Abstract
This study aimed to examine an assumption regarding whether generative artificial intelligence (GAI) tools can overcome the cognitive intensity that humans suffer when solving problems. We examine the performance of ChatGPT and GPT-4 on NAEP science assessments and compare their performance to students by cognitive demands of the items. Fifty-four 2019 NAEP science assessment tasks were coded by content experts using a two-dimensional cognitive load framework, including task cognitive complexity and dimensionality. ChatGPT and GPT-4 answered the questions individually and were scored using the scoring keys provided by NAEP. The analysis of the available data for this study was based on the average student ability scores for students who answered each item correctly and the percentage of students who responded to individual items. The results showed that both ChatGPT and GPT-4 consistently outperformed most students who answered each individual item in the NAEP science assessments. As the cognitive demand for NAEP science assessments increases, statistically higher average student ability scores are required to correctly address the questions. This pattern was observed for Grades 4, 8, and 12 students respectively. However, ChatGPT and GPT-4 were not statistically sensitive to the increase of cognitive demands of the tasks, except for Grade 4. As the first study focusing on comparing cutting-edge GAI and K-12 students in problem-solving in science, this finding implies the need for changes to educational objectives to prepare students with competence to work with GAI tools such as ChatGPT and GPT-4 in the future. Education ought to emphasize the cultivation of advanced cognitive skills rather than depending solely on tasks that demand cognitive intensity. This approach would foster critical thinking, analytical skills, and the application of knowledge in novel contexts among students. Furthermore, the findings suggest that researchers should innovate assessment practices by moving away from cognitive intensity tasks toward creativity and analytical skills to more efficiently avoid the negative effects of GAI on testing.
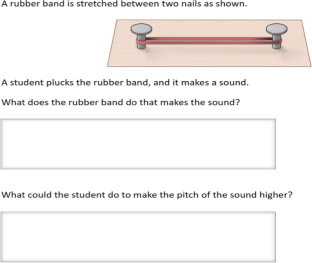



Similar content being viewed by others
Data Availability
Data are available from NEAP.
References
Adiguzel, T., Kaya, M. H., & Cansu, F. K. (2023). Revolutionizing education with AI: Exploring the transformative potential of ChatGPT. Contemporary Educational Technology, 15(3), ep429.
Aktay, S., Seçkin, G., & Uzunoğlu, D. (2023). ChatGPT in education. Türk Akademik Yayınlar Dergisi (TAY Journal), 7(2), 378–406.
Assaraf, N. (2022, December 8). OpenAI’s ChatGPT: Optimizing Language Models for Dialogue. cloudHQ. Retrieved May 10, 2023, from https://blog.cloudhq.net/openais-chatgpt-optimizing-language-models-for-dialogue/
Bang, Y., Cahyawijaya, S., Lee, N., Dai, W., Su, D., Wilie, B., et al. (2023). A Multitask, Multilingual, Multimodal Evaluation of ChatGPT on Reasoning, Hallucination, and Interactivity. https://doi.org/10.48550/arXiv.2302.04023
Behmke, D. A., & Atwood, C. H. (2013). Implementation and assessment of Cognitive Load Theory (CLT) based questions in an electronic homework and testing system. Chemistry Education Research and Practice, 14(3), 247–256. https://doi.org/10.1039/C3RP20153H
Bergen, K. J., Johnson, P. A., de Hoop, M. V., & Beroza, G. C. (2019). Machine learning for data-driven discovery in solid Earth geoscience. Science, 363(6433), eaau0323.
Bergner, Y., & von Davier, A. A. (2018). Process data in NAEP: Past, present, and future. Journal of Educational and Behavioral Statistics, 44(6), 706–732. https://doi.org/10.3102/1076998618784700
Bian, N., Han, X., Sun, L., Lin, H., Lu, Y., & He, B. (2023). ChatGPT is a knowledgeable but inexperienced solver: An investigation of commonsense problem in large language models. https://doi.org/10.48550/arXiv.2303.16421
Brüggemann, T., Ludewig, U., Lorenz, R., & McElvany, N. (2023). Effects of mode and medium in reading comprehension tests on cognitive load. Computers & Education, 192, 104649.
