Abstract
Economic growth in Spain has largely relied on certain water-intensive sectors including construction, leisure, and agriculture; but considerable heterogeneity is found across the country’s regions, both in terms of water stress and economic structure. Using Data Envelopment Analysis and a panel Tobit model, we analyze the determinants of water efficiency in Spain, differentiating by groups of agents (companies, households, and municipalities) and paying particular attention to inter-regional differences and specifically the sectoral structure of the various regions. To the best of our knowledge, such an analysis has never before been conducted with a focus on Spain’s economy. Moreover, we emphasize the importance of considering the circular aspects of water management by including treated wastewater and recycled water in our analysis. We argue that this approach provides more credible and accurate measures of efficiency as well as more robust results around the key driving and explanatory factors. Our analysis reveals that water efficiency has a very significant inertia component with respect to one-year and even two-year lags, and this is found to react positively to water scarcity. Moreover, water efficiency depends on income in a nonlinear way, and it is sensitive to the economic structures of the diverse regions, with more efficient regions tending to export more at the national level. In terms of policy implications, our results provide an empirical basis from which to advocate for water tariffication as a means to spur efficiency.
Similar content being viewed by others
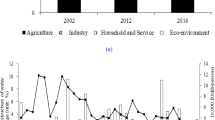
1 Introduction
Water, a critical resource across all realms (economic, social, political) is increasingly a matter of concern due to scarcity of supply and population growth, with great potential for future conflict. In this context, efficient water management becomes a crucial element in economic growth, social stability, and environmental sustainability (Berbel and Esteban 2019; Garrick et al. 2020). The inefficient utilization of resources – particularly water itself – further exacerbates issues of scarcity, while a transition to more efficient practices can help alleviate this problem.
Traditionally, the economy in Spain has relied on certain very water-intensive sectors including construction, leisure, and agriculture (Pulido et al. 2020), all of which may contribute to aggravating the problem of water stress. Even so, the situation is not uniform throughout Spain, as distinct regions are subject to different weather conditions and economic structures. To illustrate some of these differences, Fig. 1 shows the water-use levels per capita in the various regions of Spain.
In this research, we analyze water efficiency in Spain, paying close attention to regional differences, and we attempt to identify the factors that can best explain the differences noted. In terms of methodology, we employ Data Envelopment Analysis (DEA) to measure efficiency as well as an econometric panel-data Tobit model to identify the driving forces behind differences in efficiency. At the same time, we emphasize the circular aspects of water management.
When addressing efficiency, it is useful to bear in mind the circular nature of water. The total mass of water on Earth remains constant, but the precise locations of major water reservoirs (as in ice, in rivers and oceans, in the atmosphere) are in constant change. This circularity constitutes an essential aspect of water-use efficiency and sustainability.
To the best of our knowledge, the only study offering comparisons of Spain’s regions in terms of water efficiency is André et al. (2023), where different groups of water users (households, the productive sector, municipalities) are considered and attention is paid to the cyclical nature of water management. The first portion of the present research resembles André et al. (2023) in that it draws from the same database and deals with water efficiency in Spain’s regions. Moreover, our analysis is performed both with and without the variables that capture the circular nature of water (treated wastewater and reused water), in order to assess how efficiency may vary when examining these elements. One important difference between the two studies is that André et al. (2023) focus on the measurement of efficiency and perform a separate analysis of the components of efficiency resulting from a DEA model: the multiplicative (or radial) component and the additive (or slack) component. Because we are more concerned with the driving factors behind geographic differences, we instead compute an overall measurement of water efficiency. This is done by comparing the observed amount of water consumed with its projection on the ‘efficient frontier’ (see Methodology section) after accounting for any sources of inefficiency.
The main difference from the study by André et al. (2023) is that our research is not merely or mainly concerned with the measurement of efficiency but also with the driving forces behind the differences observed across regions. This analysis is conducted by means of a Tobit (or censored regression) model, selected due to the bounded nature of the explained variable (efficiency index) between 0 and 1. As to explanatory variables, we consider the income level, the weights of diverse sectors on economic activity, degrees of economic openness, plus several variables specifically related to water management, such as a measurement of water availability, the shares of water used by different groups of agents, a relative measurement of reused water, and the price of water. We conclude that the estimated effect of these variables differs greatly depending on whether or not the regional capacity to treat and reuse wastewater is considered.
In summary, this study addresses the following research questions. First, we seek to provide an operational measurement of water efficiency that can be used to compare Spain’s regions at the aggregate level. Second, using that measurement, we enquire as to which regions have proven efficient in terms of water use and management during the period observed. Third, we attempt to identify the main factors that drive the observed differences in efficiency. We are specifically interested in the impact of economic growth, economic structure, trade openness, and water abundance on efficiency. In terms of policy, we consider whether the pricing of water can spur efficiency. Fourth, we examine the relevance of explicitly considering the circular aspects of water management, such as treatment and reuse, and how such elements affect our measurement of efficiency and the main driving factors behind it.
