Abstract
There is a raise in public awareness on environmental and health issues in recent years, therefore many municipalities changed their transport policy direction to become more sustainable, especially active mobility based. This study makes use of an activity-based demand model to simulate urban mobility and policies for sustainable transport modes in the Usti nad Labem district using an agent-based model simulator driven by a co-evolutionary algorithm. Two policy scenarios were created by considering the transport literature and analyzing the characteristics and behaviors of citizens as well as the properties of the study area. Three scenarios—the actual situation, a cycleway-infrastructure case, and a bus priority case—were simulated for the study area with MATSim software. Both policy scenarios resulted in a decrease in car usage, with a higher drop seen in the cycleway-infrastructure scenario. 9.11% higher public transport ridership and 2.45% more of public transport modal share are observed in the bus priority compared to the actual situation, however the car-related emissions did not decrease. 6.36% more of cycling modal share was also noticed in the cycleway-infrastructure scenario which, the transport modal shift is enhanced by 2.6 more times than in the bus priority scenario. Car driving hours were significantly reduced in the cycleway scenario (5535 h less in a day) where 445.3 tons of car-related CO2 emissions would be saved annually, therefore environmental benefits of cycling modal share increase in the study area is undoubtable in long-term.
Similar content being viewed by others
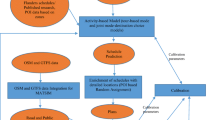
Avoid common mistakes on your manuscript.
Introduction
Mathematical transport models
Multidimensional co-evolutionary dynamics occur between the society and contextual technical factors, creating a socio-technical backdrop for any city in time marked by differences in the built environment and user choices (Dingil et al. 2021), making urban systems inherently complex (Pribyl et al. 2021). This complexity poses challenges in transport planning as the dynamic nature makes it hard to predict the outcomes of transport-related projects. Consequently, transport planning necessitates continuous updates for sustainable outcomes. Mathematical transportation models, originating from the traditional four-step model (FSM), have been pivotal in guiding transport planning, allowing us to predict and compare the outcomes of transport proposals, urban traffic conditions, and sustainability impacts. The FSM model encompasses trip generation, trip distribution, mode choice, and route choice. The initial two sub-models use descriptive statistics for the reproduction of steady patterns connected to the utilization of land-use types (e.g., home-school trips), while the latter two use discrete choice models and traffic assignment models (e.g. utilization of routes per trip as traffic flows), respectively, in order to reflect user mode choices given the region’s transport infrastructure performance (Le Pira, Inturri, and Ignaccolo 2021). However, newer approaches have emerged offering greater realism for urban mobility scenarios based on long-term investments, such as land use and transport interaction (LUTI) models accounting for factors like land use, transport connection, and accessibility (Geurs and van Wee 2004; Hensher and Button 2008; Geurs et al. 2010) and activity-based travel demand (ABTD) models focusing on understanding the link between spatiotemporal patterns of facilities and activity-based trip planning (Pribyl and Goulias 2005; Hensher and Button 2008). This study utilizes the ABTD modeling approach, as outlined in the methodology section, to assess transport-related policies. ABTD approach is based on mimicking the daily activity patterns of citizens in a study area while considering spatiotemporal constraints. The behavior of each person is modeled separately based on accessibility, locational, temporal, and interpersonal interdependencies (Pereira et al. 2022). ABTD models utilize the precise coordinates of each agent inside the zone-based approach. The assignment of precise location and facility types for each agent’s activity are the main characteristic of the model; thus, the land-use effect is considered with a bottom-up approach. To the best of our knowledge, ABTD models are the most comprehensive means of providing realism to mobility scenarios but they have large data requirements, as detailed in the data subsection.
Simulation approach
There are many software programs available for travel demand and traffic modelling. Ferrara et al. (2018) provide a comprehensive study on this topic. Simulation tools can be classified into three groups based on the representation level of urban traffic: microscopic, mesoscopic, and macroscopic. The main difference between them is the level of detail of traffic variables and their interactions in the model. The macroscopic scale uses a compressible fluid modeled by fluid mechanics equations (Diallo et al. 2021) without considering the events occurring at the agent level, while the microscopic scale focuses on these agents discretely. The mesoscopic scale is a hybrid of these two scales in which the behavior of individual traffic elements (vehicles or agents) stems from macroscopic properties. The main advantage of the mesoscopic approach is the reflection of both small and large-scale features with relatively rapid processing. Given the importance of long-term transport planning in promoting sustainable infrastructure, we selected MATSim in this study which was used to reflect the mesoscopic scale of the study area for testing transport policy scenarios, as presented in the methodology section. Uniting a coevolutionary algorithm and a robust transport model base (ABM framework), MATSim offers rapid processing capabilities and realistic simulation environment. This makes it highly suitable for assessing potential future scenarios and understanding the impacts of travel behavior change in realistic manner (Ciari et al. 2016). The MATSim is generally preferred in literature for examining long-term transport policies for large scenarios. MATSim has been employed in recent studies for a variety of transport modeling purposes including the scenario of Croatia’s nationwide transport energy demand (Novosel et al. 2015), analyzing travel demand in Barcelona and Zurich to inform cordon toll policy decisions (Freitas et al. 2017; Bassolas et al. 2019), examining mode-choice behavior in Jakarta (Ilahi et al. 2019), assessing bike-sharing demand in the San Francisco Bay Area to evaluate first/last-mile solutions for public transit (Balac and Hörl 2021), capturing travel demand fluctuations in New York City during the COVID pandemic (Wang et al. 2021), and modeling of dynamic demand for an automated taxi service in Zurich (Hörl et al. 2021). The realistic performance of these scenarios is greatly influenced by the quality and content of the demand model, as noted in Pereira et al. (2022), which will be elaborated upon in Sect. “Data” for our specific case.
Aim and organization
Evidence-based policy analysis greatly increase the chances of transport planning agreements (Lovelace et al. 2020) and simulation of policy scenarios in realistic manner is an important analysis tool to provide a pre-evidence of failure or success of a transport project. This study used an activity-based demand model and an agent-based model simulator, MATSim software as noted above, in order to analyze two transport policy suggestions against a base scenario created for the Usti nad Labem district by taking the recent transport literature and the study area conditions into consideration. This article presents the first-ever simulation of transportation scenarios within a daily mobility framework for a city model in the Czech Republic, employing MATSim. This study also distinguishes itself from the majority that use MATSim for transport modeling by testing transport infrastructure scenarios which is not present at the study area, which is shedding light on implementation of different transport infrastructures on MATSim for scholars. Furthermore, to the best of our knowledge, this study pioneers the implementation of a city-wide cycleway trial in MATSim. The research aims to reflect the potential outcomes of transport policy scenarios within the study area by using MATSim, with a particular emphasis on sustainable transportation modes, as elaborated in the subsequent section. The transport system and policy scenarios for the study area are presented below, and then the simulation results are presented and evaluated. Section “Study area and scenario background” presents details about the study area, the actual transport system by providing preliminary insights into the shortcomings and operations, and the backdrop for the scenarios. Section “Methodology” focuses on the methodology applied to define the study framework, the activity-based demand model, and infrastructure requirements. Section “Results and discussions” demonstrates and evaluates the simulation results for the study area, while the Sect. “Conclusions” summarizes study conclusions.
