range). Two-tailed P-values < 0.05 and false discovery rate (FDR) < 0.05 were regarded as statistically significant.
Results
Demographic information and clinical features of the cohort including 370 patients with kidney neoplasm
The comprehensive analysis steps of our study were illustrated in Fig. 1A and Figure S1. We selected 370 patients with kidney neoplasm, who received surgery from 2018 to 2022 to constitute a large retrospective cohort. The inclusion and exclusion criteria of the clinical cohort were shown in Fig. 1B. Table S1 illustrated the demographic information and clinical features of the cohort. As for the histopathological classification of neoplasm, 88.92% of the patients were diagnosed with KIRC, while 4.86%, 2.16%, and 4.05% of them were diagnosed with KIRP, KICH, and other kidney neoplasms, respectively. Considering the Fuhrman nuclear stage, patients with stage 1, 2, 3, 4 accounted for 18.65%, 63.24%, 13.78%, and 4.32% of all patients, separately. Besides, the majority of the patients were diagnosed with kidney neoplasm of an early clinical stage, as “Stage 1” (76.22%) accounted for the majority of the pathological sections. Regarding the patients’ survival, 74.59% of them were alive, and most of the patients were alive without the progression of the disease (69.73%).
The analysis flow and the results of immunohistochemical (IHC) staining and scoring. (A) The workflow highlighted the important steps of our analysis. (B) The inclusion and exclusion criteria of the retrospective cohort were illustrated. (C) The IHC scores of TSPAN7 in kidney neoplasm tissues were higher than those in normal tissues (P-value = 0.0019). (D) Several IHC staining results of kidney neoplasm tissues and normal tissues were exhibited. Abbreviations: ceRNA, competing endogenouse RNA; PPI, protein-protein interaction
Immunohistochemical staining and scoring of TSPAN7 in kidney neoplasm tissues and normal tissues
IHC staining was employed on the tissue sections obtained from the 370 patients in the cohort (Fig. 1B). The mean values of the IHC scores of TSPAN7 in tumor and normal tissues were 10.45 and 9.77, respectively, suggesting an up-regulation of TSPAN7 protein expression in kidney neoplasm (P-value = 0.0019) (Table S1 and Fig. 1C). The IHC scores were graded as 0–9 (low), 10–12 (high) by the cut-off value (Figure S2A). Specifically, the distribution of tumor tissue sections within low- and high-IHC score subgroups was 31.62% and 67.84% while the distribution of normal tissues sections was 33.51% and 48.65%, separately (Table S1). In Fig. 1D, we exhibited several IHC staining pictures of the tumor and normal tissue sections from the cohort.
Clinical correlations of TSPAN7 in kidney neoplasm
The specific clinicopathological data of each patient was shown in the heatmap in Fig. 2A. Then, through K-M survival analysis, we discovered that TSPAN7 protein expression in tumor tissues harbored significant correlation with the OS (P-value = 0.009) and PFS (P-value = 0.009) of patients with kidney neoplasm, and high TSPAN7 protein expression usually led to poor clinical outcomes (Fig. 2B-C). Through Chi-square tests, we further revealed that the IHC scores of TSPAN7 in tumor tissues were significantly associated with three variables, including the IHC scores of TSPAN7 in normal tissues (P-value < 0.001), PFS classification (P-value < 0.05), and OS classification (P-value < 0.01) (Figure S2B and Table S1).
Exploring the clinical correlation of TSPAN7 in kidney neoplasm
(A) The demographic information and clinical features of the cohort including 370 patients with kidney neoplasm were illustrated in the heatmap. (B-C) The Kaplan-Meier survival curves showed that high expression of TSPAN7 was usually associated with lower overall survival (OS) (P-value = 0.009) and progression free survival (PFS) (P-value = 0.009) in kidney neoplasm. (D) High IHC scores of TSPAN7 in tumor tissues served as a risk factor of OS (hazard ratio (HR) = 5.66, confidential interval (CI) = 1.155–27.8, P-value = 0.0326) in kidney neoplasm. *, P-value < 0.05; **, P-value < 0.01; ***, P-value < 0.001. Abbreviations: OS, overall survival; PFS, progression free survival; KIRC, Kidney clear cell carcinoma; KICH, kidney chromophobe; KIRP, kidney renal papillary cell carcinoma.
Subsequently, we incorporated seven variables to conduct multivariable Cox regression analysis. From the forest plot, we could identify high TSPAN7 protein expression as an independent risk factor of the OS of patients with kidney neoplasm. The hazard ratio (HR) value of high IHC scores of TSPAN7 in tumor tissues within the multivariable Cox regression model was 5.66, with confidential interval (CI) = 1.155–27.8, P-value = 0.0326 (Fig. 2D). The nomogram was also constructed (Figure S2C). Based on the median value of the risk scores, we separated the patients into high- or low-risk subgroups (Figure S2D). The survival time, OS censor, and risk score of each patient were shown in the scatter plot (Figure S2E). In the K-M survival analysis, patients in the low-risk subgroup usually harbored better prognosis (P-value < 0.001) (Figure S2F). Last but not least, residual analysis, calibration curves, and ROC were applied for model diagnosis. Through residual analysis, the proportional hazards assumption of the multivariable Cox regression model was tested (Figure S2G-H). In the calibration curves, the observed OS was approximately consistent with the predicted OS at 1 year, 2 years, 3 years, 4 years, and 5 years (Figure S2I). Notably, in the ROC analysis, the area under the curve (AUC) for OS at 1 year, 2 years, 3 years, 4 years, and 5 years were 0.971, 0,975, 0,939, 0.982, and 0.980, respectively, suggesting the outstanding prognostic prediction value of the multivariable Cox regression models (Figure S2J).
Differential expression, ceRNA network, and PPI network of TSPAN7 in pan-cancer
Considering the differential expression and strong clinical correlations of TSPAN7 in kidney neoplasm, we further questioned if TSPAN7 also played a vital role in pan-cancer. The abbreviations of 33 cancer types in TCGA were shown in Table S2. At the transcriptomic level, TSPAN7 expression in tumor tissues was lower than that in normal tissues in BLCA, BRCA, CESC, COAD, GBM, HNSC, KIRC, KIRP, LIHC, LUAD, LUSC, PRAD, READ, SARC, STAD, THCA, and UCEC. The results were opposite when considering KICH and PCPG (Fig. 3A). Subsequently, we searched for TSPAN7-targeted miRNAs from DIANA-microT, miRWalk, miRDB, and miRcode databases, and identified hsa-miR-140, hsa-miR-302 as key TSPAN7-targeted miRNAs by taking the intersections of all miRNAs (Figure S3A). The expression level of hsa-miR-140-3p, hsa-miR-140-5p, hsa-miR-302a-3p, hsa-miR-302a-5p, hsa-miR-302b-3p, hsa-miR-302b-5p, hsa-miR-302c-3p harbored close correlations with TSPAN7 in various cancers (Figure S3B). Finally, integrated with hsa-miR-140-targeting- and hsa-miR-302-targeting lncRNAs, we revealed the TSPAN7-miRNAs-lncRNAs interactions and constructed the ceRNA network of TSPAN7 (Figure S3C and Fig. 3B). Moreover, we also investigated the possible interactions of TSPAN7 with other proteins. At the proteomic level, TSPAN7 was closely associated with OPHN1, IL1RAPL1, GRIA3, PICK1, GRIA2, HSPA5, TSPAN32, CD81, ITGB1, IGSF3, etc. (Fig. 3C). The ceRNA and PPI network might indicate the possible mechanisms of TSPAN7’s role in cancer progression.
