Abstract
The present study investigates the convergence process of per-worker output for a panel of 15 countries in the Middle East and North African (MENA) region over the period 1970–2016. Further, the study discusses the dynamic impact of various productive factors (physical capital, human capital and total factor productivity) on per-worker output. In order to estimate the long-run relationship, the paper employs various dynamic panel autoregressive distributed lag (ARDL) models. The econometric approach controls for cointegration, nonstationarity, heterogeneity and cross-sectional dependence in the variables and residuals. The results suggest the presence of cross-sectional dependence and mixed order of integration of the variables. The statistical results of Pedroni and Westerlund cointegration tests indicate that per-worker output, physical capital stock, human capital stock and total factor productivity tend to have a long-run relationship. The four-step methodology adopted in the present study consistently accepts the conditional β-convergence towards steady state of output per worker. Besides, the paper finds a positive impact of physical capital and total factor productivity on long-run economic growth.
Similar content being viewed by others
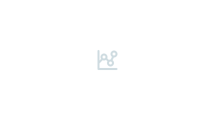
Notes
Galor (1996) has pointed out the three-fold classification of convergence: absolute convergence, conditional convergence and club convergence. The hypothesis that poor countries grow faster than rich ones and eventually every country convergence to the same steady-state irrespective of their structural characteristics is known as absolute convergence. The concept of conditional convergence implies that countries will converge to their unique steady states conditional on fundamental parameters such as population growth rate, savings rate, resource endowment and institutions etc. Club convergence allows a distinct homogenous cluster of countries to approach same per-capita output levels in the long run (Durlauf and Johnson, 1995).
Absolute (unconditional β) and conditional β-convergence examines whether or not convergence occurs after conditioning for a vector of country-specific factors which in turn determines cross-section differences in long-run output levels.
Neoclassical equation for convergence relies on the assumption of identical technologies across the countries (homogeneity assumption). Omitted variable bias, heterogeneity and endogeneity problems represent serious limitations in neoclassical convergence equations (Islam, 1995).
Carlino and Mills (1993) proposed the notion of stochastic convergence which implies that the shocks to the \(D{y}_{it}\) series are temporary; thus, the series \({y}_{it}\) approaches to long-run equilibrium, \(\overline{y_{it}}\). Li and Papell (1999) proposed the notion of deterministic convergence which reflects that \(D{y}_{it}\) is mean stationary. It implies that \({y}_{it}\) will move parallel to \(\overline{y_{it}}\) over the long run..
For the purpose of the present paper, the MENA region consists of the following 19 countries unless stated otherwise: Algeria, Oman, United Arab Emirates, Qatar, Bahrain, Kuwait, Saudi Arabia, Turkey, Iraq, Iran, Syria, Morocco, Lebanon, Palestine, Jordan, Egypt, Libya, Tunisia and Yemen. Israel has been eliminated from the sample of countries as it follows a different economic environment.
Algeria, Bahrain, Kuwait, Oman, Qatar, Saudi Arabia, United Arab Emirates, Iran, Iraq, Turkey, Tunisia, Egypt, Jordan, Morocco and Syria. The other remaining countries of the region are not included in the analysis due to the lack of relevant data.
This discussion is based on Ditzen (2018).
One of the crucial elements in panel unit root tests is the degree of parameter heterogeneity in the Dickey-Fuller regressions across countries. It means that the time series properties of the panel may differ across countries. In other words, all countries may not follow the same unit root process. Accordingly, formulation of null and alternative hypothesis of various panel unit root tests becomes quit complex. In these situations one draws inferences about the integration levels of variables on the basis of predominating evidence about order of integration across countries.
When the assumption of pooling or that the slope coefficients are same across the panel does not hold true, the panel is referred to as heterogeneous panel. An immediate advantage of introducing heterogeneity is to capture the effect of different development levels, forms of government, macroeconomic policies, custom and culture etc.
For first-generation unit root testing, refer to Appendix 3, Tables 7 and 8.
