Abstract
A novel defect-based fatigue model for the prediction of S–N (stress versus number of cycles) data points and curves is proposed in this paper. The model is capable of predicting the material fatigue performance based on defect size and location from the surface. A defect factor was introduced and obtained based on notch theory, which considers the notch sensitivity of the material as well as the stress concentration obtained using the finite element method. A newly developed equation was applied to represent the relationship between the defect factor, defect size and defect location from the surface. AlSi10Mg samples were manufactured using laser powder bed fusion, and then machined. The samples were tested under rotational bending cyclic loading until failure. The failed samples were analysed using scanning electron microscopy and it was found that cracks initiated from defects located at the surface. The measured defect size and location were used to predict the number of cycles for an applied stress using the proposed defect-based fatigue model. This model was validated by comparing the predicted and experimentally obtained S–N data. The proposed model has the potential to be applied to component-level fatigue assessment and integrated into industrial quality assurance workflows. For instance, defects can be measured for each produced industrial component and directly assessed against fatigue performance using the developed defect-based fatigue model. This could enable the rapid approval and certification of future additively manufactured industrial components, which can unleash the commercial potential of additive manufacturing for light-weight multi-functional component designs.
Similar content being viewed by others
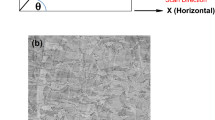
Avoid common mistakes on your manuscript.
1 Introduction
Additive manufacturing (AM) technologies, in particular laser powder bed fusion (LPBF), have been widely used to deliver designs with complex geometries enabling light-weighting and more functionality. However, besides the potential of AM to produce complex parts for industry, the formation of defects during the process introduces challenges [1]. Regardless of the current existence of advanced AM technologies with optimised AM process parameters, the occurrence of defects is almost unavoidable and it can lead to failure for AM manufactured parts, especially in applications subject to cyclic loads and fatigue [2,3,4]. The generation of defects can be due to lack of fusion, delamination, trapped gasses and unmelted particles [5]. AM machine variability (i.e., interactions between the beam source and the material, and geometry) has resulted in inconsistent microstructures as well as defects [6]. In this regard, the AM defect characteristics such as size, location and morphology are dependent on the process parameters (i.e., input energy, powder characteristics, scanning strategy, scanning speed, hatch spacing, layer thickness and build direction).
Brandao et al. [7] evaluated AlSi10Mg samples processed by LPBF using X-ray tomography and found pores in the range of 0.02%-0.77% of the volume which are highly dependent on the process parameters. Tang and Pistorius [8] reported that the building direction and differences in hatch distance resulted in different pore sizes and shapes which affected the fatigue performance for LPBF AlSi10Mg samples. Larrosa et al. [9] used computer tomography (CT) to conclude that pores were the trigger for crack initiation. Findings from Zhao et al. [10] showed that fatigue cracks originated from external or subsurface circular gas openings and the fatigue lifespan of LPBF samples depend on the direction of the build, and those built in the vertical direction showed a lower fatigue strength. Romano et al. [11] used X-ray micro-CT data to predict the fatigue limits of components. A study by Andreau et al. [12] highlighted the significance of near-surface pores as initiators of critical cracks. Benedetti et al. [13] indicated that pores nearer the surface (in the outermost 400 μm impenetrable layer) steered the commencement of cracks. Shrestha et al. [14] also reported crack initiation from near-surface pores. For AlSi10Mg processed with LPBF, Raja et al. [15] reported, based on their conducted literature review, that fatigue cracks mainly initiate from pores as well as inclusions and surface roughness of large depths (for as-build and non-machined surfaces).
One of the key research questions is how to relate defects to fatigue life. Different approaches have been researched to find the relationship between defects, applied stress and number of cycles to failure. Finite element analyses (FEA) have been employed to understand the effect of defects on the stress field and potential fatigue performance [16, 17]. The FEA is capable of predicting the local stress for a modelled defect. However, the predicted local stress field needs to be related to the number of cycles to failure. One of the applied approaches is the fracture mechanics theory, where the stress intensity is related to a number of cycles for an applied load [18]. This method requires further testing to obtain the fracture mechanics properties. Another approach is the use of machine learning methods (e.g., artificial neural network) which can be employed to find the relationship between defect characterisation, applied stress and number of cycles [19]. This approach would require a large set of data to train the model.