Differential expression, protein–protein interaction (PPI) network, and survival analysis of TSPAN7 in pan-cancer. (A) The expression of TSPAN7 in tumor was down-regulated in BLCA (P-value < 0.001), BRCA (P-value < 0.001), CESC (P-value < 0.01), COAD (P-value < 0.001), GBM (P-value < 0.01), HNSC (P-value < 0.05), KIRC (P-value < 0.001), KIRP (P-value < 0.001), LIHC (P-value < 0.001), LUAD (P-value < 0.001), LUSC (P-value < 0.001), PRAD (P-value < 0.001), READ (P-value < 0.001), SARC (P-value < 0.05), STAD (P-value < 0.001), THCA (P-value < 0.001), and UCEC (P-value < 0.001), however, up-regulated in KICH (P-value < 0.001) and PCPG (P-value < 0.05). (B) The ceRNA network was constructed by Cytoscape, illustrating the possible TSPAN7-miRNAs-lncRNAs interactions. (C) The PPI network illustrated the close association of TSPAN7 with OPHN1, IL1RAPL1, GRIA3, PICK1, GRIA2, HSPA5, TSPAN32, CD81, ITGB1, and IGSF3.. (D) The Kaplan-Meier survival curves showed that low expression of TSPAN7 was associated with low probability of OS in KIRC (P-value < 0.001). Besides, low expression of TSPAN7 was also correlated with lower PFS in KICH (P-value = 0.021), KIRC (P-value < 0.001), but higher PFS in KIRP (P-value < 0.001). Moreover, TSPAN7 was negatively related to the disease free survival (DFS) of patients in KIRP (P-value = 0.006). As for disease specific survival (DSS), patients with high expression of TSPAN7 usually harbored lower DSS in KIRP (P-value < 0.001), but higher DSS in KIRC (P-value < 0.001). (E) TSPAN7 served as a risk factor of OS in BLCA (HR = 1.144, CI = 1.037–1.263, P-value = 0.007), HNSC (HR = 1.123, CI = 1.027–1.228, P-value = 0.011), STAD (HR = 1.181, CI = 1.010–1.380, P-value = 0.037), UCEC (HR = 1.227, CI = 1.071–1.406, P-value = 0.003), however, served as a protective factor in KICH (HR = 0.456, CI = 0.278–0.746, P-value = 0.002), KIRC (HR = 0.650, CI = 0.571–0.741, P-value < 0.001), LGG (HR = 0.523, CI = 0.433–0.632, P-value < 0.001), LUAD (HR = 0.866, CI = 0.774–0.968, P-value = 0.012), OV (HR = 0.908, CI = 0.832–0.991, P-value = 0.030), PAAD (HR = 0.679, CI = 0.561–0.821, P-value < 0.001). As for PFS, TSPAN7 acted as a risk factor of ACC (HR = 1.289, CI = 1.004–1.656, P-value = 0.047), HNSC (HR = 1.099 CI = 1.004–1.203, P-value = 0.041), while played an opposite role in KICH (HR = 0.532, CI = 0.370–0.763, P-value < 0.001), KIRC (HR = 0.681, CI = 0.602–0.770, P-value < 0.001), LGG (HR = 0.533, CI = 0.444–0.641, P-value < 0.001), LUAD (HR = 0.913, CI = 0.836–0.997, P-value = 0.043), OV (HR = 0.910, CI = 0.839–0.986, P-value = 0.022), PAAD (HR = 0.690, CI = 0.581–0.820, P-value < 0.001), SARC (HR = 0.814, CI = 0.701–0.945, P-value = 0.007), and THCA (HR = 0.775, CI = 0.612–0.982, P-value = 0.035). Moreover, TSPAN7 was protective of DFS in LUAD (HR = 0.782, CI = 0.673–0.908, P-value = 0.001), OV (HR = 0.891, CI = 0.796–0.998, P-value = 0.047), PAAD (HR = 0.505, CI = 0.335–0.761, P-value = 0.001), and SARC (HR = 0.720, CI = 0.574–0.903, P-value = 0.005), but served as a risk factor of HNSC (HR = 1.408, CI = 1.056–1.878, P-value = 0.020). As for DSS, while TSPAN7 was a risk factor in BLCA (HR = 1.189, CI = 1.064–1.330, P-value = 0.002), KIRP (HR = 1.246, CI = 1.013–1.533, P-value = 0.037), LIHC (HR = 1.262, CI = 1.005–1.585, P-value = 0.046), and UCEC (HR = 1.209, CI = 1.028–1.422, P-value = 0.021), it played a protective role in KICH (HR = 0.348, CI = 0.197–0.615, P-value < 0.001), KIRC (HR = 0.578, CI = 0.499–0.669, P-value < 0.001), LGG (HR = 0.435, CI = 0.345–0.547, P-value < 0.001), LUAD (HR = 0.881, CI = 0.781–0.994, P-value = 0.040), OV (HR = 0.909, CI = 0.828–0.998, P-value = 0.046), and PAAD (HR = 0.702, CI = 0.570–0.865, P-value < 0.001). *, P-value < 0.05; **, P-value < 0.01; ***, P-value < 0.001. Abbreviations: DFS, disease free survival; DSS, disease specific survival
Clinical correlations of TSPAN7 in pan-cancer
We further studied the associations between TSPAN7 and patients’ survival in pan-cancer. Through K-M survival analysis, we discovered that low TSPAN7 expression was typically associated with decreased OS in KIRC, LGG, LUAD, and PAAD. Besides, patients with lower expression level of TSPAN7 usually harbored lower PFS in KICH, KIRC, LGG, PAAD, SARC, and THCA, except for ACC and KIRP. As for DFS, TSPAN7 expression was negatively associated with the DFS of patients in DLBC, KIRP, but was positively correlated with LUAD, PAAD, and SARC. Moreover, TSPAN7 was a prognostic prediction index of low DSS in KIRP and LIHC. The results were opposite in KIRC, LGG, and PAAD (Fig. 3D and Figure S4). Subsequently, the univariable Cox regression analysis was applied, and the results were shown in the forest plots. TSPAN7 served as a risk factor in BLCA, HNSC, STAD, and UCEC, but was a protective factor in KICH, KIRC, LGG, LUAD, OV, and PAAD. As for PFS, TSPAN7 was a risk factor of ACC, HNSC, while served as a protective factor of KICH, KIRC, LGG, LUAD, OV, PAAD, SARC, and THCA. In addition, TSPAN7 was a protective factor of DFS in LUAD, OV, PAAD, and SARC, but a risk factor of HNSC. As for DSS, the role of TSPAN7 was two-sided. While it was a risk factor in BLCA, KIRP, LIHC, and UCEC, it functioned as a protective role in KICH, KIRC, LGG, LUAD, OV, and PAAD (Fig. 3E).
Besides, TSPAN7 was positively correlated with the clinical stage of BLCA, KIRP, and MESO, but was negatively associated with that of BRCA, KIRC, and THCA (Figure S5A). For four types of tumors which usually metastasized to bones, including BLCA, BRCA, MESO, and PRAD, the expression level of TSPAN7 did not show a statistical difference between the primary and metastatic tumor (Figure S5B). Last but not least, the expression of TSPAN7 varied significantly among different molecular subtypes of BRCA (Figure S5C).
The correlations of TSPAN7 with tumor purity in pan-cancer
To further reveal the molecular mechanism of TSPAN7’s role in the development of pan-cancer, we conducted the following research. First of all, we used the ESTIMATE algorithm to calculate the immune score and stromal score of tumors. Generally speaking, TSPAN7 was negatively correlated with immune scores in most types of cancers, including ACC, GBM, KIRC, LGG, LUSC, OV, and THCA, except for BRCA, KIRP, PAAD, and PRAD. However, TSPAN7 harbored a positive correlation with stromal scores in most cancer types, including BLCA, BRCA, CESC, HNSC, KIRP, LAML, PAAD, PRAD, STAD, TGCT, and THYM, except for ACC, GBM, LGG (Fig. 4A).