Study area and scenario background
Study area and transport system
The urban transport system of the Ústí nad Labem district, located in the northwest part of the Czech Republic, is studied here. 116,916 inhabitants reside in the city area and its surrounding 23 municipalities, together forming a catchment area. The city area is a regional center with administrative and educational institutions, and it represents an important transport hub connecting many Czech and international cities through a complex railway network (Pereira et al. 2022). The city area is also important transit and destination locations for both passenger and freight road transport. The road network of Usti nad Labem’s district and city area is visualized in Fig. 1. The district has 1902 km of bidirectional road network, of which 412 km are located in larger development areas with high traffic flows, as calculated using the tool OSMnx (Boeing 2017). There are two important international road routes passing through the city area, the E442 (east–west direction) and E55 (south–north direction) with I and II class roads. The route between the Czech Republic and Germany generates the majority of the passing-through traffic in the city area (CIVITAS 2012a).
The map of the transit network of the city area is presented in Fig. 2, which highlights public transport lines by their actual names and stops by points while travel routes are presented by colored roads. A major part of the public transport network in the inner-city area is served by trolleybuses, while buses are used primarily to reach suburban zones. The daily operation performance of the public transportation system in the study area is 42700 km ride for a working day, 2900 km of which happen in the city center (CIVITAS 2012b). The connection between the city and surrounding municipalities is mainly supplied by regional buses, meanwhile commuter trains supply some of these connections. The network of buses and trolleybuses within the city area shares roads with cars and other motorized vehicles, except for some restricted streets (e.g. Hrnčířská and Revoluční streets), where the accessibility of motorized vehicles is limited to residents (CIVITAS 2012b). The majority of intersections controlled by traffic signals are used by public transport vehicles in the city, buses and trolleybuses do not have traffic light priorities or bus-private lanes on roads. As a result, significant public transport service delays occur in the city area, especially during peak hours. For example, public transport vehicles must stop at least twice at the busy Předmostí intersection, controlled by traffic lights (CIVITAS 2012a).
The city area does not have a distinct cycling infrastructure beyond the Elbe cycling route, which contains parts of the EuroVelo European cycling network passing through the city from the south (connecting the Czech cities of Ústí nad Labem, Litoměřice, and Prague) and, to the north, connecting Ústí nad Labem to Germany (Děčín, Bad Schandau, and Dresden) (Usti nad Labem Municipality 2019). The availability of segregated bike paths along the Elbe Route is useful for commuters working in areas surrounding the city to some extent, though there is no dedicated route connecting the Elbe Route with the city itself. Nevertheless, there are also biking centers and biking camps along this cycling route.
The transport mode utilization in the study area is visualized in Fig. 3 based on CIVITAS report data (2012a), which combines various trip-based measurements on local roads and urban traffic, passing-through traffic data, and the movements of inhabitants in the Ústí nad Labem city area. On average, personal cars are used for 85% of day-long urban traffic, followed by light trucks (7%), public transport (6%), freight vehicles (1%), and motorcycles (1%). Urban trips in the city center are, in terms of modal split: 35% public transport, 24% personal vehicles, 16% PTC and personal vehicles combined, and 25% walking. However, a recent work shows a deeper view of travel behavior change in Czech towns (Matowicki et al. 2022). According to a survey study introduced by Bartuska et al. (2022), there were travel behavior changes in commuting patterns in the Czech Republic during the COVID-19 pandemic, notably a 10% decrease in public transportation usage. Additionally, a significant increase in bike flows on cycling routes was observed according to sensors monitored by the municipality of Prague, while volumes increased from 5% in 2019 to 40% in 2020 (GOLEMIO 2022), Moreover, data from 2021 to 2022 indicate the pandemic modal shift may be permanent.
Background of policy scenarios
The highest level of global CO2 emissions in history, 36.3 billion tons, was recorded in 2021 (International Energy Agency 2022). Global warming is reaching a critical level, in large part due to the transportation sector. The contribution of car-dependent transport systems to the global warming has been highlighted in many studies over the last ten years (Dingil et al. 2021), but significant changes to such systems have not yet been made. Observations of incremental growth in the use of active transport modes (International Energy Agency 2020) and air quality improvements (United Nations 2021) on public health during the Covid-19 pandemic helped raise public awareness of these issues, with some studies observing about the public becoming more sensitive about health and climate issues (Dingil and Esztergár-Kiss 2021). In light of environmental and health considerations, along with insights from municipal reports and EU projects focusing on the sustainability of the study area, as referenced in the previous and this subsection, we have selected two policy implementation scenarios. The first one focused on implementing the bike transport infrastructure and the second on implementing a bus priority policy, were simulated in this study for the Usti nad Labem district.
Regarding biking, a significant transport modal shift towards cycling was observed worldwide in the first year of the Covid-19 pandemic, referred to as the so-called “bike boom” (Bernhard 2020). Greater public awareness and transport mode shifts towards walking and cycling during the first year of the Covid-19 pandemic were also noticed by policy and decision makers around the world; therefore, many municipalities changed their transport policies to become more active-based (Laker 2021).
As the first policy approach, this study proposes a city-wide cycling infrastructure plan, as described in Sect. “Methodology”. To the best of our knowledge, it presents the first city-wide cycleway implementation trail in MATSim for a city without a pre-existing biking infrastructure. The municipality of Ústí nad Labem planned a segregated cycling network in its city master plan funded by the CIVITAS project (CIVITAS 2013), but this plan was never implemented. A major prerequisite to support cycling is the successful implementation of a separated cycling infrastructure, demonstrated in many studies (e.g., Dill and Carr 2003; Pucher and Buehler 2008). Taking a cycling infrastructure seriously can overcome perceived limitations such as bad weather conditions and hilliness, as demonstrated in several cities. For example, Amsterdam and Copenhagen are rainy, but the successful implementation of cycling infrastructures and policies have resulted in their becoming the bicycle capitals of the world (Ligtermoet 2010). San Francisco, the second hilliest city in the world and the hilliest in the US, has succeeded in becoming one of the most biking-friendly cities in the US after the successful cycling infrastructure and policy implementation (Collins et al. 2020). Regarding improving public transport options and bus/trolleybus options in particular, while there was a significant decrease in public transport usage worldwide as a result of the Covid-19 pandemic demonstrated in many recent works (Dingil and Esztergár-Kiss 2021; Eisenmann et al. 2021; Molloy et al. 2021), the importance of public transport systems for a sustainable urban mobility system is frequently emphasized (Miller et al. 2016), so gaining the trust of passengers who shifted to motorized vehicles during the pandemic can be achieved by increasing the comfort, sanitation, and speed of public transport systems (Dingil and Esztergár-Kiss 2021). The Usti nad Labem city has a network of public transportation consisting primarily of trolleybuses for the inner city, as mentioned above. Although trolleybuses are favorable, in terms of CO2 outputs, being powered by electricity, they can be slow; for example, the average speed of the Sofia (Bulgaria) trolleybus system during 2020 was 15.7 km/h (Sofia Municipality 2020). As the aging of the current population in the study area increases (Pereira et al. 2022), the system’s comfort and speed are more important than ever.
