Abstract
Background
This multicenter observational study aimed to determine whether dyslipidemia or obesity contributes more significantly to unfavorable clinical outcomes in patients experiencing a first-ever ischemic stroke (IS).
Methods
The study employed a machine learning predictive model to investigate associations among body mass index (BMI), body fat percentage (BFP), high-density lipoprotein (HDL), triglycerides (TG), and total cholesterol (TC) with adverse outcomes in IS patients. Extensive real-world clinical data was utilized, and risk factors significantly linked to adverse outcomes were identified through multivariate analysis, propensity score matching (PSM), and regression discontinuity design (RDD) techniques. Furthermore, these findings were validated via a nationwide multicenter prospective cohort study.
Results
In the derived cohort, a total of 45,162 patients diagnosed with IS were assessed, with 522 experiencing adverse outcomes. A multifactorial analysis incorporating PSM and RDD methods identified TG (adjusted odds ratio (OR) = 1.110; 95% confidence interval (CI): 1.041–1.183; P < 0.01) and TC (adjusted OR = 1.139; 95%CI: 1.039–1.248; P < 0.01) as risk factors. However, BMI, BFP, and HDL showed no significant effect. In the validation cohort, 1410 controls and 941 patients were enrolled, confirming that lipid levels are more strongly correlated with the prognosis of IS patients compared to obesity (TC, OR = 1.369; 95%CI: 1.069–1.754; P < 0.05; TG, OR = 1.332; 95%CI: 1.097–1.618; P < 0.01).
Conclusion
This study suggests that dyslipidemia has a more substantial impact on the prognosis of IS patients compared to obesity. This highlights the importance of prioritizing dyslipidemia management in the treatment and prevention of adverse outcomes in IS patients.
Graphical abstract

Similar content being viewed by others
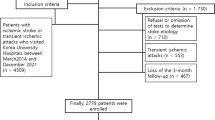
Background
Ischemic stroke (IS) remains a significant challenge in intensive care and ranks as the second leading cause of global mortality and morbidity [1, 2]. Historically, obesity has been linked to an unfavourable prognosis in patients with ischemic stroke, encompassing outcomes such as stroke-related mortality and recurrence. However, recent studies have highlighted a paradoxical observation of improved prognosis in obese patients compared to underweight and normal-weight individuals, known as the “obesity paradox” [3, 4]. This phenomenon suggests that obesity may not be unequivocally associated with negative health outcomes in the ischemic stroke population. Additionally, individuals with obesity may exhibit localized fat accumulation, such as excess subcutaneous and visceral fat, as well as elevated blood lipid levels, including various dyslipidaemias. These factors may be present in patients individually or concurrently, prompting the need to discern the relationship between prognosis in IS patients and obesity, and abnormal blood lipid levels.
Current studies have endeavoured to untangle the individual associations of lipid levels and obesity with IS risk, yet these clinical investigations have yielded conflicting results [5,6,7]. For instance, Ovbiagele et al. [6] conducted a 2.5-year follow-up study involving 20,332 patients with recent IS and found that obesity was not significantly related to the risk of recurrent stroke, contrasting a cohort study by Andersen et al. [7], which observed a significantly lower risk of readmission for recurrent stroke in obese patients. Given the rising prevalence of ischemic stroke (IS) and the unique genetic and lifestyle attributes of its demographic, it is essential to investigate the individual contributions of lipid levels and body mass index in forecasting prognosis following an ischemic stroke [8, 9].
Traditionally, obesity has been primarily measured using the body mass index (BMI), a recognized indicator of obesity that has also been linked to the development of cardiovascular risk factors and stroke incidence [10, 11]. From the BMI metric, the body fat percentage (BFP) is derived and employed in calculating a patient’s body fat percentage [12]. For lipid assessment, total cholesterol (TC) and triglycerides (TG) act as the primary risk indicators. Lipid levels, including cholesterol and triglycerides, have long been associated with atherosclerosis and ischemic stroke pathogenesis [13,14,15]. This study aims to compare the indicators and gather additional covariates to assess whether obesity or elevated lipids are the predominant factors contributing to poor prognosis in IS patients.
