Abstract
The current Sub 6 GHz band is highly occupied and unable to meet the demands of future wireless data traffic. As the millimeter wave technology is a promising way of enhancing the performance of next-generation wireless networks, it is essential to explore FR2 frequency bands. Massive Multiple-Input Multiple Output (mMIMO) technology has been introduced to improve the capacity and coverage of 5G wireless networks. Hybrid beamforming (HB) is an efficient technique to improve the channel gain and minimize the interference at reasonable complexity. The aim of HB in mMIMO systems is to maximize the sum rate that can approach the fully-digital beamforming system performance. Combination of mMIMO systems, mmWave, and HB techniques achieve higher data rates and cell coverage in 5G wireless networks. It mitigates the hardware complexity, energy consumption, and cost as number of required radio-frequency chains at base station gets minimized. In this paper, we have investigated the performance of a multi user-mMIMO HB system at 28, 39 GHz of FR2 frequency bands as well as at 66 GHz by analyzing the effect of changing variables that include the number of users, transmitting and receiving antennas, and the type of modulation. Simulation results include RMS values of error vector magnitude (EVM) that gives quantitative analysis and receive constellation of the equalized symbols that shows qualitative analysis. EVM is computed for different scenarios and it is used as an indicator of performance to assess the suitable design configuration of mMIMO system.
Similar content being viewed by others
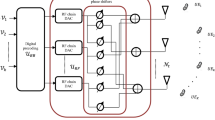
Avoid common mistakes on your manuscript.
1 Introduction
The ever-growing demand for user capacity and higher data rates lead to effective utilization of available frequency spectrum. The recent research work has focused on usage of mmWave frequency bands for 5G cellular systems to achieve higher spectral utilization as the wavelengths are very smaller at mmWave bands. On the other side, mmWave frequencies cause higher propagation losses and limit the communication range. To overcome these losses, power consumption, and hardware cost in 5G NR networks, large scale antenna arrays are used where each antenna element or multiple antenna elements (subarrays) are connected to a TR module and this technique is called as “Hybrid Beamforming (HB)”. The HB and mMIMO technologies together combines the analog pre/post processing and digital processing at mmWave bands. It provides the tradeoff between energy and spectral efficiencies which is key the designing aspect in 5G wireless networks.
In order to allow the BS to serve multiple UEs with same available frequency-time resources, SDMA is used in Multi-User mMIMO (MU-mMIMO) wireless communication networks which provides maximum multiplexing gain, spectral efficiency, and system throughput. Design of transmit vectors to distinguish UEs and reduce co-channel interferences is challenge in MU-mMIMO systems, especially in UE dense areas where MU pairs are higher. However, mMIMO designs enhances the spatial resolution with higher number of narrow beams and maximum degree of freedom for MU paring. mMIMO HB system allows tens to hundreds number of antennas at the BS and serve UEs with maximum number of data streams in a cell.
The higher propagation losses occur at mmWave bands can overcome using larger antennas arrays which are deployed in 5G cellular systems. As the physical dimensions are relatively smaller at mmWave bands, we can accommodate higher number of antenna elements in given physical area. However, providing one RF chain per antenna element is not at all feasible and cost effective method. Therefore, HB transceivers are designed where the number of RF chains are much less than the number of antenna elements when we compare in a pure analog (in RF domain) and digital (in baseband domain) beamforming systems. Multiple-TR path designs in 5G NR addresses inter carrier interference, improves spectral efficiency and reliability. It also increases the data transmission by aggregating resources of multiple-TR paths.
Fully digital beamforming designs are not effective in terms of power consumption and hardware cost as each antenna should have a dedicated RF-to-baseband chain. To have maximum throughputs in a scattering-rich environment where there may exist only non-LOS paths between Tx and Rx antennas, mMIMO HB is implemented by the use of precoding at Tx side and combining at Rx side to improve SNR and separate independent spatial channels. mMIMO HB systems as shown in Fig. 1 minimizes the required number of RF-to-baseband chains. With appropriate selection of precoding and combining weights, HB gives much better performance compared to complete analog or digital beamforming. Present modern wireless communication systems including 5G cellular systems extensively use mMIMO HB technology for SNR improvements and SDMA to enhance data throughput in scattering rich environments. Using HB, spectral efficiency can be improved significantly by supporting more number of data streams.
2 Literature Review
HB can be classified based on the parameters like required instantaneous/average CSI in the analog beamforming part, carrier frequency, complexity (fully, reduced, and switched). There is no single design/algorithm that can give the best trade-off between these parameters [1]. The performance of MU-mMIMO HB FDD system at FR2 frequency range (24.25 GHz—52.6 GHz) is very attractive to facilitate higher bandwidths and support extremely higher data rates [2]. Finding a suitable configuration in designing a mMIMO HB system is very essential to have optimum performance in 5G wireless networks [3]. There are lots of research is happening in designing mmWave mMIMO HB system and realize it with different precoding/combining techniques, number of BS antennas, and different channel conditions [4]. Optimal design of analog and digital precoder/combiner in a MU-mMIMO HB DL system reduces the number of RF chains and support multiple data streams per UE and maximum sum rate close to channel capacity under perfect CSI [5, 6]. This system gives the tradeoff between BS antennas and data streams per user. It is recommended to have more number of parallel data streams per user to achieve higher throughputs [7]. MU-MIMO HB systems focus on optimization of analog RF precoding/combining in fully connected and partially connected structures [8]. Partitioning RF baseband and digital domain with optimal precoding and combining are important aspects in MU-mMIMO HB systems. It provides the tradeoff between beamforming gains and hardware cost [9].
As fully-digital beamforming is not feasible in mMIMO systems due to their more energy consumption of RF chains at mmWave bands, HB is introduced to minimize the number of RF chains. HB in mMIMO systems gives tradeoff between system performance and implementation cost (in terms of both power consumption and hardware cost). It reduces energy consumption and the cost of implementation. Even though, the mmWave communications with FD provides higher SE compared to half-duplex, the inherent properties of higher path loss at mmWave bands and self-interference in FD may minimize the overall performance of the system. HB at mmWave bands exploit a large bandwidth and also overcome the large path loss of Rayleigh fading channels. It gives the trade-off between the cost efficiency and energy consumption by RF chains [10].
Optimizing the analog beamformers and digital combiners of HB for the DL of MU-mMIMO in FD mode reduces the CSI acquisition overhead [11]. mMIMO DL can provide maximum tradeoff between SE and EE when the number of BSs for the given SE is high and every cell is allocated with optimal transmit power [12]. Interference (both inter-user and intra-user) can be cancelled in a mmWave MU-mMIMO HB DL systems using techniques like ZF and SIC. But, SIC based HB algorithms outperforms in terms of SE at the cost of higher computational complexity [13]. The joint design of Tx and Rx in mmWave MU-mMIMO HB system for DL reduces the computational complexity where “piecewise dual joint iterative approximation” method is used which can provide closed form solutions in the analog beamforming and “baseband piecewise successive approximation” in designing digital beamforming that maximizes the number of simultaneous users served [14, 15].