Despite the existing research in understanding the impact of defects on the fatigue performance, there is a lack of understanding of how to relate fatigue strength, number of cycles and defect characteristics, in particular defect size and location. The novelty of this paper is the development of a fatigue model capable of predicting S–N data points and curves based on the size and location of defects. The fatigue model is validated for machined AlSi10Mg samples produced by LPBF in the vertical direction.
2 Research methods
2.1 Experimental procedures
AlSi10Mg samples were manufactured on the LPBF EOS M290 machine. The samples were built in the vertical direction using a layer thickness of 60 µm, a laser power of 400 W, a laser scanning speed of 1 m/s, hatch spacing of 0.2 mm, laser beam diameter of 0.1 mm and preheated baseplate to 35℃. EOS Aluminium AlSi10Mg powder with partials size in the range of 25–70 µm was used. The powder is compliant with DIN EN 1706 (EN AC—43,000). No heat treatment was applied to the samples after printing. The samples were designed and manufactured with a stock allowance (extra material) of 0.25 mm. The extra material was machined using turning to produce the desirable dimensions, including a minimum diameter of 4 ± 0.05 mm. The turning was conducted by a form tool that matched the required radius of the sample. The surface roughness after machining was measured to be Ra = 0.51 ± 0.23 µm.
All fatigue samples were tested on a rotational bending machine with a stress ratio (ratio of minimum to maximum fatigue stress) of R = − 1 at a frequency of 40 Hz. After the samples were fractured, the surfaces were analysed using scanning electron microscopy (SEM) to identify the location of the crack initiation as well as the size of the defect. The JSM-7100F LV SEM (JEOL UK) was operated in secondary electron imaging mode at an accelerating voltage of 15 kV. The working distance used was 10–15 mm depending on the field of view required. All fractured samples were examined without coating or pre-treatment.
2.2 Defect-based fatigue model
The fatigue model for prediction of S–N curves presented by Serjouei and Afazov [20] was used in this study as the basis for understanding the concept of the defect factor using the endurance limit. The authors used this model to identify the defect factor for a stainless steel 316 produced by LPBF with various post-processing conditions. In this study, the model is further advanced to consider the defect size and location using the defect factor. The model considers the stress ratio (mean stress effect based on the Goodman’s approach) and predicts the fatigue stress range at 103 and 106 cycles by:
where the fatigue stress range (\({\sigma }_{r}^{f}\)) is expressed as a function of the ultimate tensile strength (\({\sigma }_{u}\)) defect factor (\(d\)) and stress ratio (R). The ultimate tensile strength of AlSi10Mg processed on the EOS 290 M using a layer of 60 µm is 440 MPa [21]. For comparison, AISi10Mg samples produced by LPBF without any applied heat treatment is in the range of 400 – 465 MPa [22, 23]. Based on the prediction of two points of the S–N curve, the relationship between the fatigue stress range, \({\sigma }_{r}^{f}\), and the number of cycles to failure, \({N}_{f}\), is obtained by fitting using:
where \(A\) and \(n\) are material constants obtained after fitting.
The defect factor, \(d\), was obtained empirically by Serjouei and Afazov [20] by fitting the defect factor to fatigue data to create a S–N curve representing the lower band of the data, which can be considered as a conservative approach from design perspective. In this study, the goal is to find a relationship between the defect factor and defect characteristics (size and location). This is done using the concept of fatigue stress concentration factor. The relationship between defect factor (\(d\)) and fatigue stress concentration factor (\({k}_{f}\)) is given by [24]:
The fatigue stress concentration factor is a function of the static stress concentration factor (\({k}_{scf}\)) which depends on the geometry and loading, as well as the notch sensitivity (\(q\)). The relationship is given by [24]:
The static stress concentration factor is obtained using FEA described in Sect. 2.3. The notch sensitivity factor is a function of the notch diameter [25]:
where \(D\) is the diameter of the notch and \(a\) is a material constant. The material constant a is dependent on the ultimate tensile strength for steel as reported in [24]. The increase of the ultimate strength in steels could be associated with reduction of ductility (elongation), hence increasing the brittleness. In this study, the material constant a is obtained based on published notch sensitivity data for aluminium 2024-T6 (see Fig. 1). The ultimate tensile strength of this aluminium series is 427 MPa, which is comparable with 440 MPa for AlSi10Mg. Also, the elongation for 2024-T6 is approximately 5%, which is comparable with approximately 6% for AlSi10Mg [21]. Figure 1 shows the fitted Eq. 6 with \(a\) = 0.75. It needs to be noted that the notch diameter represents the dimeter of a defect with a spherical shape.