Thus, the second policy proposal made as part of this study includes the implementation of a bus priority policy along trolleybus routes, also described in Sect. “Methodology”. By “bus priority policy,” we mean providing a lane only for public transport at certain times of a day such as during morning, noon, and evening peaks in order to make public transport faster. Such bus priority policies have been shown to bring important benefits to the urban mobility systems, especially growing semi-dense cities. For example, after the implementation of bus priority policy in Toulouse (France), a 52% reduction in the average waiting times of buses at traffic lights was observed, and a similar implementation in Malmö (Sweden) reducing the waiting time for buses from 10 to 7.5 min, expected resulting in a 4% punctuality increase across the public transport system (CIVITAS 2015).
Methodology
Framework
In this section, we present the simulation framework, demand model, and infrastructure requirements of transport scenarios created for the Ústí nad Labem district. MATsim version 13.0 was used in this study to simulate urban transport scenarios for the Ústí nad Labem district. MATSim uses agent-based modules to optimize daily activity plans for simulated individuals, considering transport infrastructure and locational attributes, with agents independently making decisions to maximize personal benefits in iterative simulations framed by co-evolutionary principle, differing from traditional evolutionary algorithms (Horni et al. 2016).
Figure 4 provides the holistic MATSim framework used in this study, which each simulation presents a loop of execution, scoring, and re-planning. Also, note that the full population of study area was simulated here. The main MATSim inputs are: (1) a population file (a demand model containing daily activity and trip plan characteristics for all citizens), (2) a multimodal network file (containing the street segments, intersections, etc.), (3) a public transport schedule file (containing stops, transfer points, routes, and trips of transit system), (4) vehicle files, for both regular and transit vehicles (containing the characteristics of the vehicles utilized in the model), (5) a facilities file (containing points of interest in the study area), (6) emission files (providing information needed to calculate CO2 exhaust emissions in detail based on HBEFA, the Handbook Emission Factors for Road Transport). Additional data processes were also conducted to provide the required networks for the policy scenarios; cycleway infrastructure (P1), bus priority implementation (P2), and 3D presentation of the network, explained in detail in the next subsections.
MATSim employs queue traffic flow models, QSim and JDEQSim, for simulating traffic dynamics, particularly beneficial for large-scale scenarios over intricate car-following models. Using QSim, a time-step based model, the transport network was adapted to the needed format as explained in the next subsection, with road network links defined by storage and flow capacities. The network capacity factors were set to 0.92 (i.e. 92% of the road capacity is simulated) in this study to reflect the presence of about 8% of freight transport and transit vehicles in the simulation environment based on the road network occupancy according to transport mode from CIVITAS report data (2012a). This fine tuning was applied because of freight and transit-pass data deprivation, as in other studies (Freitas et al. 2017; Bassolas et al. 2019), to better-scale network capacity in order to reflect more realistic conditions in our demand model. Nevertheless, it may still lead to minor capacity errors for cars on the major roads. For better presentation of non-stationary traffic flows, we preferred the kinematic wave model over the spatial queue model (program default). This preference lies on the unrealistic behavior of the default configuration when presenting the gaps between vehicles and estimating interlink traffic congestion (Agarwal et al. 2016). MATSim has two main routing options, teleportation and “on network.” Simulated vehicles can take advantage of existing road networks (public transport, car, or bike), configured to simulate the transport network more realistically. A-Star Landmarks algorithm was selected as car routing as it is more time-efficient for larger scenarios. A seepage model (Agarwal and Lämmel 2016) was utilized for simulating cyclists, allowing them to navigate through a vehicle queue for the base scenario. The Raptor algorithm (Delling et al. 2015; Rieser et al. 2018) was used as transit router instead of the default MATSim transit router, which is Dijkstra-based. The Raptor algorithm has a round-based routing approach and has more advanced features and a faster computational speed than the default transit router. The ride mode was not physically simulated because it would create simulated car overuse; only the car network and instant vehicle speeds were used and simulated as being on the network. Only the walking mode was configured as a teleportation mode, using a walking distance factor as default of 1.3, and average walking speed of 1.11 m/s. Walking was also configurated as access and egress transport modes. As standard MATSim value, a Euclidian distance of 1000 m was assigned to each agent as the maximum walking distance in order to access public transport stop; first, transit stops in a 500 m radius were searched, and if there is no transit stop option existed, the search radius was extended by 500 m.
Daily activity utilities were calculated in a scoring step based on the first plan of each agent’s memory using a utility function as described in Charypar and Nagel (2005) and Nagel et al. (2016), shown in Eqs. (1)–(4). The default utility function was extended and integrated with an external biking algorithm in order to calculate bike scoring more realistically (Ziemke et al. 2018). The external bicycle function is demonstrated in Eq. (5), which additionally considered infrastructure type, riding comfort, and road gradient for bicycle utility calculation. The glossary of all formulas used in this study is presented in Table 1.
The utility function was used to maximize the utility of activity performance and to minimize travel disutility during a day. The function considers traveling, early departure for an activity, late arrival for an activity, waiting duration until open time of an activity location, waiting public transport, transfer, and too short activity duration (time press) as the disutility of a daily plan (negative utility), while only actual activity duration is considered as a positive utility of a daily plan. The default program marginal utility parameters of an activity leg were used. A logarithmic form was applied while calculating the utility of actual activity duration, as shown in the Eq. (3). Travel disutility was calculated based on the characteristics of travel times and travel cost for performing activities during a day. Since a specific survey study needed to estimate the required travel utility parameter values has not yet been performed for the study area, we used the parameter values from the calibrated simulation of the city of VelbertFootnote 1 because of sociodemographic and transport infrastructure similarity to our study area. Utility parameter values of simulations used in this study are presented in Table 2. In the context of car travel and its marginal utility of time, it’s worth noting that it is assumed to be negligible in our case, primarily due to the typically low levels of traffic congestion in small cities. Also, given the context of our study area, a small city where a significant majority of residents possess a public transport pass card and considering we did not delve into bike-sharing systems, the influence of a distance-based price for these modes is neglegted. The maximum bike speed was set to 4.16 m/s, while the marginal parameters of bike utility function were used as default, presented in Table 2. The related bicycle data implementations are presented in the next subsections.
A final re-planning step allows each agent to provide modifications to their plans either by selecting another plan from the set of alternative plans in their memories or by making an innovation in a plan selected from their memory. The maximum plan memory size of agents was set to five in this study, the optimal value. If maximum plan memory size of any agent was exceeded, the plans with the worst utility score were erased from the memory pool in order to prevent memory overload during the simulations. The re-planning step uses a discrete choice modelling approach resembling multinomial logit models, which is expressed in Eqs. (6), (7) and (8) (Flötteröd and Kickhöfer 2016, 2016).
MATSim has several innovation strategies available to optimize scenarios by using mutation feature of co-evolutionary algorithms, such as travel route choice, transport mode choice, and departure time choice. The innovations of travel route, activity end time, and transport mode were utilized for the scenarios presented here. The time mutation range, the frequency of random activity end time shift, was set as two hours. Because each agent learns and gains experience during each iteration, re-planning strategies were evolved accordingly. The innovations are drawn from the distribution of PA(CA|UA), while the selections are drawn from the distribution of PA(i|CA) as seen in Eqs. (6), (7) and (8). MATSim makes several selection strategies possible, such as choosing a plan with the highest score in memory of each agent, keeping an agent’s last plan, choosing a plan randomly from the agent’s memory, or choosing a plan based on multinomial logit model (MNL) from the agent’s memory. We tweaked the exponential beta parameter “ChangeExpBeta” for the all scenarios (i.e., cycleway infrastructure and bus priority) because it is more realistic from a discrete choice theoretical perspective, and convergence to a steady state is provided more smoothly than with other selection strategies (Lämmel et al. 2010). The exponential beta parameter utilizes the exponential value of the difference between the actual plan score and possible plan scores in the memory of each agent as the probability of assigning a new plan, as shown in Eq. (9).