The optimal design of MU-mMIMO HB FD DL system uses 2nd-order channel statistics where UE needs to feed back only intra-group effective channel. The strongest Eigen beam of the Rx correlation matrix forms optimal analog combiner. The joint optimization of the digital precoder/combiner with limited instantaneous CSI can enhance the sum-rate [16]. Formulating precoding/combining as a sparse reconstruction and designing algorithms based on the basis pursuit principle leads to low-cost RF hardware [17]. “Channel hardening” in a mMIMO system makes the effective channels as almost deterministic in spite of the random channel response. Efficient CSI can be estimated using TDD that uses channel reciprocity where only UL pilot signals are needed with no feedback [18]. With optimal design of transmit vectors (which mitigates the co-channel interference), SDMA provides maximum system throughput in MU-mMIMO DL systems. “Block-diagonalization” method optimizes the maximum transmission rate and “successive optimization” method optimizes the minimum power [19]. In a MIMO system, fading correlations between Tx and Rx depends on scatterers and the physical dimensions of the antenna elements. Characterizing these fading correlations is important as they contribute in defining the channel capacity [20].
“Joint spatial division and multiplexing” method in MU-mMIMO DL system can minimize the dimensions of CSI at Tx and allows FDD to enhance SE. In this, DFT based “pre-beamforming” matrix is constructed based on channel second order properties in designing a precoder and ensure that there is no loss of optimality compared to full CSI case [21]. “manifold optimization” based HB algorithm is proposed to achieve MMSE and enhance the transmission reliability. To minimize the computational complexity in HB design, eigenvalue decomposition and OMP methods are suitable for narrowband and broadband scenarios respectively [22]. Fully digital BF is not suitable for mmWave mMIMO due to their large number of RF chains and channel bandwidths. Polarization enhances the performance further for mmWave mMIMO, particularly for LOS channels. While for NLOS channels where they have greater path loss, it is essential to have HB designs with SDMA features. MU-mMIMO HB design for mmWave networks supports sending data to multiple users using low-rank channels and have multiplexing gain. The extensive channel measurements at 28 GHz and 73 GHz show that the directional beams exist with low path loss and less multipath time dispersion. It means that higher amount of link powers can be received with beamforming design without a tedious equalization process [23]. mMIMO system with HB is envisioned to provide excellent tradeoff between system performance and hardware complexity. Joint design of digital and analog precoder/combiner in a single cell MU-mMIMO DL system avoids loss of information at individual stages and supports multiple streams per UE to achieve maximum sum-rate that approaches channel capacity under perfect CSI [5].
HB with selection method reduces the computational as well as the hardware costs of MU-mMIMO systems without affecting the beamforming gain. In this method, the antenna array of transceiver is fed with analog is a number greater than up/down conversion chains, K̅) and selects instantaneous best K̅ ports as input to the up/down conversion chains [24]. Higher path attenuations at THz bands also can be compensated by beamforming, aperture gains using mMIMO, IRS techniques. Joint and cooperative HB techniques reduces the channel estimation cost under perfect CSI and achieves performance close to the fully digital beamforming system [25]. In the given frequency band, FD radio doubles the data rates using simultaneous transmission and reception. The BS in a single-cell mmWave mMIMO HB FD system suffers from limited dynamic range noise because of non-ideal hardware impairments. Optimal power allocation schemes that consider self-interference at UEs in designing HB reduces the number of RF chains and maximizes the weighted sum-rate [26]. In mMIMO systems, RF-baseband precoding using “uniform circular arrays” through phase mode transformation suppresses the inter-user interference and maximizes sum-rate with minimum CSI [27]. Spatial modulation scheme minimizes the required number of RF chains without compromise for spectral efficiency in mmWave mMIMO HB systems. The gain of these systems can be enhanced by increasing the number of antennas in the array at the Tx with no increase in the number of RF chains [28]. Acquisition of CSI in designing mMIMO systems plays a major role where instantaneous CSI gives better SINR and average (second-order) CSI provides lower overhead. A joint design of linear precoder and decoder using unweighted minimum MSE criterion can reduce ISI there by reducing BER. For practical implementations, it depends on the trade-off between the system complexity and accuracy of CSI [29].
In designing an optimal HB for minimizing the transmission power under individual SINR constraints in a MU-mMIMO system, we need to consider two cases. If the number of UEs less than or equal to number of RF chains, a globally optimal solution achieves optimal transmitting power similar to fully-digital beamforming. Otherwise, if the number of UEs are greater than number of RF chains, a stationary point is obtained using a globally convergent alternating algorithm [30]. Selection of UE is an important function in MU mMIMO system as it is severely affected by inter-user interference. The computational complexity of UE selection increases with size of the channel matrix and user-fairness because it involves re-computation of precoding and combining matrices. We can overcome this by selecting UE based on “proportional fairness” criteria and chordal distance [31]. HB designs in MU mMIMO-OFDM systems maximizes the SE when we use “locally optimal alternating maximization” and minimum MSE based iterative algorithms for analog and digital beamformers respectively [32]. Higher propagation losses at mmWave bands can be compensated by applying relays in MU mMIMO systems. HB designs in such relay assisted systems include “transmit-receive coordinated beam alignment procedure” for analog beamforming and “non-linear precoding” for digital beamforming [33]. Most of the HB designs in MU MIMO-OFDM systems considered frequency-flat channels, however it is also essential to evaluate the frequency-selective channels to maximize the received signal strength and to reduce the leakage powers [34].
In MU-MIMO HB systems, in order to support maximum number of UEs simultaneously in the give time–frequency slot, high dimensional channel information is needed in which case, feedback overhead is very high. The channel feedback overhead can be reduced using the properties “common sparsity of channel” and “nonlinear quantization”. The channel sparsity matrices are calculated using minimum MSE-OMP algorithm and they are quantized using nonlinear codebook generated by “conditional random vector quantization” [35]. ZF precoding in HB enhances the EE as it uses minimum number of RF chains with increased number of BS antennas [36]. When “conjugate beamforming” and ZF methods are used in mMIMO systems, the spectral efficiencies at 28 GHz is better than at 73 GHz and it increases with number of BS antennas. However, with higher number of UEs, conjugative beamforming outperforms the ZF in terms of complexity [37]. Design of sub-antenna arrays with non-uniform and optimal number of antennas per RF chain at the Rx of a mMIMO HB system enhances the total achievable rate by 10% with marginal increment in power consumption [38]. The IQ imbalance at the Tx of UEs in mMIMO HB UL system leads to finite ceiling of achievable sum-rate even at higher SNR. Introducing ZF techniques at the BS can compensate for undesirable IQ imbalance under different channel conditions [39].