2.3 Finite element model
A plane stress 2D finite element model is created in ANSYS to represent different sizes and locations of a spherical defect. The maximum stress is in the direction of the applied load; therefore, a 2D plane stress model is sufficient to capture the maximum stress needed to obtain the stress concentration factor. The modelled internal defect is shown in Fig. 2. Quadratic 8-node elements were used to generate the mesh. The edge of the modelled defect was split into small partitions of 1 µm for all modelled defect sizes and locations. This created a refined mesh around the modelled defect (notch). A Young’s modulus of 71 GPa and Poisson’s ratio of 0.3 were assigned in an elastic material model. A nominal stress of 1 MPa was applied in the form of a pressure in the opposite direction. Boundary conditions were applied to represent a quarter of a model as depicted in Fig. 2. The zero displacements in the y direction are applied to represent a symmetry of a defect. The zero displacements in the x direction are applied to avoid rigid body motion. Also, this boundary condition is applied far from the defect to enable realistic prediction of the maximum stress. Static structural analyses were performed to predict the maximum principal stresses. The stress concentration was found as the ratio between the predicted maximum principal stress and the applied nominal stress of 1 MPa. The defects were modelled with diameters (D) of 50 µm, 125 µm, 250 µm and 500 µm at distances from the surface (L) in the range of 25–400 µm.
3 Results and discussion
Following the experimental procedures from Sect. 2.1, the results from the fatigue testing (R = − 1) of the machined AlSi10Mg samples produced by LPBF in the vertical direction are shown in Fig. 3. The results are compared to experimental test data of machined samples for the same stress ratio (R = − 1). It can be seen that the produced data in this study aligns with the trends from the literature. A key point from the scattered data in Fig. 3 is that the fatigue strength of the AlSi10Mg is greatly dependant on the presence of defects generated during LPBF using different machines with various process parameters and post-process conditions.
The broken samples were analysed using SEM. The analyses included the following steps: (i) identification of the location of the crack initiation; (ii) identification of the defect that has caused the crack initiation; (iii) measurements of the defect size and its location from the surface. Figure 4 shows a SEM micrograph for one of the samples where the crack initiated from a defect (a pore) located at the surface. The pore can be assumed to have a “spherical” shape with a diameter of 142 µm. It was also observed for the remaining test samples that the critical cracks initiated from a pore with a “spherical” shape located at the surface. The diameters of all pores that initiated the cracks were measured to be in the range of 75–142 µm. In comparison, Romano et al. [31] reported measured defects in the range 25–500 µm for AlSi10Mg processes by LPBF.
To correlate defect size and location to fatigue strength and number of cycles, the results from the finite element model are first analysed. Figure 5 shows the stress concentrations predicted with the finite element model for modelled spherical pores with different diameters and locations from the surface. The stress concentration increases by decreasing the distance to the surface (L). The location of the highest stress consecration is at the side close to the surface. The observed trend reveals that the stress concentration increases exponentially by decreasing L. The main purpose of the predicted stress concentrations was to obtain the defect factors for each of the modelled pores. Figure 6 shows the calculated defect factors based on the stress concentrations obtained from the finite element model. The general trend is that the defect factor decreases by increasing the diameter of the defect. Also, the closer a defect is to the surface, the lower the defect factor is.
Calculated and represented defect factors with Eq. 7
Based on the calculated defect factor, a new equation (see Eq. 7) is proposed to provide the relationship between the defect factor (d), the defect size (D) and location from the surface (L):
Figure 6 shows the capability of the equation to representatively fit the calculated defect factors. It should be noted that for D = 0 and L = 0, the equation will produce a mathematical error. Therefore, a small negligible value such as 10–7 mm should be added to D and L (units in mm). Also, Eq. 7 would predict values for the defect factor (d) greater than 1 for small sized defects located in the sub-surface (e.g., defects smaller than 25 µm at a distance from the surface greater than 250 µm). In those cases, the values should be truncated to 1.