This study proposed the hypothesis that dyslipidaemia, rather than obesity, may be a significant risk factor for adverse prognosis in individuals who have suffered a stroke. Data from three medical centers overseeing 45,162 stroke patients were collated to scrutinize five obesity indices – specifically BMI, BFP, TC, TG, and High-Density Lipoprotein (HDL), and their preliminary associations with poor prognosis. Furthermore, propensity score matching (PSM) and regression discontinuity design (RDD) were employed to ascertain the connections between different fat indicators and poor prognosis. Finally, the associations between fat indicators and adverse outcomes were further substantiated by corroborating the results with data from a previously conducted national multicentre prospective cohort study [16].
Methods
Study designs
The study involved two separates’ cohorts: the derivation cohort and the validation cohort. The primary objective was to utilize the derivation cohort to initially investigate the correlation between various fat metrics and adverse outcomes. Subsequently, the aim was to validate these findings in a separate validation cohort and evaluate the influence of fat metrics on clinical outcomes at various time intervals. The details of the study’s design are depicted in Fig. 1.
Study population and study endpoints
The study population included patients who had experienced their first-ever IS, diagnosed according to the International Classification of Diseases, Ninth Revision [17]. All subjects were evaluated using rigorous neuroimaging techniques, such as head computed tomography and/or magnetic resonance imaging, and the images were reviewed by two or more experienced neuroradiologists. Adverse outcomes were defined as composite endpoint events, including mortality and recurrences. The primary endpoint was adverse outcomes, and the secondary endpoint was recurrence-free survival (RFS).
Inclusion/exclusion criteria and study variables for the derivation cohort
Clinical data for the derivation cohort were obtained from three branch areas of the Tongji Hospital. These data were extracted from Tongji Hospital’s electronic medical records, the largest healthcare facility in the Hubei region, China. Their extensive system contains over 60 million medical records dating back to 1980. Information on patients admitted for their first-ever ischemic stroke from January 2012 to January 2022 was collected retrospectively. The study excluded individuals under 18 years of age and pregnant women. The variables collected included the primary test parameters of patients, covering age, gender, smoking and alcohol consumption status, arterial blood pressure, fat-related indicators, and comorbidities.
Obesity, traditionally defined as abnormal accumulation of adipose tissue with potential health consequences [18, 19], has often been characterized using BMI in clinical studies [1, 6, 7, 20,21,22,23,24]. However, BMI alone is insufficient due to its inability to differentiate fat from muscle mass and consider factors like age, gender, and ethnicity [12, 25]. This study expanded fat-related indicators to include BFP, HDL, TG, and TC, offering a more comprehensive evaluation of the impact of fat on patients.
The BMI categories were determined according to Chinese standards as follows: underweight: BMI < 18.5 kg/m2; normal range: 18.5 ≤ BMI < 24 kg/m2; overweight: BMI ≥ 24 kg/m2. The fat metrics considered for this study comprised BMI, BFP, HDL, TG, and TC. The BFP is determined using the Formula 1 [12, 26].
Validation cohort
A national multicentre prospective cohort study from 5 centres in China was used as the validation cohort. This validation group consisted of 1210 controls and 941 patients with an initial IS, monitored for 5 years. The study excluded patients with concomitant chronic kidney disease or chronic respiratory disease. Further details are disclosed in previous studies [16].
Clinical correlation analysis
The correlation between fat-related markers and adverse outcomes in IS was investigated using local weighted regression scatterplot smoothing (LOWESS) and trend tests. Nonlinear correlations were identified through restricted cubic spline regression (RCS). The connections between covariates and outcomes were assessed utilizing a generalized additive model (GAM) fitted by maximizing the penalized likelihood with a binomial family and logit link functions [27]. In the univariate analysis, each potential risk factor was individually assessed for its association with the outcome variable. Factors demonstrating a statistically significant association (P < 0.1) were further evaluated in the multivariate analysis.
Machine learning and quasi experimental design
Five machine learning models—GaussianNB (GNB), LogisticRegression (LR), DecisionTreeClassifier (DTC), RandomForestClassifier (RF), and GradientBoostingClassifier (GBC)—were employed to develop a predictive model for forecasting adverse outcomes in IS based on fat-related metrics. The assessment was based on the area under the receiver operating characteristic (ROC) curve and model performance to determine the most effective risk prediction model. Subsequently, the SHapley Additive exPlanations (SHAP) value theory was leveraged to delineate the impact of different obesity indicators on adverse outcomes in IS patients [28].