The correlations of TSPAN7 with tumor purity and tumor genomics in pan-cancer. (A) The scatter plots and the corresponding fitting curves illustrated the correlations of TSPAN7 with the immune score and stromal score in pan-cancer. TSPAN7 harbored a negative correlation with the immune scores in most types of tumors, including ACC (R = -0.59, P-value < 0.001), GBM (R = -0.36, P-value < 0.001), KIRC (R = -0.34, P-value < 0.001), LGG (R = -0.53, P-value < 0.001), LUSC (R = -0.18, P-value < 0.001), OV (R = -0.3, P-value < 0.001), and THCA (R = -0.27, P-value < 0.001), except for BRCA (R = 0.31, P-value < 0.001), KIRP (R = 0.21, P-value < 0.001), PAAD (R = 0.32, P-value < 0.001), and PRAD (R = 0.53, P-value < 0.001). As for stromal scores, TSPAN7 was positively correlated with the stromal scores in most types of cancers, including BLCA (R = 0.23, P-value < 0.001), BRCA (R = 0.44, P-value < 0.001), CESC (R = 0.21, P-value < 0.001), HNSC (R = 0.2, P-value < 0.001), KIRP (R = 0.41, P-value < 0.001), LAML (R = 0.27, P-value < 0.001), PAAD (R = 0.27, P-value < 0.001), PRAD (R = 0.69, P-value < 0.001), STAD (R = 0.37, P-value < 0.001), TGCT (R = 0.6, P-value < 0.001), and THYM (R = 0.45, P-value < 0.001), except for ACC (R = -0.44, P-value < 0.001), GBM (R = -0.4, P-value < 0.001), LGG (R = -0.44, P-value < 0.001). (B) The radar map highlighted the negative correlation of TSPAN7 with TMB in BLCA (P-value < 0.001), BRCA (P-value < 0.001), COAD (P-value < 0.001), DLBC (P-value < 0.05), ESCA (P-value < 0.001), KIRC (P-value < 0.001), KIRP (P-value < 0.001), LGG (P-value < 0.001), LIHC (P-value < 0.05), LUAD (P-value < 0.001), PAAD (P-value < 0.001), PRAD (P-value < 0.001), READ (P-value < 0.05), SARC (P-value < 0.001), STAD (P-value < 0.001), THCA (P-value < 0.001), UCEC (P-value < 0.001), and UCS (P-value < 0.05). (C) The radar map highlighted the negative correlation of TSPAN7 with MSI in BLCA (P-value < 0.05), COAD (P-value < 0.001), SARC (P-value < 0.05), SKCM (P-value < 0.05), STAD (P-value < 0.001), and UCEC (P-value < 0.001). (D) The major copy number alterations of TSPAN7 were shallow deletion. (E) The mutation site of TSPAN7 was exhibited in the plot. *, P-value < 0.05; **, P-value < 0.01; ***, P-value < 0.001
The correlations of TSPAN7 with tumor genomics in pan-cancer
As for genomics, in Fig. 4B, we could find that TSPAN7 was negatively associated with TMB in most types of cancers, including BLCA, BRCA, COAD, DLBC, ESCA, KIRC, KIRP, LGG, LIHC, LUAD, PAAD, PRAD, READ, SARC, STAD, THCA, UCEC, and UCS. In the MSI radar map, we also identified the negative association between TSPAN7 and MSI in BLCA, COAD, SARC, SKCM, STAD, and UCEC (Fig. 4C). Besides, through cBioPortal analysis, we also discovered the main type of CNVs of TSPAN7 as shallow deletion (Fig. 4D). The mutation site of TSPAN7 was also exhibited in Fig. 4E. Employing GSCA, we further explored the SNV, CNV, and methylation profiles of TSPAN7. The main SNV type of TSPAN7 across various cancer types were “C > T” (Figure S6A), and there were no statistically significant differences between the survival of SNV and wildtype TSPAN7 (Figure S6B). The CNV of TSPAN7 was negatively correlated with its mRNA expression in KIRP and SARC, but was positively associated with its mRNA expression in PAAD, PRAD, and OV (Figure S6C). Regarding the CNV subtypes, we observed more hetezygous amplification of TSPAN7 in ACC, KIRP, SARC, UCS, and more hetezygous deletion in CHOL, ESCA, KICH, OV (Figure S6D). Besides, there were also more homozygous amplification of TSPAN7 in DLBC, SARC, UCS, but more homozygous deletion in ESCA, HNSC, OV (Figure S6E). As for patients’ survival, the CNV of TSPAN7 was significantly correlated with OS in KIRP, PAAD, PRAD, and UCEC, with PFS in KIRP, PAAD, THYM, and UCEC, with DSS in KIRP, LUAD, PAAD, PRAD, THCA, and UCEC, with DFS in COAD, KIRP, and UCEC (Figure S6F). In consideration of methylation, it had negative correlations with the mRNA expression of TSPAN7 in CESC, COAD, ESCA, HNSC, KICH, KIRC, LAML, LUAD, LUSC, PCPG, SKCM, STAD, OV, UCEC, UCS, UVM, except for BRCA (Figure S6G). The methylation of TSPAN7 served as a risk factor of OS in PAAD, of PFS in KIRP, PRAD, of DSS in PAAD, however, served as a protective factor of OS in COAD, MESO, of PFS in COAD, of DSS in COAD, ESCA, STAD (Figure S6H).
The correlations of TSPAN7 with tumor immunity in pan-cancer
In the following studies, we explored the associations of TSPAN7 with tumor immunology and immunotherapy. Among 33 types of cancer in TCGA database, TSPAN7 showed a positive co-expression with both the immune inhibitory genes and stimulatory genes in BRCA, KIRP, LAML, LIHC, PAAD, PRAD, STAD, etc., but was negatively correlated with them in ACC, GBM, KICH, KIRC, LGG, LUSC, OV, THCA, UCEC, etc. The immune correlations of TSPAN7 in pan-cancer were two-sided and complicated (Fig. 5A).
The correlations of TSPAN7 with immune gene sets and immune cell infiltration in pan-cancer. (A) TSPAN7 was positively associated with both the immune inhibitory genes and stimulatory genes in BRCA, KIRP, LAML, LIHC, PAAD, PRAD, STAD, etc., but showed a negative correlation with them in ACC, GBM, KICH, KIRC, LGG, LUSC, OV, THCA, UCEC, etc. (B) TSPAN7 was negatively associated with the infiltration of B cells memory (R = -0.16, P-value < 0.001), macrophages M0 (R = -0.29, P-value < 0.001), plasma cells (R = -0.17, P-value < 0.001), T cells CD4 memory activated (R = -0.2, P-value < 0.001), T cells CD8 (R = -0.18, P-value < 0.001), T cells follicular helper (R = -0.16, P-value < 0.001), T cells gamma delta (R = -0.18, P-value < 0.001), and T cells regulatory (R = -0.37, P-value < 0.001), but positively associated with the infiltration of B cells naïve (R = 0.22, P-value < 0.001), dendritic cells resting (R = 0.17, P-value < 0.001), mast cells resting (R = 0.44, P-value < 0.001), monocytes (R = 0.18, P-value < 0.001), NK cells resting (R = 0.34, P-value < 0.001), and T cells CD4 memory resting (R = 0.15, P-value < 0.001) in KIRC. TSPAN7 was positively associated with the infiltration of B cells naïve (R = 0.29, P-value < 0.001), macrophages M1 (R = 0.42, P-value < 0.001), and T cells CD8 (R = 0.22, P-value < 0.001), except for mast cells resting (R = -0.22, P-value < 0.001) in KIRP. *, P-value < 0.05; **, P-value < 0.01; ***, P-value < 0.001
Then, we used the ESTIMATE algorithm as well as the TIMER2.0 database to further discover the associations of TSPAN7 with immune cell infiltration. Notably, TSPAN7 played an immune inhibiting role in most types of cancers, especially for KIRC, GBM, THCA, and UCEC. In KIRC, TSPAN7 exhibited a significantly negative correlation with the infiltration of macrophages (macrophages M0), myeloid cells, B cells (B cells memory, plasma cells), and T cells (T cells CD4 memory activated, T cells CD8, T cells follicular helper, T cells gamma delta, T cells regulatory), but a weak positive correlation with NK cell infiltration (NK cell resting). In GBM, TSPAN7 was negatively associated with the infiltration of macrophages, myeloid cells, CD8 + T cells, but was positively associated with B cells. In THCA, TSPAN7 was negatively associated with the infiltration of macrophages, myeloid cells (dendritic cells activated, monocytes), and T cells (T cells CD4 memory activated, T cells regulatory). In UCEC, TSPAN7 also harbored a negative correlation with the infiltration of macrophages, myeloid cells, and T cells (T cells CD4 memory activated). However, in KIRP, TSPAN7 seemed to played an opposite role, as tumors with high TSPAN7 expression were enriched with macrophages (macrophages M1), B cells (B cells naïve), and CD8 + T cells (T cells CD8). Interestingly, TSPAN7 was strongly positively correlated with endothelial cells and cancer-associated fibroblasts in almost all types of tumors (Fig. 5B, Figure S7, and Figure S8).
CTLs were one of the most important components in tumor immunity. Thus, we retrieved the TIDE database to further explore the association of TSPAN7 with CTLs (Figure S9A). From Figure S9B, we could find that TSPAN7 was positively correlated with CTLs in BRCA, but was negatively correlated in UCEC. Regarding the survival status, patients with higher TSPAN7 expression usually harbored better prognosis in LAML, but had worse prognosis in UCEC (Figure S9C). However, there was no statistical difference between the survival of patients with high- or low-CTL infiltration in the high- and low-TSPAN7 subgroups, indicating that TSPAN7 was not statistically associated with the CTL dysfunction phenotype in our study (Figure S9D).