To validate the proposed model, the measurements for the defect size from the SEM are used as an input into Eq. 7. As discussed, the critical cracks initiated at the surface for the eight tested samples, thereby it is used that L = 0. After the defect factor is calculated for each defect that initiated a crack, the S–N curves were created for each defect. Based on the created S–N curves, the number of cycles were predicted for the applied nominal stress during the rotational bending testing. Figure 7 shows the predicted and experimentally obtained data points. The proposed model is in close correlation with the experimentally obtained data points; hence, it could be applied in fatigue life assessment workflows. It needs to be pointed out that the validation is for defects at the surface. There might have been defects with a greater defect factor within the tested samples, but cracks did not initiate at those defects during the rotational bending testing because the stress within the material is lower than the stress at the surface. For instance, a very large defect at the centre of the sample might have the greatest defect factor, but a crack would not initiate in rotational bending at that defect because the stress would be very low (zero at the absolute centre from the bending theory). Uniaxial fatigue testing can provide uniform distribution of the applied nominal stress across the cross section of the samples enabling the initiation of cracks at defects in the bulk of the material for cases where the defect factors are greater than those at the surface.
The advantage of the proposed model is that it can be directly implemented in existing industrial workflows for fatigue assessment. For instance, all methods for stress analyses (e.g., finite element analyses) of fatigue load cases do not need to be changed because there is no need to model the defect into the geometry. The defect can be characterised by CT scanning (size and location) and the defect factor can be directly calculated to create an S–N curve for each defect. Knowing the position of the defect relative to the geometry and the predicted stress field (e.g., from finite element analyses), the number of cycles or the fatigue damage (e.g., Miner’s rule) can be calculated. This fatigue assessment approach has the potential to be applied to other AM technologies as well as welding and casting where pores are also generated.
Another advantage of the proposed model is that it can consider the mean stress effect using the stress ratio (R). This is particularly relevant for industry applications where materials are subject to transient loads and the stress ranges can undergo tension-tension, tension–compression or compression-compression loading conditions. This means that the material will experience different mean stress (R ratio) for each loading and unloading. As the proposed model is capable of capturing the mean stress effect, it can be employed into established fatigue assessment methodologies (e.g., fatigue damage calculations incorporating rainflow algorithms [32]). To understand the effect of the R ratio on the fatigue properties, Fig. 8 shows predicted S–N curves for R ratio values representing tensile mean stress (R = 0.5 and R = 0.1), zero mean stress (R = − 1), and compressive mean stress (R = − 5 and R = 10) effects for a given surface defect of 125 µm using the proposed defect-based fatigue model. It should be noted that the compressive mean stress at R = − 5 and R = 10 represents compression-tension and compression-compression loading conditions, respectively. The predicted S–N curves clearly show the expected trend that the compressive mean stress increases the fatigue performance in contrast to the tensile mean stress. The predicted S–N data at R = 0.1 are comparable to the S–N data tested for AlSi10Mg samples and “wishbone” components of comparable defect sizes [33]. Figure 9 shows the impact of the R ratio on the predicted fatigue stress range at 107 cycles for different sizes of a surface defect. The predicted results show that the R ratio has a greater impact on the fatigue performance for small in size surface defects. This indicates that for small in size surface defects (less than 50 µm) subject to compressive mean stress, the material can withstand greater stress ranges. The same is valid for internal defects because the defect factor is greater in the bulk of the material (see Fig. 6).
The current model assumes that a crack is initiated in a single defect. In AM, the porosity is randomly distributed, and multiple scenarios can be present. For instance, the largest defect factor for a single defect could be greater if surrounded with other defects, or if a group of defects are close to each other. The presented model can address those effects by obtaining the stress concentration for different scenarios and predict the defect factor and the S–N curves.
4 Conclusions
A novel defect-based fatigue model was developed and validated against fatigue data obtained in rotational bending testing of machined AlSi10Mg samples produced by laser powder bed fusion. The model showed that it is capable of generating an S–N curve for a defect based on its size and location from the surface. This can enable the detailed life prediction of industrial components based on identified defect size and location (e.g., through targeted X-ray computer tomography) and their incorporation in the developed defect-based fatigue model.
The model can be applied to other additive manufacturing materials. This would require identifying the notch sensitivity of the material. It is a general rule that brittle materials are more sensitive to defects (notches) which could be a topic of further research in additive manufacturing.
To gain further confidence for the proposed defect-based fatigue model, further validations are planned in the future. This includes validations at different stress ratio using uniaxial testing as well as manufacturing seeded defects in the samples to gain further confidence for the predictions at the bulk of the material. The defect aspect ratio could be researched and potentially incorporated into the defect-based fatigue model. The long-term vision is to integrate the proposed defect-based fatigue model into industrial workflows for life assessment of components subject to multi-axial loads as well as to enable rapid certification of designs using holistic digital tools.