Associations between obesity indicators and prognosis were examined using univariate and multivariate logistic regression, as well as PSM and RDD [20, 29]. RDD is a quasi-experimental design providing insights into the practical effects of treatments and strategies in real-world settings [20, 30,31,32]. Covariate selection and combination for PSM were performed using the “psestimate” command [33]. The primary objective of the program was to select a linear or quadratic function of covariates for inclusion in the estimation function of the propensity score.
Statistical analysis
The analysis utilized R software (version 4.3.0), Stata 17.0 software (StataCorp LP, College Station, TX), and employed machine learning through Anaconda3 software. For numerical variables with a sample size ≤5000, either the mean ± standard deviation or the median (upper and lower quartiles) were computed, selected based on the data distribution’s normality. Meanwhile, the mean ± standard deviation was calculated for numerical variables exceeding a sample size of 5000. Categorical variables were presented as frequencies and percentages. Comparison of continuous variables between two groups was executed using t-tests for normally distributed data with homogeneity of variance, and for three-group comparisons under similar conditions, one-way analysis of variance (ANOVA) was employed. In cases where normal distribution was met, but not the homogeneity of variance test, comparisons were made using the Welch t-test and Welch one-way ANOVA for two- and three-group analyses, respectively. For non-normally distributed data, two- and three-group comparisons were performed using the Wilcoxon test and Kruskal-Wallis test, respectively. The chi-square test was applied to assess the comparison of categorical variables between groups, with statistical significance set at two-tailed P < 0.05. Furthermore, the odds ratio (OR) and the associated 95% confidence interval (CI) values were calculated. Additionally, the Kaplan-Meier (KM) curves were used to analyse survival data, providing visualization and comparison of the survival experience across different groups over time.
Ethical considerations
This study received approval from the Institutional Review Board of the Tongji Hospital, Tongji Medical College, Huazhong University of Science and Technology (Wuhan, China; Approval No. TJ-I TJ-IRB20230830) and adhered to the principles of the Declaration of Helsinki. Written informed consent was waived due to the observational nature of the study.
Results
Demographic and clinical characteristics of the study population
The study population’s demographic and clinical characteristics are presented in Table 1, representing the derivation cohort of 45,162 individuals diagnosed with IS, with an average age of 60.4 ± 14.4 years. Among them, males constituted the majority at 61.31%, with 30.72% being smokers and 20.03% alcohol consumers. Adverse outcomes were observed in 1.16% of this cohort. Patients were categorized into two groups based on the occurrence of adverse outcomes during hospitalization. Significant differences in HDL, BFP, TC, and TG were observed between the two groups concerning obesity indicators (all P < 0.01), while no significant disparity was found in BMI (P > 0.05). Baseline characteristics of the validation cohort are presented in Supplementary Table 1.
Non-linear association of fat-related indicators with adverse outcomes
As illustrated in Fig. 2a, the LOWESS regression suggests a non-linear relationship between fat-related indicators, excluding BMI, potentially demonstrating a non-linear association with adverse outcomes (all P for trend< 0.001). The RCS regression further validated the non-linear relationship between fat-related indicators, excluding BMI, and adverse outcomes (P for overall< 0.001, Fig. 2b). The ROC curves revealed a positive correlation between the fat indicators and adverse outcomes (Area Under Curve (AUC) > 0.5, Fig. 2c), except for BMI, which exhibited a negative correlation with adverse outcomes (AUC = 0.480, Supplementary Table 2).
Exploratory analysis of the correlation between fat-related indicators and clinical outcomes. a The LOWESS curves depict the associations between fat-related indicators and clinical outcomes, while the box diagram reveals variations in clinical outcomes observed across quintiles of the indicators. b Curves from the restricted cubic spline regression depicting various fat-related indicators and their impact on clinical outcomes. c ROC curves for fat-related metrics in predicting clinical outcomes and ROC curves for machine learning-based methods in prognosticating clinical outcomes. d Ranking the importance of various obesity indicators in Gradient Boosting Classifier machine learning models. e Summary plot of SHAP values for the predictive features in the Gradient Boosting Classifier model. In the SHAP summary plot, each row represents a feature, and each point represents a sample. The color denotes the magnitude of the feature value, with red indicating high values and blue indicating low values. Positive SHAP values indicate a positive impact of the feature on the model, while negative values indicate a negative impact. Abbreviations: SHAP, Shapley’s additive interpretation
Supplementary Fig. 1 shows the process of parameter tuning for machine learning. The GBC model achieved optimal predictive performance in the machine learning model based on fat-related clinical indicators (AUC = 0.772, 95%CI (0.751–0.793), Supplementary Table 3). The SHAP suggested that BFP made the highest contribution to adverse outcomes, while BMI contributed the least (Fig. 2d). Higher BMI and HDL were found to be protective against adverse outcomes, while elevated levels of triglycerides, total cholesterol, and BFP were identified as risk factors for adverse outcomes in IS patients (Fig. 2e).
