Abstract
The partial stochastic asymptotic stability of neutral stochastic functional differential equations with Markovian switching (NSFDEwMSs) has been investigated, the sufficient conditions for partial stochastic asymptotic stability have been given. We have used the boundary condition and to replace the condition and have generalized some results of Sharov and Ignatyev to cover a class of much more general NSFDEwMSs.
MSC:39A11, 60H10, 37H10.
Similar content being viewed by others
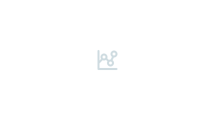
1 Introduction
In recent years, Lyapunov’s second method has been a very important method to study the stability of a stochastic differential equation, which has attracted much attention since it is not only academically challenging, but also of practical importance, and has played an important role in many fields such as insurance, finance, population dynamic, control (see, e.g., Øsendal [1]). Recently, much work has been done on stochastic differential equations with Markovian switching (see, e.g., Mao and Yuan [2]). In particular, significant work has appeared on the stability of stochastic differential delay equations with Markovian switching (see, e.g., Kolmanovskii et al. [3], Luo [4], Luo et al. [5], Mao et al. [6], Yuan and Mao [7], Yuan et al. [8], Mao et al. [9], Liu [10, 11]).
On the other hand, many dynamical systems depend not only on present and past states, but also involve derivatives with delays. Motivated by chemical engineering systems as well as the theory of aero elasticity, many researchers have been interested in the neutral stochastic functional differential equations, and the neutral part, which has been used in investigating the qualitative properties of derivatives of delays for dynamical systems, has been added to all kinds of equations. In particular, the significant work has been done (see, e.g., Mao [12], Luo et al. [13]).
The global stability of SDEwMSs with respect to sample paths or moments, which is too strong for many cases, appeared in many more papers. So, the notion of the partial stability (see, e.g., Peiffer and Rouche [14], Rouche et al. [15]) has been involved, and the second method of Lyapunov as an indispensable tool has been used to investigate the partial stability (see, e.g., Sontag and Wang [16], Vorotnikov [17], Vorotnikov and Rumyantsev [18], Ignatyev [19, 20]).
However, in the process of investigating the qualitative properties of equilibria and boundedness properties of motions of dynamical systems, the partial stability as a new concept plays the key role, and the systems are often determined by all kinds of equations, including stochastic differential equations, of course. Motivated by the previously mentioned topic, and since the results of the partial stochastic asymptotic stability of neutral stochastic functional differential equations with Markovian switching are very few, we make the attempt to study this topic to fill the gap.
This paper is organized as follows. In Section 2, we present some basic preliminaries and the form of neutral stochastic functional differential equations with Markovian switching. In Section 3, the sufficient conditions for partial stochastic asymptotic stability have been obtained and proofs have been given. In Section 4, some well-known results are generalized in the remarks. In Section 5, there is the discussion about the main results.
2 Preliminaries and definitions
Let be a complete probability space with a filtration satisfying the usual conditions, i.e., the filtration is continuous on the right and contains all P-zero sets. is an m-dimensional Brownian motion defined on the probability space. Let denote the family of functions φ from to that are right-continuous and have limits on the left. is equipped with the norm and for any . If A is a vector or matrix, its trace norm is denoted by , while its operator norm is denoted by . Denote to be the family of all -measurable bounded -valued random variables . Let , , denote the family of all measurable, -valued random variables such that .
Let be a right-continuous Markov chain on the probability space taking values in a finite state space with a generator given by
where . Here is the transition rate from i to j, if , while
We assume that the Markov chain is independent of the Brownian motion . It is known that almost every sample path of is a right-continuous step function with a finite number of simple jumps in any finite sub-interval of .
Consider the following neutral stochastic functional differential equation with Markovian switching:
with the initial condition , where and , , which is independent of , and . Let . Here, we furthermore assume that
For our purpose, we impose the following assumptions which can ensure the existence and uniqueness of a solution, denoted by on , for equation (2.1). Let f and g satisfy the local Lipschitz and linear growth condition. That is, for each , there exist constants , and such that
Denote , where and , , and the definitions of and are similar to . The domain , and the stopping time is the first exit time from the of the sample path of the process . Let , then . Denote the set of functions .