Each re-planning strategy had an assigned weight in the configuration step of MATSim before starting execution. The probability of being chosen for the selection strategy and innovation strategy were assigned as 85 and 15% respectively (5% of route change, 5% of activity end time change, 5% of transport mode change) for each scenario. Because this tuning level provided a fair accord between exploration and exploitation (Lämmel et al. 2010). Riders (car passengers) were excluded from the mode innovation as in other studies, because riding is a dependent mode. The definition of car as a chain mode and driving license availability of agents were configurated with the transport mode innovation strategy as the constraint. Through this approach, we emphasize that citizens without cars cannot opt for driving, and if a journey begins with an owned car, it will conclude with it.
The MATSim loop process repeats iteratively until the average score of executed plans for all agents reaches the steady state, with each cycle score recorded to be analyzed at the end of a simulation. Because the preferred number of iterations varies in MATSim scenarios2, depending on data size and quality, we set it to 300 here with considering our demand model and the fraction of iteration to disable transport mode innovation was set at 80% of iterations in scenarios to avoid overestimation as used in other MATSim scenarios.Footnote 2 Additionally, an external emission module (Kickhöfer et al. 2013; Kickhöfer 2016) was integrated with the simulation environment for the calculation of car-related CO2 exhaust emissions after each event. The algorithm calculates exhaust emissions based on the “Handbook on Emission Factors for Road Transport” (HBEFA); the emission process is explained in next subsections.
Data
Activity-based demand model
A MATSim loop requires initial demand model, which requires a synthetic population data for a study area containing the activity chains and travel characteristics for each agent. As highlighted in the introduction, the quality and content of the demand model play a pivotal role in delivering a robust analysis. A robust version of this type of demand model can be generated by utilizing household travel surveys combined with census data sources. For this, an activity-based demand model (ABM) presenting the 2016 population conditions from a recent study (Pereira et al. 2022) for the study area was used. The model was imported in MATSim format from the Github repository of this study.Footnote 3 This demand model provided the most integrated approach presenting disaggregated characteristics and activities at an individual level currently available in the Czech Republic up to date, and it is prepared using both census data and data from two household travel surveys (HTS). This data model is created by extending Eqasim framework (Hörl and Balac 2021) focusing on synthetic demand model generation in realistic manner. The Eqasim framework is extended for estimating inbound and outbound trips by utilizing synthetic gates as hubs and ensuring a more accurate match of travel destinations and activity locations by assigning capacities to each identified facility in the study area. The model includes inbound and outbound travel demands for the city of Ústí nad Labem and its 23 surrounding municipalities. Facilities, in the model, were comprehensively considered in the demand assignment process, so that the capacity for each facility in the study area, the expected number of visitors to each facility, and the number of residents in each building were used to assign trips for each agent while creating transport demand for the study area. This is noteworthy because the robustness of the activity-based scenarios in this study were based on the accurate assignment of the locations, including fields of activity, capacities, expected number of visitors, and residents. These extensions helped to reduce implausible reflections of the distribution of travel and activity characteristics in household travel surveys, thus it increased realism for the traffic conditions of the study area. This approach was not studied in this fashion before. The demand model results of the base year were compared with the relevant transport statistics of the study area primarily focusing on the distribution of scenario features such as sociodemographic attributes, mainly age groups and gender; travel features, including average travel times and primary modes of transport; travel-distance attributes based on activity type; and activity-chain characteristics (e.g., the distribution of h-w–h trips) while a high level of match is observed. Several highlights providing a comparative view on the distribution of daily travel distance, daily trip count, and activity chain characteristics of the city and entire area for the ABM and HTS outputs are visualized in Figs. 5 and 6, please see Pereira et al. 2022 for a more detailed comparative view. The only limitation of the present model is that the freight and transit transport data linked to the study area were not included due to no relevant datasets. Nevertheless, this situation was overcome by utilizing road network occupancy data, as mentioned in the previous subsection. The availability of driving license was retrieved from the model and utilized as the constraint for transport mode strategy.
Infrastructure
The road network for this study area was imported from OpenStreetMap (OSM)Footnote 4; however, the road network had to be converted to MATSim format in order to estimate free speeds, lane numbers, storage capacities, and transport modes for the road links. MATSim also requires vehicle and transit vehicle files listing all vehicles and their features, in which for the non-transit vehicles, this file was created manually. Additionally, transit information for the study area was originally obtained from the Ústí nad Labem municipality in the Czech National Information System of Timetables format and later transformed into the General Transit Feed Specification (GTFS) format. The final GTFS file contained information about transit stop locations, transit vehicle routes, a calendar of the transit system, expected arrival and departure times of the transit vehicles at transit stations, and transfer points. Pt2matsim is a Java package (Poletti 2016) was used to convert the OSM data to a projected network in MATSim format. The tool also uses capacity assumptions when any essential data is missing in the original OSM network. Moreover, the Pt2matsim package maps the transit information to the MATSim schedule format. The tool also assumes the missing routes for some transit vehicles, thus providing route connectivity. Pt2matsim was also utilized to create a transit vehicle file from the GTFS file. The facility dataset containing the information about buildings and other points of interest for the study area was imported from the Github repository of the demand model.Footnote 5
For the cycleway-infrastructure scenario, the planned and segregated cycling routes were provided by the Ústí nad Labem municipality, which consisted of several shape files with different timelines. A sample of the cycleway plan provided is shown in Fig. 7. Importing such a cycling infrastructure into MATSim format was challenging, thus some adjustments were required to merge the route files and to convert them into MATSim format. First, a net editor was required to adjust and clean the network. SUMO’s Netedit tool (Lopez et al. 2018) was used. After that, SUMO’s Netconvert tool converted the network from SUMO’s format to MATsim’s format.
The used bicycle module requires road type, cycleway type, surface type, and road grade of data for each link. Surface type is related to the comfort of agents, which is assigned as default for all links except cycleway lanes (in where we defined as asphalt). The presence of a cycling infrastructure is related to the comfort and safety of agents; if a segregated cycleway is present on a road, a cycleway type is assigned as lane to consider by the utility function. If there is not a cycleway type on a link, bicycle utilization is considered by the utility function based on road types. In our study, network roads were categorized based on OSM data.
Street grades were calculated based on the height of nodes/intersections (z coordinate) extracted from Google Maps, as explained below. OSMnx is a Python package (Boeing 2017) that was utilized following the methodology presented in (Dingil et al. 2018). The road transport network for the study area was imported in networkX graph format by keeping the OSM keys and values as features, then, a z coordinate attribute (height) was assigned to each OSM node of the graph by utilizing the Google Elevation API. A Python algorithm was then created to match MATSim network road nodes with graph nodes in order to assign z values for the MATSim network based on OSM node IDs. In case of ID mismatch, an average elevation value for the study area of 250 m was assigned for this node, also calculated with OSMnx. The normalized average grade of the roads (0–1) in the study area was mapped with a sequential plot (see Fig. 8), in which a monotonal increase of light value through the plasma colormap is based on road grade values. As a result, the final network is defined by including city-wide cycleway network and bike users who were not allowed to use car roads when cycleway is present. Also, note that e-bikes are not considered in this study.
