Abstract
This article explores spatial patterns of demographic change and residential segregation in Italy over the past decade, a topic which has not been studied in detail in the literature. Population ageing and migration are unfolding rapidly in a number of European countries, generating tensions and challenges at local level. Aggregate regional or national statistics can conceal significant variations at local level, which are of considerable interest and relevance. This is particularly the case in Italy, where spatial heterogeneity and regional disparities are marked. The analysis presented in this paper uses a new source of data derived from large public archives, which permits comparisons to be made at local level with the 2011 census of population. In this way, it is possible to map out and to analyse demographic trends at a fine level of spatial definition. In order to exploit the potential of these data, the authors use a new set of spatial units which were derived by applying automatic rezoning procedures. These output areas are well suited to the study of the age structure of local populations, population change, and migration in a uniform way across the entire national territory, as the empirical results confirm.
Similar content being viewed by others
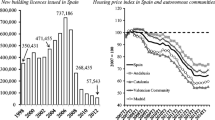
Avoid common mistakes on your manuscript.
1 Introduction
This article explores spatial patterns of demographic change and residential segregation in Italy over the past decade, a topic which has not been studied in detail due to the lack of longitudinal data for comparable spatial units at local level. In the analysis presented below, we use a new source of data derived from large administrative databases which permits comparisons to be made at local level with data from the 2011 census of population. This is possible for the first time because the new indicators were released using the small area definitions that were used in the last census. In this way, it is possible to map out and to analyse demographic trends regarding characteristics such as population movement, changes in the age structure and migration at a fine level of detail.
This information is potentially of interest to policy-makers, practitioners in health, local development and social work, and researchers working with spatial models or geocoded data. As the boundaries of census enumeration areas in Italy are revised at regular intervals—leading to sweeping changes in their boundaries—it has proved difficult to carry out longitudinal analysis across the whole country. In order to exploit the potential of the new data, we use a new set of spatial units which we derived by applying automatic rezoning procedures to the census enumeration areas for 2011. We begin by describing how these areas were defined, before using them to look at the age structure of local populations, population change and geographical distribution, making comparisons at a range of levels in order to illustrate how these phenomena are structured in spatial terms.
This article contributes to the literature on spatial patterns of social and demographic change and on the reproduction of local populations in Italy and Europe. This is an important area of research because of the sharp spatial disparities that exist between different areas and regions, both in terms of the North–South divide and other cleavages which are related to the morphology of the Italian peninsula and the form of urban areas: between mountainous and low-lying regions, mainland and islands, coastal and inland areas, centre and periphery, to name only the most important.
Demographic processes involving population ageing, declining fertility rates, immigration and emigration are unfolding rapidly in Italy, generating tensions and challenges at local level. Detailed local data and maps are useful tools when seeking to understand these pressures and to develop policies for managing them. In particular, it is important to assess how migration relates to demographic decline and how these processes are influencing the age profile and sustainability of local communities in different parts of the country. The development of a new set of spatial units and the use of longitudinal data allows us to provide a detailed but robust overview of population change at local level which is without precedent in Italy.
2 Use of Small Area Population Statistics in Italy
Small areas are subnational units for which area-specific samples from national surveys are too small to provide reliable estimates (Rao, 1999). They include census tracts, electoral districts, school catchment areas, postcode areas, city blocks and municipalities. In applied research, it is often useful to have spatial data at different spatial scales, as this facilitates the measurement of socio-economic conditions at the appropriate level.
Many studies of social conditions in Italy have been confined to a subset of geographical areas, and Cadum et al. (1999) are practically alone in studying the entire national territory. Andreoli et al. (2017) examine the spatial distribution of deprivation in the main Italian cities, Bressan et al. analyse Prato (Bressan et al., 2006), Lemmi confines his attention to the Provinces of Modena and Trento (Lemmi, 2007), Benassi studies Milan and Naples (Benassi, 2002a, 2002b), Morlicchio and Pratschke focus on Naples (Morlicchio & Pratschke, 2004), Pratschke (2007) and Lelo et al. (2018), examine Milan, Rome and Naples, Lillini et al. study Liguria (Lillini et al., 2012), Valerio and Vitullo confine their attention to Basilicata (Valerio & Vitullo, 2000) and Cabras et al. focus on Sardinia (Cabras et al., 2012).
Many of these authors note that the use of large and heterogeneous spatial units is sub-optimal and emphasise the difficulties involved in accessing appropriate data for smaller units with a uniform coverage. Some researchers have even used the tiny census enumeration areas to analyse the characteristics of local communities (Barbieri et al., 2018; Caranci et al., 2010) or have combined them with non-census data (Marinacci et al., 2009; Schifano et al., 2009). However, measures based on units with such small populations tend to have low reliability and the choice of variables is necessarily limited to those with large denominators.
Similar observations have been made in relation to health, with Costa and Marinacci (2007) observing that the use of municipalities when modelling health data leads to weaker and less regular associations than are observed in other countries where smaller units are typically used (Costa et al., 2011; Succi, 2016). The opposite problem has also been noted:
Another critical aspect in the use of [deprivation indices] is related to the population size of the territorial unit as reducing it does not automatically lead to a better estimate of deprivation. In fact, smaller units are more homogeneous but [the deprivation index] becomes unstable due to greater sensitivity to local variation. (Pasetto et al., 2010, p. 193)
This is the fundamental dilemma that researchers face when seeking to explore social phenomena in Italy from a small area perspective: to use large and heterogeneous units that vary in size and population or to use units with very small populations which are sensitive to random variation. Where researchers are forced to use inappropriate scales and sub-optimal aggregations, the potential for bias is considerable and the range of techniques available is artificially curtailed. At the international level, Zhao and Exeter (2016) have developed new spatial units for studying social phenomena in New Zealand, Verhoef and Van Eeden (2015) have done the same in the South African context.
3 The Census and Small Area Data
Not all social research is carried out at very fine spatial scales, of course, and the boundaries of spatial units should arguably match the geography of the social process that is under scrutiny (Martin, 2000; Stillwell et al., 2018). The size of the spatial units used in research can have a considerable impact on the patterns and relationships observed, a phenomenon that has been studied since at least the 1930s (Gehlke & Biehl, 1934). The shape of the boundaries of spatial units is also relevant, as the way in which they are defined can influence the results, which is known as the Modifiable Areal Unit Problem (MAUP) (Fotheringham, 2009). This means that when defining or choosing areas, care must be taken to ensure that their boundaries are sociologically meaningful in the context of the phenomena under study and that the areas themselves are as homogeneous as possible.
In recent years, a number of national statistics offices have developed output geographies to facilitate social research. Perhaps the most well-known of these is the hierarchy of areas designed in the UK for the 2001 census, which was updated subsequently (Vickers & Rees, 2007). The smallest of these units is the Output Area (OA), with an average population of 309 (in 2011). A total of 175,434 Output Areas were created for the 2001 census, increasing to 181,408 in 2011. For analyses requiring areas with larger populations, two other sets of units are available: Lower Layer Super Output Areas (LSOAs, with a population of around 1,600) and Middle Layer Super Output Areas (with an average population of approximately 7,800).