TG and TC was an independent risk factor against adverse outcome
In univariate analyses, all fat-related indicators, except BMI, were identified as risk factors (all OR > 1, and P < 0.001, Table 2). The subsequent multivariate analysis utilized GAM regression, enabling the simultaneous assessment of multiple potential risk factors while adjusting for confounding variables. The multivariate analysis revealed that both TG (adjusted OR = 1.110, 95% CI: (1.041–1.183), P = 0.001) and TC (adjusted OR = 1.139, 95% CI: (1.039–1.248), P = 0.005) were significantly associated with adverse outcomes as independent risk factors. Additionally, no multicollinearity was observed (Supplementary Table 4).
Following PSM (Supplementary Fig. 2), it was observed that the levels of TG (1.71 (1.17, 2.4) vs. 1.47 (1.02, 2.13), P < 0.001) and TC (4.60 (3.93, 5.59) vs. 4.51 (3.82, 5.30), P = 0.033) were significantly increased in patients experiencing adverse outcomes (Table 3).
The RDD revealed substantial associations with TC and TG, indicating that patients were at significantly higher risk of adverse outcomes upon reaching cut-off point (all P < 0.05, Fig. 3). Sensitivity tests, including Discontinuity estimate and Placebo Tests, were performed, and consistent results were obtained across different bandwidths (Supplementary Table 5, 6). Furthermore, covariates were found to be balanced on both sides of the cut-off point (Supplementary Table 7, 8), supporting the robustness of findings.
Analyzing the Relationship Between TC, TG, and Adverse Outcomes Using Regression Discontinuity Design. a, h Scatterplot Analysis: Total Cholesterol and Probability of Adverse Outcomes, Triglycerides and Probability of Adverse Outcomes. b, i Regression Discontinuity plot: At a triglycerides (TG) level of 1.14 mmol/L, patients exhibited a notable decrease in the risk of adverse outcomes. Similarly, at a total cholesterol (TC) level of 5.34 mmol/L, patients demonstrated a substantial reduction in the risk of adverse outcomes. The dots represent Odds Ratio (OR) values, while the shading represents confidence intervals. c-e, j-i Sensitivity analysis. f, m Continuity tests; (g, n) Placebo tests: The robustness of the final model results is assessed at various percentile values, including 1, 10, 25, 50, 75, 90 and 99%, respectively
Various fat-related indicators manifest distinct effects over time
A national multicentre prospective cohort study was conducted to validate the association of various fat-related markers with poor prognosis. The study included 1210 healthy individuals and 941 first-time IS patients. The demographic and clinical characteristics of the study population, stratified by BMI, are presented in Table 4. Importantly, patients with different weight statuses exhibited significant clinical outcomes at both 1 and 2 years, indicating that the protective effect of BMI varies over time, being most pronounced during the 1–2-year period. Furthermore, the correlation between obesity metrics in different population groups is depicted in Supplementary Fig. 3 and Supplementary Table 9.
The investigation involved assessing the association between various obesity indicators and clinical outcomes at different time intervals using GAM. Clinical outcomes were treated as a binomial variable, with the logit function used as the link (Fig. 4a). The findings indicate that TC and TG, both of which are indicators related to obesity, act as risk factors for adverse outcomes in individuals with IS within a one-year timeframe. Furthermore, the impact of both indicators on adverse outcomes diminishes gradually with the extension of the time frame. Additionally, obesity as defined by BMI did not exhibit significant protective effects compared to normal weight (all P > 0.05), while wasting emerged as a risk factor over a one to two-year time horizon (12-month: OR = 2.900, 95% CI: (1.108–7.592), P = 0.030; 18-month: OR = 3.089, 95% CI: (1.085–8.792), P = 0.035). Table 5 presents the model after adjustment for confounding variables, indicating that both TG and TC persist as risk factors 1 year following disease onset. Furthermore, the impact of total cholesterol endured for a longer duration compared to that of triglycerides. Finally, the Kaplan-Meier curve demonstrated that individuals with elevated levels of TC or TG experienced a worse prognosis within a one-year timeframe (Fig. 4b, Supplementary Fig. 4–5).