Conventional HB methods are highly complex and strongly dependent on the quality of CSI. An extreme learning machine based HB can optimize beamformers of Tx and Rx by reducing the computational time and enhance the sum-rate [40]. Machine learning techniques to select beam-user combination reduces the complexity in MU mMIMO HB DL system even under poor channel conditions. In orthogonal HB design, analog beamforming matrix is generated by household reflectors and feedforward neural network to select beam-user. It gives (SE, EE) of (41.35 b/s/Hz, 0.8 b/Hz/J) compared with (33.77 b/s/Hz, 0.63 b/Hz/J) of DFT based state-of-the art techniques [41]. Most of the existing HB approaches in a mmWave mMIMO systems using FD links are strongly relay on optimization processes, which are either dependent on quality of CSI or too complex. To overcome this, CNN and machine learning based algorithms are introduced to guarantee 22.1% higher SE compared to OMP algorithm [42]. “Federated learning” based ML framework for HB design overcomes the need of training a global model with a larger data set. Instead, the model is trained at the BS with the gradients collected from UEs and it is shown that it is tolerant to the corruption and imperfections of channel data [43]. Beamforming designs with minimum number of RF chains and phase-shifters under imperfect CSI is challenging in a mmWave mMIMO systems. Deep learning based beamforming designs provide higher SEs even under imperfect CSI [44]. mMIMO with HB enhances the computational accuracy of “over-the-air computation” in IoT networks with reduced mean squared errors [45]. The digital beamforming using “block diagonalization” mitigates intra-user and inter-user interferences also it provides more sum rate over OMP based HB if the number of RF chains are at least double the number of data streams [46]. An optimal HB design to reduce the total transmission power under individual SINR constraints in a MU-mMIMO system leads to a “non-convex optimization problem”. In order to solve this problem, two low-complex algorithms are proposed based on the number of UEs and RF chains that give globally optimal solution [30].
A novel HB design for mMIMO DL is proposed which combines the RF and baseband precoding based on “regularized channel diagonalization” to have a low complex solution with maximum sum-rate [47]. Aiming at minimizing the ratio of computation to communication power in MU-mMIMO systems, a joint optimization problem is formulated with partially-connected RF structures and the proposed algorithm enhances the EE, cost efficiency, and maximum power saving by 76.59% [48]. The capacity of a MU-MISO DL system can approach the fully digital precoding system if the iterative hybrid precoding scheme is used. In this scheme, ZF digital precoding is used to get large baseband gain and then obtain the optimized RF precoder by reducing the total power [49]. In a mmWave mMIMO systems, the number of RF chains are dependent on hybrid precoding designs and therefore efficient precoding architectures are essential to enhance the EE and SE in the system. Design of hybrid precoder based on closed-form expressions can minimize the required active RF chains and the computational complexity, also enhance the EE and SE even at the low SNR values [50]. If the HB system has higher number of antenna ports to support maximum bandwidth, sub-band level precoding leads to higher feedback overhead. Transform domain precoding based on channel sparsity can enhance SE by 2.38% ∼ 21% and reduce the feedback overhead by 60% ∼ 89% over the existing techniques [51]. It has been concluded that a single algorithm or structure is not existing to give best tradeoffs among the design parameters of HB.
3 Proposed Methodology
In a mmWave MU-mMIMO HB systems, precoding/combining, beamforming processes are performed partly in the in analog RF domain and partly in digital baseband domain. It is challenging to derive four matrices jointly for analog and digital precoder, analog and digital combiners. Therefore, we have separately designed analog and digital beamformers at Tx and Rx using MMSE technique with separate transmit precoding and receive combining to maximize the sum-rate. Using the spatial structure of mmWave channels, we have designed RF and baseband filters jointly via OMP and approach the fully digital beamformers.
The proposed MU-mMIMO HB system consists of mainly four modules: Tx, Rx, channel and hybrid weight calculations as shown in Fig. 2. Data streams are generated at the MIMO Tx and precoded followed by modulation. The modulated signal is travelled through a scattering MIMO channel and at the Rx, it is decoded and demodulated. The precoding weights are used to modulate the data streams at the Tx and recovered at Rx using combining weights. There exists always a trade-off between computational load and optimal weights calculations of the above four matrices. The communication link between the Tx and Rx is validated based on scattering-spatial channel model and flat-static MIMO channel which considers various antenna patterns and TR spatial locations. The periodic updating of channel matrix mimics the variations of MIMO channel with respect to time. Digital Rx reconstructs the transmitted data streams and RMS EVM is calculated, also compared for different channels at FR2 frequency bands. Beamforming is obtained in analog/RF domain by applying phase shift at each antenna element of the subarray. Beamforming is achieved in the digital baseband domain using channel matrix to derive precoding/combining weights which help to transmit and recover multiple independent data streams in a single channel.
In the Figs. 3 and 4, NT and NR are the number of Tx and Rx antennas respectively; NS is the number of data streams; NTRF and NRRF are the number of transmit and receive RF chains respectively; ‘H’ represents MIMO scattering channel; FBB and FRF are the digital decoder of size NS \(\times\) NTRF and analog precoder of size NTRF \(\times\) NT respectively; WRF & WBB are the analog combiner with NR \(\times\) NRRF size and digital combiner with NRRF \(\times\) NS size respectively. In a HB system, NTRF < NT it means that the number of TR modules are less than the number of antenna elements. The design flexibility is achieved by connecting each antenna element to one or more TR modules. The design of HB using mathematical representation is shown in Eqs. 1 and 2. FRF and WRF matrices represent the signal phase values.
We have used two HB algorithms named as “quantized sparse HB”, and “quantized HB with peak search”. For computing the hybrid weights in a channel matrix, OMP algorithm is used and the channel model considered is “MIMO scattering channel”. The outputs are analog precoding/combining weights and they become steering vectors corresponding to dominant modes in the channel matrix. Hybrid weights of the channel matrix are computed iteratively to replicated the channel variations. Data streams which use the most dominant mode of MIMO channel gives maximum SNR. When we use HB with peak search algorithm, it avoids iterative searching for dominant modes in channel matrix and provides all the digital weights with peaks to extract the corresponding analog beamforming weights. This algorithm is well suited for mMIMO systems of larger size.