For the bus-priority scenario, the transit schedule file was adjusted to be 25% faster for the trolleybus system and 5% faster for buses as in the Malmö bus priority case (CIVITAS 2015). This was achieved by adjusting the timetables and by simulating buses and trolleybuses passing through current trolleybus routes on restricted lanes, in addition to reflect this situation for the car users, storage capacities of roads having these lanes diminished by half and free-speed of these roads are readjusted based on the Pt2matsim’s default capacity values and their equivalent free-speeds.
To calculate car-related CO2 emissions of each MATSim event, an external emission module, based on Kickhöfer (2016), was utilized. The algorithm applies a mapping process to the MATSim network in order to assign HBEFA road types and consequently features to each link. The module requires two main inputs: cold and hot emission files. These files contain information such as emission factors, HBEFA road types, traffic situations, distances, average gradients, and free speeds in order to calculate emissions for each event. For the (average) warm emission file, the emission factor (CO2 emissions in g/km) depends on car type distribution for a study area. To calculate the weighted warm emission factor for our study area, a vehicle register dataset from the European Environmental Agency containing registered car distributions and weighted CO2 emissions for registered cars for the Czech Republic between 2010 and 2021 was used (EEA 2022). The cars registered in Czech Republic before 2010 were imported from the European Central Bank’s statistical data warehouse (ECB 2022), and the International Council on Clean Transportation’s average weighted warm CO2 emission factor for the EU was used (ICCT 2011) for each corresponding year. As a result, the average warm emission factor (CO2 emissions in g/km) was calculated by taking 1995–2021 car registrations and their distribution into account to provide module input. For the cold emission file, the CO2 emissions in g/km are based on distance, and they were taken from the emission module repository prepared as part of the HBEFA data.
Results and discussions
In this section, simulation results are presented and evaluated. In all simulations, a 64–bit computer running with Windows 10, 16 GB of RAM, and i7-118G7 processor was used. The average iteration speed of simulations was around 40 s which is high considering the complexity of simulation environment; therefore, the benefit of the co-evolutionary algorithm in terms of computational cost was observed in this study. Plan score patterns of the scenarios throughout the iterative simulations show a similar pattern which is visualized for the base scenario in Fig. 9. Given that no sudden drop or implausible patterns were observed for the average executed scores throughout scenario iterations, which is a consistency indicator for the original demand model. The average executed score closes the gap with the average best score, and finally a steady state is reached for all scenario populations.
The evolution of the departure, in route, arrival distributions of car, bicycle, and transit users throughout the time of the day for the first and last iterations of each scenario is presented in the Figs. 11, 12 and 13 in the Appendix, demonstrating the visualization of how the optimization results by co-evolutionary algorithm for transport modes, travel routes, and departure time changes influence the distribution of the travel demand. The distribution of departure, arrival, and in route number of agents over time evolved distinctly. As seen after evolving with iterative simulations, agents in route for each transport mode completed their day time trips in the 300th iteration. Departure and arrival numbers for agents were also considerably improved by the last iteration. Focusing on the last iteration results, the highest car usage in morning peak times was observed in the base scenario, while the lowest car usage in the evening peak was seen in the cycleway. The highest transit usage during morning scenario and evening peak times was observed for the bus priority scenario. These ridership increases are an indicator of the possibility of commute mode changes if transport mode scenarios would be adopted. The cycling pattern look different than other scenarios in the cycleway scenario. For example, the cycling flows are considerably higher around the morning peak than afternoon stage. The established patterns look more stable and having clear morning and evening rush hour peaks, similar to the patterns observed for the dominant transport modes (car and transit), which indicates the adoption of cycling for commuting.
The transport mode share evolution of scenarios throughout iterative simulations is shown in Fig. 10. Transport mode usage reached a steady state after 80% of iterations. The highest car usage was observed for the base scenario, while transit usage and bike usage were the highest for the bus-priority scenario and cycleway-infrastructure scenario, respectively. Table 3 presents the transport mode choice differences between the base scenario and policy scenarios in percentages and person counts (absolute values). Table 3 indicates that 885 and 1096 agents stopped using cars in the cycleway-infrastructure and bus-priority scenarios compared to base case, respectively. Precisely, it was observed 6.36% more of cycling modal share in the cycleway-infrastructure scenario and 2.45% more of transit modal share in the bus-priority scenario. 2071 agents shifted their transport modes towards public transport in the bus-priority scenario, and 5371 agents shifted their transport mode towards bicycle in the cycling-infrastructure scenario. In the cycleway-infrastructure scenario, transport modal shift is enhanced by 2.6 times more than in the bus-priority scenario.
Compared to a similar case, a study (dell’Olio et al. 2014) analyzed transport users and their willingness to change from traditional modes to cycling by using a mixed logit model in order to estimate the bicycle user potential in urban mode share in Santander, a city in the northern Spain. Santander has a similar population size of Usti nad Labem. As well, both cities have hilly zones, therefore the expectation of bike usage is not high and cycling mode share is not even pronounced. The results of the study were surprising for a city with the characteristics of Santander (dell’Olio et al. 2014), the availability of infrastructure was one of the most important variables for potential bike users and the city has potential of increasing mode share from its current 0.30% up to 5.52% after the successful implementation of infrastructure. In here, we found a similar level of mode share increase (6.36% more) after cycling infrastructure policy, which supports the previously mentioned finding. Another study (Litman 2016) estimates up to 9% bus ridership increase after a typical bus lane policy as a result of 15% travel time decrease based on the elasticity calculations of bus trips with respect to travel time conducted in Paulley et al. (2006). In the present study, the results show 9.11% increase of public transport usage in bus priority scenario, which is consistent with the previous estimations.
The Table 4 demonstrates the distribution of daily passenger hours traveled per mode of simulation scenarios. Public transport waiting time is reduced throughout iterations completed for all scenarios. The smallest volume of car driving hours traveled is observed in the cycleway-infrastructure scenario where car driving hours traveled is reduced by 5535 h per day. The car-related emissions per day of each scenario is shown in Table 5. Daily total average CO2 emissions are the lowest in the cycleway scenario, with a value of 25.97 tones CO2, which can be understood by examining Table 5. Cold exhaust emissions are produced when the vehicle warm-up phase, and which is the highest in the base scenario while the highest car share is also seen in the base scenario, which explains this. Hot exhaust emissions are released during vehicle operation when normal operating temperatures are reached in an engine. Hot exhaust emissions for this study were slightly higher in the bus-priority scenario than in the base case. A slightly higher of time traveled with car in the bus-priority scenario was also noticed in Table 5 which causes this.
As car road capacity dropped, vehicle speeds became lower than usual, especially in the central zones, causing the increase of transit ridership providing high-speed access, which has benefits to economically disadvantaged groups (Litman 2016). Nevertheless, the present study did not find any environmental benefits of the bus lane policy in term of the reduction of car-related CO2 emissions.