Refer to Table 10 in Appendix 3 for country-specific parameters and speed of convergence.
These results are not surprising, since empirical research, in particular that of Islam (1995), has shown that unlike the cross-sectional estimates, human capital ceases to have a significant impact on economic growth once the temporal dimension is taken into consideration.
Maddala and Wu (1999) employ probability values on t-statistic for the estimate of (\({\rho }_{i}-1\)) in country-specific ADF regression and construct Fisher statistic such as \(-2\sum (\mathrm{log}({p}_{i}))\). It is distributed as standard chi-square with 2 N degrees of freedom. To deal with cross-section dependency problem, Pesaran (2007)’s test captures cross-section dependence in the form of a single unobserved common factor. In practice, Pesaran (2007) adds cross-section means of dependent and independent variables (with lags of dependent variables) to country-specific ADF regressions as
$$\Delta y_{it}=\mu_i+\beta_it+\left(\rho_i-1\right)y_{i,t-1}+\omega_{1i}\overline{\Delta y_{it}}+\omega_{2i}\overline{y_{it-1}}+u_{it}$$where bars reflect cross-section means. The test statistic is then constructed from cross-section average of the t-ratios on \(({\rho }_{i}-1\)) coefficients and is referred to as cross-sectionally augmented Dickey Fuller (CADF) test.
Homogeneous cointegration assumes that long-run relationship (cointegrating vector) is the same for all countries, whereas heterogeneous cointegration assumes long-run relationship differs across countries.
The alternative hypothesis allows for cointegrating vectors to vary across different countries of the panel.
Westerlund (2007) allows for heterogeneous cointegration and is called second-generation cointegration tests by allowing for cross-section dependence.
References
Abu-Qarn, A. s., & Abu-Bader, S. (2007). Sources of growth revisited: evidence from selected MENA countries. World Development, pp.752–771.
Andreano, M. S., Laureti, L., & Postiglione, P. (2013). Economic growth in MENA countries: Is there convergence of per-capita GDPs? Journal of Policy Modeling, 35(4), 669–683.
Barro, R. J. (1991). Economic growth in a cross section of countries. The quarterly journal of economics, 106(2), 407–443.
Barro, R. J., & Sala-i-Martin, X. (1990). Economic growth and convergence across the United States (No. w3419). National Bureau of Economic Research.
Barro, RJ., Sala-i-Martin, X. (1992) Convergence. Journal of political Economy 100 2 223 251
Barro, R. J., & Sala-i-Martin, X. (2003). Economic growth, volume 1 of MIT Press Books.
Baumol, W. J. (1986). Productivity growth, convergence, and welfare: what the long-run data show. The American Economic Review, 1072–1085.
Bernard, A. B., & Durlauf, S. N. (1995). Convergence in international output. Journal of applied econometrics, 10(2), 97–108.
Bernard, A. B., & Durlauf, S. N. (1996). Interpreting tests of the convergence hypothesis. Journal of econometrics, 71(1–2), 161–173.
Blackburne, E. F., III., & Frank, M. W. (2007). Estimation of nonstationary heterogeneous panels. The Stata Journal, 7(2), 197–208.
Carlino, G. A., & Mills, L. O. (1993). Are US regional incomes converging?: a time series analysis. Journal of monetary economics, 32(2), 335–346.
Comin, D., & Hobijn, B. (2010). An exploration of technology diffusion. American Economic Review, 100(5), 2031–2059.
De Long, J. B. (1988). Productivity growth, convergence, and welfare: comment. The American Economic Review, 78(5), 1138–1154.
De Long, J. B., & Summers, L. H. (1991). Equipment investment and economic growth. The Quarterly Journal of Economics, 106(2), 445–502.
Ditzen, J. (2016). XTDCCE2: Stata module to estimate heterogeneous coefficient models using common correlated effects in a dynamic panel.
Ditzen, J. (2018). Contributions to the analysis of economic growth and cross-sectional dependence (Doctoral dissertation, Social Sciences).