3.1 Design of MU-mMIMO HB Systems
In the MU-mMIMO HB systems, the channel rank is higher and it creates a rich number of effective channels using spatial separation for users. The mMIMO channel can be modelled as
where H is the channel matrix given as \( {\mathbf{H}} = \left[ {\begin{array}{*{20}c} {h_{11} } & {h_{21} } & {...} & {h_{M1} } \\ {h_{12} } & {h_{22} } & {...} & {h_{M2} } \\ {...} & {...} & {...} & {h_{M3} } \\ {h_{1M} } & {h_{2M} } & {...} & {h_{MM} } \\ \end{array} } \right],{\mathbf{s}} \to {\text{transmitted vector}},{\mathbf{y}} \to {\text{received vector}},{\text{ and }}{\mathbf{n}} \to {\text{noise vector}}. \) where each element, hij in the channel matrix is a complex random variable of gaussian in nature and represents the fading gain between ith transmitted & jth receiver antenna.
High dimensional channels are estimated to design a precoder of MU-mMIMO system at mmWave frequency bands. Even though, the analog precoders are less complex, but they provide limited performance (only one data stream is supported). On the other hand, digital precoders give high performance but at the cost of power consumption and hardware complexity (higher number of ADCs and RF chains). However, hybrid precoding combines analog and digital precoding techniques to minimize the required number of RF chains and maximum spatial multiplexing gain in MU-mMIMO HB systems as shown in Fig. 5. Under known CSI conditions, the diagonalization of H provides optimal precoding weights by extracting the first NTRF dominating modes. Therefore, for the known CSI at the Tx of BS, we can perform MU precoding to allow all users to transmit their data streams at the same frequency & time slots, still allow users to recover data streams with lower complexity.
The observation vector for user ‘i’ (for the DL signal),
where xi → signal meant for user k, ni → noise vector, Hi → channel matrix that represents the channel from BS to user ‘i’. 2nd term of Eq. (4) gives the signals for remaining users.
To transmit multiple data streams, we perform precoding as xi = Wisi.
Then the DL signal for user ‘i’ becomes,
where Wi → precoding matrix si → transmitted QAM symbol vector for user ‘i’.
2nd term of the Eq. (5) represents precoding for remaining users.
The scalar observation for user ‘i’ is given as,
where hTi → DL channel for user ‘i’, ni → scalar noise.
1st term of Eq. (6) shows the effective channel experienced the user ‘i’ and 2nd term represents the interference caused by other users.
Therefore, the MU-mMIMO HB system is represented as,
To achieve the optimum performance of MU-mMIMO HB system, the precoding matrix, W shown in Eq. (7) can be designed at the BS using techniques like MMSE, and ZF.
At the Rx, all signals are summed to have an observation vector,
From Eq. (8), the observation vector for UL is given as,
[HT1 HT2 HT3 ………. HTi] is the channel matrix of size Mr \(\times\)(iMt) which is the concatenation of all the UL channels.
Mr rows represent the Rx antennas at BS and Mt represents the number of Tx antennas per user.
3.2 Precoding and Combining in MU-mMIMO HB Systems
Consider K users and BS with M antennas, then the channel matrix H of DL becomes K \(\times\) M size where each row represents a DL channel of a particular user.
The ZF precoding matrix,
where H+ → channel pseudo inverse.
Under the poor channel conditions, W in Eq. (9) becomes large and it causes higher transmission powers. By enforcing the constraint PTotal = \(\left\| W \right\|^{2}\).
The total power,
In order to get the Eq. (10), scale the precoding matrix with a lower values and ensure equal SNR at each user.
In case 1, where BS has large number of Tx antennas, then the channel matrix, H will have almost orthogonal rows and in case 2 where the UE has large number of Rx antennas, then H will have almost orthogonal columns. The channel capacity of such channels are given as.
The capacity of a mMIMO channel,
where \({\text{B}} \to {\text{bandwidth}}, \, \rho \to {\text{SNR}}\).
In a MU-mMIMO HB DL systems, the channels are nearly orthogonal with reciprocity (apply TDD) and the corresponding channel matrices can be decomposed into the Equations shown in (12) and (13).
For UL channel, the channel matrix
For DL Channel,
GTG* ≈ M IK are the orthogonal channels with hi = d \(_{i}^{0.5}\) gi. where D → diagonal matrix which represents path loss per UE, G → multi-path fading matrix, hi → column vector of H matrix.
The DL is complex as additional precoding is required at BS to avoid inter-user interference and the observation model is given in Eq. (14) as,
where HT → DL channel matrix, W → optimal precoding matrix, n → noise vector at each user,
s → data for each user.
An optimal precoder is pseudo inverse of channel matrix.
Therefore, we select the precoding in the form,
where \({\mathbf{H}}^{*} \to\) complex conjugate of the channel \(\surd {\text{M}} \to\) scaling factor.
Dp → diagonal matrix that has square root of powers at each user.
To meet the power constraints in Eq. (15), power allocation Dp to ensure \(\left\| W \right\|^{2} = {\text{ trace}}\left( {{\mathbf{W}}^{{\text{H}}} {\mathbf{W}}} \right) \, = {\text{ P}}_{{{\text{Total}}}}\).
We find that \({\mathbf{W}}^{{\text{H}}} {\mathbf{W}} = \, \surd {\mathbf{D}}_{{\text{p}}} {\mathbf{H}}^{{\text{T}}} {\mathbf{H}}^{*} \surd {\mathbf{D}}_{{\text{p}}} /{\text{M}}_{{}} = \, \surd {\mathbf{D}}_{{\text{p}}} {\mathbf{DD}}\surd {\mathbf{D}}_{{\text{p}}} = {\mathbf{D}}_{{\text{p}}} {\mathbf{D}}\).
\({\mathbf{D}}_{{\mathbf{p}}} \to\) diagonal matrix of powers \({\mathbf{D}} \to\) diagonal matrix of path loss values.
The observation vector,
From the Eq. (16), the observations at each user, \({{\rm{y}}_{\rm{k}}} = {\rm{ }}\surd \left( {{\rm{M}}{{\rm{d}}_{\rm{k}}}} \right){\rm{ }}\surd {\left[ {{{\bf{D}}_{\rm{p}}}} \right]_{{\rm{k}},{\rm{ k}}}}{{\rm{s}}_{\rm{k}}} + {\rm{ }}{{\rm{n}}_{\rm{k}}}\)
The channel in the DL for user i,\({\mathbf{h}}_{{\text{i}}}^{{\text{T}}} = \, \surd {\text{d}}_{{\text{i}}} {\mathbf{g}}_{{_{{\text{i}}} }}^{{\text{T}}} \quad {\mathbf{h}}_{{\text{i}}}^{{\text{T}}} \to {\text{row vector of length M}}.\)
From the Eqs. (16) and (17), we can understand that MU-mMIMO HB DL channel is a matched filter with asymptotically optimal linear precoder. The conclusion is that with MU-interference can be eliminated at all UEs with simple linear processing at BS.