Environmental and health issues in recent years are pronounced more as a result of noticeable global warming effect and the COVID-19 pandemic. The environmental benefit of cycling modal share increase for the study area is undoubtable in long-term. As average, 445.3tons of car-related CO2 emissions would be saved annually in case of cycleway scenario. Cycling has also health economic benefits in the long term. A study (Deenihan and Caulfield 2014) estimates the impact of cycling on population health in a region of Ireland based on the increase of cycling modal share to 2.5%, 5% and 10% by using World Health Organization’s (WHO) Health Economic Assessment Tool (HEAT). In the case of 5% modal share increase (similar output with the present work), the study shows that the current value of the total benefits accumulated over 10 years would be equal to €64,534,000 with the benefit-to-cost ratio 5.38:1.
Effective bike policies such as providing city-wide cycling infrastructure as in this study reduce traffic congestion and emissions but also promote public health. For policymakers, the crucial point lies in intertwining safe infrastructure like protected bike lanes with focusing long-term plans as stated in literature (e.g., Dill and Carr 2003; Pucher and Buehler 2008). On the other hand, increasing comfort and speed of the public transport services would increase ridership as shown in this study, nevertheless the environmental performance of urban mobility would increase only if there is a clear modal shift from cars to public transport, which was not achieved for the bus priority scenario. Also, while our policy formulations in this study were based on municipal reports and literature relevant to the city’s size and context, there are other micro-mobility options like scooters, as well as public transport policies like bike-and-ride, that could be explored if sufficient data is available. That would help to drawn more explicit outputs and these avenues present promising future research directions. Although this study aimed to simulate a realistic environment, there is potential for improvement to reflect user choices if more data becomes available. For instance, in-depth surveys focused on travel utility could provide valuable insights (Dingil and Esztergár-Kiss 2023). This would enable us to expand the utility function with additional parameters based on community perceptions in the study area, leading to more refined results. Furthermore, the current study does not simulate the interactions of urban mobility at a microscopic level, which might result in minor differences.
Conclusions
Traditional travel demand modeling approaches such as the four-step model are well suited for modeling aggregated behavior within a zone of interest. However, if we want to understand the behavior of individual citizens and thus be able to model the impact of certain policies (in our case, supporting cycling or public transport use), a more comprehensive approach is needed. Therefore, this study used an activity-based demand model (ABTD). ABTD model offers the possibility to define the daily plans of each person based on participation in certain activities and required trips (the sequence of activities and trips in time and space). Using a co-evolutionary algorithm-based software in this study, activities and trips evolve and reschedule, taking into constrains and consideration of interactions with other citizens (agents) to get closer to the real situation.
This study presented a transport policy comparison for the Ústí nad Labem district in the Czech Republic using an agent-based model simulator. Three scenarios (the actual situation, a city-wide cycleway infrastructure case, and a bus-priority case) are simulated using MATSim software. To the best of our knowledge, this study presented the first city-wide cycleway implementation trail and analysis in MATSim for a city without a pre-existing biking infrastructure. Significant improvements in agents’ travel route, activity timing, and transport mode choices after the co-evolutionary optimization were demonstrated for each scenario compared to their first iteration results. The policy scenario results were evaluated and compared to the actual situation. Both scenarios resulted in a decrease in car usage, with a higher drop seen in the cycleway infrastructure scenario.
9.11% higher public transport ridership and 2.45% more of public transport modal share were observed in the bus priority case compared to the actual situation. Providing high-speed access, especially in the central zones, has benefits to economically disadvantaged groups. Nevertheless, the present study did not find any environmental benefits of the bus lane policy in terms of the reduction of car-related CO2 emissions. 6.36% more of cycling modal share was noticed in the cycleway infrastructure scenario which, the transport modal shift is enhanced by 2.6 more times than in the bus priority scenario. Car driving hours were significantly reduced in the cycleway scenario (5535 h less in a day), where 445.3 tons of car-related CO2 emissions would be saved annually, therefore the environmental benefits of cycling modal share increase in the study area is undoubtable in long-term.
This study also illuminates the adaptability of MATSim by demonstrating its ability to model varied transport infrastructures, serving as a valuable resource for researchers and underscoring the software’s flexibility. In recent years, micro mobility sharing systems has gained attraction in Czech Republic. Consequently, future research would aim to explore the impact of micro-mobility infrastructure and sharing systems on the transport sustainability of a Czech city within the MATSim framework. This exploration would provide important environmental and operational insights into micro mobility systems in Czech cities.
References
Agarwal, A., Lämmel, G.: Modeling seepage behavior of smaller vehicles in mixed traffic conditions using an agent based simulation. Transp. Dev. Econ. 2, 8 (2016). https://doi.org/10.1007/s40890-016-0014-9
Agarwal, A., Lämmel, G., Nagel, K.: Modelling backward travelling holes in mixed traffic conditions using an agent based simulation. In: Knoop, V., Daamen, W. (eds.) Traffic and Granular Flow’15. Springer, Cham (2016)
Balac, M., Hörl, S.: Simulation of intermodal shared mobility in the San Francisco Bay Area using MATSim. In: 2021 IEEE International Intelligent Transportation Systems Conference (ITSC), Indianapolis, IN, USA, pp. 3278–3283 (2021)
Bartuska, L., Stopka, O., Hanzl, J., Sedivy, J., Rybicka, I.: Changes in transport behaviour of the Czech population caused by state of emergency. Transp. Probl. (2022). https://doi.org/10.20858/tp.2022.17.1.09
Bassolas, A., Ramasco, J.J., Herranz, R., Cantú-Ros, O.G.: Mobile phone records to feed activity-based travel demand models: MATSim for studying a cordon toll policy in Barcelona. Transp. Res. Part A Policy Pract. 121, 56–74 (2019). https://doi.org/10.1016/j.tra.2018.12.024
Bernhard, A.: The great bicycle boom of 2020, BBC News. https://www.bbc.com/future/bespoke/made-on-earth/the-great-bicycle-boom-of-2020.html#:~:text=Covid%2D19%20brought%20about%20a,%2Dcalled%20%E2%80%9Cbike%20boom%E2%80%9D (2020)