Denote by the family of all non-negative functions on , which are twice continuously differential with respect to x and once continuously differential with respect to t. For any , define an operator LV by
where
Definition 2.1 The trivial solution of equation (2.1) is said to be partial stochastic stability with respect to if, for any and , there exists a such that
whenever .
Definition 2.2 The trivial solution of equation (2.1) is said to be partial stochastic asymptotic stability with respect to if it is the -stochastic stability and for any , there exists a such that
whenever .
3 Main results
First of all, we should present Sharov’s theorem [21].
Theorem 3.1 (Sharov [21])
If in the domain there exists a function which satisfies the following conditions:
-
(I)
, where ;
-
(II)
, where ;
then the trivial solution of system (2.1) is -stochastic asymptotic stability.
The condition of Theorem 3.1 is more difficult to be satisfied, which is demonstrated by the following example.
Example 3.1 Consider the system of stochastic differential equations
where a, b, c are constants and . The system has the trivial solution , .
Denote , we have .
Now, by Theorem 3.1, we cannot give whether the solution of (3.1) is stochastic asymptotic stability or not. So, we present the main results of this paper as follows.
Theorem 3.2 Let there exist a non-negative functional such that
-
(I)
, where ;
-
(II)
;
-
(III)
(Boundary condition) For any sufficiently small , , any solution of equation (2.1), beginning in the domain , is such that
Then the trivial solution of equation (2.1) is said to be partial stochastic asymptotic stability with respect to .
Before giving the proof of Theorem 3.1, we should present the following lemma.
Lemma 3.1 Let be a function in the class , bounded in the domain , and suppose that in this domain. Then the process is a supermartingale so that , for .
Proof As the generalized Itô formula, we have
where is the martingale.
For any , and , we derive that
So, is a supermartingale. Moreover, due to in the domain,
so the proof is completed. □
Proof of Theorem 3.2 By Lemma 3.1 and Doob’s supermartingale convergence theorem, the process is a supermartingale, and we can get
Denote . Owing to and the partial stochastic stability theorem (Sharov [21]), the solution of equation (2.1) is the partial stochastic stability with respect to , so we have
Combining this with condition (III) and for all the paths of , then
By the lemma of Has’minskii [22], that is, the coefficients of equation (2.1) satisfy the local Lipschitz and linear growth condition in every domain bounded, and the process is regular, then the set is inaccessible to any sample path of the process if , where , so we get
Noting the similar definition of , obviously,
and we get that
Therefore,
and (3.4), we have
Similar to the proof of (3.5), we get
Combining this with condition (I) () and (3.7), we obtain
Owing to (3.2), the limit
exists for almost all paths in . By (3.8) and (3.9), we have the limit of the above equation , a.s., since the condition (I) (). For all the paths of , we can imply that
Moreover,
Noting the similar definition of , obviously,
we get that
By (3.11), then
Combining this with conditions (3.5) and (3.12) yields
So, we have obtained that the trivial solution of equation (2.1) is partial stochastic asymptotic stability with respect to with (3.3) and (3.13). □
4 Remarks
Remark 4.1 When , and , equation (2.1) reduces to
which has recently been studied in Ignatyev [23], that is to say, Theorem 3.1 of Ignatyev [23] has been generalized.
Remark 4.2 The operator LV of equation (4.1) of Theorem 3.1 (Sharov [21]) must be negative, but we can obtain the partial stochastic asymptotic stability of equation (2.1) under LV being non-positive (), which makes the conditions of the solution more feasible to be satisfied.
5 Discussion of main results
Lyapunov methods have been developed to research the conditions of the partial asymptotic stochastic stability of neutral stochastic functional differential equations with Markovian switching. We have the non-positive Lyapunov operator and boundary condition to weaken the conditions of the previous theorems, but there is a small problem that the boundary condition of Theorem 3.2 is a stochastic domain. We will obtain the equivalent and practical results for the boundary condition in the future investigation.
References
Øsendal B: Stochastic Differential Equations. 5th edition. Springer, Berlin; 2003.
Mao X, Yuan C: Stochastic Differential Equations with Markovian Switching. Imperial College Press, London; 2006.