2 Literature Review
Water scarcity and water efficiency have been the objects of recent attention in social, political, and scientific terms. For example, Jin et al. (2019) analyze the green total factor efficiency of industrial water resources in 30 Chinese provinces and the connection of such an efficiency indicator with several socio-economic variables. Cheng et al. (2023) present an evaluation of a projectFootnote 1 aimed at alleviating water scarcity in northern China and improving water-use efficiency. Bernabé-Crespo and Loáiciga (2024) analyze the policy options followed in three territories (Southeastern Spain, Los Angeles, and Sydney) to address water supply and reduce water stress. Ma et al. (2023) employ the Super-SBM model to calculate the city-water resource green efficiency of the Yangtze River Economic Belt.
Many authors have used DEA to study water efficiency in numerous countries and regions, including China (Liu et al. 2013; Deng et al. 2016; Zhang et al. 2020; Jiang et al. 2024) , India (Raju and Nagesh Kumar 2013; Veettil et al. 2013) , Turkey (Yilmaz et al. 2009), Kenya (Njiraini and Guthiga 2013), Southern Alberta (Ali and Klein 2014), and Australia (Azad et al. 2015). DEA has also been used to calculate water supply efficiency in Italy (Guerrini et al. 2013), Germany (Zschille and Walter 2012), France (Lannier and Porcher 2014), and Australia (Ananda 2014; Azad and Ancev 2014).
There are several studies on water efficiency in Spain using DEA, but very few perform comparisons across regions as we do (except André et al. 2023, as explained in the Introduction). For example, González-Gómez et al. (2013) apply DEA to compare the efficiency of different management approaches for water services in Southern Spain. Hernández-Sancho et al. (2011) and Hernández-Chover et al. (2018) focus on the efficiency of wastewater plants at the municipal level. García-Sánchez (2006) evaluates the efficiency of water supply, concluding that observed scale inefficiency is higher than technical inefficiency.
In terms of methodology, our study is closest to several papers that combine water efficiency measurements and Tobit models. For example, Hu et al. (2006) perform an efficiency analysis in Chinese regions and obtain a U-shaped relation between total-factor efficiency and per capita real income. Deng et al. (2016) estimate the water efficiency of 31 provinces in China using a slack-based measure (SBM) DEA model, and they identify certain socio-economic variables driving water efficiency. Qu (2020) use a DEA and Tobit approach to evaluate the current status of water pollution in Shenzhen city, China. Sala-Garrido et al. (2023) employ cross-efficiency DEA techniques to assess a sample of water companies and identify both customer density and ownership of water companies as key drivers of economic and environmental efficiency. Similar approaches are followed by Xuedong et al. (2021) and Wang et al. (2018).
Tobit models have been applied to diverse research fields, whether in combination with DEA or other efficiency measurement techniques, mostly in China. For instance, Rong et al. (2022) measure regional ecological efficiency and estimate how this depends on green finance and natural resource abundance. Xu and Wang (2020) analyze the urban agglomeration of 30 cities in five provinces of Zhongyuan. Li and Ma (2015) analyze 30 provinces in mainland China to compare industrial water efficiency. Liang et al. (2021) measure the efficiency of water use (WU), wastewater treatment (WT), and the overall water resource systems for eleven provinces in western China. Zhou et al. (2019) examine eco-efficiency using DEA for 48 cities in Bohai Rim, China. Song et al. (2018) apply the undesirable-output-based Malmquist-Luenberger productivity index to 30 Chinese provinces and study the factors that affect water resource efficiency.
3 Methodology
3.1 DEA Model
Our measurement of water efficiency is implemented by means of an input-oriented, constant-returns-to-scale (CRS) DEA model (see for example Charnes et al. 1978). Conceptually, DEA builds an ‘efficient frontier’ – the envelope of the observed Decision Making Units (DMUs) – so that the efficient units are on the frontier and the inefficient ones are inside the feasible set. Each inefficient DMU is radially projected onto the frontier to determine whether it is possible to decrease all its inputs proportionally without reducing the outputs (radial inefficiency). A second type of inefficiency can appear when, from the radial projection, any slacks are observed – i.e., if it is possible to further reduce certain input(s) without increasing any other or reducing the output. In practice, these two components of efficiency can be disentangled by: 1) solving two sequential optimization problems; or 2) minimizing a weighted combination of the radial reduction and the slacks (as in this study), assigning to the latter a relatively very small weight commonly known as a non-Archimedean number (in our case, \(\varepsilon =0.0001\)). Specifically, we solve problem [1] below for each different DMU, \(o\), (in our case, for every region) and for every year in the sample:
where \({x}_{ij}\) and \({y}_{rj}\) represent the amount of the i-th input and the r-th output of DMU \(j\). Parameter \(\theta\), which is bounded by construction between \(0\) and \(1\), is the radial measure of efficiency, as it determines in what proportion the inputs of region \(o\) can be equi-proportionally reduced when being projected onto the efficient frontier. The frontier is built as a linear weighted combination (using ponderations λj) of all the efficient regions (an efficient region is projected onto itself). The slack variables assigned to the inputs and the outputs are denoted as \({s}_{i}^{-}\), \(i=\mathrm{1,2},\dots m\), and \({s}_{i}^{+}\), \(r=\mathrm{1,2},\dots s\), respectively.