Discussion
This study suggests that dyslipidaemia (TG, TC) rather than obesity (BMI, BFP) acts as a significant factor contributing to the unfavourable prognosis of IS patients. Data from the derivation cohort, incorporating a comprehensive range of adiposity measures such as BMI, BFP, HDL, TG, and TC, revealed that TG and TC consistently emerged as predictors of negative clinical outcomes. Failing to support the obesity paradox, the study did not identify a significant protective effect associated with higher BMI values. These conclusions were further reinforced by utilizing robust techniques such as multivariate analysis, PSM and RDD. The results of a rigorous national prospective cohort study underscored that the prognostic impact of dyslipidaemia distinctly presents within the initial year following IS onset and weakens considerably beyond this period, indicating that TG and TC, as markers of dyslipidaemia, continue to represent risk factors for IS.
The novelty of the study lies in its examination of the interplay between lipids, obesity, and prognosis in ischemic stroke patients. Traditionally, obesity has been viewed as potentially impacting the prognosis of stroke patients, with conflicting perspectives on whether it acts as a risk factor for adverse outcomes or as a protective factor [34]. The conventional definition of obesity based on BMI was deemed flawed due to its inability to differentiate between muscle and fat mass, potentially misclassifying individuals. It is crucial to evaluate the distinct components of obesity to accurately assess its impact [12]. While international obesity criteria rely on BMI, this measurement fails to differentiate between fat and muscle proportions in body weight and does not elucidate lipid levels. Consequently, the analysis delved into the effects of distinct obesity components – BMI, BFP, and blood lipids – on the prognosis of IS patients.
Initially, this study identified elevated levels of TG and TC as significant risk factors influencing the prognosis of patients with IS. This conclusion aligns with existing research [13], stressing the correlation between dyslipidaemia and the heightened risk of IS relapse. Hyperlipidemia arises from excessive consumption of a high-cholesterol diet, leading to elevated blood lipid levels [35]. Lipid levels play a role in the development of carotid and intracranial atherosclerosis, additional risk factors for IS. TG and TC are significant lipid components present in the bloodstream. Elevated TG levels may contribute to atherosclerosis development through mechanisms such as excess free fatty acid provision, production of pro-inflammatory cytokines, fibrinogen, coagulation factors, and impaired fibrinolysis [36]. Cholesterol is crucial for cell membranes, brain and nerve cells, bile function, and aids in fat and fat-soluble vitamin absorption. Elevated total cholesterol levels also heighten the risk of atherosclerosis. This finding suggests that monitoring TG and TC levels during the acute and subacute phases of IS crucial, as the highest risk of adverse outcomes occurs within the first year of disease onset. The temporal effect partially accounts for the evolving impact of blood lipid on the prognosis of critically ill patients over time [37, 38].
Furthermore, the current study did not find a significant prognostic effect of obesity, either as a risk factor or a protective factor, in the initial occurrence of IS among the East Asian population. This discrepancy contradicts some previous studies that hint at a protective impact attributed to high BMI in the case of stroke patients [7, 24]. The variation possibly stems from the complex BMI-stroke outcome relationship [6], exacerbated by age and sex differences, implying that generic metrics like BMI are inadequate to illustrate nuanced risk profiles spanning different demographic groups [12, 39]. To delve deeper into this complexity, the study incorporated BFP, an advanced measure that considers BMI, age, and gender. The results confirmed that neither BFP nor BMI exhibited a significant correlation with adverse outcomes in IS cases, raising doubts about obesity’s presumed protective effects.35.