Cao, C., Ding, Z., Lee, G.-G., Jiao, J., Lin, J., & Zhai, X. (2023). Elucidating STEM concepts through generative AI: A multi-modal exploration of analogical reasoning. https://doi.org/10.48550/arXiv.2308.10454
Center for Standards, Assessment, and Accountability (CSAA) (2019). Cognitive loading in three-dimensional NGSS assessment: Knowledge, skills, and know-how. Retrieved June 12, 2023 from, https://csaa.wested.org/wp-content/uploads/2019/11/CSAI-Whitepaper_Cog-Load-3D-NGSS1.pdf
Daher, W., Diab, H., & Rayan, A. (2023). Artificial intelligence generative tools and conceptual knowledge in problem solving in chemistry. Information, 14(7), 409.
Estrella, S., Zakaryan, D., Olfos, R., & Espinoza, G. (2020). How teachers learn to maintain the cognitive demand of tasks through Lesson Study. Journal of Mathematics Teacher Education, 23, 293–310.
Feldon, D. F., Callan, G., Juth, S., & Jeong, S. (2019). Cognitive load as motivational cost. Educational Psychology Review, 31(2), 319–337. https://doi.org/10.1007/s10648-019-09464-6
Gerjets, P., Scheiter, K., & Cierniak, G. (2009). The scientific value of cognitive load theory: A research agenda based on the structuralist view of theories. Educational Psychology Review, 21(1), 43–54. https://doi.org/10.1007/s10648-008-9096-1
Gupta, U., & Zheng, R. Z. (2020). Cognitive load in solving mathematics problems: Validating the role of motivation and the interaction among prior knowledge, worked examples, and task difficulty. European Journal of STEM Education, 5(1), 5.
Hadie, S. N., & Yusoff, M. S. (2016). Assessing the validity of the cognitive load scale in a problem-based learning setting. Journal of Taibah University Medical Sciences, 11(3), 194–202.
Herdiska, A., & Zhai, X. (2023). Artificial intelligence-based scientific inquiry. In X. Zhai & J. Krajcik (Eds.), Uses of Artificial Intelligence in STEM Education (pp. 1–21). Oxford University Press.
Ignjatović, A., & Stevanović, L. (2023). Efficacy and limitations of ChatGPT as a biostatistical problem-solving tool in medical education in Serbia: A descriptive study. Journal of Educational Evaluation for Health Professions, 20, 28. https://doi.org/10.3352/jeehp.2023.20.28
Johnson, C. E., & Boon, H. J. (2023). Identifying and challenging the narrow cognitive demands of science textbooks. In: Thomas, G. P., & Boon, H. J. (Eds.), Challenges in Science Education. Palgrave Macmillan, Cham. https://doi.org/10.1007/978-3-031-18092-7_13
Jordan, M. I., & Mitchell, T. M. (2015). Machine learning: Trends, perspectives, and prospects. Science, 349(6245), 255–260. https://science.sciencemag.org/content/349/6245/255.long
Kung, T. H., Cheatham, M., Medenilla, A., Sillos, C., De Leon, L., Elepaño, C., Madriaga, M., Aggabao, R., Diaz-Candido, G., & Maningo, J. (2023). Performance of ChatGPT on USMLE: Potential for AI-assisted medical education using large language models. PLOS Digital Health, 2(2), e0000198.