Dowrick, S., & Nguyen, D. T. (1989). OECD comparative economic growth 1950–85: catch-up and convergence. The American Economic Review, 1010–1030.
Durlauf, S. N., & Johnson, P. A. (1995). Multiple regimes and cross-country growth behaviour. Journal of applied econometrics, 10(4), 365–384.
Eberhardt, M. (2011). Xtcd: Stata module to investigate variable/residual cross-section dependence.
Engle, R. F., & Granger, C. W. (1987). Co-integration and error correction: representation, estimation, and testing. Econometrica: journal of the Econometric Society, 251–276.
Evans, P. (1996). Using cross-country variances to evaluate growth theories. Journal of Economic Dynamics and Control, 20(6–7), 1027–1049.
Evans, P., & Karras, G. (1996). Convergence revisited. Journal of monetary economics, 37(2), 249–265.
Feenstra, R. C., Inklaar, R., & Timmer, M. P. (2015). The next generation of the Penn World Table. American economic review, 105(10), 3150–3182.
Galor, O. (1996). Convergence? Inferences from theoretical models. The Economic Journal, 106(437), 1056–1069.
Gerschenkron, A. (1962). Economic Backwardness in Historical Perspective (1962) (pp. 211–228). Markets as Institutions.
Guetat, I. (2006). The effects of corruption on growth performance of the MENA countries. Journal of Economics and Finance, 30(2), 208–221. https://doi.org/10.1007/BF02761487
Guetat, I., & Serranito, F. (2007). Income convergence within the MENA countries: a panel unit root approach. The Quarterly Review of Economics and Finance, 46(5), 685–706.
Hausman, J. A. (1978). Specification tests in econometrics. Econometrica: Journal of the econometric society, 1251–1271.
Islam, N. (1995). Growth empirics: a panel data approach. The quarterly journal of economics, 110(4), 1127–1170.
Islam, N. (2003). What have we learnt from the convergence debate? Journal of economic surveys, 17(3), 309–362.
Kao, C. (1999). Spurious regression and residual-based tests for cointegration in panel data. Journal of econometrics, 90(1), 1–44.
Levin, A., & Lin, C. F. (1993). Unit root tests in panel data: new results. University of California at San Diego, Economics Working Paper Series.
Lewandowski, P. (2006). PESCADF: Stata module to perform Pesaran's CADF panel unit root test in presence of cross section dependence.
Li, Q., & Papell, D. (1999). Convergence of international output time series evidence for 16 OECD countries. International review of economics & finance, 8(3), 267–280.
Lucas, R. E., Jr. (1988). On the mechanics of economic development. Journal of monetary economics, 22(1), 3–42.
Lucas, R. E. (1990). Why doesn’t capital flow from rich to poor countries? American Economic Review, 80(2), 92–96.
Maddala, G. S., & Wu, S. (1999). A comparative study of unit root tests with panel data and a new simple test. Oxford Bulletin of Economics and statistics, 61(S1), 631–652.
Makdisi, S., Fattah, Z., & Limam, I. (2007). Determinants of growth in the MENA countries. In J. Nugent & H. Pesaran (Eds.), Contributions to Economic Analysis (pp. 31–57). Elsevier.
Mankiw, N. G., Romer, D., & Weil, D. N. (1992). A contribution to the empirics of economic growth. The quarterly journal of economics, 107(2), 407–437.
Martín-Mayoral, F., & Sastre, J. F. (2017). Determinants of social spending in Latin America during and after the Washington consensus: a dynamic panel error-correction model analysis. Latin American Economic Review, 26(1), 10.
Nabli, M. M. K., & Arezki, M. R. (2012). Natural resources, volatility, and inclusive growth: perspectives from the Middle East and North Africa (No. 12–111). International Monetary Fund.
Neal, T. (2014). Panel cointegration analysis with xtpedroni. The Stata Journal, 14(3), 684–692.
Nehru, V., & Dhareshwar, A. (1993). Sources, methodology and results. Revista de análisis económico, 8(1), 37–59.