Table 1 shows our inputs and outputs (the only output considered is GDP). As regards inputs, we first present an initial framework (which we refer to as the untreated version) that includes only capital, labor, energy use, and water used by different groups of economic agents (households, firms, municipalities). Next we perform the so-called treated version by introducing two additional inputs, treated wastewater and reused water, as an approximation to the circular use of water, permitting us to then evaluate the impact of considering these circular aspects in analysis (see André et al. 2023 for details).
While reused water is a substitute for freshwater and can thus be understood as a conventional input, water treatment can be seen as a positive economic activity, because the alternative (leaving water untreated) is prone to causing negative impacts. One way to account for this fact would be to include it as an output; we instead follow André et al. (2023) and include it as an “undesirable input” (in the sense introduced by Seiford and Zhu (2002) and by Lewis and Sexton (2004), indicating that decision-makers may want to maximize rather than minimize this input).
3.2 Measuring Water Efficiency
For a certain region (\(j\)) and a given year \((t)\), we denote as \({w}_{jkt}\) the observed consumption of water by a certain group of agents (\(k\)= households, firms, municipalities) and as \({\widehat{w}}_{jkt}\) the objective consumption of water, defined as the efficient value after the observed value has been projected radially onto the frontier and any slack has been subtracted. We then define the potential water savings, \(PWS\), as the difference between the two quantities:
The overall water inefficiency ratio, \(OWIR\) (where “overall” refers to the fact that we are jointly considering the radial inefficiency component plus the slack), is defined as:
The indicators introduced in (2) and (3) can be respectively considered as absolute and relative measures of inefficiency in water use for each group of agents \(k\), in a given region \(j\) at time \(t\), indicating the maximum amount (proportion) in which water use could conceivably be reduced by moving toward the frontier. A value \({PWS}_{jkt}=0\), or equivalently \(O{WIR}_{jkt}=0\), indicates that the water consumption of agent \(k\) in region \(j\) is efficient in year \(t\), in the sense that there is no potential for reducing water consumption without reducing output or increasing other inputs. The aggregate potential water savings of region \(j\) at time t, \({PWS}_{jt}\), can be computed as the sum of potential water savings from all three groups of agents:
The aggregate \(OWIR\) is obtained by dividing the aggregate potential water savings, as defined in (4), by total observed water consumption:
From (5) we obtain the endogenous variable in our Tobit model, which is the complementary value of \(OWIR\): overall water efficiency ratio, \(OWER\):
By construction, \(OWER\) is bounded between 0 and 1, where 1 means that the region under consideration is positioned at the frontier and is thus efficient at time \(t\) in terms of water use.
3.3 Identifying the Driving Forces Behind Water Efficiency
We estimate a panel data Tobit model with the following structure (for the aggregate versionFootnote 2):
where \({OWER}_{jt}\) is the observable variable, computed according to (6). \(\beta\) is a vector of parameters, \({X}_{jt}\) is a vector of explanatory variables, \({\varepsilon }_{jt}\) is an independent, normal error term with constant variance \(0\) mean. \(N\times T\) is the sample size, with \(N\) units observed at \(T\) periods of time. \({E}_{jt}^{*}\) is a latent variable that can be observed only when it takes a value between \(0\) and \(1\). According to Shuai and Fan (2020), the maximum likelihood estimation of a fixed-effects Tobit model is biased and inconsistent; we therefore estimate the random-effects version. We estimate four versions of model (7): one for aggregate water use and one for each group of agents (households, firms, municipalities).
Although measurements of efficiency are allegedly subject to circumstantial factors, efficiency has an important structural component that is prone to constancy through time. In order to capture this component of inertia, we include as regressors two lags of the explained variable. Additionally, we include a selection of socio-economic variables (see Table 2 for details):
-
First, we consider the log of per capita GDP to check whether wealthier and more developed regions tend to be more efficient. We include this in linear and quadratic terms to capture any concavity or convexity in the relationship.
-
Second, we consider the activity structure of each region by including as explanatory variables the percentage of different activity sectors on the gross value added. The weight of the real estate (RE) sector is the omitted variable.
-
Third, we include the trade activity (imports and exports) of each region with respect to the rest of Spain and the world, in order to check how trade openness impacts water efficiency.
-
The last group includes variables specifically related to water management. First, a measurement of per capita water abundance, “Drinking water available and ready for purification”, is introduced to capture (the inverse of) water stress. The hypothesis is that water scarcity generates incentives to save water and become more efficient. We also include the proportion (‘share’) of the different agents in total consumption, where the omitted group is households, to avoid collinearity. We also include the ratio of reused water to treated wastewater as an indicator of how regions deal with wastewater. Finally, the price of water is expected to foster efficiency.