Data availability
The authors confirm that the data supporting the findings of this study are available within the article.
References
Afazov S, Roberts A, Wright L, Jadhav P, Holloway A, Basoalto H, Milne K, Brierley N (2022) Metal powder bed fusion process chains: an overview of modelling techniques. Prog Additive Manuf 7:289–314
Ngo TD, Kashani A, Imbalzano G, Nguyen KT, Hui D (2018) Additive manufacturing (3D printing): A review of materials, methods, applications, and challenges. Compos B Eng 143:172–196
Concli F, Fraccaroli L, Nalli F, Cortese L (2022) High and low-cycle-fatigue properties of 17–4 PH manufactured via selective laser melting in as-built, machined and hipped conditions. Prog Add Manuf 7:99–109
Fatemi A, Molaei R, Simsiriwong J, Sanaei N (2019) Fatigue Behavior of Additive Manufactured Materials: An Overview of Some Recent Experimental Studies on Ti-6Al-4V Considering Various Processing and Loading Direction Effects. Fatigue Fract Eng Mater Struct 42:991–1009
Sanaei N, Fatemi A (2021) Progress in Materials Science Defects in additive manufactured metals and their effect on fatigue performance: A state-of-the-art review. Prog Mater Sci 117:100724
Yang H, Rao P, Simpson T, Lu Y, Witherell P, Nassar AR, Reutzel E, Kumara S (2021) Six-Sigma Quality Management of Additive Manufacturing. Proc IEEE 109(4):347–376
Brandao AD, Gumpinger J, Gschweitl M, Seyfert C, Hofbauer P, Ghidini T (2017) Fatigue properties of additively manufactured AlSi10Mg–surface treatment effect. Procedia Struct Integ 7:58–66
Tang M, Pistorius PC (2019) Fatigue life prediction for AlSi10Mg components produced by selective laser melting. Int J Fatigue 125:479–490
Larrosa NO, Wang W, Read N, Loretto MH, Evans C, Carr J, Withers PJ (2018) Linking microstructure and processing defects to mechanical properties of selectively laser melted AlSi10Mg alloy. Theoret Appl Fract Mech 98:123–133
Zhao J, Easton M, Qian M, Leary M, Brandt M (2018) Effect of building direction on porosity and fatigue life of selective laser melted AlSi12Mg alloy. Mater Sci Eng, A 729:76–85
Romano S, Brandão AD, Gumpinger J, Gschweitl M, Beretta S (2017) Qualification of AM parts: Extreme value statistics applied to tomographic measurements. Mater Des 131:32–48
Andreau O, Pessard E, Koutiri I, Penot JD, Dupuy C, Saintier N, Peyre P (2019) A competition between the contour and hatching zones on the high cycle fatigue behaviour of a 316L stainless steel: Analyzed using X-ray computed tomography. Mater Sci Eng, A 757:146–159
Benedetti M, Fontanari V, Bandini M, Zanini F, Carmignato S (2018) Low-and high-cycle fatigue resistance of Ti-6Al-4V ELI additively manufactured via selective laser melting: Mean stress and defect sensitivity. Int J Fatigue 107:96–109
Shrestha R, Simsiriwong J, Shamsaei N (2019) Fatigue behavior of additive manufactured 316L stainless steel parts: Effects of layer orientation and surface roughness. Addit Manuf 28:23–38
Raja A, Cheethirala SR, Gupta P, Vasa NJ, Jayaganthan R (2022) A review on the fatigue behaviour of AlSi10Mg alloy fabricated using laser powder bed fusion technique. J Market Res 17:1013–1029
Bruna-rosso C, Demir AG, Previtali B (2018) Selective laser melting finite element modeling: Validation with high-speed imaging and lack of fusion defects prediction. Mater Des 156:143–153
Echeta I, Dutton B, Leach RK, Piano S (2021) Finite element modeling of defects in additively manufactured strut-based lattice structures. Addit Manuf 47:102301
Becker TH, Kumar P, Ramamurty U (2021) Fracture and fatigue in additively manufactured metals. Acta Mater 219:117240
Peng X, Wu S, Qian W, Bao J, Hu Y, Zhan Z, Guo G, Wither P (2022) The potency of defects on fatigue of additively manufactured metals. Int J Mech Sci 221:107185
Serjouei A, Afazov S (2022) Predictive model to design for high cycle fatigue of stainless steels produced by metal additive manufacturing. Heliyon 8(11):e11473. https://doi.org/10.1016/j.heliyon.2022.e11473