In order to maintain a uniform unit-level population, a target is set and upper and lower thresholds enforced. For example, the lower threshold for the UK Output Areas is 100 (roughly 40 households), while the upper threshold is 625 people (or 250 households). These thresholds are sufficient to protect individual privacy and to prevent areas from having excessively large populations. It should be noted, however, that even when thresholds like these are imposed, it is impossible to avoid some small areas having larger populations, such as where a large number of residents reside in a single apartment building.Footnote 1
Although it is useful to have an output geography that is based on areas with just a few hundred residents, these are too small for many applications. From this perspective, the LSOAs, with roughly 1,600 residents, are more suitable for studying local populations, as they are large enough to yield reliable rates but small enough to be relatively homogeneous (Haynes et al., 2007). They are in line with the population size of the recently-introduced IRIS units in France, which have proved useful in applied social research (Oberti & Preteceille, 2017). We will therefore adopt a mean population 1,600 as a target when constructing output areas for Italy.
The Italian census of population relies on a single set of enumeration areas, known as the sezioni di censimento. The country was divided into 410,441 such areas in 2011, more than twice the number of Output Areas in the UK. The enumeration areas have a very small average population and many have no residents at all, as there is no lower limit to their population. The data files provided by ISTAT contain data on 366,863 areas; the remainder (43,578) represent special geographical features which have only boundary definitions with the sole aim of representing topographical features.Footnote 2
Due to their small populations and irregular boundaries, Italian census enumeration areas are not well-suited to social or demographic research. Other administrative units are, by contrast, too large and have heterogeneous populations. By selectively aggregating enumeration areas, it is possible to obtain a new set of intermediate spatial units with relatively uniform populations, a simpler geometry and greater internal homogeneity.
The population of the Italian census enumeration areas in 2011 ranged from 0 to 7,647,Footnote 3 with a mean of 162.01 and a standard deviation of 233.50. Even after removing special geographical features, there were still 17,830 tracts with no inhabitants (4.86%) and 157,013 with a population below 50 (42.80%). At the other extreme, only 51 tracts had more than 2,500 residents.
A step up in size from these enumeration areas, Italy also has a set of spatial units known as the aree di censimento (ACE), which were developed to facilitate sampling operations as the Italian census was moving away from complete enumeration and towards sampling and estimation (Bianchi et al., 2007). The population of the ACE areas ranges from 13,000 to 18,000 residents, and they are defined for all municipalities with at least 20,000 residents (i.e. urban areas). There is at least one precedent for using ACE areas to map out census data in social research (Andreoli et al., 2017). However, these areas do not cover the entire territory of urban areas, with the result that some enumeration areas remain in a heterogeneous, residual, non-contiguous set.
The next largest spatial units are the urban circoscrizioni (which form part of the decentralised government structure of large cities, and are generically referred to as aree subcomunali—ASC), followed by comuni (municipalities). Like the ACE, the circoscrizioni are generally (but not always) large in size; for example, the population of the largest unit in Milan in 2011 was 57,087 (Buenos Aires—Venezia), while in Rome it was 80,311 (Torre Angela) and in Naples it was 71,808 (Fuorigrotta). Outside the main cities, however, even the municipalities can have small populations—well below 1,000 residents in some cases. Sociological analyses based on spatial units such as these reveal only macroscopic differences in the spatial structure of the large cities and perform poorly when seeking to explore residential patterns.
Other subdivisions exist for specific parts of the country, such as school catchment areas (137 for Milanese primary schools, for example) or planning areas (155 zone urbanistiche in Rome and 71 unità urbanistiche in Genoa). None of these aggregate-level units are universal, uniform or suitable for comparative social research across cities and regions, spanning the urban–rural divide. There are also postcode areas in Italy, but these are very large; for example, there are just 38 for the whole of Milan.
A second specificity of the Italian census enumeration areas is that their boundaries are quite unlike those of the elementary spatial units found in other countries. The criteria that guides the definition of these units lead to highly irregular and often encapsulated polygons that do not facilitate mapping or modelling applications. This is because they are defined in relation to settlements, and their boundaries are continually revised to keep track of how settlements expand (see Fig. 1).
The Italian spatial data infrastructure reflects a different view of how spatial units should be defined: in most countries, these units are held stable so that they can be used to measure change over time in relation to an indicator, while in Italy the units themselves reflect demographic change. Unfortunately, there is no easy way of aligning tracts from two or more censuses to carry out longitudinal analysis.Footnote 4 All three censuses carried out since 1991 have been preceded by extensive redefinition of the boundaries of the enumeration areas (Crescenzi, 2002).
4 Rezoning Methodology
In order to construct a new output geography, we started with the existing census enumeration areas and aggregated them selectively using an automated, iterative algorithm. The aim was to obtain output areas that were as socially homogeneous as possible, had a uniform population size, respected upper and lower population thresholds, had a more regular geometrical form and did not cross the boundaries of the circoscrizioni and municipalities, which are the most important higher-level administrative areas. Optimally aggregating the many hundreds of thousands of tracts in Italy whilst respecting these criteria cannot be achieved through manual coding operations or visual inspection.
An automated algorithm to deal with this challenge was proposed by Stan Openshaw in Transactions of the Institute of British Geographers (Openshaw, 1977, p. 462). He distinguishes between the basic spatial units for which data are available and the zones required for a specific application or analysis. A partition is a disjoint set of zones which completely covers a country, region, or other area so that each basic spatial unit is allocated to one zone and all units that belong to a zone are spatially contiguous. Openshaw’s algorithm uses an arbitrary initial partition as a starting point and then seeks to improve it through an iterative hill-climbing approach. His procedure was improved by Openshaw and Rao (Openshaw & Rao, 1995) and has been discussed within several disciplines (Duque et al., 2007).
In constructing a new output geography for Italy, we used David Martin’s operationalisation of Openshaw’s AZP algorithm (Martin, 1997, 2000, 2002; Martin et al., 2001; Ralphs & Ang, 2009).Footnote 5 Previous analyses using this approach have yielded satisfactory outcomes (Cockings & Martin, 2005; Flowerdew et al., 2008; Grady & Enander, 2009; Haynes et al., 2008; Mokhele et al., 2016).
Auxiliary data from the census (roughly 140 socio-demographic indicators for 2011) are available for the enumeration areas and can be downloaded from the ISTAT website.Footnote 6 To guide the process of aggregation, we created a simple composite measure based on the unemployment rate, mean number of persons per room and educational attainments. Scores were not calculated for areas with a population below 10, which were assigned to a special category which was merged during the final stage of aggregation. This permitted greater control over the population of the output areas as well as yielding simpler geometrical forms and smoother boundaries.