The correlations of TSPAN7 with drug sensitivity in pan-cancer
Ulteriorly, we discovered that TSPAN7 expression had significant correlation with drug sensitivity in pan-cancer based on GDSC (Fig. 6A), CTRP (Fig. 6B), and CellMiner database (Fig. 6C). Notably, high expression of TSPAN7 was related with high drug sensitivity of most drugs, specifically for Navitoclax and Vorinostat, which served as the top associated drugs in all three databases. Exceptionally, the drug sensitivity of Dasatinib was found to be negatively correlated with TSPAN7 expression. The above results suggested that TSPAN7 might function in drug therapies of cancers and TSPAN7-target treatment was promising in improve the therapeutic effect of anti-cancer drugs.
The correlations of TSPAN7 with drug sensitivity in pan-cancer. (A) In GDSC database, TSPAN7 expression was positively correlated with IC50 of 17-AAG, Afatinib, AUY922, AZD6482, BEZ235, Bleomycin, Bleomycin (50 μm), CGP-60,474, Dasatinib, Docetaxel, Elesclomol, Epothilone B, Midostaurin, NU-7441, piperlongumine, QL-VIII-58, Shikonin, TGX221, Tipifarnib, WZ-1-84, Z-LLNle-CHO, but was negatively correlated with IC50 of BMS345541, BX-912, CX-5461, GSK1070916, NPK76-II-72-1, TL-2-105, Vorinostat, ZM-447,439. (B) In CTRP database, TSPAN7 expression was positively correlated with IC50 of Dasatinib, YM-155, but was negatively correlated with IC50 of ABT-737, Apicidin, BI-2536, BRD-A94377914, BRD-K70511574, CD-437, Ceranib-2, Cucurbitacin I, Entinostat, GSK461364, ISOX, JQ-1, Leptomycin B, Linsitinib, LRRK2-IN-1, LY-2,183,240, Marinopyrrole A, Mitomycin, Nakiterpiosin, Navitoclax, Neuronal differentiation inducer III, Nutlin-3, Panobinostat, Phloretin, SCH-79,797, Tacedinaline, Triazolothiadiazine, Vorinostat. (C) In CellMiner database, TSPAN7 expression was positively correlated with compound activity of Nelarabine (R = 0.825, P-value < 0.001), Zalcitabine (R = 0.757, P-value < 0.001), Methylprednisolone (R = 0.681, P-value < 0.001), Chelerythrine (R = 0.548, P-value < 0.001), ANCITABINE HYDROCHLORIDE (R = 0.510, P-value < 0.001), Pipobroman (R = 0.489, P-value < 0.001), Ribavirin (R = 0.485, P-value < 0.001), Cytarabine (R = 0.473, P-value < 0.001), XK-469 (R = 0.470, P-value < 0.001), Fludarabine (R = 0.469, P-value < 0.001), Barasertib (R = 0.462, P-value < 0.001), ST-3595 (R = 0.456, P-value < 0.001), IDOXURIDINE (R = 0.451, P-value < 0.001), Asparaginase (R = 0.449, P-value < 0.001), PX-316 (R = 0.446, P-value < 0.001), ZM-336,372 (R = 0.440, P-value < 0.001), Dexamethasone Decadron (R = 0.429, P-value < 0.001), Bendamustine (R = 0.399, P-value < 0.01), Thiotepa (R = 0.396, P-value < 0.01), Chlorambucil (R = 0.388, P-value < 0.01), Fluphenazine (R = 0.382, P-value < 0.01), DECITABINE (R = 0.380, P-value < 0.01), Triethylenemelamine(R = 0.380, P-value < 0.01), SNS-314 (R = 0.378, P-value < 0.01), Uracil mustard (R = 0.364, P-value < 0.01), Noscapine (R = 0.359, P-value < 0.01), Hydroxychloroquine Sulfate (R = 0.358, P-value < 0.01), Melphalan (R = 0.357, P-value < 0.01), Navitoclax (R = 0.353, P-value < 0.01), Ifosfamide (R = 0.351, P-value < 0.01), FENRETINIDE (R = 0.343, P-value < 0.01), LMP-400 (R = 0.343, P-value < 0.01), Vorinostat (R = 0.339, P-value < 0.01), Idarubicin (R = 0.338, P-value < 0.01), Dasatinib (R = -0.336, P-value < 0.01). Abbreviations: GDSC, Genomics of Drug Sensitivity in Cancer; CTRP, The Cancer Therapeutics Response Portal; Cor, correlation coefficient
Functional enrichment analysis of TSPAN7 in pan-cancer through GSEA
To further explore the role of TSPAN7 in pan-cancer, we applied GSEA to identify the enriched KEGG pathways in high-TSPAN7 subgroups. Generally speaking, “Calcium signaling pathway”, “Cytosolic DNA sensing pathway”, “Drug metabolism cytochrome P450”, “Metabolism of xenobiotics by cytochrome P450”, “Neuroactive ligand receptor interaction”, “Olfactory transduction”, “Regulation of autophagy”, and “RIG I like receptor signaling pathway” were the most common KEGG pathways in our analysis. Specifically, “Calcium signaling pathway” was up-regulated in KIRP, PRAD, PAAD, and UVM. “Drug metabolism cytochrome P450” and “Metabolism of xenobiotics by cytochrome P450” was up-regulated in TGCT, KIRP, LUAD, and HNSC. “Neuroactive ligand receptor interaction” was up-regulated in KIRP, PAAD, UVM, and SARC. “Olfactory transduction” was down-regulated in CESC, STAD, SARC, but up-regulated in UVM. “Cytosolic DNA sensing pathway”, “Regulation of autophagy” and “RIG I like receptor signaling pathway” was down-regulated in BLCA, CESC, PAAD, and UCS. These enriched KEGG pathways might suggest the molecular biological functions of TSPAN7 in pan-cancer (Fig. 7 and Figure S10).
The enriched signaling pathways of TSPAN7 in pan-cancer. (A) In BLCA, the top five enriched down-regulated KEGG pathways were “Cytosolic DNA sensing pathway”, “DNA replication”, “Nucleotide excision repair”, “Regulation of autophagy”, and “RIG I like receptor signaling pathway”. (B) In CESC, the top five enriched down-regulated KEGG pathways were “Autoimmune thyroid disease”, “Cytosolic DNA sensing pathway”, “Olfactory transduction”, “Regulation of autophagy”, and “RIG I like receptor signaling pathway”. (C) In HNSC, the top five enriched up-regulated KEGG pathways were “Arrhythmogenic right ventricular cardiomyopathy_ARVC”, “Cardiac muscle contraction”, “Hypertrophic cardiomyopathy_HCM”, “Drug metabolism cytochrome P450”, and “Metabolism of xenobiotics by cytochrome P450”. (D) In KIRP, the top five enriched up-regulated KEGG pathways were “Calcium signaling pathway”, “Drug metabolism cytochrome P450”, “Neuroactive ligand receptor interaction”, “PPAR signaling pathway”, and “Retinol metabolism”. (E) In LUAD, the top five enriched up-regulated KEGG pathways were “Adipocytokine signaling pathway”, “Drug metabolism cytochrome P450”, “GnRH signaling pathway”, “Metabolism of xenobiotics by cytochrome P450”, and “Steroid hormone biosynthesis”. (F) In PRAD, the top five enriched up-regulated KEGG pathways were “Calcium signaling pathway”, “Cell adhesion molecules_CAMs”, “Chemokine signaling pathway”, “Cytokine-cytokine receptor interaction”, and “focal adhesion”. (G) In SARC, the four enriched up-regulated KEGG pathways were “Cardiac muscle contraction”, “Dilated cardiomyopathy”, “Hypertrophic cardiomyopathy_HCM”, and “Neuroactive ligand receptor interaction”. However, “Olfactory transduction” was down-regulated in SARC. (H) In STAD, the four enriched up-regulated KEGG pathways were “Adipocytokine signaling pathway”, “Arrhythmogenic right ventricular cardiomyopathy_ARVC”, “Maturity onset diabetes of the young”, and “Renin angiotensin system”. However, “Olfactory transduction” was down-regulated in STAD
Discussion
TSPAN7, a member of TSPANs, is found to be closely associated with tumor progression. Several studies have reported TSPAN7’s role in tumor cell invasion, metastasis, and immunotherapy [11,12,13]. However, the previous studies have contradictory results, and the exact function of TSPAN7 in tumor development is not clear enough. Thus, we conducted a pan-cancer study of TSPAN7 in order to comprehensively reveal the unique role of TSPAN7 across various types of tumors. First of all, we employed IHC staining on a large retrospective cohort consisted of 370 patients with kidney neoplasm, and discovered the differential expression of TSPAN7 between tumor and normal tissues. Clinical correlation analyses were then applied, suggesting the potential function of TSPAN7 in the development of kidney neoplasm. Subsequently, we questioned if TSPAN7 also harbored an important role in pan-cancer level, and successively conducted differential expression analysis, ceRNA analysis, PPI analysis, correlation analysis of TSPAN7 with patients’ survival, clinical stages, tumor purity, tumor genomics, tumor immunology, and drug sensitivity. Last but not least, we also conducted GSEA to discover the enriched signaling pathways of TSPAN7, indicating the possible molecular mechanisms of TSPAN7 in pan-cancer.