Kolmanovskii V, Koroleva N, Maizenberg T, Mao X, Matasov A: Neutral stochastic differential delay equations with Markovian switching. Stoch. Anal. Appl. 2003, 21: 819–847. 10.1081/SAP-120022865
Luo J: Comparison principle and stability of Itô stochastic differential delay equations with Poisson jump and Markovian switching. Nonlinear Anal. 2006, 64: 253–262. 10.1016/j.na.2005.06.048
Luo J: Second-order quasilinear oscillation with impulses. Comput. Math. Appl. 2003, 46: 279–291. 10.1016/S0898-1221(03)90031-9
Mao X: The Lasalle-type theorems for stochastic differential equations. Nonlinear stud. 2000, 7: 307–328.
Yuan C, Mao X: Robust stability and controllability of stochastic differential delay equations with Markovian switching. Automatica 2004, 40: 343–354. 10.1016/j.automatica.2003.10.012
Yuan C: Stability in terms of two measures for stochastic differential equations. Dyn. Contin. Discrete Impuls. Syst. 2003, 10: 895–910.
Mao X: Stabilisation of hybrid stochastic differential equations by delay feedback control. Syst. Control Lett. 2008, 57: 927–935. 10.1016/j.sysconle.2008.05.002
Liu D, Yang Y: Doubly perturbed neutral diffusion processes with Markovian switching and Poisson jumps. Appl. Math. Lett. 2010, 23: 1141–1146. 10.1016/j.aml.2009.07.004
Liu D, Yang G, Zhang W: The stability of neutral stochastic delay differential equations with Poisson jumps by fixed points. J. Comput. Appl. Math. 2011, 10: 3115–3120.
Mao X: Stochastic Differential Equations and Their Applications. Horwood, Chichester; 1997.
Luo Q, Mao X, Shen Y: New criteria on exponential stability of neutral stochastic differential equations. Syst. Control Lett. 2006, 55: 826–834. 10.1016/j.sysconle.2006.04.005
Peiffer K, Rouche N: Liapunov’s second method applied to partial stability. J. Méc. 1969, 6(2):20–29.
Rouche N, Habets P, Laloy M: Stability Theory by Liapunov’s Direct Method. Springer-Verlag, New York; 1977.
Sontag ED, Wang Y: Lyapunov characterizations of input to output stability. SIAM J. Control Optim. 2001, 39(1):226–249.
Vorotnikov VI: Partial Stability and Control. Birkhauser, Boston; 1998.
Vorotnikov VI, Rumyantsev VV: Stability and Control with Respect to a Part of the Phase Coordinates of Dynamic Systems: Theory, Methods, and Applications. Scientific World, Moscow; 2001. in Russian
Ignatyev AO: On the partial equiasymptotic stability in functional differential equations. J. Math. Anal. Appl. 2002, 268: 615–628. 10.1006/jmaa.2001.7835
Ignatyev OA: On the partial asymptotic stability in nonautonomous differential equations. Differ. Integral Equ. 2006, 19(7):831–839.
Sharov VF: Partial stability and stabilization of stochastic systems. Avtom. Telemeh. 1978, 11: 63–71. in Russian
Has’minskii RZ: Stochastic Stability of Differential Equations. Sijthoff & Noordhoff, Rockville; 1980.
Ignatyev OA: Partial asymptotic stability in probability of stochastic differential equations. Stat. Probab. Lett. 2009, 79: 597–601. 10.1016/j.spl.2008.10.005
Acknowledgements
The authors are very grateful to the anonymous referees for their valuable suggestions. This work was partially supported by HSSF for the Ministry of Education (10YJC630143), NNSF of China (61074006, 71171001), NSSF of China (12BTJ008), PNSF of Anhui (1208085QG131, KJ2011B005, 2011SQRL068).
Author information
Authors and Affiliations
Corresponding author
Additional information
Competing interests
The authors declare that they have no competing interests.
Authors’ contributions
The authors have achieved equal contributions to each part of this paper. All the authors read and approved the final manuscript.
Rights and permissions
Open Access This article is distributed under the terms of the Creative Commons Attribution 2.0 International License (https://creativecommons.org/licenses/by/2.0), which permits unrestricted use, distribution, and reproduction in any medium, provided the original work is properly cited.
About this article
Cite this article
Liu, D., Wang, W., Ignatyev, O. et al. Partial stochastic asymptotic stability of neutral stochastic functional differential equations with Markovian switching by boundary condition. Adv Differ Equ 2012, 220 (2012). https://doi.org/10.1186/1687-1847-2012-220
Received:
Accepted:
Published:
DOI: https://doi.org/10.1186/1687-1847-2012-220