The criteria used during the aggregation included population (with soft upper and lower thresholds of 2,500 and 500 respectively), homogeneity (based on the aforementioned composite measure of deprivation), geometrical shape (simple forms were preferred over complex ones), spatial contiguity and respect for the boundaries of important administrative areas. A large number of iterations was used to ensure that the algorithm converged on a stable outcome. The final result was a set of zones with a mean population of 1,653 and a standard deviation of 489 using the 2011 census data.Footnote 7
Table 1 shows the number of output areas and the minimum, maximum, mean and standard deviation of their 2011 population by region, excluding special physical features like rivers and lakes. The distribution is roughly normal, with a large share of areas having between 1,000 and 2,500 residents. Figure 2 shows the boundaries of both the tracts and the new output areas for one region (Lombardy). The rezoning procedure yields a ten-fold reduction in the number of spatial units, whilst preserving an appropriate level of detail which is well-suited to the production of maps.
5 Measuring Change Over Time
Small area data from the 2011 Italian census were originally released to the research community in May 2015, covering a subset of the information that had been collected four years previously. It was possible to obtain additional small area data from the office of national statistics, but this involved a complex procedure potentially involving the suppression or alteration of counts for small areas in order to protect the privacy of citizens.
In June 2023, ISTAT released a new set of small area estimates based primarily on registry data, with a reference date of 31st December 2021.Footnote 8 As the new enumeration areas (basi territoriali 2021) were not yet ready, the office of national statistics decided to release the new data using the older 2011 enumeration areas. Although this decision was unexpected, it created an opportunity to explore patterns of change over time at the local level. For example, it is possible to study demographic decline in isolated rural communities (as measured by population decline or the age dependency ratio) as well as the residential geographies of foreign citizens. This also means that we can analyse both sets of statistical data using the output areas described above, comparing 2011 and 2021 data directly. Table 2 summarises key demographic indicators by region, showing the values observed in 2011 and 2021 at this level.
The two most striking forms of demographic change observed between 2011 and 2021 involve population decline and immigration, with both processes manifesting strong spatial patterns. Population loss was greatest in Molise, Abruzzo, Basilicata and Calabria in the South, which have extensive mountainous areas. For example, there was an overall decline of more than 6% in the population of both Molise and Basilicata over the course of just ten years. Some of the output areas in these regions recorded population losses of 15–20%, indicating that many communities are rapidly declining due to the combined effects of emigration (both historical and contemporary) and population ageing (see Fig. 3).
Population change was less negative in the North of Italy, with some increases in the mountainous areas of Bolzano, Sondrio and Aosta, as well as along the urban corridors that extend from Milan towards Piacenza, Modena and Bologna to the South-East and through Bergamo, Brescia, and Verona towards Venezia, to the East. Lazio also recorded impressive growth (3.85%, compared to a national decline of -0.69%).
Turning to the age structure,Footnote 9 a clear pattern is evident from Fig. 4, which is due to persistent low level of fertility and to emigration from relatively isolated, mountainous areas along the Appennines, stretching from Liguria to Calabria. People tend to leave these areas when they reach working age, leaving behind a population where the share of children (under 15 years) and elderly people (65 years and over) is relatively large. In Liguria, the age dependency ratio is 65.75%, compared to 57.46% in Italy as a whole. In many parts of the area that falls with the Antola Regional Park to the East of Genoa, the age dependency ratio exceeds 100. These are also areas of rapid population loss, as Fig. 3 confirms. It is interesting to see that this phenomenon does not affect the Alps to the same extent, presumably due to opportunities associated with tourism. Demographic change has accelerated in recent years in areas like Grosseto (Maremma), Arezzo and in the delta of the River Po, having peaked in isolated rural areas across the South in previous decades.
Figure 5 shows the number of foreign citizens in output areas across Italy. This variable shows people who are officially resident, excluding undocumented migrants as well as those who may have acquired Italian citizenship after settling in Italy. As the population of the output areas is relatively uniform, the spatial distribution shown in the map is immediately interpretable. The cities of the Centre-North have the greatest population diversity, with foreign citizens being attracted primarily to Lazio, Lombardy and Emilia-Romagna as a result of demand for low-skilled labour. In many neighbourhoods across the North of Milan, foreign citizens account for one-quarter or even one-third of the population (11.62% in Lombardy as a whole) (Figs. 5, 6, 7).
The migrant population grew rapidly in Lombardy and Emilia-Romagna between 2011 and 2021, although Lazio had the highest increase in percentage terms (from 7.74 to 10.82% of the population). The foreign population in Lazio increased from 425,707 to 618,142 (+ 45%), with the population of Italian nationals remaining more or less stable (moving from 5,077,179 to 5,096,740). In short, the impressive increase recorded in this regional population was almost entirely due to immigration from abroad.
In the South of Italy, most regions have less than 5% foreign residents and the only Southern city with a significant concentration of immigrants is Naples, where up to a quarter of residents in certain central districts are foreign citizens. In the South, more generally, the only areas that have experienced significant increases are situated in or near important, labour-intensive agricultural districts (in the Province of Caserta, to the South of Battipaglia, or around Foggia, for example). The contrast is sharp between regions like Puglia, in the South, where only 3.45% of the population are foreign citizens, and Emilia-Romagna, in the North, where this applies to 12.43% of the population and no less than 17.36% of young people (aged under 30).
6 Patterns of Residential Segregation
To explore these phenomena further, we calculated global indices of residential segregation and local indices of spatial autocorrelation. The global indices cover the dimensions of evenness, concentration and clustering (Massey & Denton, 1988). Evenness refers to the distribution of population groups across the spatial units of a metropolitan area. Indices measuring evenness assess a group’s under- or over-representation at local level, with segregation being lowest when the majority and minority populations are evenly distributed. By contrast, concentration “refers to the relative amount of physical space occupied by a minority group in the metropolitan area” (Massey & Denton, 1988, p. 289). As the amount of metropolitan space occupied by a group decreases, the concentration increases; segregated minorities occupy only a small portion of the metropolitan area. Finally, clustering measures “the extent to which area units inhabited by minority members adjoin one another, or cluster, in space” (Massey & Denton, 1988, p. 293).
To measure the first dimension of segregation, we computed the Index of dissimilarity D (Duncan & Duncan, 1955). This measures the degree of under- or over-representation of a population group within a set of spatial units. We use this indicator to compare the spatial distribution of foreign citizens (minority group) to the one of the Italian nationals (majority group) using the new output areas. D varies from 0 (complete integration) to 1 (complete segregation) and it represents the share of a group’s population that would have to change residence so that each neighbourhood has the same composition as the urban area as a whole. Values above 0.6 are often observed in the presence of severe residential segregation although this threshold may vary depending on the national and local context (Massey & Denton, 1993).