TSPAN7 might serve as a potential biomarker across various types of tumors
Previous studies have reported the prognostic prediction value of TSPAN7 in several tumor types. Low TSPAN7 expression was found to be related to poor prognosis in BRCA, GBM, LUAD, KIRC, LIHC, PDAC, SARC, BLCA, and multiple myeloma [13, 51,52,53,54,55,56,57,58]. However, in STAD and osteosarcoma, oppositely, TSPAN7 could promote the progression of tumor, and was usually correlated with worse clinical outcomes [12, 59]. In our study, through IHC staining, we discovered an up-regulation of TSPAN7 in kidney neoplasm tissues compared to normal ones. High TSPAN7 expression was predictive of shorter OS and PFS of patients with kidney neoplasm. In pan-cancer, TSPAN7 expression in tumor tissues was lower than normal tissues in most types of tumors, including BLCA, BRCA, CESC, COAD, GBM, HNSC, KIRC, KIRP, LIHC, LUAD, LUSC, PRAD, READ, SARC, STAD, THCA, and UCEC, except for KICH and PCPG at the transcriptomic level. From the K-M survival analysis and univariable Cox regression analysis, we further identified TSPAN7 as a protective factor of OS in KIRC, LGG, LUAD, PAAD, of PFS in KICH, KIRC, LGG, PAAD, SARC, THCA, of DFS in LUAD, PAAD, SARC, of DSS in KIRC, LGG, PAAD, but as a risk factor of PFS in ACC, of DSS in KIRP, LIHC. Besides, TSPAN7 was also positively correlated with the clinical stage of BLCA, KIRP, and MESO, but was negatively associated with that of BRCA, KIRC, and THCA. Although the function of TSPAN7 in various types of tumors was two-sided, our study still indicated TSPAN7 as a promising biomarker in pan-cancer.
In addition, TSPAN7 might also serve as a potential therapeutic biomarker in various cancers. In our study, we found that high TSPAN7 expression was of significant correlation with high drug sensitivity of Navitoclax and Vorinostat, but oppositely associated to low drug sensitivity of Dasatinib. Navitoclax was a double antagonist of BCL-2 and BCL-XL, and had been approved for patients with 17p-deficient chronic lymphocytic leukemia who had received at least one therapy type [60]. The application of Navitoclax in other solid tumors, especially small cell lung cancer, was also under clinical research [61]. Besides, Vorinostat was a FDA-approved drug for cutaneous T-cell lymphoma [62], and “Vorinostat plus other drugs” therapy was gradually catching more researchers’ attention in treating Hodgkin lymphoma [63, 64]. Last but not least, Dasatinib, a BCR-ABL inhibitor [65], had been widely studied in treatment of metastatic breast cancer [66], small cell lung cancer [67], non-clear cell renal cell carcinoma [68], etc. However, there were no previous studies reporting the correlations between TSPAN7 and the sensitivity of these drugs. Pharmaceutical research concerned TSPAN7 in pan-cancer seemed to be a promising research direction in the future.
Further, we constructed the ceRNA and PPI network to suggest the possible mechanism of TSPAN7 in cancer progression. In ceRNA network, hsa-miR-140 and hsa-miR-302 were identified as two key miRNA family closely associated with TSPAN7. Although there were not previous studies directly reporting the correlations between the two miRNA families and TSPAN7, both of them played an important role in the development of cancers. Especially for hsa-miR-140, it was found that hsa-miR-140 could not only inhibit the proliferation, migration, and invasion of gastric cancer, but also function in the chemoresistance of pancreatic ductal adenocarcinoma [69, 70]. Among the target lncRNAs of key miRNAs, KCNQ1OT1 stands out as the most widely associated one. It was found that KCNQ1OT1 took part in the progression of cholangiocarcinoma through the regulation of miR-140-5p/SOX4 axis [71]. In the PPI network, we found TSPAN7 was possibly interacted with IGSF3, IL1RAPL1, PICK1, GRIA2, and GRIA3 (known interactions from curated databases or experiments), especially for PICK1 with the highest predicted score. However, there were not previous studies of TSPAN7 with the above potential protein partners in the field of cancers. Maria Passafaro et al. reported that TSPAN7 could regulate excitatory synapse development and AMPAR trafficking through PICK1 in X-linked intellectual disability [72]. Deeper investigations of the ceRNA and PPI network are conducive to reveal the possible mechanisms of TSPAN7 in cancer progression.
TSPAN7 was possibly associated with immune infiltration and immunotherapy across various types of tumors
Moreover, we explored the correlations of TSPAN7 with tumor purity, tumor genomics, and tumor immunology. Notably, TSPAN7 was found to have a close correlation with immune infiltration and immunotherapy across various types of tumors. Previous studies have validated our findings. In GBM, TSPAN7 was negatively associated with the infiltration of cancer-associated M2 macrophages, and the expression of CTLA-4, PD-1, and PD-L1 [13]. In BRCA, TSPAN7 was reported to be associated with the T and B cell infiltration as well as the occurrence of tertiary lymphoid structures, which was predictive of the therapeutic effect of immune checkpoint inhibitors [51]. In our study, we discovered that TSPAN7 was positively co-expressed with both the immune inhibitory genes and stimulatory genes in BRCA, KIRP, LAML, LIHC, PAAD, PRAD, STAD, and negatively correlated with them in ACC, GBM, KICH, KIRC, LGG, LUSC, OV, THCA, UCEC. Considering immune cell infiltration, TSPAN7 usually functioned as an immune inhibitory factor in pan-cancer, especially for KIRC, GBM, THCA, and UCEC. Among these four types of tumors, TSPAN7 was mostly negatively associated with the infiltration of macrophages, myeloid cells, and T cells. Macrophages were reported to stimulate angiogenesis, malignant cell invasion, and inhibit the anti-tumor immunity, thus promoting the initiation and progression of tumor [73]. However, T cells usually served as a tumor-killing actor, and were associated with favorable clinical outcomes. Among T cells, CD4 + T cells could communicate with B cells, antigen presenting cells (APCs), and other immune cells, in order to facilitate their anti-tumor functions [74, 75]. Most importantly, CD4 + T cells could contribute to the proliferation and differentiation of CD8 + T cells, and enhance the tumor-killing role of CD8 + T cells through various ways [76, 77]. CTLs, the main subtypes of CD8 + T cells, were of the greatest importance in tumor immunity. CTLs could secrete perforin and apoptosis-related cytokines to kill tumor cells [78]. The above results suggested the close relationships between TSPAN7 and macrophages, myeloid cells, T cells, and identified TSPAN7 as a promising immunotherapeutic target for tumors. Apart from this, it was noteworthy that TSPAN7 was positively associated with the infiltration of endothelial cells and cancer-associated fibroblasts in almost all cancer types in TCGA. Although there was no study reporting the relationship between TSPAN7 and cancer-associated fibroblasts, TSPAN7 was found to harbor a far higher expression within endothelial cells than tumor cells in KIRC [53]. In BRCA, TSPAN7 was reported to be associated with the formation of high endothelial venules, which could recruit naïve lymphocytes to lymph nodes and enhance the anti-tumor immunity [51].
Nonetheless, CTL dysfunction and exclusion were the main reason for the failure of anti-tumor immunity [79]. Thus, we conducted our subsequent analysis among BRCA, SKCM, UCEC, LAML, and neuroblastoma based on the TIDE database. We discovered that patients with high TSPAN7 expression were usually accompanied by enriched CTLs in BRCA, but low infiltration of CTLs in UCEC. However, there were no statistical correlations between TSPAN7 and CTL dysfunction.