3.4 Data Sources
The sample includes annual data at the regional level spanning from 2001 to 2018, where the availability of information on water proved to be a limiting factor. The data on inter-regional trade are obtained from the C-Interreg project of CEPREDE Economic Prediction Center.Footnote 3 Due to the lack of data on energy use at the regional level, we have instead taken electricity demand, obtained from the Spanish Electricity Grid Database (REE).Footnote 4 The capital stock is obtained from the Valencian Institute of Economic Research (Instituto Valenciano de Investigaciones Económicas, IVIE).Footnote 5 The remaining data are obtained from the Spanish Statistical Institute (Instituto Nacional de Estadística, INE).Footnote 6 Specifically, the data on GDP, labor input, and the participation of the different activity sectors are retrieved from the Spanish Regional Accounts, while data on water consumption is taken from the Satellite Accounts (“Statistics on water supply and sanitation”). All of the variables related to water were missing values for the years 2015 and 2017, which we have interpolated linearly. Table A1 in the Appendix presents additional statistical information about the variables included in the Tobit model.
4 Results: Efficiency Measures
As a first step, we arrive at our efficiency measurements by solving the two versions (untreated and treated) of our DEA model. For simplicity we discuss only the aggregate value of OWER, but the conclusions are qualitatively similar when observing the agent-specific results.
In both versions (treated and untreated), our results reveal that there are just four regions (Madrid, Cataluña, Islas Canarias, and Islas Baleares) that qualify as efficient \(\left(O{WER}_{jt}=1\right)\) for all the sample years. The remaining regions qualify as inefficient for one or more years. The most apparent effect from including the water circular variables (moving from the untreated to the treated version) is to increase the efficiency measurements (or, alternatively, to reduce the observed differences in efficiency across regions). In average terms, OWERit increases from 0.42 to 0.59. In terms of individual efficiency, in the untreated version, and apart from the four efficient regions, only Andalucía displays OWERit=1 in two years of the sample. The remaining regions exhibit some positive degree of inefficiency for each and every year of the sample. In the second exercise, however, and apart from the four consistently efficient regions, some regions appear to be efficient through several years of the sample (Navarra, 13 years; Andalucía and Extremadura, 12 years; Galicia, 10 years; Aragón and Cantabria, 5 years; País Vasco and La Rioja, 4 years).
5 Results: Efficiency Drivers
Tables 3 and 4 present the estimated parameters of the Tobit model applied to the untreated and treated scenarios and, for each scenario, four versions of the same model addressing efficiency at the aggregate level and for each group of agents. The regressions are run independently. We begin by trying as regressors all the variables introduced in Subsection 2.3, and we iteratively eliminate the non-significant variables, starting with those with the highest p-value, until we select the best model by keeping all the significant variables.
By comparing the two tables, we can confirm that differing methods of measuring efficiency have a noticeable impact on how the measurements respond to different driving forces. In the Discussion section below, we reflect on and analyze these differences more deeply.
Detected as being an important feature of our estimates is strong inertia over time, suggesting that efficiency is to some extent determined by structural and long-term issues. Looking first at the untreated scenario, in the aggregate, the first-order autoregressive coefficient is around \(0.8\), which is very similar to the value for households, but this is slightly weaker for businesses and municipalities, with values just below \(0.7\). The second-period lag is weaker but still significant. In the treated scenario, although inertia is still noticeable, the autoregressive parameters are lower than in the untreated scenario, especially the first lag, with values ranging between \(0.45\) and \(0.56\) for all four versions of the model.
One notable difference between the two models relates to the impact of income. In the untreated version, an inverted U-shaped relationship is observed at the aggregate level and for households (but not firms or municipalities), suggesting that economic growth tends to foster efficiency at the household level, but only to a certain point. Conversely, a U-shaped relationship – as opposed to an inverted U – is observed in the treated version, which in this case holds consistent across all four versions of the model. This U-shaped effect seems to indicate a negative effect on efficiency for low values of income, with a reversed sign for high values. As a possible interpretation of this difference, one speculation is that short-run growth can improve traditional water management to a certain extent, while improvements in more circular and sustainable pattern are to be expected in the long run, when economic growth and associated technological changes are sufficiently consolidated. Nevertheless, the common message drawn from both estimates seems to be that the effect of income on water efficiency does not present a clear sign, and complex first-order and second-order effects that affect water management are probably present along the economic growth process.
As regards the effect of economic structure on efficiency, because Real Estate (RE) is our omitted sector, all the parameters associated with the shares of other sectors must be interpreted in terms relative to RE. Again, the results differ between the two versions, although some coincidences are also found. Notably, the Primary sector as well as “Leisure and wholesale-retail trade” and “Professional and scientific activities” show positive coefficients in both versions whenever they are significant.