As far as concentration is concerned, we use a one-group index known as the delta index (DEL) (Hoover, 1941; Duncan et al., 1961) to compare the spatial distribution of Italian nationals and foreigners. This index “computes the proportion of [minority] members residing in area units with above average density of [minority] members” (Massey & Denton, 1988, p. 290). The index varies between 0 and 1 and indicates the proportion of a group that would have to move across areal units in order to achieve a uniform density. The higher the value, the higher the absolute concentration of the group concerned and the smaller the amount of physical space it occupies (Conti et al., 2023).
The more contiguous spatial units a group occupies, the more clustered and therefore segregated it is likely to be. A high degree of clustering is observed in the presence of racial or ethnic enclaves. In this dimension we computed the Relative Clustering Index (RCL) (Massey & Denton, 1988; White, 1986). The index refers to the relative amount of physical space occupied by a minority group in the urban environment (Massey & Denton, 1988) and equals 0 when minority members display the same amount of clustering as the majority (Italian nationals, in our case), is positive when minorities display greater clustering than the majority and is negative when they are less clustered than the majority population.
We are well aware that these indices have their limitations. D, for example, depends on the spatial scale of the units employed (Wong, 2003), and its sensitivity to random allocation implies a risk of upward bias when dealing with smaller spatial units, smaller minority populations and lower segregation levels (Mazza, 2020; Mazza & Punzo, 2015). Despite these limitations, this index remains the most widely used in the study of residential segregation (Piekut et al., 2019; Mazza, 2020). The other two global indexes (DEL and RCL) are also frequently used in studies of residential segregation (Conti et al., 2023; Townsend & Walker, 2002; Xie, 2010; Yang et al., 2017). In this paper, we base our observations on careful comparisons between local labour market systems using a stable set of spatial units, which means that we avoid many of the aforementioned limitations.
In order to provide a detailed overview of changes in the spatial distribution of the foreign population in the period 2011–2021, and to shed light on distinct models of settlement, we calculated the aforementioned indices for the three largest urban centres (Milan in the North, Rome in the Centre, and Naples in the South). There has been a significant expansion of the periphery of these cities over recent decades, accompanied by the functional integration of previously autonomous urban centres in their hinterland. The transformation of these areas as a result of deindustrialisation, changing residential preferences, the growth of tourism and the expansion of leisure services in the urban core has had a significant impact on the settlement patterns of both Italians and foreign citizens. In order to explore these processes, we calculated segregation indices not only for the urban core (central municipality) but also for the municipalities that form part of the larger local labour market system (urban periphery).
The local labour market systems in Italy were defined by the Italian National Institute of Statistics (Istat) using data on work commuting from the census of population, initially with reference to 1991 and then for 2001 and 2011 (ISTAT, 2015). The method used traces a boundary around each urban area to maximise the degree of self-containment in labour market terms.Footnote 10 These boundaries coincide with a functional definition of the metropolitan urban area which has proved useful in studies of urban inequalities and internal mobility (Ascani et al., 2021; Barbieri et al., 2018; Bonifazi et al., 2021). We excluded the central municipality when calculating indices of dissimilarity and concentration for the peripheral areas, in order to permit comparisons (see Table 3 and Figs. 8, 9, 10).
As Table 3 shows, the degree of dissimilarity in the spatial distribution of foreigners compared to Italian citizens is relatively contained (< 0.6 in all cities) at both points in time. There is a broad trend towards a reduction in segregation in Rome, and the lowest level of segregation was observed in the periphery of this city in 2011 (0.226). In the other two urban areas, the dissimilarity index increased between 2011 and 2021, indicating differentiation in the residential choices of foreigners and Italian citizens. The level of dissimilarity was particularly high in the municipality of Naples in 2011 (0.442), compared to both Rome (0.266) and Milan (0.264), and the gap increased over the following decade (leading to a difference of 0.19 with respect to Milan and 0.23 with respect to Rome).
These figures suggest that the residential preferences of foreign citizens in Milan and Rome were increasingly similar to those of younger, lower-class Italian nationals, many of whom settled in more peripheral areas of these cities in the period in question, due to rising housing costs. In Naples, however, the choices of foreign citizens were increasingly different from those of Italian nationals, as they increased their presence within a cluster of quarters near the centre of the city, which were rapidly acquiring the features of an ethnic enclave. In the labour market system outside the central municipality, a similar trend was occurring, due to the concentration of foreign citizens around Castel Volturno in the Province of Caserta, and at the foothills of Mount Vesuvius.
As far as dissimilarity is concerned, peripheral areas followed the same broad trends observed in the central municipalities, with higher levels of segregation in Naples and an increase over time in the gap between the cities of the Centre-North and South. In all three cases, the periphery is less segregated than the urban core, and this disparity is particularly marked in the case of Naples, where a difference of 0.11 is observed in 2021.
These patterns of settlement are likely to have been influenced by the greater difficulties that migrants face when entering the labour and housing markets in Naples and more generally in the South of Italy. Due to lower levels of economic growth and higher levels of poverty, the social fabric of these cities is more fragile, there is more competition for low-paid jobs and the urban area is segregated between affluent and disadvantaged districts. In Milan, by contrast, higher levels of growth are associated with a higher demand for labour, including both industry (often situated in the urban periphery) and personal services which are used by Italian households on the basis of proximity. This has created greater opportunities for migrants to settle across the city, reducing competition at the lower end of the labour and housing markets. The results for Naples are in line with recent research on ethnic segregation (Benassi et al., 2020) and highlights the existence of a vicious circle whereby specific patterns of residential segregation are reproduced over time due to the way in which they intersect with social inequalities across various spheres of life (van Ham et al., 2018).
The measure of relative clustering shown in Table 3 sheds light on other aspects of these settlement patterns. In 2011, foreigners were more strongly clustered than Italians in all three urban cores, particularly in Naples (1.09), followed at a considerable distance by Rome (0.16) and Milan (0.05). Over time, the degree of clustering observed in Rome and Milan has further decreased, presumably due to the distribution of foreign citizens across the metropolitan area. However, in the municipality of Naples, the level of clustering of foreign citizens actually increased, indicating a spatial polarisation which brought the RCL index to no less than 1.60 in 2021, compared with -0.01 (no clustering) in Milan. In the other local labour market systems, the opposite process was observed between 2011 and 2021. In both Milan and Rome, there was an increase in clustering in the periphery (from 0.37 to 0.49 in Milan and from 0.21 to 0.24 in Rome), while in Naples this remained stable at approximately 0.11. Once again, a sharp North–South divide (with Rome clearly belonging to the Northern model) is evident not only from the levels but also the internal dynamics of the systems, as is evident from the changes observed over this 10-year period.