Study strengths and limitations
The strength of the study is employing a comprehensive and rigorous methodological framework, including retrospective and prospective cohort analyses bolstered by advanced statistical methods (such as PSM and RDD), enhanced the robustness of the study and potentially its applicability to a broader patient population. Additionally, the nationwide multicentre nature of the study allowed for a large and diverse patient population to be included, increasing the generalizability of the findings. By utilizing both retrospective and prospective data, the study provides a comprehensive and reliable assessment of the relationship between fat-related indicators and adverse outcomes in IS patients. A novel approach was employed, integrating a machine learning predictive model using SHAPE values to decipher the impactful contributions of various adiposity measures on IS outcomes. The results confirmed that dyslipidaemia metrics are primary predictors for adverse clinical events. The analysis revealed that body fat percentage, TG, and TC score emerged as the top three contributors to adverse clinical outcomes. These findings provide further evidence supporting the notion that fat-related indicators serve as significant risk factors for adverse outcomes in stroke patients. These methodological strengths contribute to the significance and reliability of the findings, providing valuable insights for clinical practice and future research in this area. The study recognizes its notable strengths and acknowledges inherent limitations, including potential selection bias and confounding variables typical of observational studies. Additionally, while associations between fat-related indices and IS outcomes were established, the study did not delve into the underlying biological mechanisms that could possibly explain these observations. Future research should seek to unveil the biological pathways through which lipid levels and other fat-related factors impact IS patient prognosis, thereby gaining a deeper comprehension of the underpinning pathophysiology and potential intervention targets.
Conclusions
In conclusion, this study emphasizes the importance of prioritizing lipid management over weight management in individuals with ischemic stroke, highlighting the critical role of dyslipidaemia, particularly elevated TG and TC levels, as significant risk factors for adverse outcomes in IS patients.
Availability of data and materials
The data and code used in this study could be obtained from the corresponding author upon reasonable request.
Abbreviations
- ANOVA:
-
one-way analysis of variance
- BFP:
-
Body fat percentage
- BMI:
-
Body Mass Index
- CHD:
-
Chronic cardiac disease
- CKD:
-
Chronic Kidney Disease
- CI:
-
Confidence interval
- CRD:
-
Chronic respiratory disease
- DBP:
-
Diastolic blood pressure
- DTC:
-
DecisionTreeClassifier
- GBC:
-
GradientBoostingClassifier
- GAM:
-
Generalized additive model
- GNB:
-
GaussianNB
- HDL:
-
High-Density Lipoprotein
- IS:
-
Ischemic stroke
- KM:
-
Kaplan-Meier
- LR:
-
LogisticRegression
- LOWESS:
-
Local weighted regression scatterplot smoothing
- PSM:
-
Propensity score matching
- RFS:
-
Recurrence-free survival
- RF:
-
RandomForestClassifier
- RCS:
-
Restricted cubic spline regression
- RDD:
-
Regression discontinuity designs
- ROC:
-
Receiver operating characteristic
- SBP:
-
Systolic blood pressure
- SHAP:
-
SHapley Additive exPlanations
- TC:
-
Total Cholesterol
- TG:
-
Triglyceride
References
Feigin VL, Stark BA, Johnson CO, Roth GA, Bisignano C, Abady GG, et al. Global, regional, and national burden of stroke and its risk factors, 1990-2019: a systematic analysis for the global burden of disease study 2019. Lancet Neurol. 2021;20:795–820.
Feigin VL, Brainin M, Norrving B, Martins S, Sacco RL, Hacke W, et al. World stroke organization (WSO): global stroke fact sheet 2022. Int J Stroke. 2022;17:18–29.
Rexrode K, Rundek T. Body mass index and stroke in UK women: "obesity paradox" revisited. Neurology. 2016;87:1432–3.
Liu Z, Sanossian N, Starkman S, Avila-Rinek G, Eckstein M, Sharma LK, et al. Adiposity and outcome after ischemic stroke: obesity paradox for mortality and obesity parabola for favorable functional outcomes. Stroke. 2021;52:144–51.
Chaudhary D, Khan A, Gupta M, Hu Y, Li J, Abedi V, et al. Obesity and mortality after the first ischemic stroke: is obesity paradox real? PLoS One. 2021;16
Ovbiagele B, Bath PM, Cotton D, Vinisko R, Diener HC. Obesity and recurrent vascular risk after a recent ischemic stroke. Stroke. 2011;42:3397–U3129.
Andersen KK, Olsen TS. The obesity paradox in stroke: lower mortality and lower risk of readmission for recurrent stroke in obese stroke patients. Int J Stroke. 2015;10:99–104.
Carter JL, Abdullah N, Bragg F, Murad NAA, Taylor H, Fong CS, et al. Body composition and risk factors for cardiovascular disease in global multi-ethnic populations. Int J Obes. 2023;47:855–64.