The results of trade are also quite different between the two versions. In the untreated model, trade seems to matter only for the private and not for the public sector. Moreover, international exports (but not national exports) appear to have a negative impact on efficiency, whereas national imports (but not international imports) have a positive impact. In the treated model, international trade does not seem to matter – only inter-regional trade does. Specifically, an increase in inter-regional exports has a positive effect, while imports have scarcely any effect (with a negative, weakly significant effect being perceived only in municipalities).
Regarding \({W}_{pc}\), our measure of per capita water availability, in the untreated scenario we found the expected effect – i.e., regions with more available water tend to be less efficient. The natural interpretation is that water scarcity increases the incentives to foster efficiency or, alternatively, that water abundance may deter efficiency. There are, however, two symptoms that indicate that this effect may not be sufficiently strong. First, in the untreated scenario, this effect seems to be driven by the private sector alone and is not detected in the municipalities regression. More importantly, once we refine the efficiency measurement in the treated scenario, it does not appear to be significant.
The two versions also differ in terms of the shares of different agents in water consumption. In the untreated scenario, we detect a negative effect of the share of municipal water in water efficiency only for municipalities, although this effect is not observed in the aggregate. Once we account for circularity, we observe a kind of mirror effect in the sense that the share of firms – which is the ‘other’ variable, households having been omitted – is the only significant one, and this occurs in the regressions for private sectors (households and firms) but not for municipalities.
The share of reused water consumed from the treated wastewater generated is revealed to have a negative sign in terms of efficiency; finally, the price of water appears to have a positive and significant sign, but this is only detected in the treated scenario.
6 Discussion and Policy Implications
This section discusses the interpretation and implications of the results, paying particular attention to the differences between the treated and untreated versions, followed by comments on the sample and the difficulties encountered in obtaining updated information.
Our treated version has been set out to account for circularity, which (as argued in the Introduction) is an essential characteristic of water when considering efficiency of management and sustainability. Note that according to the untreated setting, economic agents do not consider a fundamental part of the inputs defining their production processes, and in any case they focus solely on available freshwater. The treated framework instead incorporates the capacity of economic agents to increase their water resources as part of their production processes. Accounting for these circular processes and incentives constitutes a non-negligible difference, and for that reason we consider the second version to be more comprehensive, accurate, and credible.
Section 3 shows that inclusion of the circular variables results in an increase in average efficiency for the whole sample. In order to provide a more detailed vision, Fig. 2 shows the time evolution (across regions) of the average value of \(OWER\) in both versions. This figure indicates that the efficiency measurements in the treated version are larger on average, both in the whole panel and also year-by-year. Another fact illustrated in the graph is that while the values for untreated average efficiency are relatively stable over time, the treated values display more variability, permitting us to clearly detect an evolution. In the years of financial crises (2007 and 2008) we see a clear rise in average efficiency, arguably because all regions were subject to a common exogenous market pressure. The effect of this shock seems to last for a few years and then progressively weaken.
As an additional argument in support of the treated version, the comparison of Tobit estimates is here found to be more robust and consistent, in several aspects. Note first that in the untreated scenario (Table 3), water efficiency in the private sector components (households and firms) seems to respond differently to the driving factors than does the public sector component (municipalities), whereas in the treated scenario, the public sector component (municipalities) seems to behave much more in line with the private sectors. This can be seen as a sign of robustness, as we observe essentially the same effects whatever the specific component being considered.
Second, we have shown that inertia over time seems to be somewhat weaker in the treated version. One way to interpret this result is that, as this second measure is more sophisticated (and arguably accurate) than the first, the model is more capable of detecting how efficiency responds to different socio-economic variables, with a smaller portion being left to the inertia component alone.
As for the effects of economic structure on efficiency, while some positive and some negative effects are detected in the untreated model, in the treated version all significant impacts are found to be consistently positive – another sign of robustness and an indication that the role of the RE sector in the economy tends to generate a non-positive effect (positive in some cases and insignificant in other cases) on water efficiency, at both the aggregate and disaggregate levels.
As regards trade, the results in the treated version are also more consistent with what one would expect, at least in inter-regional trade (where international trade does not figure). Specifically, an increase in exports has a positive effect, and this is in line with the common belief that more efficient regions tend to export more.
The result regarding the share of reused water consumed from treated wastewater is also more robust in the treated scenario, in a double sense: first, the estimated value is higher, and second, this appears to be significant in all the regressions (and not only for municipalities, as in the untreated version). This result may seem counterintuitive, given that the ability to reuse recycled water may be seen as a sign of efficiency. However, the other side of this coin (probably the one showing up in these results) is that recycled water is a substitute for freshwater, and so this variable can be understood simply as an indicator of intensity of water consumption. Using more recycled water is more sustainable only if it allows a reduction in freshwater consumption, and this is not automatically implied by our indicator.
Finally, only in the treated scenario do we detect a positive sign for the price of water. This is in line with a priori economic intuition and has an important implication for policy: higher water prices should introduce an incentive for more efficient use.