Nelson, C. R., & Plosser, C. R. (1982). Trends and random walks in macroeconomic time series: some evidence and implications. Journal of monetary economics, 10(2), 139–162.
Pedroni, P. (2004). Panel cointegration: asymptotic and finite sample properties of pooled time series tests with an application to the PPP hypothesis. Econometric theory, 20(3), 597–625.
Péridy, N., & Bagoulla, C. (2012). Regional integration and real convergence: evidence from MENA countries. Journal of Economic Integration, 80–114.
Persyn, D., & Westerlund, J. (2008). Error-correction–based cointegration tests for panel data. The STATA journal, 8(2), 232–241.
Pesaran, M. H. (2004). General diagnostic tests for cross section dependence in panels.
Pesaran, M. H. (2006). Estimation and inference in large heterogeneous panels with a multifactor error structure. Econometrica, 74(4), 967–1012.
Pesaran, M. H. (2007). A simple panel unit root test in the presence of cross-section dependence. Journal of applied econometrics, 22(2), 265–312.
Pesaran, M. H. (2015). Testing weak cross-sectional dependence in large panels. Econometric Reviews, 34(6–10), 1089–1117.
Pesaran, M. H., & Smith, R. (1995). Estimating long-run relationships from dynamic heterogeneous panels. Journal of econometrics, 68(1), 79–113.
Pesaran, M. H., Shin, Y., & Smith, R. P. (1999). Pooled mean group estimation of dynamic heterogeneous panels. Journal of the American Statistical Association, 94(446), 621–634.
Psacharopoulos, G. (1994). Returns to investment in education: a global update. World development, 22(9), 1325–1343.
Quah, D. (1993). Galton’s fallacy and tests of the convergence hypothesis. The Scandinavian Journal of Economics, 427–443.
Romer, P. M. (1986). Increasing returns and long-run growth. Journal of political economy, 94(5), 1002–1037.
Romer, P. M. (1990). Endogenous technological change. Journal of political Economy, 98(5, Part 2), S71-S102.
Sala-i-Martin, X., & V Artadi, E. (2003). Economic growth and investment in the Arab world.
Satti, S. L., Farooq, A., Loganathan, N., & Shahbaz, M. (2014). Empirical evidence on the resource curse hypothesis in oil abundant economy. Economic Modelling, 42, 421–429.
Solow, R. M. (1956). A contribution to the theory of economic growth. The quarterly journal of economics, 70(1), 65–94.
Wane, M. A. A. (2004). Growth and convergence in WAEMU countries. International Monetary Fund.
Westerlund, J. (2007). Testing for error correction in panel data. Oxford Bulletin of Economics and statistics, 69(6), 709–748.
Acknowledgements
We thank the anonymous referees of this journal for the useful inputs. We would also like to thank the Journal Editorial Office, for editorial assistance as well as inputs on earlier versions of the article.
Author information
Authors and Affiliations
Corresponding author
Ethics declarations
Conflict of Interest
The authors declare that they have no conflict of interest.
Additional information
Publisher's Note
Springer Nature remains neutral with regard to jurisdictional claims in published maps and institutional affiliations.
Appendices
Appendix 1
This section introduces the concepts of parameter heterogeneity, cross-sectional dependence, non-stationarity and cointegration in the panel framework. Parameter heterogeneity and cross-section dependence allows us to model differences in technology across countries; global shocks having differential impact across the countries and common (unobservable) factors to which individual countries react idiosyncratically.
Parameter Heterogeneity
In the long panel time series data, we can estimate separate regressions for each unit. Hence, the assumption of homogeneity (\({\beta }_{i}=\upbeta \forall i=1,\dots ,N\) in Eq. 1, “Model Specification”) becomes implausible in long panels. Formally, heterogeneity is modelled by allowing all the parameters, \({\alpha }_{i} \mathrm{and} {\beta }_{i}\) (in Eq. 1, “Model Specification”) to vary across countries.