Apart from this, in our study, we also found that TSPAN7 was closely associated with tumor genomics. TSPAN7 exhibited a negative correlation with TMB in most cancer types including BLCA, BRCA, COAD, DLBC, ESCA, KIRC, KIRP, LGG, LIHC, LUAD, PAAD, PRAD, READ, SARC, STAD, THCA, UCEC, UCS, and was negatively correlated with MSI in BLCA, COAD, SARC, SKCM, STAD, UCS. Besides, we also revealed the close relationships between TSPAN7 and genomics instability in pan-cancer through cBioPortal analysis and GSCA. Notably, the genomic instability of tumors was of close associations with immunotherapy. It has been reported that tumors with large amounts of somatic mutations driven by mismatch-repair defects were more sensitive to PD-1 targeted therapy [80, 81], and MSI might serve as a reliable biomarker for the therapeutic effect of PD-1 blockade [82]. TSPAN7 possibly played a role in tumor immunotherapy by regulating tumor genomics.
Then, we used GESA and identified several enriched signaling pathways of TSPAN7, which were possibly associated with its role in tumor immunity across various types of tumors. “Cytosolic DNA sensing pathway” is involved in the recognition of double-stranded cytosolic DNA, and functioned in the innate immune system [83]. Cyclic GMP-AMP synthase (cGAS) is one of the DNA sensors in the pathway, and could activate STING to initiate type I interferon (IFN) response, which could promote the maturity of dendritic cells and the presentation of tumor antigens towards T cells [84, 85]. Besides, the activation of cGAS/STING is also associated with PD-L1 expression [86]. However, the activation of “Neuroactive ligand receptor interaction” is associated with the inhibition of “Cytosolic DNA sensing pathway”, and could lead to the reduction of CD8 + T cell infiltration in COAD [87]. The recognition of DNA could also be indirectly accomplished by RNA sensor RIG-I through “RIG I like receptor signaling pathway” [88]. The “RIG I like receptor signaling pathway” could enhance the function of Vγ9Vδ2 T cells [89], and induce apoptosis, pyroptosis, and autophagy of tumor cells [90,91,92]. The above signaling pathways had close correlation with immunity, and might be interacted with TSPAN7’s role of anti-tumor immunity across various types of tumors. In spite of this, there have not been previous studies directly illustrating the correlations of TSPAN7 with these signaling pathways. Further studies should be focused on revealing the precise molecular mechanisms of TSPAN7’s role in pan-cancer.
Limitations of the study
In spite of this, there were still some limitations in our study. Firstly, all 370 patients with kidney neoplasm were from Xinhua Hospital Affiliated to Shanghai Jiao Tong University School of Medicine. The findings of our study were not validated by patients from other medical centers. Besides, the pan-cancer data of our study was from public databases like TCGA, cBioPortal, TIMER2.0, TIDE, etc. The majority of these samples derived from patients in Western countries, which might lead to the racial deviance of our study. Last but not least, our study only preliminarily identified TSPAN7 as a promising biomarker in pan-cancer, and discovered its relationships with tumor purity, tumor genomics, tumor immunology, and drug sensitivity. As studies concerned TSPAN7 in tumors were still in the initial stages, we did not deeply investigate the molecular mechanisms of it, and more studies need to be conducted in the future to unveil the precise regulatory role of TSPAN7 in tumor progression.
Conclusion
In summary, we comprehensively studied the unique role of TSPAN7 in tumor progression across various types of tumors based on a large retrospective cohort of kidney neoplasm and subsequently online databases in pan-cancer level. Our study identified TSPAN7 as a promising biomarker of various tumors, and illustrated its close relationships with tumor purity, tumor genomics, tumor immunology, and drug sensitivity. Last but not least, we preliminarily explored the enriched signaling pathways of TSPAN7 in different types of tumors. In the future, more efforts need to be made to unveil the precise molecular mechanism of TSPAN7’s role in tumor progression.
Data availability
The datasets generated and/or analyzed during the study are available in TCGA database (https://tcga-data.nci.nih.gov/tcga/), DIANA-microT database (https://dianalab.e-ce.uth.gr/microt_webserver/#/), miRWalk database (http://mirwalk.umm.uni-heidelberg.de/), miRDB database (https://mirdb.org/), miRcode database (http://www.mircode.org/), ENCORI database (https://rnasysu.com/encori/), STRING database (https://cn.string-db.org/), cBioPortal database (https://www.cbioportal.org/), TIMER2.0 (http://timer.cistrome.org/) database, TIDE database (http://tide.dfci.harvard.edu/), GDSC (https://www.cancerrxgene.org/), CTRP (https://pubmed.ncbi.nlm.nih.gov/23993102/), CellMiner database (https://discover.nci.nih.gov/cellminer/home.do) and KEGG database (https://www.genome.jp/kegg/).
Abbreviations
- TSPAN:
-
tetraspanin
- EMT:
-
epithelial-mesenchymal transition
- TCGA:
-
The Cancer Genome Atlas
- ceRNA:
-
competing endogenouse RNA
- PPI:
-
protein-protein interaction
- IHC:
-
immunohistochemical
- SNV:
-
single nucleotide variation
- CNV:
-
copy number variation
- miRNA:
-
microRNA
- ENCORI:
-
Encyclopedia of RNA Interactomes
- lncRNA:
-
long noncoding RNA
- MSI:
-
microsatellite instability
- TIMER:
-
Tumor Immune Estimation Resource
- CTL:
-
cytotoxic T-lymphocyte
- TIDE:
-
Tumor Immune Dysfunction and Exclusion
- GDSC:
-
Genomics of Drug Sensitivity in Cancer
- CTRP:
-
The Cancer Therapeutics Response Portal
- KEGG:
-
Kyoto Encyclopedia of Genes and Genomes
- EDTA:
-
Ethylene Diamine Tetraacetic Acid
- HRP:
-
Horseradish Peroxidase
- DAB:
-
Diaminobenzidine
- OS:
-
overall survival
- PFS:
-
progression free survival
- K-M:
-
Kaplan-Meier
- ROC:
-
Receiver Operating Characteristic
- DFS:
-
disease free survival
- DSS:
-
disease specific survival
- ESTIMATE:
-
Estimation of STromal and Immune cells in MAlignant Tumors using Expression data
- TME:
-
tumor microenvironment
- TMB:
-
tumor mutation burden
- GSCA:
-
gene set cancer analysis
- WES:
-
Whole Exome Sequencing
- IC50:
-
half maximal inhibitory concentration
- GSEA:
-
gene set enrichment analysis
- FDR:
-
false discovery rate
- HR:
-
hazard ratio
- AUC:
-
area under the curve
- APC:
-
antigen presenting cell
- cGAS:
-
cyclic GMP-AMP synthase
- IFN:
-
interferon
References
Luan M, et al. The peptide mimicking small extracellular loop domain of CD82 inhibits tumor cell migration, adhesion and induces apoptosis by inhibiting integrin mediated signaling. Biochem Biophys Res Commun. 2018;503(4):2206–11.
Charrin S, et al. Lateral organization of membrane proteins: tetraspanins spin their web. Biochem J. 2009;420(2):133–54.
Hemler ME. Tetraspanin functions and associated microdomains. Nat Rev Mol Cell Biol. 2005;6(10):801–11.
Hemler ME. Tetraspanin proteins promote multiple cancer stages. Nat Rev Cancer. 2014;14(1):49–60.
Huang R, et al. The role of tetraspanins pan-cancer. iScience. 2022;25(8):104777.
Zhu R, et al. TSPAN8 promotes cancer cell stemness via activation of sonic hedgehog signaling. Nat Commun. 2019;10(1):2863.
Li Y, et al. TET2-mediated mRNA demethylation regulates leukemia stem cell homing and self-renewal. Cell Stem Cell. 2023;30(8):1072–e109010.
Garcia-Mayea Y, et al. TSPAN1, a novel tetraspanin member highly involved in carcinogenesis and chemoresistance. Biochim Biophys Acta Rev Cancer. 2022;1877(1):188674.
Hosokawa Y, et al. Molecular cloning of a cDNA encoding mouse A15, a member of the transmembrane 4 superfamily, and its preferential expression in brain neurons. Neurosci Res. 1999;35(4):281–90.
Piluso G, et al. Assessment of de novo copy-number variations in Italian patients with schizophrenia: detection of putative mutations involving regulatory enhancer elements. World J Biol Psychiatry. 2019;20(2):126–36.
Gulati S, et al. Systematic evaluation of the prognostic impact and intratumour heterogeneity of clear cell renal cell carcinoma biomarkers. Eur Urol. 2014;66(5):936–48.