Lagalante, M. C. (2023). High school science students’ cognitive load using virtual reality compared to traditional instruction (order No. 30638839). Available from ProQuest Dissertations & Theses A&I; ProQuest Dissertations & Theses Global; ProQuest Dissertations & Theses Global: The Humanities and Social Sciences Collection. (2861076861). https://www.proquest.com/dissertations-theses/high-school-science-students-cognitive-load-using/docview/2861076861/se-2
Latif, E., Mai, G., Nyaaba, M., Wu, X., Liu, N., Lu, G., Li, S., Liu, T., & Zhai, X. (2023). AGI: Artificial general intelligence for education. arXiv:2304.12479. https://doi.org/10.48550/arXiv.2304.12479
Lee, G.-G., & Zhai, X. (2023). NERIF: GPT-4V for automatic scoring of drawn models. https://doi.org/10.48550/arXiv.2311.12990
Li, P. H., Lee, H. Y., Cheng, Y. P., Starčič, A. I., Huang, Y. M. (2023). Solving the self-regulated learning problem: Exploring the performance of ChatGPT in Mathematics. In: Huang, YM., Rocha, T. (Eds.), Innovative technologies and learning. ICITL 2023. Lecture Notes in Computer Science (vol. 14099). Springer, Cham. https://doi.org/10.1007/978-3-031-40113-8_8
Lim, H., & Sireci, S. G. (2017). Linking TIMSS and NAEP assessments to evaluate international trends in achievement. Education Policy Analysis Archives, 25, 11. https://doi.org/10.14507/epaa.25.2682
McCormick, M. (2016). Exploring the cognitive demand and features of problem solving tasks in primary mathematics classrooms. Mathematics Education Research Group of Australasia.
Najafabadi, M. M., Villanustre, F., Khoshgoftaar, T. M., Seliya, N., Wald, R., & Muharemagic, E. (2015). Deep learning applications and challenges in big data analytics. Journal of Big Data, 2(1), 1–21.
National Assessment Governing Board. (2019). Science framework for the 2019 national assessment of educational progress. Retrieved June 12, 2023, from https://www.nagb.gov/content/dam/nagb/en/documents/publications/frameworks/science/2019-science-framework.pdf
NGSS Lead States. (2013). Next generation science standards: For states, by states. National Academies Press.
Nyaaba, M., Zhai, X. (2024). Generative AI professional development needs for teacher educators. Journal of AI, 8(1), 1–13. https://doi.org/10.61969/jai.1385915
OpenAI. (2022). ChatGPT: Optimizing Language Models for Dialogue. Retrieved June 14, 2023, from https://openai.com/blog/chatgpt/
OpenAI. (2023). GPT-4. Retrieved January 11, 2024, from https://openai.com/research/gpt-4
Orrù, G., Piarulli, A., Conversano, C., & Gemignani, A. (2023). Human-like problem-solving abilities in large language models using ChatGPT. Frontiers in artificial intelligence, 6. https://doi.org/10.3389/frai.2023.1199350
Paas, F., & Van Merriënboer, J. J. G. (2020). Cognitive-load theory: Methods to manage working memory load in the learning of complex tasks. Current Directions in Psychological Science, 29(4), 394–398. https://doi.org/10.1177/0963721420922183
Paas, F., Renkl, A., & Sweller, J. (2003). Cognitive load theory and instructional design: Recent developments. Educational Psychologist, 38(1), 1–4.
Park, S., Jang, J. Y., Chen, Y. C., & Jung, J. (2011). Is pedagogical content knowledge (PCK) necessary for reformed science teaching? Evidence from an Empirical Study Research in Science Education, 41(2), 245–260. https://doi.org/10.1007/s11165-009-9163-8
Pengelley, J., Whipp, P. R., & Rovis-Hermann, N. (2023). A testing load: Investigating test mode effects on test score, cognitive load and scratch paper use with secondary school students. Educational Psychology Review, 35(3), 67. https://doi.org/10.1007/s10648-023-09781-x
Prisacari, A. A., & Danielson, J. (2017). Computer-based versus paper-based testing: Investigating testing mode with cognitive load and scratch paper use. Computers in Human Behavior, 77, 1–10.
Rosenfeld, S. (2011). Common sense: A political history. Harvard University Press.