Cross-Sectional Dependency Tests
In the long panel, observations from individual countries are more likely than not to be correlated across the panel either by some spatial process or by effects of common factors, formally known as cross-sectional dependence. Presence of cross-section dependence affects inference and leads to biased estimates unless we account for it in our empirical model. This cross-correlation may arise as a result of global shocks (e.g. oil shock, global technological shock, spatial spill overs, world interest rates, and supply chains) and/or local shocks (countries reliant on exports of oil resources, trade networks and business cycles). We use the Pesaran (2004) CD test as a pre-estimation check on the variables or residuals to get an idea whether or not the data is cross-sectionally dependent. It calculates correlation coefficient (\({\rho }_{ij}\)) between time-series variable of the \(i\) individual with the remaining \((j=i+1\dots . N)\) members in the panel. In our case of 15 countries, the test would calculate 15*14 correlations. Pesaran (2004)’s cross-section dependence test statistic is given as
where N is the number of countries and \({T}_{ij}\) refers to the number of observation used in calculating the pairwise correlation between \(i\) and \(j=i+1\dots . N\) countries. The null hypothesis of the test assumes cross-section independence and is distributed as asymptotically standard normal. Pesaran (2004)’s CD test is found to be robust to structural break, non-stationarity and parameter heterogeneity.
Unit Root Tests
An important step in the formal analysis of long time series panel data is to determine the order of integration of the variables. Most of the macro-variables, such as per-worker GDP, indeed contain a unit root (Nelson & Plosser, 1982). The literature identifies two strands of panel unit root tests, namely first-generation and second-generation tests. These tests are based on the rationale of Dickey-Fuller and augmented Dickey-Fuller regressions, given by
The subscript i on \({\mu }_{i}\), \({\beta }_{i}\) and \({\rho }_{i}\) makes it explicit that the time-series properties of the panel vary across the individual countries. The lag structure corrects for serial correlation in the errors. We use Maddala and Wu (1999) and Pesaran (2007) panel unit root tests. Maddala and Wu (1999) is an example of first-generation panel unit root test and assumes cross-section independence in data, whereas Pesaran (2007) is a second-generation test and allows for the presence of cross-section dependence. These tests run augmented Dickey-Fuller regressions one by one for each N countries to obtain an estimate of \({(\rho }_{i}-1)\). They, however, use different procedures for summarising that information into a single panel test statistic.Footnote 13 Both of the tests examine the null hypothesis of nonstationary in all panel member series. The alternative hypothesis is stationarity in at least some country series (heterogeneity in autoregressive coefficients of the Dickey-Fuller regressions) and is more appropriate than the homogeneous alternative (stationarity in all the country series). If the variables are found to be nonstationary, then cointegration test (Engle & Granger, 1987) can be applied to test the long-run relationship between the variables. Engle and Granger test the time series properties of the residuals obtained from a regression of nonstationary variables using the (augmented) Dickey-Fuller test. The null hypothesis assumes that the residuals are nonstationary. Rejection of null indicates that the residuals are stationary, and the variable series are cointegrated.
Panel Cointegration Tests
After using panel unit root tests to determine the order of integration of the variables, panel cointegration tests are used to examine the tendency of convergence in output and its sources among a cross-section of MENA countries over time. Cointegration means the existence of a long-run relationship in the data. Panel cointegration testing can be divided into first- and second-generation procedures, where the former assumes cross-section independence, and the later relax that assumption. The residual-based tests by Kao (1999) and Pedroni (2004) are examples of first-generation residual-based cointegration tests, where the former assumes homogenous cointegrationFootnote 14 and the latter assumes heterogeneous cointegration. Westerlund (2007) is an example of second-generation error correction test that allows for heterogeneous cointegration as well. Pedroni (2004)’s cointegration test is based on residuals estimated from the possible cointegrating equation between nonstationary variables y and x (Eq. 1, “Model Specification”) under the null hypothesis of no cointegration:
Pedroni (2004) computes seven cointegration statistics with null of no cointegration in a heterogeneous mixed (I(0) and I(1) variables) panel framework.Footnote 15 These tests can be divided into two groups on the basis of cointegrating residuals, \({\widehat{\varepsilon }}_{it}\). The first group includes four ‘panel statistic’ cointegration tests based on pooling along within dimension (pooling the AR coefficients across different countries of the panel for the unit-root test on the residuals). The second group, ‘group-mean statistic’ includes three tests based on pooling the ‘between’ dimension (averaging the AR coefficients for each member of the panel for the unit-root test on the residuals).