For a MU-mMIMO HB UL systems, the observation model is given in Eq. (18) as,
The combining matrix is given as,
In Eq. (19),
The SNR of kth user, \({\text{SNR}}_{{\text{k}}} = {\text{ Md}}_{{\text{k}}} {\text{E}}_{{\text{x}}} /{\text{N}}_{0 } {\text{where N}}_{0} {\text{Md}}_{{\text{k}}} \to {\text{noise variance}}\), where \({\text{N}}_{0} \to {\text{noise power}}\).
Data rate for kth user,
Therefore, total sum-rate in a MU-mMIMO HB UL system is given as,
The rate of each user given in Eq. (20) can be summed up to get the total system capacity as shown in Eq. (21). The conclusion is that the simple linear combing at BS with asymptotic increase in number of antennas leads to optimal results even in the presence of MU-interference.
In MU-mMIMO HB systems, the users are orthogonal and LOS depends on the antenna array geometry as well as far field conditions. If the antenna elements are spaced at 0.5λ and the incoming signal has an angle ‘θ’ with a path difference of δ = d sin(θ). Then, the passband and baseband received signals are given in the Eqs. (22) & (23) respectively.
The condition for LOS orthogonality is given in Eq. (24).
The favorable conditions in NLOS propagation depends orthogonal columns of UL channel matrix HHH≈MIK, scaling of off-diagonal elements, and Rayleigh fading entries in CN (0,1).
4 Result Analysis
In this paper, spatial and static-flat fading MIMO channels were used for conducting simulations with simulation parameters as shown Table 1. “Single-bounce ray tracing” scattering model is applied with random placement of scatters. A common channel is used to model path loss under LOS and non-LOS (more close to real scenarios) conditions. The same channel is used for data transmissions and sounding. Simulations were conducted at 28 GHz, 39 GHz, and 66 GHz carrier frequencies for different MU-mMIMO configurations with isotropic antenna arrays having linear and rectangular geometry. For achieving maximum SE, each RF chain transmits a data stream at every UE and each UE is assigned with independent channels. A complex Gaussian distribution is considered with circular symmetry for scattered ways to define the path gain. The Rx of UE is modeled to compensate for thermal noise and path loss. Four users are considered in a MU-mMIMO HB system with 3, 2, 1, and 2 number of independent data streams per UE and the number of antennas at each UE are 12, 8, 4, and 8 respectively. RMS EVM values (quantitative analysis) and receive constellations (qualitative analysis) are observed using different modulation schemes.
Figure 6 shows the EVM values at 28 GHz for various modulation schemes with 256 number of BS antennas. With 64 BS antennas, the lowest EVM which is 0.37929% is achieved for User 1 and highest EVM, 2.1165% is noted for User 3. As User 1 has three independent data streams and User 3 has only one independent data stream, it means that EVM is decreasing with higher number of independent data streams. If 128 BS antennas are used, there is moderate EVM values are achieved for Users 1, 2 and interestingly EVM values are decreased for User 3 and 4. If the number of BS antennas are increased to 256, there is a slight increment in the values of EVM for all users. User 4 has optimal performance compared to all other users as it has 8 UE antennas with two independent data streams. User 3 has highest EVM as it has only one data stream with 4 UE receiving antennas. For 64 BS antennas, EVM is minimum at higher order modulation scheme that is 256-QAM. For 128 BS antennas, EVM is very slightly increasing with increasing modulation order from 4 to 8.
From the overall results, it shows that 128 × 8 mMIMO gives optimum performance at 28 GHz carrier frequency. Figures 7, 8, 9 represent radiation pattern of the signal in a scattering channel with multiple BS antennas. It has clearly shown that increasing number of antennas in the MU-mMIMO HB system leads to focused radiation beams. This improves SNR at the Rx and provide high reliability for data transmissions. Figure 10 shows the equalized symbol constellation/stream of 256-QAM modulation with 256 base station antennas and it shows that for user 3 the received symbols are more deviated from the signal constellation points that leads to errors in detection.
Figures 11, 12, 13 indicate the RMS EVM values at 39 GHz carrier frequency with different number of BS antennas and various modulation schemes. From the observations of simulated results at 39 GHz, the lowest EVM value is 0.55795% and it is achieved by user 4 with 256 BS antennas using 64-QAM. The maximum EVM value is noted as 2.2252% with 64 BS antennas using 64-QAM modulation scheme. For user 1, minimum EVM values are achieved with 64 BS antennas for all the modulation schemes and moderate EVM values are noted when the BS antennas are 128. Errors are slightly increased when the number of BS antennas are increased to 256. For user 2, minimum EVM values are achieved with 256 number of BS antennas. For user 3 where it has only one independent data stream, EVM values are minimum when BS antennas are 128 and 256. For user 4, for all the modulation schemes, it is very clearly showing that EVM is proportionally decreasing with increase in number of antennas.
From the overall results carried at 39 GHz carrier frequency, it is clear that 256 BS antennas and higher order modulation schemes are preferred to have minimum EVM values for data transmissions. Figures 14, 15, 16 represent the radiation pattern of a signal transmitted with 64, 128 and 256 BS antennas respectively, it shows that the radiation beams are more sharp and focused with increasing number of BS antennas. It helps to improve SNR and minimize the errors at the Rx. Figure 17 shows the 16-QAM equalized symbol constellation/stream of a signal received at Rx with 64 BS antennas. It is observed that for user 3, the signal constellation points are more close and it leads to more errors at the Rx while signal detection.
Figures 18, 19, 20 represent RMS EVM values at 66 GHz carrier frequency with multiple BS antennas and various modulation schemes. At 66 GHz carrier frequency, minimum EVM is 0.4631% with 128 BS antennas and maximum EVM is 3.7507% noted when BS antennas are 64. For users 1 & 2 with given modulation scheme, EVM is increasing as number of BS antennas increases. Also, EVM is very slightly increasing as the order of modulation is increasing. For users 3 & 4, optimum performance is achieved with number of BS antennas is equal to 128 compared to 64, 256 number of antennas. For a given number of BS antennas, EVM is decreasing with increasing the order of modulation. Figures 21, 22, 23 represent the radiation patterns of a signal with multiple BS antennas and various modulation schemes. The patterns prove that with increasing number of BS antennas, sharpness of beams increases and helps to improve SNR at Rx and reduce error rates. Figure 24 shows the equalized symbol constellation/stream of 64-QAM modulation for a scattering channel with 64 BS antennas and it proves that deviations are minimum in the symbol points if the user maintains more number of independent data streams.