Boeing, G.: OSMnx: new methods for acquiring, constructing, analyzing, and visualizing complex street networks. Comput. Environ. Urban Syst. 65, 126–139 (2017). https://doi.org/10.1016/j.compenvurbsys.2017.05.004
Charypar, D., Nagel, K.: Generating complete all-day activity plans with genetic algorithms. Transportation 32, 369–397 (2005). https://doi.org/10.1007/s11116-004-8287-y
Ciari, F., Balac, M., Axhausen, K.W.: Modeling carsharing with the agent-based simulation MATSim: state of the art, applications, and future developments. Transp. Res. Rec. 2564(1), 14–20 (2016). https://doi.org/10.3141/2564-02
City-Vitality-Sustainability (CIVITAS): Measure 60-cycle transport improvements. (2013)
City-Vitality-Sustainability (CIVITAS): SUTP development in Ústí nad Labem, Report. https://civitas.eu/sites/default/files/documents/Archimedes_deliverable_11_12.pdf (2012a)
City-Vitality-Sustainability (CIVITAS): Feasibility study of access control in Ústí nad Labem, Report. https://www.usti-nad-labem.cz/files/civitas/R27.1-Feasibility-study-of-access-control-in-UL.pdf (2012b)
CIVITAS: Intelligent transport systems and traffic management in urban areas, EC-policy notes-4. https://civitas.eu/content/civitas-policy-note-intelligent-transport-systems-and-traffic-management-urban-areas-0 (2015)
Collins, A.J., Jordan, C.A., Robinson, R.M., et al.: Exploring good cycling cities using multivariate statistics. Environ. Syst. Decis. 40, 526–543 (2020). https://doi.org/10.1007/s10669-019-09753-z
Deenihan, G., Caulfield, B.: Estimating the health economic benefits of cycling. J. Transp. Health 1(2), 141–149 (2014). https://doi.org/10.1016/j.jth.2014.02.001
Delling, D., Pajor, T., Werneck, R.F.: Round-based public transit routing. Transp. Sci. 49, 591–604 (2015). https://doi.org/10.1287/trsc.2014.0534
dell’Olio, L., Ibeas, A., Bordagaray, M., Ortúzar, J.D.D.: Modeling the effects of pro bicycle infrastructure and policies toward sustainable urban mobility. J. Urban Plan. Dev. 140, 04014001 (2014). https://doi.org/10.1061/(ASCE)UP.1943-5444.0000190
Diallo, A.O., Lozenguez, G., Doniec, A., Mandiau, R.: Comparative evaluation of road traffic simulators based on modeler’s specifications: an application to intermodal mobility behaviors. In: Proceedings of the ICAART 2021—13th International Conference on Agents and Artificial Intelligence, Vol. 1, pp. 265–272 (2021)
Dill, J., Carr, T.: Bicycle commuting and facilities in major U.S. cities: if you build them, commuters will use them. Transp. Res. Rec. 1828, 116–123 (2003). https://doi.org/10.3141/1828-14
Dingil, A.E., Schweizer, J., Rupi, F., Stasiskiene, Z.: Road network extraction with OSMNx and SUMOPy. EPiC Ser. Eng. 2, 111–117 (2018). https://doi.org/10.29007/t7pk
Dingil, A.E., Esztergár-Kiss, D.: The influence of the Covid-19 pandemic on mobility patterns: the first wave’s results. Transp. Lett. 13(5–6), 434–446 (2021). https://doi.org/10.1080/19427867.2021.1901011
Dingil, A.E., Rupi, F., Esztergár-Kiss, D.: An integrative review of socio-technical factors influencing travel decision-making and urban transport performance. Sustainability 2021(13), 10158 (2021). https://doi.org/10.3390/su131810158
Dingil, A.E., Esztergár-Kiss, D.: An activity-based travel personalization tool driven by genetic algorithm. J. Adv. Transp. 2023, 6678628 (2023). https://doi.org/10.1155/2023/6678628
Eisenmann, C., Nobis, C., Kolarova, V., Lenz, B., Winkler, C.: Transport mode use during the COVID-19 lockdown period in Germany: the car became more important, public transport lost ground. Transp. Policy 103, 60–67 (2021). https://doi.org/10.1016/j.tranpol.2021.01.012
European Central Bank (ECB): Statistical data warehouse: new passenger car registration. https://sdw.ecb.europa.eu/home.do (2022)
European Environment Agency (EEA): Monitoring of CO2 emissions from passenger cars-regulation (EU) 2019/631. https://www.eea.europa.eu/data-and-maps/data#c0=5&c11=&c5=all&b_start=0 (2022)
Ferrara, A., Sacone, S., Siri, S.: Microscopic and mesoscopic traffic models. In: Freeway Traffic Modelling and Control Advances in Industrial Control. Springer, Cham (2018)
Flötteröd, G., Kickhöfer, B.: Choice models in MATSim. In: Horni, A., Nagel, K., Axhausen, K.W. (eds.) The Multi-Agent Transport Simulation MATSim, pp. 337–346. Ubiquity Press, London (2016)
Geurs, K., van Wee, B.: Accessibility evaluation of land-use and transport strategies: review and research directions. J. Transp. Geogr. 12(2), 127–140 (2004). https://doi.org/10.1016/j.jtrangeo.2003.10.005
Geurs, K., Zondag, B., de Jong, G., de Bok, M.: Accessibility appraisal of land-use/transport policy strategies: more than just adding up travel-time savings. Transp. Res. Part D: Transp. Environ. 15(7), 382–393 (2010). https://doi.org/10.1016/j.trd.2010.04.006
GOLEMIO: Bicycle transport in Prague. https://golemio.cz/data/cyklodoprava#section-2 (2022)
Hensher, D.A., Button, K.J.: Handbook of Transport Modelling, 2nd edn. Emerald Group Publishing Limited (2008)
Horni, A., Nagel, K., Axhausen, K.W. (eds.): The Multi-Agent Transport Simulation MATSim. Ubiquity Press, London (2016). https://doi.org/10.5334/baw
Hörl, S., Balac, M.: Introducing the eqasim pipeline: from raw data to agent-based transport simulation. Proc. Comput. Sci. 184, 712–719 (2021). https://doi.org/10.1016/j.procs.2021.03.089
Hörl, S., Becker, F., Axhausen, K.W.: Simulation of price, customer behaviour and system impact for a cost-covering automated taxi system in Zurich. Transp. Res. 123, 102974 (2021). https://doi.org/10.1016/j.trc.2021.102974
Ilahi, A., Balac, M., Li, A., KayW, A.: The first agent-based model of greater Jakarta integrated with a mode-choice model. Proc. Comput. Sci. 151, 272–278 (2019). https://doi.org/10.1016/j.procs.2019.04.039
International Council on Clean Transportation (ICCT): The importance of mandatory standards: CO2 performance standards in the European Union. https://theicct.org/the-importance-of-mandatory-standards/ (2011)
International Energy Agency (IEA): Changes in transport behaviour during the Covid-19 crisis, IEA, Paris. https://www.iea.org/articles/changes-in-transport-behaviour-during-the-covid-19-crisis (2020)
International Energy Agency (IEA): Global CO2 emissions rebounded to their highest level in history in 2021, Press release, IEA, Paris https://www.iea.org/news/global-co2-emissions-rebounded-to-their-highest-level-in-history-in-2021 (2022)
Kickhöfer, B.: Emission modeling. In: Horni, A., Nagel, K., Axhausen, K.W. (eds.) ¨ The Multi-Agent Transport Simulation MATSim, pp. 247–252. Ubiquity Press, London (2016)
Kickhöfer, B., Hülsmann, F., Gerike, R., Nagel, K.: Rising car user costs: comparing aggregated and geo-spatial impacts on travel demand and air pollutant emissions. In: Vanoutrive, T., Verhetsel, A. (eds.) Smart Transport Networks, pp. 180–207. Edward Elgar Publishing (2013)
Laemmel, G., Grether, D., Nagel, K.: The representation and implementation of time-dependent inundation in large-scale microscopic evacuation simulations. Transport. Res. C Emerg. Tech. 18(1), 84–98 (2010). https://doi.org/10.1016/j.trc.2009.04.020