The sample employed in this study is rather significantly limited by data non-availability. The most limiting factor has been the availability of regional water data, which is reported only after some considerable time. The most recent year for which official data was published for all the variables is 2020. An additional difficulty is that this information was published annually until 2014, when publication was reduced to once every two years. As a consequence, we lack some of the water variables for the years 2015, 2017, and 2019.
To take full advantage of all the available information and to build a balanced panel, we opted for interpolating the data for the years 2015 and 2017. We feel this approach to be justified given that the time evolution in these years was relatively smooth for the variables involved, and no atypical events were observed. Interpolation is therefore not expected to exert a drastic effect on the results. However, this situation was radically different for the years 2019 and 2020, which we have chosen to exclude from the sample. The reason for this exclusion is that 2020 was without question a strong outlier due to the COVID-19 pandemic and lockdown, and all economic indicators corresponding to that year display extremely atypical behavior.
In order to check the potential impact of this atypical character on our results, we repeated our analysis including data from 2020 (observed values) and 2019 (interpolated values). Figure 2 illustrates the impact of having included these years on the average evolution of our efficiency measurement, which clearly displays atypical behavior at the end of the series. We also confirmed that the Tobit estimates are highly sensitive to this enlargement. We therefore ultimately decided to adhere to our 2001–2018 sample and omit these distortionary values.
7 Conclusions and Further Research
The main contributions of this research are as follow. First, we have constructed a water efficiency indicator that aggregates the two components of efficiency (radial and slack) in Data Envelopment Analysis. Second, we have used that indicator to compare – for the first time in the literature – the various regions of Spain in terms of water efficiency. Third, we have identified the main economic driving forces behind the observed differences in water efficiency across regions. Fourth, we have emphasized the importance of considering the circular aspects of water, particularly in terms of wastewater treatment and reused water, and we have argued that taking this approach provides more robust and credible results.
As regards the most salient results, we first conclude that only four regions (Madrid, Cataluña, and the two island regions) have been consistently efficient over the entire period of analysis, whether or not one considers circularity. Efficiency displays strong inertia over time but is also sensitive to economic growth, economic structure, trade openness, and some water-specific variables such as abundance, the share consumed by certain agents, and the percentage of water reused.
Consideration of the circular aspects has an impact of increasing the average efficiency measurements. Moreover, when circularity is accounted for, we find significant differences in the estimated impact of some crucial variables, including income, economic structure, and trade, among others. We consider the treated measurement of efficiency and thus the associated estimates for the socio-economic drivers to be more credible and accurate. This belief is in line with the fact that the estimated results tend to be more robust – and in many cases more consistent with economic logic.
One specific result with important policy implications is that the price of water plays a crucial role in incentivizing efficiency in water management. Traditionally, water has been seen as (almost) free in Spain, with lower prices than other European countries, and this fact has been attracting increased attention. As a matter of fact, water governance and its impact on efficiency and growth is a very relevant and fruitful research field – see for example Pan et al. (2023) on the impact of water-rights trading. Our finding challenges the traditional perception of water as nearly free in Spain and provides empirical support to advocate for water tariffication as a means to foster water efficiency.
Future research should be aimed at delving into the time evolution of efficiency and providing a suitable framework by which to analyze the dynamic evolution of efficiency and its determinants. Connecting this analysis to economic growth and technological change would be another interesting line of research. Additionally, although we have addressed the heterogeneity across regions, there is significant scope for studying variation within regions. An analysis at the city or town level would reveal additional differences that might merit further research.
Data Availability
Data availability through author: a.buendia@ccee.ucm.es.
Notes
South-to-North Water Diversion Project (SNWDP).
For the agent-specific regressions, we instead use \(OW{ER}_{jkt}\), and the rest of the specification is adapted accordingly.