From the overall results at different carrier frequencies shown in Figs. 25, 26, 27, following observations are made: for given number of BS antennas, EVM is slightly increasing with order of modulation scheme. For a given modulation scheme, EVM is decreasing with increase in number of BS antennas. For a given number of BS antennas, EVM is increasing as carrier frequency increases. User 4 has optimal performance, it has lowest EVM and it is further decreasing at 66 GHz. User 3 has more EVM compared to all other users as it has only four receiving antennas with one data stream at UE.
5 Conclusions and Future Scope
The future demands of next-generation wireless networks can be addressed using mMIMO systems and mmWave technology. This paper has focused on the hybrid beamforming design in mMIMO systems at mmWave frequency bands aiming to achieve higher data rates that approaches fully-digital system with reduced hardware complexity. The performance of MU-mMIMO HB system is evaluated at 28 GHz, 39 GHz, and 66 GHz using “MIMO” and “Scattering” channels to mimic the channel behavior in real scenarios. The system parameters considered are number of Tx/Rx antennas, number of data streams per users, modulation schemes (modulation orders from 2 to 8). RMS EVM values and symbol constellations are measured for various combinations of BS and UE antennas, carrier frequencies, and modulation schemes to identify the best fit. Based on the results obtained, it has been shown that with optimal selection of precoding, combining weights HB performs better than a fully analog beamforming or digital beamforming, also HB with peak search is a very much suitable for simulating 128 × 8 MU-mMIMO system. The overall results show that usage of HB technique in MU-mMIMO wireless communication systems improves capacity by transmitting multiple independent streams simultaneously. The HB designs aiming at minimizing the EVM values and fine tuning of phase shifters resolution are considered as future research directions.
Availability of Data and Material (Data Transparency)
MATLAB Tool is used for simulations.
Code availability (Software Application or Custom Code)
Not Applicable.
Abbreviations
- 3GPP:
-
3rd Generation project partnership
- 5G:
-
Fifth generation
- BS:
-
Base station
- CSI:
-
Channel state information
- DFT:
-
Discrete Fourier transform
- DL:
-
Down link
- EE:
-
Energy efficiency
- EVM:
-
Error vector magnitude
- FD:
-
Full duplex
- HB:
-
Hybrid beamforming
- ISI:
-
Inter symbol interference
- LOS:
-
Line-of-sight
- MSE:
-
Mean square error
- NR:
-
New radio
- OFDM:
-
Orthogonal frequency division multiplexing
- OMP:
-
Orthogonal matching pursuit
- RF:
-
Radio frequency
- RMS:
-
Root mean square
- Rx:
-
Receiver
- SDMA:
-
Spatial division multiple access
- SE:
-
Spectral efficiency
- SIC:
-
Successive interference cancellation
- SINR:
-
Signal-to-interference-plus-noise ratio
- SISO:
-
Single-input and single-output
- SNR:
-
Signal to noise ratio
- Tx:
-
Transmitter
- UE:
-
User equipment
- UL:
-
Uplink
- ZF:
-
Zero forcing
References
Molisch, A. F., Ratnam, V. V., Han, S., Li, Z., Nguyen, S. L. H., Li, V., & Haneda, K. (2017). Hybrid beamforming for massive MIMO: A survey. IEEE Communications Magazine, 55(9), 134–141. https://doi.org/10.1109/MCOM.2017.1600400
Dilli, R. (2020). Analysis of 5G Wireless Systems in FR1 and FR2 Frequency Bands, In 2020 2nd International Conference on Innovative Mechanisms for Industry Applications (ICIMIA). 767–772. Doi: https://doi.org/10.1109/ICIMIA48430.2020.9074973
Bouchenak, S., Merzougui, R., & Harrou, F. (2021). A hybrid beamforming Massive MIMO system for 5G: Performance assessment study, In: 2021 International Conference on Innovation and Intelligence for Informatics, Computing, and Technologies (3ICT). Doi: https://doi.org/10.1109/3ICT53449.2021.9581878
Singh, A., & Joshi, S. (2021). A Survey on Hybrid Beamforming in MmWave Massive MIMO System. Journal of Scientific Research, 65(1), 201–213. Doi: https://doi.org/10.37398/JSR.2021.650126
Wu, X., Liu, D., & Yin, F. (2018). Hybrid beamforming for multi-user massive MIMO systems. IEEE Transactions on Communications, 66(9), 3879–3891. https://doi.org/10.1109/TCOMM.2018.2829511
Dilli, R. (2021). Performance analysis of multi user massive MIMO hybrid beamforming systems at millimeter wave frequency bands. Wireless Networks, 27, 1925–1939. https://doi.org/10.1007/s11276-021-02546-w
Dilli, R., Ravi Chandra, M.L., & Deepthi, J. (2021). Ultra-Massive MIMO Technologies for 6G Wireless Networks. Engineered Science, 16, 308–318. Doi: https://doi.org/10.30919/es8d571
Hu, C., Liu, Y., Liao, L., & Zhang, R. (2019). Hybrid beamforming for multi-user MIMO with partially-connected RF architecture. IET Communications, 13(10), 1356–1363. https://doi.org/10.1049/iet-com.2018.5830
Dilli, R. (2021). Performance of multi-user massive MIMO in 5G NR networks at 28 GHz band. Telecommunications and Radio Engineering., 80(2), 61–74. https://doi.org/10.1615/TelecomRadEng.2021036864
Abdullah, Q., Salh, A., ModhShah, N.S., Abdullah, N., Audah, L., Hamzah, S. A., Farah, N., Aboali, M., & Nordin, S. (2020). A Brief Survey And Investigation Of Hybrid Beamforming For Millimeter Waves In 5G Massive MIMO Systems. Solid State Technology, 63(1), 1699–1710. Doi: https://doi.org/10.48550/arXiv.2105.00180
Li, Z., Han, S., Sangodoyin, S., Wang, R., & Molisch, A. F. (2018). Joint Optimization of hybrid beamforming for multi- user massive MIMO downlink. IEEE Transactions on Wireless Communications, 17(6), 3600–3614. https://doi.org/10.1109/TWC.2018.2808523
Salh, A., Audah, L., Shah, N. S. M., & Hamzah, S. A. (2019). Trade-off energy and spectral efficiency in a downlink massive MIMO system. Wireless Personal Communications, 106(2), 897–910. https://doi.org/10.1007/s11277-019-06194-4
Zhan, J., & Dong, X. (2021). Interference cancellation aided hybrid beamforming for mmwave multi-user massive MIMO systems. IEEE Transactions on Vehicular Technology, 70(3), 2322–2336. https://doi.org/10.1109/TVT.2021.3057547