Laker, L.: Europe doubles down on cycling in post-Covid recovery plans, The Guardian. https://www.theguardian.com/lifeandstyle/2021/mar/12/europe-cycling-post-covid-recovery-plans (2021)
Le Pira, M., Inturri, G., Ignaccolo, M.: Modeling and simulation for transport planning. In: Vickerman, R. (ed.) International Encyclopedia of Transportation, pp. 184–190. Elsevier (2021)
Ligtermoet, D: The bicycle capitals of the world: Amsterdam and Copenhagen, Fietsberaad, June 2010, Publication number 7a. https://fietsberaad.nl/getmedia/92e655d5-7cbc-4ea7-a0d0-5b9cfdaf9bae/Fietsberaadpublication-7a-The-bicycle-capitals-of-the-world-Amsterdam-and-Copenhagen.pdf.aspx?ext=.pdf (2010)
Litman, T: When are bus lanes warranted? Considering economic efficiency, social equity and strategic planning goals. http://www.vtpi.org/blw.pdf (2016)
Lopez, P.A. et al.: Microscopic traffic simulation using SUMO. 2018 21st International Conference on Intelligent Transportation Systems (ITSC), pp. 2575–2582 (2018)
Lovelace, R., Parkin, J., Cohen, T.: Open access transport models: a leverage point in sustainable transport planning. Transp. Policy 97, 47–54 (2020). https://doi.org/10.1016/j.tranpol.2020.06.015
Matowicki, M., Pecherkova, P., Pribyl, O.: The potential of changing habitual car user travel modes: the case of Czech towns. Int. J. Transp. Sci. Technol. (2022). https://doi.org/10.1016/j.ijtst.2022.11.001
Meyer de Freitas, L., Schuemperlin, O., Balac, M., Ciari, F.: Equity effects of congestion charges: an exploratory analysis with MATSim. Transp. Res. Rec. 2670(1), 75–82 (2017). https://doi.org/10.3141/2670-10
Miller, P., de Barros, A.G., Kattan, L., et al.: Public transportation and sustainability: a review. KSCE J. Civ. Eng. 20, 1076–1083 (2016). https://doi.org/10.1007/s12205-016-0705-0
Molloy, J., Schatzmann, T., Schoeman, B., Tchervenkov, C., Hintermann, B., Axhausen, K.W.: Observed impacts of the Covid-19 first wave on travel behaviour in Switzerland based on a large GPS panel. Transp. Policy 104, 43–51 (2021). https://doi.org/10.1016/j.tranpol.2021.01.009
Nagel, K., Kickhöfer, B., Horni, A., Charypar, D.: A closer look at scoring. In: Horni, A., Nagel, K., Axhausen, K.W. (eds.) The Multi-Agent Transport Simulation MATSim, pp. 23–34. Ubiquity Press, London (2016)
Novosel, T., Perković, L., Ban, M., et al.: Agent based modelling and energy planning—utilization of MATSim for transport energy demand modelling. Energy 92, 466–475 (2015). https://doi.org/10.1016/j.energy.2015.05.091
Paulley, N., et al.: The demand for public transport: the effects of fares, quality of service, income and car ownership. Transp. Policy 13(4), 295–306 (2006). https://doi.org/10.1016/j.tranpol.2005.12.004
Pereira, A.M., Dingil, A.E., Přibyl, O., Myška, V., Vorel, J., Kříž, M.: An advanced travel demand synthesis process for creating a MATSim activity model: the case of Ústí nad Labem. Appl. Sci. 12, 10032 (2022). https://doi.org/10.3390/app121910032
Poletti, F.: Public transit mapping on multi-modal networks in MATSim, Master Thesis, IVT, ETH Zurich, Zurich. https://ethz.ch/content/dam/ethz/special-interest/baug/ivt/ivt-dam/publications/students/501-600/sa530.pdf (2016)
Přibyl, O., Goulias, K.G.: Simulation of daily activity patterns incorporating interactions within households: algorithm overview and performance. Transp. Res. Rec. 1926(1), 135–141 (2005). https://doi.org/10.1177/0361198105192600116
Přibyl, O., Přibyl, P., Svítek, M.: Interdisciplinary urban tunnel control within smart cities. Appl. Sci. 11, 10950 (2021). https://doi.org/10.3390/app112210950
Pucher, J., Buehler, R.: Making cycling irresistible: lessons from the Netherlands, Denmark, and Germany. Transp. Rev. 28, 495–528 (2008). https://doi.org/10.1080/01441640701806612
Rieser, M., Métrailler, D., Lieberherr, J.: Adding realism and efficiency to public transportation in MATSim. In: 18th Swiss Transport Research Conference, Monte Verità/ Ascona, 16–18 May 2018. https://strc.ch/2018/Metrailler_Lieberherr.pdf (2018)
Sofia Municipality: Sofiaplan transport infographic. https://sofiaplan.bg/wp-content/uploads/2021/02/Sofiaplan-Infographics-Transport-A4.pdf (2020)
United Nations: Air quality improvements from COVID lockdowns confirmed. Report. https://news.un.org/en/story/2021/09/1099092 (2021)
Ústí nad Labem Municipality: Cycling in Ústí nad Labem. https://www.usti-nad-labem.cz/files/en/tourists/top-tips-trips/cykloturistika-en-plakat.pdf (2019)
Wang, D., He, B.Y., Gao, J., Chow, J.Y., Ozbay, K., Iyer, S.: Impact of covid-19 behavioral inertia on reopening strategies for New York City transit. Int. J. Transp. Sci. Technol. 10(2), 197–211 (2021). https://doi.org/10.1016/j.ijtst.2021.01.003
Ziemke, D., Metzler, S., Nagel, K.: Bicycle traffic and its interaction with motorized traffic in an agent-based transport simulation framework. Futur. Gener. Comput. Syst. (2018). https://doi.org/10.1016/j.future.2018.11.005
Acknowledgements
This study is a result of the research performed in the “Smart City–Smart Region–Smart Community” (Project No. CZ.02.1.01/0.0/0.0/17 048/0007435) financed by the “Research, Development and Education” Czech Operational Programme for the implementation of the European Social Fund (ESF) and the European Regional Development Fund (ERDF).
Author information
Authors and Affiliations
Contributions
Contributions: study conception and design: AED; investigation: AED; methodology: AED; software: AED and AMP; analysis and interpretation of results: AED; visualization: AED; draft manuscript preparation: AED; review and editing, AED, AMP, OP, JV. All authors approved the final version of the manuscript.
Corresponding author
Ethics declarations
Conflict of interest
The authors have no conflicts of interest to declare.
Additional information
Publisher's Note
Springer Nature remains neutral with regard to jurisdictional claims in published maps and institutional affiliations.
Appendix
Appendix
Rights and permissions
Open Access This article is licensed under a Creative Commons Attribution 4.0 International License, which permits use, sharing, adaptation, distribution and reproduction in any medium or format, as long as you give appropriate credit to the original author(s) and the source, provide a link to the Creative Commons licence, and indicate if changes were made. The images or other third party material in this article are included in the article's Creative Commons licence, unless indicated otherwise in a credit line to the material. If material is not included in the article's Creative Commons licence and your intended use is not permitted by statutory regulation or exceeds the permitted use, you will need to obtain permission directly from the copyright holder. To view a copy of this licence, visit http://creativecommons.org/licenses/by/4.0/.
About this article
Cite this article
Dingil, A.E., Pereira, A.M., Přibyl, O. et al. Evidence-based transport policy analysis driven by agent-based simulation: the case of mobility in Ústí nad Labem. Transportation (2023). https://doi.org/10.1007/s11116-023-10453-6
Accepted:
Published:
DOI: https://doi.org/10.1007/s11116-023-10453-6