Seetharaman, R. (2023). Revolutionizing medical education: Can ChatGPT boost subjective learning and expression? Journal of Medical Systems, 47(1). https://doi.org/10.1007/s10916-023-01957-w
Sinha, R. K., Deb Roy, A., Kumar, N., & Mondal, H. (February 20, 2023). Applicability of ChatGPT in assisting to solve higher order problems in pathology. Cureus 15(2), e35237. https://doi.org/10.7759/cureus.35237
Stokel-Walker, C. (2022). AI bot ChatGPT writes smart essays - should professors worry? Nature. https://doi.org/10.1038/d41586-022-04397-7
Sweller, J. (2011). Cognitive load theory. In Psychology of learning and motivation (Vol. 55, pp. 37–76). Elsevier.
Tekkumru-Kisa, M., Stein, M. K., & Schunn, C. (2015). A framework for analyzing cognitive demand and content-practices integration: Task analysis guide in science. Journal of Research in Science Teaching, 52(5), 659–685.
The Nation’s Report Card. (2022). Question Tool. Retrieved May 13 from https://www.nationsreportcard.gov/nqt/searchquestions
Tugtekin, U., & Odabasi, H. F. (2022). Do interactive learning environments have an effect on learning outcomes, cognitive load and metacognitive judgments? Education and Information Technologies, 27(5), 7019–7058. https://doi.org/10.1007/s10639-022-10912-0
Wang, T., Li, M., Thummaphan, P., & Ruiz-Primo, M. A. (2017). The effect of sequential cues of item contexts in science assessment. International Journal of Testing, 17(4), 322–350. https://doi.org/10.1080/15305058.2017.1297818
Williams, A. E. (2023). Has OpenAI achieved artificial general intelligence in ChatGPT?. Artificial Intelligence and Applications. https://doi.org/10.47852/bonviewaia3202751
Zeng, F. (2023). Evaluating the problem solving abilities of ChatGPT. McKelvey School of Engineering Theses & Dissertations (vol. 849). https://openscholarship.wustl.edu/eng_etds/849
Zhai, X., & Wiebe, E. (2023). Technology-based innovative assessment. In C. J. Harris, E. Wiebe, S. Grover, & J. W. Pellegrino (Eds.), Classroom-based STEM assessment (pp. 99–125). Community for Advancing Discovery Research in Education.
Zhai, X., Yin, Y., Pellegrino, J. W., Haudek, K. C., & Shi, L. (2020). Applying machine learning in science assessment: A systematic review. Studies in Science Education, 56(1), 111–151.
Zhai, X., & Pellegrino, J. (2023). Large-scale assessment in science education. In N. G. Lederman, D. L. Zeidler, & J. S. Lederman (Eds.), Handbook of research on science education (Vol. III, pp. 1045–1098). Foutledge.
Zhai, X. (2022). ChatGPT user experience: Implications for education. Available at SSRN 4312418.
Zhai, X. (2023). ChatGPT and AI: The game changer for education. SSRN. https://ssrn.com/abstract=4389098
Acknowledgements
We are grateful to the team members Xinyu He, Yuxi Huang, and Cheng-Wen He.
Funding
This material is based upon work supported by the National Science Foundation (NSF) under Grant Nos. 2101104 and 2138854.
Author information
Authors and Affiliations
Corresponding author
Ethics declarations
Conflict of Interest
The authors declare that they have no conflict of interest.
Disclaimer
Any opinions, findings, and conclusions or recommendations expressed in this material are those of the author(s) and do not necessarily reflect the views of the NSF.
Additional information
Publisher's Note
Springer Nature remains neutral with regard to jurisdictional claims in published maps and institutional affiliations.
Rights and permissions
Springer Nature or its licensor (e.g. a society or other partner) holds exclusive rights to this article under a publishing agreement with the author(s) or other rightsholder(s); author self-archiving of the accepted manuscript version of this article is solely governed by the terms of such publishing agreement and applicable law.
About this article
Cite this article
Zhai, X., Nyaaba, M. & Ma, W. Can Generative AI and ChatGPT Outperform Humans on Cognitive-Demanding Problem-Solving Tasks in Science?. Sci & Educ (2024). https://doi.org/10.1007/s11191-024-00496-1
Accepted:
Published:
DOI: https://doi.org/10.1007/s11191-024-00496-1