Zhang, Y., Du, J., Chen, Y., Li, X., Rabie, K. M., & Khkrel, R. (2020). Dual-iterative hybrid beamforming design for millimeter-wave massive multi-user MIMO systems with sub-connected structure. IEEE Transactions on Vehicular Technology, 69(11), 13482–13496. https://doi.org/10.1109/TVT.2020.3029080
Zhang, Y., Du, J., Chen, Y., Li, X., Rabie, K. M., & Kharel, R. (2020). Near-optimal design for hybrid beamforming in mmwave massive multi-user MIMO systems. IEEE Access, 8, 129153–129168. https://doi.org/10.1109/ACCESS.2020.3009238
Li, Z., Han, S., & Molisch, A. F. (2016). Hybrid beamforming design for millimeter-wave multi-user massive MIMO downlink. In IEEE International Conference on Communications (ICC), 22–27,. https://doi.org/10.1109/ICC.2016.7510845
Ayach, O. E., Rajagopal, S., Abu-Surra, S., Pi, Z., & Heath, R. W. (2014). spatially sparse precoding in millimeter wave MIMO systems. IEEE Transactions on Wireless Communications, 13(3), 1499–1513. https://doi.org/10.1109/TWC.2014.011714.130846
Bjornson, E., Hoydis, J., & Sanguinetti, L. (2017). Massive MIMO networks: Spectral, energy, and hardware efficiency. Foundations and Trends in Signal Processing, 11(3–4), 154–655. https://doi.org/10.1561/2000000093
Spencer, Q. H., Swindlehurst, A. L., & Haardt, M. (2004). Zero-forcing methods for downlink spatial multiplexing in multiuser MIMO channels. IEEE Transactions on Signal Processing, 52(2), 461–471. https://doi.org/10.1109/TSP.2003.821107
Shui, D. S., Foschini, G. J., Gans, M. J., & Kahn, J. M. (2000). Fading correlation and its effect on the capacity of multielement antenna systems. IEEE Transactions on Communications, 48(3), 502–513. https://doi.org/10.1109/26.837052
Adhikary, A., Nam, J., Ahn, J., & Caire, G. (2013). Joint spatial division and multiplexing—The large-scale array regime. IEEE Transactions on Information Theory, 59(10), 6441–6463. https://doi.org/10.1109/TIT.2013.2269476
Lin, T., Cong, J., Zhu, Y., Zhang, J., & Letaief, K. B. (2019). Hybrid beamforming for millimeter wave systems using the MMSE criterion. IEEE Transactions on Communications, 67(5), 3693–3708. https://doi.org/10.1109/TCOMM.2019.2893632
Sun, S., Rappaport, T. S., Heath, R. W., Nix, A., & Rangan, S. (2014). MIMO for millimeter-wave wireless communications: Beamforming, spatial multiplexing, or both? IEEE Communications Magazine, 52(12), 110–121. https://doi.org/10.1109/MCOM.2014.6979962
Ratnam, V. V., Molisch, A. F., Bursalioglu, O. Y., & Papadopoulos, H. C. (2018). Hybrid beamforming with selection for multiuser massive MIMO systems. IEEE Transactions on Signal Processing, 66(15), 4105–4120. https://doi.org/10.1109/TSP.2018.2838557
Ning, B., Chen, Z., Chen, W., Du, Y., & Fang, J. (2021). Terahertz multi-user massive mimo with intelligent reflecting surface: Beam training and hybrid beamforming. IEEE Transactions on Vehicular Technology, 70(2), 1376–1393. https://doi.org/10.1109/TVT.2021.3052074
Sheemar, C. K., Thomas, C. K., & Slock, D. (2022). Practical hybrid beamforming for millimeter wave massive MIMO full duplex with limited dynamic range. IEEE Open Journal of the Communications Society, 3, 127–143. https://doi.org/10.1109/OJCOMS.2022.3140422
Koc, A., Masmoudi, A., & Le-Ngoc, T. (2019). Hybrid Beamforming for Uniform Circular Arrays in Multi-User Massive MIMO Systems. In: 2019 IEEE Canadian Conference of Electrical and Computer Engineering (CCECE), 1–4. Doi: https://doi.org/10.1109/CCECE.2019.8861837
Yüzgeçcioğlu, M., & Jorswieck, E. (2017). Hybrid beamforming with spatial modulation in multi-user massive MIMO mmWave networks. In: 2017 IEEE 28th Annual International Symposium on Personal, Indoor, and Mobile Radio Communications (PIMRC), 1–6. Doi: https://doi.org/10.1109/PIMRC.2017.8292710
Huong, T. T. T., & Hiep, P. T. (2022). Joint precoder and decoder for MIMO dual-hop relay systems in delay spread channels. Wireless Personal Communications, 124, 1247–1261. https://doi.org/10.1007/s11277-021-09404-0
Zang, G., Cui, Y., Cheng, H. V., Yang, F., Ding, L., & Liu, H. (2019). Optimal hybrid beamforming for multiuser massive mimo systems with individual SINR constraints. IEEE Wireless Communications Letters, 8(2), 532–535. https://doi.org/10.1109/LWC.2018.2878766
Nonaka, N., Muraoka, K., Suyama, S., & Okumura, Y. (2019). Two-Step User Selection Algorithm for Multi-User Massive MIMO with Hybrid Beamforming. In: 2019 22nd International Symposium on Wireless Personal Multimedia Communications (WPMC), 1–5. Doi: https://doi.org/10.1109/WPMC48795.2019.9096171
Du, J., Xu, W., Zhao, C., & Vandendorpe, L. (2019). Weighted spectral efficiency optimization for hybrid beamforming in multiuser massive MIMO-OFDM systems. IEEE Transactions on Vehicular Technology, 68(10), 9698–9712. https://doi.org/10.1109/TVT.2019.2932128
Song, N., Yang, T., & Sun, H. (2019). Efficient Hybrid Beamforming for Relay Assisted Millimeter-Wave Multi-User Massive MIMO. In: 2019 IEEE Wireless Communications and Networking Conference (WCNC), 1–6. Doi: https://doi.org/10.1109/WCNC.2019.8886016
Gherekhloo, S., Ardah, K., & Haardt, M. (2020). Hybrid Beamforming Design for Downlink MU-MIMO-OFDM Doi: Millimeter- Wave Systems. In: 2020 IEEE 11th Sensor Array and Multichannel Signal Processing Workshop (SAM), 1–5. https://doi.org/10.1109/SAM48682.2020.9104405
Won-Seok, L., & Hyoung-Kyu, S. (2021). Efficient channel feedback scheme for multi-user MIMO hybrid beamforming systems. Sensors, 21(16), 1–17. https://doi.org/10.3390/s21165298
Salh, A., Audah, L., Mohd Shah, N. S., & Shipun, A. H. (2020). Energy-efficient power allocation with hybrid beamforming for millimetre-wave 5G massive MIMO system. Wireless Personal Communications, 115, 43–59. https://doi.org/10.1007/s11277-020-07559-w