Shao S, et al. Tetraspanin 7 promotes osteosarcoma cell invasion and metastasis by inducing EMT and activating the FAK-Src-Ras-ERK1/2 signaling pathway. Cancer Cell Int. 2022;22(1):183.
Chen L, et al. Functional characterization of TSPAN7 as a novel indicator for immunotherapy in glioma. Front Immunol. 2023;14:1105489.
Wang M, et al. N6AMT1 is a novel potential diagnostic, prognostic and immunotherapy response biomarker in pan-cancer. Aging. 2023;15(13):6526–44.
Ye Y, et al. Role of ARRB1 in prognosis and immunotherapy: a Pan-cancer analysis. Front Mol Biosci. 2022;9:1001225.
Tastsoglou S, et al. DIANA-microT 2023: including predicted targets of virally encoded miRNAs. Nucleic Acids Res. 2023;51(W1):W148–53.
Sticht C, et al. miRWalk: an online resource for prediction of microRNA binding sites. PLoS ONE. 2018;13(10):e0206239.
Chen Y, Wang X. miRDB: an online database for prediction of functional microRNA targets. Nucleic Acids Res. 2020;48(D1):D127–31.
Jeggari A, Marks DS, Larsson E. miRcode: a map of putative microRNA target sites in the long non-coding transcriptome. Bioinformatics. 2012;28(15):2062–3.
Li JH, et al. starBase v2.0: decoding miRNA-ceRNA, miRNA-ncRNA and protein-RNA interaction networks from large-scale CLIP-Seq data. Nucleic Acids Res. 2014;42(Database issue):D92–7.
Szklarczyk D, et al. The STRING database in 2023: protein-protein association networks and functional enrichment analyses for any sequenced genome of interest. Nucleic Acids Res. 2023;51(D1):D638–46.
Bonneville R et al. Landscape of Microsatellite Instability Across 39 Cancer Types JCO Precis Oncol, 2017. 2017.
Gao JJ et al. Integrative Analysis of Complex Cancer Genomics and Clinical profiles using the cBioPortal. Sci Signal, 2013. 6(269).
Li T, et al. TIMER2.0 for analysis of tumor-infiltrating immune cells. Nucleic Acids Res. 2020;48(W1):W509–14.
Jiang P, et al. Signatures of T cell dysfunction and exclusion predict cancer immunotherapy response. Nat Med. 2018;24(10):1550–8.
Yang W, et al. Genomics of Drug Sensitivity in Cancer (GDSC): a resource for therapeutic biomarker discovery in cancer cells. Nucleic Acids Res. 2012;41(D1):D955–61.
Rees MG, et al. Correlating chemical sensitivity and basal gene expression reveals mechanism of action. Nat Chem Biol. 2016;12(2):109–16.
Seashore-Ludlow B, et al. Harnessing connectivity in a large-scale small-molecule sensitivity dataset. Cancer Discov. 2015;5(11):1210–23.
Basu A, et al. An interactive resource to identify cancer genetic and lineage dependencies targeted by small molecules. Cell. 2013;154(5):1151–61.
Shankavaram UT, et al. CellMiner: a relational database and query tool for the NCI-60 cancer cell lines. BMC Genomics. 2009;10(1):277.
Reinhold WC, et al. CellMiner: a web-based suite of genomic and pharmacologic tools to explore transcript and drug patterns in the NCI-60 Cell Line Set. Cancer Res. 2012;72(14):3499–511.
Kanehisa M, Goto S. KEGG: kyoto encyclopedia of genes and genomes. Nucleic Acids Res. 2000;28(1):27–30.
Xiao Y, Yu D. Tumor microenvironment as a therapeutic target in cancer. Pharmacol Ther. 2021;221:107753.
Elhanani O, Ben-Uri R, Keren L. Spatial profiling technologies illuminate the tumor microenvironment. Cancer Cell. 2023;41(3):404–20.
Yoshihara K, et al. Inferring tumour purity and stromal and immune cell admixture from expression data. Nat Commun. 2013;4:2612.
Mayakonda A, et al. Maftools: efficient and comprehensive analysis of somatic variants in cancer. Genome Res. 2018;28(11):1747–56.
Campbell PJ, et al. Pan-cancer analysis of whole genomes. Nature. 2020;578(7793):82–.
Zehir A, et al. Mutational landscape of metastatic cancer revealed from prospective clinical sequencing of 10,000 patients. Nat Med. 2017;23(6):703–13.
Robinson DR, et al. Integrative clinical genomics of metastatic cancer. Nature. 2017;548(7667):297–303.
Miao D, et al. Genomic correlates of response to immune checkpoint blockade in microsatellite-stable solid tumors. Nat Genet. 2018;50(9):1271–81.
Hyman DM, et al. HER kinase inhibition in patients with HER2- and HER3-mutant cancers. Nature. 2018;554(7691):189–94.
Samstein RM, et al. Tumor mutational load predicts survival after immunotherapy across multiple cancer types. Nat Genet. 2019;51(2):202–6.
Rosen EY, et al. TRK fusions are enriched in cancers with uncommon histologies and the absence of canonical driver mutations. Clin Cancer Res. 2020;26(7):1624–32.
Bolton KL, et al. Cancer therapy shapes the fitness landscape of clonal hematopoiesis. Nat Genet. 2020;52(11):1219–26.
Wu L, et al. Landscape of somatic alterations in large-scale solid tumors from an Asian population. Nat Commun. 2022;13(1):4264.
Nguyen B, et al. Genomic characterization of metastatic patterns from prospective clinical sequencing of 25,000 patients. Cell. 2022;185(3):563–e57511.
Barrett T, et al. NCBI GEO: archive for functional genomics data sets–update. Nucleic Acids Res. 2013;41(Database issue):D991–5.
Curtis C, et al. The genomic and transcriptomic architecture of 2,000 breast tumours reveals novel subgroups. Nature. 2012;486(7403):346–52.
Weinstein JN, et al. The Cancer Genome Atlas Pan-cancer analysis project. Nat Genet. 2013;45(10):1113–20.
Yu G, et al. clusterProfiler: an R package for comparing biological themes among gene clusters. Omics. 2012;16(5):284–7.
Sawada J, et al. Molecular signature of Tumor-Associated High Endothelial venules that can predict breast Cancer survival. Cancer Immunol Res. 2022;10(4):468–81.
Yang J, et al. Identification and validation of a novel cuproptosis-related stemness signature to predict prognosis and immune landscape in lung adenocarcinoma by integrating single-cell and bulk RNA-sequencing. Front Immunol. 2023;14:1174762.
Wuttig D, et al. CD31, EDNRB and TSPAN7 are promising prognostic markers in clear-cell renal cell carcinoma revealed by genome-wide expression analyses of primary tumors and metastases. Int J Cancer. 2012;131(5):E693–704.
Qi Y, et al. Expression and function of transmembrane 4 superfamily proteins in digestive system cancers. Cancer Cell Int. 2020;20:314.
Cheong CM, et al. Tetraspanin 7 (TSPAN7) expression is upregulated in multiple myeloma patients and inhibits myeloma tumour development in vivo. Exp Cell Res. 2015;332(1):24–38.
Luo L, et al. A new 7-gene survival score assay for pancreatic cancer patient prognosis prediction. Am J Cancer Res. 2021;11(2):495–512.
Gu HY, et al. Risk score based on expression of five novel genes predicts survival in soft tissue sarcoma. Aging. 2020;12(4):3807–27.
Yu X, et al. TSPAN7 exerts Anti-tumor effects in bladder Cancer through the PTEN/PI3K/AKT pathway. Front Oncol. 2020;10:613869.
Oh S et al. Genomic and transcriptomic characterization of gastric Cancer with bone metastasis. Cancer Res Treat, 2023.
Ashkenazi A, et al. From basic apoptosis discoveries to advanced selective BCL-2 family inhibitors. Nat Rev Drug Discov. 2017;16(4):273–84.
Gandhi L, et al. Phase I study of Navitoclax (ABT-263), a novel Bcl-2 family inhibitor, in patients with small-cell lung cancer and other solid tumors. J Clin Oncol. 2011;29(7):909–16.
Siegel D, et al. Vorinostat in solid and hematologic malignancies. J Hematol Oncol. 2009;2:31.
Mei M, et al. Pembrolizumab plus Vorinostat induces responses in patients with Hodgkin lymphoma refractory to prior PD-1 blockade. Blood. 2023;142(16):1359–70.