It is interesting to consider how broader demographic trends relate to these changes, given the co-existence of demographic decline among Italians and demographic growth among foreign citizens, and the greater geographical mobility of the latter within Italy (Casacchia et al., 2022). The one-group Index of Concentration DEL shows that foreigners continue to be more spatially concentrated than Italian nationals. The difference between the two populations increased between 2011 and 2021, due to an increase in the spatial concentration of foreign citizens, driven most likely by house prices. The index was considerably higher for foreign citizens in the periphery of Milan (reaching 0.595 in 2021), and this was also the case for Naples (0.603 and 0.456 for Italians and foreign nationals respectively). In these two cities, foreign citizens are concentrated in specific areas of the urban periphery, compared with a more even distribution for Italian nationals. In Rome, however, there are smaller differences between the two groups in both the centre and periphery, the main difference being a higher degree of concentration of Italian nationals in the urban core.
The degree of heterogeneity in these spatial patterns is striking, regardless of the level of analysis. The municipalities of Milan and Rome both experienced a decline in spatial concentration and clustering at the local level, while Naples was moving in the opposite direction between 2011 and 2021. Levels of spatial concentration remained more or less stable for Italian nationals in this city, but increased rapidly for foreign citizens as a growing immigrant population was absorbed into a specific set of residential areas which acquired a stronger "ethnic" character.
These contrasts between the cities of the Centre-North and South point to the emergence of distinct models of competition for urban space. In the "centrifugal" model that characterises cities like Milan and Rome, the geographical expansion of the metropolis, together with trends towards suburbanisation involving younger, lower-class households, have generated an ethnically mixed periphery. In the South, where the perimeter of cities like Naples is also expanding, the residential trajectories of foreign and Italian households are quite different. Although rising house prices have encouraged young, lower-class families to move into the periphery, foreign citizens have ended up competing in "centripetal" fashion for residual housing and poor-quality apartments in the more run-down central districts, leading to an increase in spatial concentration and segregation. The residential preferences of the foreign population in Naples are more tightly constrained by their labour market situation, which has made it more difficult for them to access mortgages and private transport, for example, while intense competition for social housing means that this is typically not available to foreign households.
In order to evaluate whether these processes are specific to these three cities or whether they have a wider relevance, we calculated two indicators of local spatial autocorrelation for our output areas across the whole of Italy. Figure 11 show "hotspots" and "coldspots" for the presence of foreigners in 2011 and 2021, using the Getis-Ord Gi* indicator. We used the absolute number of foreign citizens in each output area as the target variable and calculated the spatial weighting matrix using the inverse of distance (Getis & Ord, 2010; Ord & Getis, 2010). In 2021, all of the main hotspots were in Lazio and the Centre-North, primarily along the two main urban corridors mentioned earlier (Milan-Bologna; Milan-Venice) and in the main urban centres. There was a much smaller number of hotspots dotted across the South—for example in areas to the North and South of Naples and in the South of Sicily—and these expanded significantly between 2011 and 2021.
By 2021, the hotspots of the Centre-North had shrunk somewhat, while those in Grosseto, Lazio, Campania, Puglia and Sicily had grown (Figure 11). As we saw in Figure 8, the foreign population spread out across the Centre-North between 2011 and 2021, flowing out from the urban centres and settling in more peripheral areas. At the same time, it was becoming more spatially concentrated in the South, crowding into well-delimited areas not only in the centre of Naples but also in the Agro Pontino (Latina), Castel Volturno, the area around Foggia, the Province of Ragusa in Sicily, and the Metaponto coast, which are areas of low-paid and labour-intensive agricultural production.
Figure 12, based on Local Moran's I Index of Spatial Association (Anselin, 1995) reveals the nature of these distinct settlement patterns even more clearly. High-High and Low–High clusters are present across the Centre-North (with the exception of the more mountainous areas), centred on the metropolitan regions and urban corridors, and extending as far South as the metropolitan area of Rome. In the South, by contrast, the areas where foreigners have settled are almost always segregated in High-Low clusters situated outside the main urban centres, in proximity to important, labour-intensive agricultural and agri-business districts. Between 2011 and 2021, these clusters grew in population and acquired a more segregated ethnic composition, set against the backdrop of a long-term decline in the number of Italian citizens.
The coexistence of contradictory spatial patterns at local level in the North and South of Italy helps to explain the differences we discussed earlier in relation to the segregation indices and illustrates the contrasting settlement models that exist for foreign and Italian citizens. The clarity of the North-South divide in relation to these models is striking and reflects the close correlation that exists between economic growth, demand for labour and the presence of foreign citizens. The Province of Rome appears to have been incorporated within a model that now characterises the whole of the Centre-North, while Latina appears to have been incorporated within the Southern model. The dividing line between North and South thus passes to the South of Rome, before cutting abruptly North to exclude Abruzzo. This sharp dualism has left Liguria, the Marche, and parts of Trentino Alto Adige and Friuli Venezia Giulia in an intermediate position.
It is important to be aware of the limits of this analysis of settlement models and urban residential segregation. Firstly, migrants who have acquired Italian citizenship are not included in the definition of foreign citizens used here, and a considerable number of people made this transition in the period in question (Strozza et al., 2021). According to the Italian National Statistical Institute, between 2001 and 2018 a total of more than one million people of foreign origins (1.33 M) acquired Italian citizenship, which implies more than 70,000 each year. However, these acquisitions were unevenly distributed across the national territory, with a much larger number of people in Lombardy acquiring Italian citizenship (5,195 per annum on average), compared to Lazio (3,588) and Campania (1,342). Moreover, in 2021, the annual national statistics suggest that 133,236 foreigners acquired Italian citizenship, implying an increase over time. As a result, the number of people of foreign origin present in the country in 2011 and particularly in 2021 was probably higher than the number of foreign citizens (4,027,627 and 5,030,466 respectively), and there may be differences in the labour market situation and residential preferences of these two groups.
Secondly, it is important to be aware that the decisions and preferences of foreign citizens is likely to vary depending on their country of origin, social class and other attributes. We know from empirical research carried out in Italy that there are considerable differences between national groups in relation to geographical mobility and settlement models, residential trajectories and labour market situation (Bitonti et al., 2023; Conti et al., 2023). Finally, as we are using cross-sectional datasets that refer to two different moments of time, we have no way of determining to what extent the populations studied actually coincide. This is particularly relevant for foreign citizens, who tend to have much higher rates of geographical mobility, not only at the national level but also internationally, implying that at least part of the population that was present in 2011 may have left the country by 2021.
7 Conclusions
In this article, we provided a brief overview of demographic change at local level in Italy between 2011 and 2021, focusing in particular on changes in the population of foreign citizens and Italian nationals. We used a new output geography which was obtained by selectively aggregating enumeration areas. Using these spatial units, it is possible to provide a detailed account of socio-spatial patterns in relation to demographic trends. The relevance of these output areas goes beyond the present analysis, providing the possibility of avoiding the dilemma described earlier, where researchers are forced to choose between levels of analysis that are either too high (municipality or quarter) or too low (enumeration areas) with respect to the phenomenon that they are studying.