References
Ali MK, Klein KK (2014) Water use efficiency and productivity of the irrigation districts in Southern Alberta. Water Resour Manag 28:2751–2766. https://doi.org/10.1007/s11269-014-0634-y
Ananda J (2014) Evaluating the performance of urban water utilities: Robust nonparametric approach. J Water Resour Plan Manag 140(9):04014021. https://doi.org/10.1061/(ASCE)WR1943-54520000387
André, FJ, Buendía, A, Santos-Arteaga, FJ (2023) Efficient water use and reusing processes across Spanish regions: A circular data envelopment analysis with undesirable inputs. J Clean Prod 434:139929. https://doi.org/10.1016/jjclepro2023139929
Azad MA, Ancev T (2014) Measuring environmental efficiency of agricultural water use: A Luenberger environmental indicator. J Environ Manag 145:314–320. https://doi.org/10.1016/jjenvman201405037
Azad MA, Ancev T, Hernández-Sancho F (2015) Efficient water use for sustainable irrigation industry. Water Resour Manag 29:1683–1696. https://doi.org/10.1007/s11269-014-0904-8
Banker RD, Charnes A, Cooper WW (1984) Some models for estimating technical and scale inefficiencies in data envelopment analysis. Manag Sci 30:1078–1092. https://doi.org/10.1287/mnsc3091078
Berbel J, Esteban E (2019) Droughts as a catalyst for water policy change Analysis of Spain Australia (MDB) and California. Glob Environ Ch 58:101969. https://doi.org/10.1016/jgloenvcha2019101969
Bernabé-Crespo MB, Loáiciga H (2024) Managing potable water in southeastern spain, los angeles, and sydney: Transcontinental approaches to overcome water scarcity. Water Resour Manag. https://doi.org/10.1007/s11269-023-03721-8
Charnes A, Cooper WW, Rhodes E (1978) Measuring the efficiency of decision making units. Eur J Oper Res 2(6):429–444. https://doi.org/10.1016/0377-2217(78)90138-8
Cheng Z, Zhao Y, Song T, Cheng L, Wang W (2023) White elephant or golden goose? An assessment of middle route of the south-to-north water diversion project from the perspective of regional water use efficiency. Water Resour Manag 37(2):819–834. https://doi.org/10.1007/s11269-022-03405-9
Deng G, Li L, Song Y (2016) Provincial water use efficiency measurement and factor analysis in China: based on SBM-DEA model. Ecol Ind 69:12–18. https://doi.org/10.1016/jecolind201603052
García-Sánchez IM (2006) Efficiency measurement in Spanish local government: the case of municipal water services. Rev Pol Res 23(2):355–372. https://doi.org/10.1111/j1541-1338200600205x
Garrick DE, Hanemann M, Hepburn C (2020) Rethinking the economics of water: An assessment. Oxf Rev Econ Pol 36(1):1–23. https://doi.org/10.1093/oxrep/grz035
González-Gómez F, García-Rubio MA, Alcalá-Olid F, Ortega-Díaz MI (2013) Outsourcing and efficiency in the management of rural water services. Water resources management 27:731–747. https://doi.org/10.1007/s11269-012-0212-0
Guerrini A, Romano G, Campedelli B (2013) Economies of scale, scope, and density in the Italian water sector: a two-stage data envelopment analysis approach. Water Resour Manag 27:4559–4578. https://doi.org/10.1007/s11269-013-0426-9
Hernández-Chover V, Bellver-Domingo Á, Hernández-Sancho F (2018) Efficiency of wastewater treatment facilities: The influence of scale economies. J Environ Manag 228:77–84. https://doi.org/10.1016/jjenvman201809014
Hernández-Sancho F, Molinos-Senante M, Sala-Garrido R (2011) Energy efficiency in Spanish wastewater treatment plants: A non-radial DEA approach. Sci Total Environ 409(14):2693–2699. https://doi.org/10.1016/jscitotenv201104018
Hu JL, Wang SC, Yeh FY (2006) Total-factor water efficiency of regions in China. Res Pol 31:217–230. https://doi.org/10.1016/jresourpol200702001
Jiang Y et al (2024) Moving towards sustainable development in China's rural counties: Ecological efficiency evaluation based on DEA-Malmquist-Tobit model. J Clean Prod 141093. https://doi.org/10.1016/jjclepro2024141093
Jin W, Zhang HQ, Liu SS, Zhang HB (2019) Technological innovation, environmental regulation, and green total factor efficiency of industrial water resources. J Clean Prod 211:61–69. https://doi.org/10.1016/jjclepro201811172
Lannier AL, Porcher S (2014) Efficiency in the public and private French water utilities: prospects for benchmarking. App Econ 46(5):556–572. https://doi.org/10.1080/000368462013857002
Lewis HF, Sexton TR (2004) Data Envelopment Analysis with reverse inputs and outputs. J Prod Anal 21:113–132. https://doi.org/10.1023/B:PROD000001686869586b4
Li J, Ma XC (2015) Econometric analysis of industrial water use efficiency in China. Environ Devel Sustain 17:1209–1226. https://doi.org/10.1007/s10668-014-9601-2
Liang X, Li J, Guo G, Li S, Gong Q (2021) Evaluation for water resource system efficiency and influencing factors in western China: A two-stage network DEA-Tobit model. J Clean Prod 328:129674. https://doi.org/10.1016/jjclepro2021129674
Liu Y, Sun C, Xu S (2013) Eco-efficiency assessment of water systems in China. Water Resour Manag 27:4927–4939. https://doi.org/10.1007/s11269-013-0448-3
Ma D et al (2023) Spatiotemporal evolution and influencing factors of water resource green efficiency in the cities of the Yangtze River Economic Belt. Environ Sci Pollut Res 30(57):120963–120983. https://doi.org/10.1007/s11356-023-30876-z