EOS GmbH® Electro Optical Systems. Material data sheet. EOS Aluminium AlSi10Mg. https://www.eos.info/en/industrial-3d-printing/additive-manufacturing-how-it-works/dmls-metal-3d-printing. Accessed Dec 2022
Tridello A, Fiocchi J, Biffi C, Chiandussi G, Rossetto M, Tuissi A, Paolino D (2020) Effect of microstructure, residual stresses and building orientation on the fatigue response up to 109 cycles of an SLM AlSi10Mg alloy. Int J Fatigue 137:105659
Qian G, Li Y, Paolino D, Tridello A, Berto F, Hong Y (2020) Very-high-cycle fatigue behavior of Ti-6Al-4V manufactured by selective laser melting: Effect of build orientation. Int J Fatigue 136:105628
Sines G, Waisman J (1959) Metal fatigue. McGraw-Hill Book Company, Inc., New York
Peterson RE (1945) Relation between Life Testing and Conventional Tests of Materials. ASTM Bull. 13:213
Romano S, Brückner-Foit A, Brandão A, Gumpinger J, Ghidini T, Beretta S (2018) Fatigue properties of AlSi10Mg obtained by additive manufacturing: Defect-based modelling and prediction of fatigue strength. Eng Fract Mech 187:165–189
Romano S, Patriarca L, Foletti S, Beretta S (2018) LCF behaviour and a comprehensive life prediction model for AlSi10Mg obtained by SLM. Int J Fatigue 117:47–62
Nadot Y, Nadot-Martin C, Kan W, Boufadene S, Foley M, Cairney J, Proust G, Ridosz L (2020) Predicting the fatigue life of an AlSi10Mg alloy manufactured via laser powder bed fusion by using data from computed tomography. Addit Manuf 32:100899
Uzan N, Ramati S, Shneck R, Frage N, Yeheskel O (2018) On the effect of shot-peening on fatigue resistance of AlSi10Mg specimens fabricated by additive manufacturing using selective laser melting (AM-SLM). Addit Manuf 21:458–464
Schneller W, Leitner M, Springer S, Grün F, Taschauer M (2019) Effect of HIP Treatment on Microstructure and Fatigue Strength of Selectively Laser Melted AlSi10Mg. J Manuf Mater Process 3(1):16
Romano S, Abel A, Gumpinger J, Brandão J, Beretta S (2019) Quality control of AlSi10Mg produced by SLM: Metallography versus CT scans for critical defect size assessment. Addit Manuf 28:394–405
Marsh G, Wignall C, Thies PR, Barltrop N, Incecik A, Venugopal V, Johanning L (2016) Review and Application of Rainflow Residue Processing Techniques for Accurate Fatigue Damage Estimation. Int J Fatigue 82:757–765
Beretta S, Patriarca L, Gargourimotlagh M, Hardaker A, Brackett D, Salimian M, Gumpinger J, Ghidinic T (2022) A benchmark activity on the fatigue life assessment of AlSi10Mg components manufactured by L-PBF. Mater Des 218:110713
Author information
Authors and Affiliations
Corresponding authors
Additional information
Publisher's Note
Springer Nature remains neutral with regard to jurisdictional claims in published maps and institutional affiliations.
Rights and permissions
Open Access This article is licensed under a Creative Commons Attribution 4.0 International License, which permits use, sharing, adaptation, distribution and reproduction in any medium or format, as long as you give appropriate credit to the original author(s) and the source, provide a link to the Creative Commons licence, and indicate if changes were made. The images or other third party material in this article are included in the article's Creative Commons licence, unless indicated otherwise in a credit line to the material. If material is not included in the article's Creative Commons licence and your intended use is not permitted by statutory regulation or exceeds the permitted use, you will need to obtain permission directly from the copyright holder. To view a copy of this licence, visit http://creativecommons.org/licenses/by/4.0/.
About this article
Cite this article
Afazov, S., Serjouei, A., Hickman, G.J. et al. Defect-based fatigue model for additive manufacturing. Prog Addit Manuf 8, 1059–1066 (2023). https://doi.org/10.1007/s40964-022-00376-6
Received:
Accepted:
Published:
Issue Date:
DOI: https://doi.org/10.1007/s40964-022-00376-6