The complexity of the spatial data infrastructure in Italy undoubtedly poses challenges to researchers who wish to use small area data to study social phenomena. Ecological data analysis is less frequently used in Italy compared to Anglo-Saxon countries, presumably due to the difficulties involved and the relative shortage of timely data. However, as we have seen, it is possible to add value to existing census data and new administrative data by applying GIS techniques to existing spatial units. Promising applications of these techniques include studies of social deprivation and of the role of socio-demographic and socio-economic factors in relation to the spread of infectious disease, which has the potential to provide public authorities with additional information for managing epidemics and protecting the population.
The analysis presented here of the residential segregation of foreign citizens in different areas of Italy demonstrates the usefulness of disaggregate analyses of demographic processes. The spatial form that these processes manifest provides additional information that helps us to understand the mechanisms involved. In addition, the detailed local knowledge that small area data provide are of great relevance from a policy-making perspective, enabling local, regional and national authorities to develop programmes and initiatives which reduce the costs associated with social and demographic change.
It would not have been possible to carry out the analysis presented in this paper without using our new set of output areas. In statistical terms, the existing enumeration areas are too heterogeneous and unstable to permit robust estimates to be obtained at local level. Even when calculating simple percentages, the number of enumeration areas with missing values due to divisions by zero is typically very large. This is particularly the case when using longitudinal data, as small variations can generate very large fluctuations in indicators. As many of the existing enumeration areas are very small, they do not facilitate the construction of maps and it is often difficult to identify spatial patterns as a result of their instability and size. By contrast, the new output areas are comparable in size and definition to the spatial units used in other European countries, preparing the ground for comparative analyses in the future.
Thematic maps showing the spatial distribution of demographic and other social characteristics represent a powerful application of small area data, as they are readily interpretable and have the ability to reveal important disparities and patterns of inequality. This is particularly relevant from the perspective of resource allocation, regional planning and in the assessment of policy impacts at local level. For example, it will be essential in coming years to evaluate the impacts of the Piano nazionale di ripresa e resilienza (PNRR) and similar programmes which promote social inclusion, cohesion and sustainable development. Without a clear understanding of the spatial distribution of socio-demographic characteristics, it will arguably be impossible to implement policies to tackle the effects of demographic decline, population ageing and migration.
Data Availability
All data used in this project are freely available from the web site of the Italian Office of National Statistics (ISTAT).
Notes
In the UK, the area with the largest population in 2011 was in Canterbury (4,140 people), involving a tower block. For further details, see:
https://www.ons.gov.uk/peoplepopulationandcommunity/populationandmigration/populationestimates/bulletins/2011censuspopulationandhouseholdestimatesforsmallareasinenglandandwales/2012-11-23 (consulted 26 June 2018).
These include rivers, lakes, lagoons, islands and uninhabited mountain areas, which can assume quite complex shapes. For example, numerous census tracts are used to represent the complex curves of the river Po and its tributaries as they pass through major cities like Turin.
The areas with the largest populations are, in a few cases, statistical artefacts, including parishes which coordinate large voluntary organisations which register thousands of migrants from Africa and Asia as formally resident at their administrative offices, presumably to enable them to access services.
This is only possible, to a limited extent, in the central areas of large cities, where the street plan has remained stable over time and the municipalities tend to make only incremental changes.
Martin was responsible for the construction of the census Output Areas in the UK for the 2001 census. See Ralphs & Ang (2009) for an accessible overview.
A mean population of 1,600 represents a good compromise when dealing with both urban and rural areas in Italy. As mentioned previously, many rural municipalities have small populations (even falling below 1,500), which means that if we wish to respect the boundaries of these administrative units we can only increase the mean population of the output areas by increasing the size of the urban units.
See https://www.istat.it/it/archivio/285267 (consulted 21 August 2023).
This shows the age dependency ratio, which is the ratio of children and elderly people, on the one hand, to those of working age (15–65). A value of 50 indicates that there are 50 "dependents" for every 100 "active adults".
See the methodological note available at:
https://www.istat.it/it/files//2014/12/nota-metodologica_SLL2011_rev20150205.pdf (consulted 18 September 2023).
References
Andreoli, A., Biagetti, M., Casavola, P., & Venanzi, D. (2017). Poverty maps: Analisi territoriale del disagio socio-economico nelle aree urbane. Un esercizio per le 14 Città metropolitane italiane. Dipartimento per le politiche di coesione.
Anselin, L. (1995). Local indicators of spatial association-LISA. Geographical Analysis, 27(2), 93–115. https://doi.org/10.1111/j.1538-4632.1995.tb00338.x
Ascani, A., Faggian, A., Montresor, S., & Palma, A. (2021). Mobility in times of pandemics: Evidence on the spread of COVID19 in Italy’s labour market areas. Structural Change and Economic Dynamics, 58, 444–454. https://doi.org/10.1016/j.strueco.2021.06.016
Barbieri, G. A., Benassi, F., Mantuano, M., & Prisco, M. R. (2018). In search of spatial justice. Towards a conceptual and operative framework for the analysis of inter- and intra-urban inequalities using a geo-demographic approach. The case of Italy. Regional Science Policy and Practice. https://doi.org/10.1111/rsp3.12158
Benassi, D. (2002a). Tra benessere e povertà: Sistemi di welfare e traiettorie di impoverimento a Milano e Napoli. FrancoAngeli.
Benassi, D. (2002b). Un’analisi territoriale del disagio sociale: Una comparazione tra Milano e Napoli. In A. Besussi & L. Leonini (A c. Di), L’Europa tra società e politica. Integrazione europea e nuove cittadinanze (pp. 331–349). Angelo Guerini e associati.
Benassi, F., Bonifazi, C., Heins, F., Lipizzi, F., & Strozza, S. (2020). Comparing residential segregation of migrant populations in selected European urban and metropolitan areas. Spatial Demography. https://doi.org/10.1007/s40980-020-00064-5
Bianchi, G., Di Pede, F., Patruno, E., Reale, A., Ronchi, I., Talice, S., & Esposito, N. (2007). Processi per la definizione di aree di censimento sub comunali da utilizzare per il censimento della popolazione e delle abitazioni del 2011. XXVIII Conferenza Italiana di scienze regionali.
Bitonti, F., Benassi, F., Mazza, A., & Strozza, S. (2023). Framing the residential patterns of Asian communities in three Italian cities: Evidence from Milan, Rome, and Naples. Social Sciences, 12(9), 480. https://doi.org/10.3390/socsci12090480
Bonifazi, C., Heins, F., Licari, F., & Tucci, E. (2021). The regional dynamics of internal migration intensities in Italy. Population, Space and Place, 27(7), e2331. https://doi.org/10.1002/psp.2331
Bressan, M., Radini, M., & Fanfani, D. (2006). La geografia del disagio sociale nelle città italiane. DPS.