Tu W-J, Hua Y, Yan F, Bian H, Yang Y, Lou M, et al. Prevalence of stroke in China, 2013-2019: a population-based study. Lancet Regional Health-Western Pacific. 2022:28.
Powell-Wiley TM, Poirier P, Burke LE, Despres JP, Gordon-Larsen P, Lavie CJ, et al. Obesity and cardiovascular disease: a scientific statement from the American Heart Association. Circulation. 2021;143:E984–E1010.
Suthahar N, Meems LMG, Withaar C, Gorter TM, Kieneker LM, Gansevoort RT, et al. Relative fat mass, a new index of adiposity, is strongly associated with incident heart failure: data from PREVEND. Sci Rep. 2022:12.
Ruan H, Zhang Q, Ran X. Unraveling the obesity paradox in Sepsis patients: insights into the role of body composition and adipose distribution. Crit Care Med. 2023;51:E249–50.
Chung JY, Lee BN, Kim YS, Shin B-S, Kang HG. Sex differences and risk factors in recurrent ischemic stroke. Front Neurol. 2023:14.
Tanne D, Koren-Morag N, Graff E, Goldbourt U, Grp BIPS. Blood lipids and first-ever ischemic stroke/transient ischemic attack in the Bezafibrate infarction prevention (BIP) registry - high triglycerides constitute an independent risk factor. Circulation. 2001;104:2892–7.
Zhang Y, Tuomilehto J, Jousilahti P, Wang Y, Antikainen R, Hu G. Total and high-density lipoprotein cholesterol and stroke risk. Stroke. 2012;43:1768–74.
Zhang Q, Ding H, Yan J, Wang W, Ma A, Zhu Z, et al. Plasma tissue Kallikrein level is negatively associated with incident and recurrent stroke: a multicenter case-control study in China. Ann Neurol. 2011;70:265–73.
Slee VN. The international classification of diseases: ninth revision (ICD-9). Ann Intern Med. 1978;88:424–6.
Bays HE, Toth PP, Kris-Etherton PM, Abate N, Aronne LJ, Brown WV, et al. Obesity, adiposity, and dyslipidemia: a consensus statement from the National Lipid Association. Journal of Clinical Lipidology. 2013;7:304–83.
Komaroff M. For researchers on obesity: historical review of extra body weight definitions. J Obes. 2016;2016:2460285–5.
Ruan H, Li S-S, Zhang Q, Ran X. Elevated MMP-8 levels, inversely associated with BMI, predict mortality in mechanically ventilated patients: an observational multicenter study. Critical care (London, England). 2023;27:290–0.
Vemmos K, Ntaios G, Spengos K, Savvari P, Vemmou A, Pappa T, et al. Association between obesity and mortality after acute first-ever stroke the obesity-stroke paradox. Stroke. 2011;42:30–6.
Dehlendorff C, Andersen KK, Olsen TS. Body mass index and death by stroke no obesity paradox. Jama Neurology. 2014;71:978–84.
Andersen KK, Olsen TS. Body mass index and stroke: overweight and obesity less often associated with stroke recurrence. J Stroke Cerebrovasc Dis. 2013;22:E576–81.
Doehner W, Schenkel J, Anker SD, Springer J, Audebert HJ. Overweight and obesity are associated with improved survival, functional outcome, and stroke recurrence after acute stroke or transient ischaemic attack: observations from the TEMPiS trial. Eur Heart J. 2013;34:268–77.
Mueller MJ, Geisler C. Defining obesity as a disease. Eur J Clin Nutr. 2017;71:1256–8.
Deurenberg P, Weststrate JA, Seidell JC. Body mass index as a measure of body fatness: age- and sex-specific prediction formulas. Br J Nutr. 1991;65:105–14.
Verbeke T. Generalized additive models: an introduction with R. J R Stat Soc Ser A Stat Soc. 2007;170:262–2.
Ali S, Akhlaq F, Imran AS, Kastrati Z, Daudpota SM, Moosa M. The enlightening role of explainable artificial intelligence in medical & healthcare domains: a systematic literature review. Comput Biol Med. 2023;166
Leisman DE. Ten pearls and pitfalls of propensity scores in critical care research: a guide for clinicians and researchers. Crit Care Med. 2019;47:176–85.