Ahmed, S. A., Ayoob, S.A., & Al Janaby, A.O. (2021). Comparative Performance of mmWave 5G System for many Beamforming Methods. In: 2nd International Multi-Disciplinary Conference Theme: Integrated Sciences and Technologies, IMDC-IST 2021, 1–7. Doi: https://doi.org/10.4108/eai.7-9-2021.2314818
Nguyen, N. T., & Lee, K. (2020). Unequally sub-connected architecture for hybrid beamforming in massive MIMO systems. IEEE Transactions on Wireless Communications, 19(2), 1127–1140. https://doi.org/10.1109/TWC.2019.2951174
Mahendra, R., Mohammed, S. K., & Mallik, R. K. (2021). Compensation of transmitter IQ imbalance in multi-user hybrid beamforming systems. IEEE Access, 9, 98231–98248. https://doi.org/10.1109/ACCESS.2021.3094560
Huang, S., Ye, Y., & Xiao, M. (2020). Hybrid beamforming for millimeter wave multi-user MIMO systems using learning machine. IEEE Wireless Communications Letters, 9(11), 1914–1918. https://doi.org/10.1109/LWC.2020.3007990
Ahmed, I., Shahid, M. K., Khammari, H., & Masud, M. (2021). Machine learning based beam selection with low complexity hybrid beamforming design for 5G massive MIMO systems. IEEE Transactions on Green Communications and Networking, 5(4), 2160–2173. https://doi.org/10.1109/TGCN.2021.3093439
Huang, S., Ye, Y., & Xiao, M. (2021). Learning based hybrid beamforming design for full-duplex millimeter wave systems. IEEE Transactions on Cognitive Communications and Networking, 7(1), 120–132. https://doi.org/10.1109/TCCN.2020.3019604
Elbir, A. M., & Coleri, S. (2020). Federated learning for hybrid beamforming in mm-wave massive MIMO. IEEE Communications Letters, 24(12), 2795–2799. https://doi.org/10.1109/LCOMM.2020.3019312
Lin, T., & Zhu, Y. (2020). Beamforming design for large-scale antenna arrays using deep learning. IEEE Wireless Communications Letters, 9(1), 103–107. https://doi.org/10.1109/LWC.2019.2943466
Zhai, X., Chen, X., Xu, J., & Kwan Ng, D. W. (2021). Hybrid beamforming for massive MIMO over-the-air computation. IEEE Transactions on Communications, 69(4), 2737–2751. https://doi.org/10.1109/TCOMM.2021.3051397
Darabi, M., Cirik, A. C., & Lampe, L. (2021). Transceiver design in millimeter wave full-duplex multi-user massive MIMO communication systems. IEEE Access, 9, 165394–165408. https://doi.org/10.1109/ACCESS.2021.3135758
Khalid, F. (2019). Hybrid beamforming for millimeter wave massive multiuser MIMO systems using regularized channel diagonalization. IEEE Wireless Communications Letters, 8(3), 705–708. https://doi.org/10.1109/LWC.2018.2886882
Ge, X., Sun, Y., Gharavi, H., & Thompson, J. (2018). Joint optimization of computation and communication power in multi-user massive MIMO systems. IEEE Transactions on Wireless Communications, 17(6), 4051–4063. https://doi.org/10.1109/TWC.2018.2819653
Feng, Y., & Kim, S. C. (2017). Hybrid precoding based on matrix-adaptive method for multiuser large-scale antenna arrays. PLoS ONE, 12(12), 1–9. https://doi.org/10.1371/journal.pone.0188723
Zheng, Z., & Gharavi, H. (2019). Spectral and energy efficiencies of millimeter wave MIMO with configurable hybrid precoding. IEEE transactions on vehicular technology, 68(6), 5732–5746. https://doi.org/10.1109/TVT.2019.2909829
Liu, W., Hou, X., Kishiyama, Y., Chen, L., & Asai, T. (2020). Transform domain precoding (TDP) for 5G evolution and 6G. IEEE Signal Processing Letters, 27, 1145–1149. https://doi.org/10.1109/LSP.2020.3004071
Funding
Open access funding provided by Manipal Academy of Higher Education, Manipal. Not applicable.
Author information
Authors and Affiliations
Contributions
This manuscript reports on a single cell downlink “Multi User-massive Multi–Input–Multi–Output (MU-mMIMO)” hybrid beamforming communication system with multiple data streams per user and emphasize partitioning of precoding at transmitter and combining at receiver. This paper gives performance analysis of a MU-mMIMO Hybrid beamforming communications system at 28 GHz, 39 GHz of FR2 frequency bands as well as at 66 GHz and compares the performance of modulation schemes having different modulation order. Simulation results include receive constellation of the equalized symbols that gives qualitative analysis and RMS EVM gives quantitative analysis. This is significant work because mMIMO communication is an essential requirement for future generation wireless communication networks and hybrid beamforming is a crucial design component in mMIMO wireless systems that gives more efficiency in terms of hardware cost and energy. I believe that MU-mMIMO operation will be much more popular for 5G NR, considering the wide deployment of mMIMO in major NR bands.
Corresponding author
Ethics declarations
Conflict of interest
I have no conflicts of interest to disclose.
Additional information
Publisher's Note
Springer Nature remains neutral with regard to jurisdictional claims in published maps and institutional affiliations.
Rights and permissions
Open Access This article is licensed under a Creative Commons Attribution 4.0 International License, which permits use, sharing, adaptation, distribution and reproduction in any medium or format, as long as you give appropriate credit to the original author(s) and the source, provide a link to the Creative Commons licence, and indicate if changes were made. The images or other third party material in this article are included in the article's Creative Commons licence, unless indicated otherwise in a credit line to the material. If material is not included in the article's Creative Commons licence and your intended use is not permitted by statutory regulation or exceeds the permitted use, you will need to obtain permission directly from the copyright holder. To view a copy of this licence, visit http://creativecommons.org/licenses/by/4.0/.
About this article
Cite this article
Dilli, R. Hybrid Beamforming in 5G NR Networks Using Multi User Massive MIMO at FR2 Frequency Bands. Wireless Pers Commun 127, 3677–3709 (2022). https://doi.org/10.1007/s11277-022-09952-z
Accepted:
Published:
Issue Date:
DOI: https://doi.org/10.1007/s11277-022-09952-z