Cabras, S., Podda, A., Serci, A., & Sistu, G. (2012). Indice di deprivazione multipla della Sardegna. Regione autonoma della Sardegna. http://www.sardegnastatistiche.it/documenti/12_117_20130130154516.pdf
Cadum, E., Costa, G., Biggeri, A., & Martuzzi, M. (1999). Deprivazione e mortalità: Un indice di deprivazione per l’analisi delle disuguaglianze su base geografica. Epidemiologia e Prevenzione, 23, 175–187.
Caranci, N., Biggeri, A., Grisotto, L., Pacelli, B., Spadea, T., Costa, G., et al. (2010). The Italian deprivation index at census block level: Definition, description and association with general mortality. Epidemiologia e Prevenzione, 34(4), 167–176.
Casacchia, O., Reynaud, C., Strozza, S., & Tucci, E. (2022). Internal migration patterns of foreign citizens in Italy. International Migration, 60(5), 183–197. https://doi.org/10.1111/imig.12946
Cockings, S., & Martin, D. (2005). Zone design for environment and health studies using pre-aggregated data. Social Science & Medicine, 60(12), 2729–2742. https://doi.org/10.1016/j.socscimed.2004.11.005
Conti, C., Mucciardi, M., & Simone, M. (2023). Exploring the settlement models of the main foreign communities residing in Italy (2003–2021). Social Sciences, 12(9), 524. https://doi.org/10.3390/socsci12090524
Costa, G., Cislaghi, C., Zocchetti, C., Agabiti, N., Biggeri, A., Caranci, N., Grisotto, L., Marinacci, C., Petrelli, A., & Spadea, T. (2011). Esistono misure valide dell’effetto della deprivazione socioeconomica sul fabbisogno sanitario? Alcune indicazioni metodologiche per il Servizio sanitario nazionale italiano. Politiche Sanitarie, 12(3), 1–13.
Costa, G., & Marinacci, C. (2007). Health impact of contextual socioeconomic conditions: Different levels of association according to aggregate socioeconomic indicators, modelling strategies and ecological level. In Società Italiana di Statistica (A c. Di), Rischio e Previsione. Atti della Riunione Intermedia. Cleup.
Crescenzi, F. (2002). I sistemi informativi geografici e la qualità del dato statistico. Sesta conferenza nazionale di statistica, Roma.
Duncan, O. D., & Duncan, B. (1955). A methodological analysis of segregation indexes. American Sociological Review, 20(2), 210–217.
Duncan, O. D., Ray, P. C., & Beverly, D. (1961). Statistical geography: Problems in analyzing areal data. The Free Press of Glencoe.
Duque, J. C., Ramos, R., & Suriñach, J. (2007). Supervised regionalization methods: A Survey. International Regional Science Review, 30(3), 195–220. https://doi.org/10.1177/0160017607301605
Flowerdew, R., Manley, D. J., & Sabel, C. E. (2008). Neighbourhood effects on health: Does it matter where you draw the boundaries? Social Science and Medicine, 66(6), 1241–1255. https://doi.org/10.1016/j.socscimed.2007.11.042
Fotheringham, P. (2009). The modifiable areal unit problem (MAUP). In A. Fotheringham & P. Rogerson (Eds.), The SAGE handbook of spatial analysis. SAGE Publications, Ltd. https://doi.org/10.4135/9780857020130.
Gehlke, C. E., & Biehl, K. (1934). Certain effects of grouping upon the size of the correlation coefficient in census tract material. Journal of the American Statistical Association, 29(185A), 169–170.
Getis, A., & Ord, J. K. (2010). The analysis of spatial association by use of distance statistics. Geographical Analysis, 24(3), 189–206. https://doi.org/10.1111/j.1538-4632.1992.tb00261.x
Grady, S. C., & Enander, H. (2009). Geographic analysis of low birthweight and infant mortality in Michigan using automated zoning methodology. International Journal of Health Geographics, 8(1), 10. https://doi.org/10.1186/1476-072X-8-10
Haynes, R., Daras, K., Reading, R., & Jones, A. (2007). Modifiable neighbourhood units, zone design and residents’ perceptions. Health & Place, 13(4), 812–825. https://doi.org/10.1016/j.healthplace.2007.01.002
Haynes, R., Jones, A. P., Reading, R., Daras, K., & Emond, A. (2008). Neighbourhood variations in child accidents and related child and maternal characteristics: Does area definition make a difference? Health & Place, 14(4), 693–701. https://doi.org/10.1016/j.healthplace.2007.11.001
ISTAT. (2015). La nuova geografia dei sistemi locali. ISTAT.
Lelo, K., Monni, S., & Tomassi, F. (2018). Disuguaglianze sociali nelle città italiane. Economia e Politica, 8.
Lemmi, A. (2007). Stima della povertà a livello locale: I casi della Regione Toscana e delle Province di Modena e Trento. https://www.researchgate.net/requests/attachment/7600682
Lillini, R., Quaglia, A., Vercelli, M., & Registro mortalità Regione Liguria. (2012). Costruzione di un indice di deprivazion e lo stato di salute in Liguria. Epidemiologia e Prevenzione, 36(3–4), 180–187.
Marinacci, C., Schifano, P., Cesaroni, G., Caranci, N., Russo, A., & Costa, G. (2009). Magnitude and shape of income inequalities in hospitalization for all causes in Italy. Public Health, 6(3), 225–234.
Martin, D. (1997). From enumeration districts to output areas: Experiments in the automated creation of a census output geography (Working Paper 38; Statistical Commission and Economic Commission for Europe).
Martin, D. (2000). Towards the geographies of the 2001 UK census of population. Transactions of the Institute of British Geographers, 25(3), 321–332. https://doi.org/10.1111/j.0020-2754.2000.00321.x
Martin, D. (2002). Geography for the 2001 Census in England and Wales. Population Trends, 108, 7–15.
Martin, D., Nolan, A., & Tranmer, M. (2001). The application of zone-design methodology in the 2001 UK census. Environment and Planning A, 33(11), 1949–1962. https://doi.org/10.1068/a3497
Massey, D. S., & Denton, N. A. (1988). The dimensions of residential segregation. Social Forces, 67(2), 281–315.
Massey, D.S. & Denton, N.A. (1993). American apartheid: Segregation and the making of the underclass. Harvard University Press.
Mazza, A. (2020). Exploiting fine-scale data in modeling migrants’ settlement patterns in Europe. Spatial Demography, 8, 211–214.
Mazza, A., & Punzo, A. (2015). On the upward bias of the dissimilarity index and its corrections. Sociological Methods and Research, 44(1), 80–107.