Venkataramani AS, Bor J, Jena AB. Regression discontinuity designs in healthcare research. Bmj-British Medical Journal. 2016;352
Maciejewski ML, Basu A. Regression discontinuity design. Jama-Journal of the American Medical Association. 2020;324:381–2.
Linden A, Adams JL. Combining the regression discontinuity design and propensity score-based weighting to improve causal inference in program evaluation. J Eval Clin Pract. 2012;18:317–25.
Imbens GW. Matching methods in practice three examples. J Hum Resour. 2015;50:373–419.
Wang Z, Wang J, Wang J, Liao Y, Hu X, Wang M. The obesity paradox in intracerebral hemorrhage: a systematic review and meta-analysis. Front Endocrinol. 2023;14
Menet R, Bernard M, ElAli A. Hyperlipidemia in stroke pathobiology and therapy: insights and perspectives. Front Physiol. 2018;9
Li F, Du X, He L, Jiang C, Xia S, Ma C, et al. Relationship between serum lipid levels and ischemic stroke in patients with atrial fibrillation: a nested case-control study based on the China atrial fibrillation registry. BMC Cardiovasc Disord. 2021;21
Kim BJ, Lee S-H, Jung K-H, Yu K-H, Lee B-C, Roh J-K. Korean stroke registry I: dynamics of obesity paradox after stroke, related to time from onset, age, and causes of death. Neurology. 2012;79:856–63.
Yan J, Li X, Long W, Yuan T, Xian S. Association between obesity and lower short- and Long-term mortality in coronary care unit patients: a cohort study of the MIMIC-III database. Front Endocrinol. 2022;13
Pasco JA, Nicholson GC, Brennan SL, Kotowicz MA. Prevalence of obesity and the relationship between the body mass index and body fat: cross-sectional, population-based data. PLoS One. 2012;7
Acknowledgements
We would like to express our deepest gratitude to Frank Hu for their invaluable statistically guidance and support throughout the course of this study. Furthermore, we acknowledge and appreciate the contribution of all individuals involved in data collection and follow-up.
Funding
This study was supported by funding from the National Natural Science Foundation of China (Grant No. 82271358), the Scientific Research Foundation for Returned Overseas Chinese Scholars of Tongji Hospital, Tongji Medical College, Huazhong University of Science and Technology, and the Talent Project of Public Health in Hubei Province (Grant No. 2022SCZ048).
Author information
Authors and Affiliations
Contributions
H.R. and X.R. was responsible for statistical analyses, and the initial draft of the manuscript. S–S.L. conducted data cleaning and contributed to the study design, data analysis and interpretation. Q.Z. contributed to revising the manuscript critically for intellectual content and approved the final version for publication. All authors reviewed the manuscript critically for intellectual content and have read and approved the final manuscript.
Corresponding authors
Ethics declarations
Ethics approval and consent to participate
This study was approved by the ethics committee (Approval No. TJ-IRB20230830).
Competing interests
The authors declare no competing interests.
Additional information
Publisher’s Note
Springer Nature remains neutral with regard to jurisdictional claims in published maps and institutional affiliations.
Rights and permissions
Open Access This article is licensed under a Creative Commons Attribution 4.0 International License, which permits use, sharing, adaptation, distribution and reproduction in any medium or format, as long as you give appropriate credit to the original author(s) and the source, provide a link to the Creative Commons licence, and indicate if changes were made. The images or other third party material in this article are included in the article's Creative Commons licence, unless indicated otherwise in a credit line to the material. If material is not included in the article's Creative Commons licence and your intended use is not permitted by statutory regulation or exceeds the permitted use, you will need to obtain permission directly from the copyright holder. To view a copy of this licence, visit http://creativecommons.org/licenses/by/4.0/. The Creative Commons Public Domain Dedication waiver (http://creativecommons.org/publicdomain/zero/1.0/) applies to the data made available in this article, unless otherwise stated in a credit line to the data.
About this article
Cite this article
Ruan, H., Ran, X., Li, Ss. et al. Dyslipidemia versus obesity as predictors of ischemic stroke prognosis: a multi-center study in China. Lipids Health Dis 23, 72 (2024). https://doi.org/10.1186/s12944-024-02061-9
Received:
Accepted:
Published:
DOI: https://doi.org/10.1186/s12944-024-02061-9