Njiraini GW, Guthiga PM (2013) Are small-scale irrigators water use efficient? Evidence from Lake Naivasha Basin, Kenya. Environ Manag 52:1192–1201. https://doi.org/10.1007/s00267-013-0146-1
Pan J, Peng J, Yang X, Xuan S (2023) Does water rights trading promote resources utilisation efficiency and green growth? Evidence from China’s resources trading policy. Res Pol 86:104235. https://doi.org/10.1016/jresourpol2023104235
Qu G (2020) An evaluation method for water pollution treatment efficiency in coastal cities based on regional management. J Coast Res 110(SI):100–103. https://doi.org/10.2112/JCR-SI110-0241
Raju KS, Nagesh Kumar D (2013) Fuzzy data envelopment analysis for performance evaluation of an irrigation system. Irrig & Drain 62(2):170–180. https://doi.org/10.1002/ird1721
Sala-Garrido R, Mocholi-Arce M, Molinos-Senante M, Smyrnakis M, Maxiotis A (2023) Benchmarking the performance of productive units using cross-efficiency techniques: An empirical approach for water companies. Water Resour Manag 37:5459–5476. https://doi.org/10.1007/s11269-023-03614-w
Seiford LM, Zhu J (2002) Modeling undesirable factors in efficiency evaluation European. J Oper Res 142:16–20. https://doi.org/10.1016/S0377-2217(01)00293-4
Shuai S, Fan Z (2020) Modeling the role of environmental regulations in regional green economy efficiency of China: Empirical evidence from super efficiency DEA-Tobit model. J Environ Manag 261:110227. https://doi.org/10.1016/jjenvman2020110227
Song M, Wang R, Zeng X (2018) Water resources utilization efficiency and influence factors under environmental restrictions. J Clean Prod 184:611–621. https://doi.org/10.1016/jjclepro201802259
Veettil PC, Speelman S, Van Huylenbroeck G (2013) Estimating the impact of water pricing on water use efficiency in semi-arid cropping system: An application of probabilistically constrained nonparametric efficiency analysis. Water Resour Manag 27:55–73. https://doi.org/10.1007/s11269-012-0155-5
Wang S, Zhou Li, Wang H, Li X (2018) Water Use Efficiency and Its Influencing Factors in China: Based on the Data Envelopment Analysis (DEA)—Tobit Model. Water 10(7):832. https://doi.org/10.3390/w10070832
Xu R, Wang W (2020) Spatiotemporal Evolution and Impacts of Water Ecological Efficiency of Zhongyuan Urban Agglomeration from the Perspective of Social Network. J Coast Res 104(SI):54–57. https://doi.org/10.2112/JCR-SI104-0091
Yilmaz B, Yurdusev MA, Harmancioglu NB (2009) The assessment of irrigation efficiency in Buyuk Menderes Basin. Water Resour Manag 23:1081–1095. https://doi.org/10.1007/s11269-008-9316-y
Zhang F, Wang H, Xue HF (2020) China’s industrial green total factor water efficiency under the constraints of environment and resource. China Environ Sci 40(11):5079–5081
Zhou Y, Kong Y, Sha J, Wang H (2019) The role of industrial structure upgrades in eco-efficiency evolution: Spatial correlation and spillover effects. Sci Total Enviroon 687:1327–1336. https://doi.org/10.1016/jscitotenv201906182
Zschille M, Walter M (2012) The performance of German water utilities: a (semi)-parametric analysis. App Econ 44(29):3749–3764. https://doi.org/10.1080/000368462011581215
Acknowledgements
Dr. Francisco J. Santos-Arteaga is grateful for the support received from the María Zambrano contract from the Universidad Complutense de Madrid financed by the Ministerio de Universidades with funding from the European Union Next Generation program.
Funding
Open Access funding provided thanks to the CRUE-CSIC agreement with Springer Nature. This publication is part of the project PID2022-138754OB-I00, funded by MCIN/AEI/10.13039/501100011033/FEDER, EU.
Author information
Authors and Affiliations
Corresponding author
Additional information
Publisher's Note
Springer Nature remains neutral with regard to jurisdictional claims in published maps and institutional affiliations.
Appendix
Rights and permissions
Open Access This article is licensed under a Creative Commons Attribution 4.0 International License, which permits use, sharing, adaptation, distribution and reproduction in any medium or format, as long as you give appropriate credit to the original author(s) and the source, provide a link to the Creative Commons licence, and indicate if changes were made. The images or other third party material in this article are included in the article's Creative Commons licence, unless indicated otherwise in a credit line to the material. If material is not included in the article's Creative Commons licence and your intended use is not permitted by statutory regulation or exceeds the permitted use, you will need to obtain permission directly from the copyright holder. To view a copy of this licence, visit http://creativecommons.org/licenses/by/4.0/.
About this article
Cite this article
Buendía Hernández, A., André, F.J. & Santos-Arteaga, F.J. On the Evolution and Determinants of Water Efficiency in the Regions of Spain. Water Resour Manage (2024). https://doi.org/10.1007/s11269-024-03814-y
Received:
Accepted:
Published:
DOI: https://doi.org/10.1007/s11269-024-03814-y