Mokhele, T., Mutanga, O., & Ahmed, F. (2016). Development of census output areas with AZTool in South Africa. South African Journal of Science, 112(7/8), 1–7. https://doi.org/10.17159/sajs.2016/20150010
Morlicchio, E., & Pratschke, J. (2004). La dimensione territoriale della povertà a Napoli. In E. Amaturo (A c. Di), Profili di povertà e politiche sociali a Napoli (pp. 1–30). Liguori.
Oberti, M., & Preteceille, E. (2017). La segregazione urbana. Aracne editrice.
Openshaw, S. (1977). A geographical solution to scale and aggregation problems in region-building, partitioning and spatial modelling. Transactions of the Institute of British Geographers, 2(4), 459–472. https://doi.org/10.2307/622300
Openshaw, S., & Rao, L. (1995). Algorithms for reengineering 1991 census geography. Environment and Planning A, 27(3), 425–446. https://doi.org/10.1068/a270425
Ord, J. K., & Getis, A. (2010). Local spatial autocorrelation statistics: Distributional issues and an application. Geographical Analysis, 27(4), 286–306. https://doi.org/10.1111/j.1538-4632.1995.tb00912.x
Pasetto, R., Sampaolo, L., & Pirastu, R. (2010). Measures of material and social circumstances to adjust for deprivation in small-area studies of environment and health: Review and perspectives. Annali dell’Istituto Superiore di Sanità, 46(2), 185–197.
Piekut, A., Pryce, G., & van Gent, W. (2019). Segregation in the twenty frst century: Processes, complexities and future directions. Tijdschrift voor Economische en Sociale Geografie, 110, 225–234.
Pratschke, J. (2007). L’articolazione territoriale dello svantaggio sociale in Italia: Una nuova misura multidimensionale per piccole aree geografiche. In A. Brandolini & C. Saraceno (A c. Di), Povertà e benessere. Una geografia delle disuguaglianze in Italia (pp. 123–146). Il Mulino.
Ralphs, M., & Ang, L. (2009). Optimised geographies for data reporting: Zone design tools for census output geographies (Statistics New Zealand Working Paper 09–01). Statistics New Zealand.
Rao, J. (1999). Some recent advances in model-based small area estimation. Survey Methodology, 25(2), 175–186.
Schifano, P., Marinacci, C., Cesaroni, G., Belleudi, V., Caranci, N., Russo, A., & Perucci, C. A. (2009). Temporal and geographic heterogeneity of the association between socioeconomic position and hospitalisation in Italy: An income based indicator. International Journal for Equity in Health, 8, 33.
Stillwell, J., Daras, K., & Bell, M. (2018). Spatial aggregation methods for investigating the MAUP effects in migration analysis. Applied Spatial Analysis and Policy, 11, 693–711. https://doi.org/10.1007/s12061-018-9274-6
Strozza, S., Conti, C., & Tucci, E. (2021). Nuovi cittadini. Diventare italiani nellera della globalizzazione. Il Mulino.
Succi, R. (2016, ottobre 6). Indicatori sintetici di deprivazione: Una proposta per la Liguria. ISTAT: Diseguaglianze sociali, condizioni di salute e accesso alle cure, Genova.
Townsend, I., & Walker, R. (2002). The structure of income residential segregation in Canadian metropolitan areas. Canadian Journal of Regional Science, 25(1), 25–52.
Valerio, M., & Vitullo, F. (2000). Sperimentazione di un indice di svantaggio socioeconomico in Basilicata. Epidemiologia e Prevenzione, 25, 219–223.
van Ham, M., Tammaru, T., & Janssen, H. (2018). A multi-level model of vicious circles of socio-economic segregation. In OECD, Divided cities (pp. 135–153). OECD. https://doi.org/10.1787/9789264300385-8-en
Verhoef, H., & Van Eeden, A. (2015). Identifying the challenges of creating an optimal dissemination geography for census. South African Journal of Geomatics, 4(1). https://doi.org/10.4314/sajg.v4i1.4
Vickers, D., & Rees, P. (2007). Creating the UK national statistics 2001 output area classification. Journal of the Royal Statistical Society Series A: Statistics in Society, 170(2), 379–403. https://doi.org/10.1111/j.1467-985X.2007.00466.x
White, M. J. (1986). Segregation and diversity measures in population distribution. Population Index, 52(2), 198. https://doi.org/10.2307/3644339
Wong, D. W. (2003). Spatial decomposition of segregation indices: A framework toward measuring segregation at multiple levels. Geographical Analysis, 35(3), 179–194.
Xie, M. (2010). The effects of multiple dimensions of residential segregation on Black and Hispanic homicide victimization. Journal of Quantitative Criminology, 26, 237–268.
Yang, T. C., Zhao, Y., & Song, Q. (2017). Residential segregation and racial disparities in self-rated health: How do dimensions of residential segregation matter? Social Science Research, 61, 29–42.
Zhao, J., & Exeter, D. J. (2016). Developing intermediate zones for analysing the social geography of Auckland, New Zealand: Intermediate geographies for Auckland. New Zealand Geographer, 72(1), 14–27. https://doi.org/10.1111/nzg.12110
Funding
Open access funding provided by Università degli Studi di Napoli Federico II within the CRUI-CARE Agreement. This paper was conceived and realised as part of the European Union – NextGenerationEU programme [PE00000018, CUP E63C2200214007] GRINS – Growing Resilient, INclusive and Sustainable) and the PRIN-PNRR research project “Foreign population and territory: Integration processes, demographic imbalances, challenges and opportunities for the social and economic sustainability of the different local contexts (For.Pop.Ter)” [P2022 WNLM7], funded by the European Union. The views and opinions expressed are those of the authors and do not necessarily reflect those of the European Union or the European Commission, who cannot be held responsible for them.
Author information
Authors and Affiliations
Corresponding author
Ethics declarations
Conflict of interest
Not applicable.
Additional information
Publisher's Note
Springer Nature remains neutral with regard to jurisdictional claims in published maps and institutional affiliations.
Rights and permissions
Open Access This article is licensed under a Creative Commons Attribution 4.0 International License, which permits use, sharing, adaptation, distribution and reproduction in any medium or format, as long as you give appropriate credit to the original author(s) and the source, provide a link to the Creative Commons licence, and indicate if changes were made. The images or other third party material in this article are included in the article's Creative Commons licence, unless indicated otherwise in a credit line to the material. If material is not included in the article's Creative Commons licence and your intended use is not permitted by statutory regulation or exceeds the permitted use, you will need to obtain permission directly from the copyright holder. To view a copy of this licence, visit http://creativecommons.org/licenses/by/4.0/.
About this article
Cite this article
Pratschke, J., Benassi, F. Population Change and Residential Segregation in Italian Small Areas, 2011–2021: An Analysis With New Spatial Units. Spat Demogr 12, 3 (2024). https://doi.org/10.1007/s40980-024-00124-0
Accepted:
Published:
DOI: https://doi.org/10.1007/s40980-024-00124-0