Westerlund (2007) proposed error correction-based cointegration test that corrects for cross-section dependenceFootnote 16 in the data. The approach involves the following error correction model of Eq. 1, “Model Specification”:
where \({\Psi }_{i}\) is referred to as error (equilibrium) correction coefficient or speed of convergence. It indicates how fast the system returns to long-run equilibrium following a shock in the system. Westerlund (2007) developed four test statistics based on the assumption that \({\Psi }_{i}=0\). Two of these tests are group mean cointegration statistic (Gt and Ga) that investigates cointegration in atleast one unit, \({\Psi }_{i}<0 \mathrm{for at least one} i\). The other two tests are the panel cointegration statistics (Pt and Pa) that investigate cointegration for the entire panel, \({\Psi }_{i}<0 \mathrm {for all} i\). Westerlund test allows bootstrapping procedure in which cointegration tests can be repeated multiple times, which is meaningful in the presence of cross-section dependence.
Appendix 2
The panel ARDL model (p, q) of the long-run relationship between variables is given by (Pesaran 1999, 2004): (B-1)
where \(X\) is a vector of explanatory variables, \({\upxi }_{\mathrm{ij}}\) and \({\uppsi }_{\mathrm{ij}}\) are the country-specific short-term coefficients. \({\alpha }_{i}\) captures country-specific effects and \({\varepsilon }_{i,t}\) is an error term. The value of \({\beta }_{i}<0\) shows the existence of long-run relationship between the variables. Three different panel ARDL estimators can be used to estimate model B-1, namely mean group (MG), pooled mean group (PMG) and dynamic fixed effect (DFE). Panel ARDL estimators provide estimates for both short- and long-run coefficients as well as an estimate of country-specific equilibrium correction term towards their own steady-state level of income. Based on Pesaran et al. (1999), re-parameterisation of the above model in error correction form is specified as follows:
where \({\lambda }_{it}\) is the error correction coefficient and is expected to be negative. \({\theta }_{t}\) is the vector of long-run coefficients. \({\xi }_{ij}\) and \({\psi }_{ij}\) are the short-run dynamic coefficient. The error correction coefficient measures the speed of short-run adjustment towards long-run equilibrium following an exogenous shock. The long-run or cointegration relationship between GDP and its regressors is inferred from the negative and statistically significant error correction term. This result can also be interpreted as evidence of β-convergence (Martín-Mayoral & Sastre, 2017; Wane, 2004).
Although MG and PMG estimators allow for heterogeneity in the slope coefficients, they may provide misleading results if the common factors are correlated across the countries. A method of allowing for the presence of common factors is based on Pesaran (2006) known as common correlated effects. This method uses cross-sectional averages of the dependent and independent variables computed from the entire panel to account for the presence of unobserved common factors:
where \(\stackrel{-}{{Z}_{t}}=(\stackrel{-}{{y}_{t}}, \stackrel{-}{{X}_{t}}\)), with \(\stackrel{-}{{y}_{t}}\) and \(\stackrel{-}{{X}_{t}}\) are the cross-country averages of the dependent and independent regressors. (See Table 9).
Appendix 3
Rights and permissions
About this article
Cite this article
Malik, M.A., Masood, T. Dynamics of Output Growth and Convergence in the Middle East and North African Countries: Heterogeneous Panel ARDL Approach. J Knowl Econ 13, 1444–1469 (2022). https://doi.org/10.1007/s13132-021-00780-3
Received:
Accepted:
Published:
Issue Date:
DOI: https://doi.org/10.1007/s13132-021-00780-3