Janku F, et al. Safety and Efficacy of Vorinostat Plus Sirolimus or Everolimus in patients with relapsed refractory Hodgkin Lymphoma. Clin Cancer Res. 2020;26(21):5579–87.
Olivieri A, Manzione L. Dasatinib: a new step in molecular target therapy. Ann Oncol. 2007;18(Suppl 6):vi42–6.
Fornier MN, et al. A phase I study of dasatinib and weekly paclitaxel for metastatic breast cancer. Ann Oncol. 2011;22(12):2575–81.
Miller AA, et al. A phase II study of dasatinib in patients with chemosensitive relapsed small cell lung cancer (Cancer and Leukemia Group B 30602). J Thorac Oncol. 2010;5(3):380–4.
Lue HW, et al. Cabozantinib and Dasatinib synergize to induce tumor regression in non-clear cell renal cell carcinoma. Cell Rep Med. 2021;2(5):100267.
Fang Z, et al. Mir-140-5p suppresses the proliferation, migration and invasion of gastric cancer by regulating YES1. Mol Cancer. 2017;16(1):139.
Lu J, et al. FTO-mediated LINC01134 stabilization to promote chemoresistance through miR-140-3p/WNT5A/WNT pathway in PDAC. Cell Death Dis. 2023;14(11):713.
Sun H, et al. Dysregulation of KCNQ1OT1 promotes cholangiocarcinoma progression via miR-140-5p/SOX4 axis. Arch Biochem Biophys. 2018;658:7–15.
Bassani S, et al. The X-linked intellectual disability protein TSPAN7 regulates excitatory synapse development and AMPAR trafficking. Neuron. 2012;73(6):1143–58.
Cassetta L, Pollard JW. Targeting macrophages: therapeutic approaches in cancer. Nat Rev Drug Discov. 2018;17(12):887–904.
Bevan MJ. Helping the CD8(+) T-cell response. Nat Rev Immunol. 2004;4(8):595–602.
Castellino F, Germain RN. Cooperation between CD4 + and CD8 + T cells: when, where, and how. Annu Rev Immunol. 2006;24:519–40.
Laidlaw BJ, Craft JE, Kaech SM. The multifaceted role of CD4(+) T cells in CD8(+) T cell memory. Nat Rev Immunol. 2016;16(2):102–11.
Ahrends T, et al. CD4(+) T cell help confers a cytotoxic T cell Effector Program including coinhibitory receptor downregulation and increased tissue invasiveness. Immunity. 2017;47(5):848–e8615.
St Paul M, Ohashi PS. The roles of CD8(+) T cell subsets in Antitumor Immunity. Trends Cell Biol. 2020;30(9):695–704.
van der Leun AM, Thommen DS, Schumacher TN. CD8(+) T cell states in human cancer: insights from single-cell analysis. Nat Rev Cancer. 2020;20(4):218–32.
Le DT, et al. PD-1 blockade in tumors with Mismatch-Repair Deficiency. N Engl J Med. 2015;372(26):2509–20.
Le DT, et al. Mismatch repair deficiency predicts response of solid tumors to PD-1 blockade. Science. 2017;357(6349):409–13.
Dudley JC, et al. Microsatellite instability as a biomarker for PD-1 blockade. Clin Cancer Res. 2016;22(4):813–20.
Yu L, Liu P. Cytosolic DNA sensing by cGAS: regulation, function, and human diseases. Signal Transduct Target Therapy. 2021;6(1):170.
Hopfner KP, Hornung V. Molecular mechanisms and cellular functions of cGAS-STING signalling. Nat Rev Mol Cell Biol. 2020;21(9):501–21.
Sprooten J, Agostinis P, Garg AD. Type I interferons and dendritic cells in cancer immunotherapy. Int Rev Cell Mol Biol. 2019;348:217–62.
Grabosch S, et al. Cisplatin-induced immune modulation in ovarian cancer mouse models with distinct inflammation profiles. Oncogene. 2019;38(13):2380–93.
Yang Y, et al. Inhibition of neuroactive ligand-receptor interaction pathway can enhance immunotherapy response in colon cancer: an in silico study. Expert Rev Anticancer Ther. 2023;23(11):1205–15.
Onomoto K, Onoguchi K, Yoneyama M. Regulation of RIG-I-like receptor-mediated signaling: interaction between host and viral factors. Cell Mol Immunol. 2021;18(3):539–55.
Li J, et al. Gastric cancer derived exosomal THBS1 enhanced Vγ9Vδ2 T-cell function through activating RIG-I-like receptor signaling pathway in a N6-methyladenosine methylation dependent manner. Cancer Lett. 2023;576:216410.
Wu Y, et al. The anticancer functions of RIG-I-like receptors, RIG-I and MDA5, and their applications in cancer therapy. Transl Res. 2017;190:51–60.
Elion DL, et al. Therapeutically active RIG-I agonist induces immunogenic Tumor Cell killing in breast cancers. Cancer Res. 2018;78(21):6183–95.
Hou P, et al. A novel selective autophagy receptor, CCDC50, delivers K63 polyubiquitination-activated RIG-I/MDA5 for degradation during viral infection. Cell Res. 2021;31(1):62–79.
Acknowledgements
We thank TCGA database, DIANA-microT database, miRWalk database, miRDB database, miRcode database, ENCORI database, STRING database, cBioportal database, TIMER 2.0 database, TIDE database, GDSC database, CTRP database, and CellMiner database for allowing us to use their data.
Funding
This work was sponsored by the National Natural Science Foundation of China (No. 82330094, 82173265, 82072806); Leading health talents of Shanghai Municipal Health Commission (2022LJ002); Shanghai Rising-Star Program (23QC1401400); Shanghai Rising-Star Program (Sailing Special Program) (23YF1458400); the Natural Science Foundation of Shanghai (23ZR1441300); 2022 medical health key project of Science and Technology Commission of Baoshan District (2023-E-37); Baoshan District Health Commission Key Subject Construction Project (BSZK-2023-A06); Hospital Funded Clinical Research, Xinhua Hospital Affiliated to Shanghai Jiao Tong University School of Medicine (21XHDB06); Jiaxing Science and Technology Plan Project (No. 2022-2). The funders had no role in study design, data collection and analysis, decision to publish, or preparation of the manuscript.
Author information
Authors and Affiliations
Contributions
X.P. and X.C. conceptualized the project. K.D. and X.P. collected the data. B.L., Y.L., Y.Y., and R.H. conducted data analysis. B.L., X.Z., D.L., Z.Z., H.Z., X.Y., and W.F. wrote the original draft of the manuscript. Y.L., Y.Y., J.C., X.P., and X.C. reviewed the manuscript. B.L., Y.L., Y.Y., D.Z., X.X., R.H., J.C., J.C., X.P., and X.C. revised the manuscript. R.H., J.C., J.C., X.P., and X.C. acquired the funding.
Corresponding authors
Ethics declarations
Ethics approval and consent to participate
Our study was approved by the Ethics Committee of Xinhua Hospital Affiliated to Shanghai Jiao Tong University School of Medicine (XHEC-C-2021-145-1). All authors confirmed that the whole study was carried out in accordance with relevant guidelines.
Competing interests
The authors declare no competing interests.
Additional information
Publisher’s Note
Springer Nature remains neutral with regard to jurisdictional claims in published maps and institutional affiliations.
Electronic supplementary material
Below is the link to the electronic supplementary material.
Rights and permissions
Open Access This article is licensed under a Creative Commons Attribution-NonCommercial-NoDerivatives 4.0 International License, which permits any non-commercial use, sharing, distribution and reproduction in any medium or format, as long as you give appropriate credit to the original author(s) and the source, provide a link to the Creative Commons licence, and indicate if you modified the licensed material. You do not have permission under this licence to share adapted material derived from this article or parts of it. The images or other third party material in this article are included in the article’s Creative Commons licence, unless indicated otherwise in a credit line to the material. If material is not included in the article’s Creative Commons licence and your intended use is not permitted by statutory regulation or exceeds the permitted use, you will need to obtain permission directly from the copyright holder. To view a copy of this licence, visit http://creativecommons.org/licenses/by-nc-nd/4.0/.
About this article
Cite this article
Lu, B., Liu, Y., Yao, Y. et al. Unveiling the unique role of TSPAN7 across tumors: a pan-cancer study incorporating retrospective clinical research and bioinformatic analysis. Biol Direct 19, 72 (2024). https://doi.org/10.1186/s13062-024-00516-8
Received:
Accepted:
Published:
DOI: https://doi.org/10.1186/s13062-024-00516-8